10:30 |
0746. |
Introduction

Simon Warfield
|
10:42 |
0747.
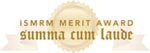 |
Fiber Orientation Mapping
using Gradient Echo MRI: R2* mapping vs Frequency
Difference Mapping 
Samuel James Wharton1, and Richard Bowtell1
1Sir Peter Mansfield Magnetic Resonance
Centre, School of Physics and Astronomy, University of
Nottingham, Nottingham, United Kingdom
Recent work has shown that magnitude images acquired
using gradient echo (GE) techniques at high field are
sensitive to the fiber-orientation in white matter.
Here, we show that frequency difference mapping (FDM)
based on acquisition of multi-echo GE data allows the
strong fiber-orientation contrast in phase images to be
exploited in producing high resolution, 3D
fiber-orientation maps. This approach was tested on
post-mortem brain samples by comparing the resulting
orientation maps to DTI data and fiber-orientation maps
generated from simultaneously acquired R2* maps. The
results suggest that FDM at high field may be used for
high resolution fiber-orientation mapping.
|
10:54 |
0748.
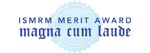 |
Multi-Channel Hybrid-Aided
Registration of Multiple Spaces (mCHARM): Diffusion and
Structural Image Co-Registration 
Frederick William Damen1,2, Yi Sui1,3,
and Xiaohong Joe Zhou1,4
1Center for MR Research, University of
Illinois at Chicago, Chicago, IL, United States, 2Department
of Biomedical Engineering, Georgia Institute of
Technology, Atlanta, GA, United States, 3Department
of Bioengineering, University of Illinois at Chicago,
Chicago, IL, United States, 4Departments
of Radiology, Neurosurgery, and Bioengineering,
University of Illinois at Chicago, Chicago, IL, United
States
Many diffusion MRI applications require co-registering a
diffusion image based on EPI acquisitions to a
high-resolution anatomical image; however, the contrast
difference between diffusion and anatomical images poses
a problem. We report a novel method, coined as
multi-channel hybrid-aided registration of multiple
spaces (mCHARM), to co-reregister diffusion images to
T1-weighted images using a synthesized diffusion
multi-channel hybrid (DMCH) image that mimics the
contrast of a T1-weighted image. Our results from ten
human volunteers have demonstrated that mCHARM improves
the registration accuracy and produces reliable FA
skeleton mask for tract-based spatial statistics
analysis.
|
11:06 |
0749. |
Registration of DCE-MRI
using Robust Data Decomposition 
Valentin Hamy1, Andrew Melbourne2,
Benjamin Trémoulhéac2, Shonit Punwani1,
and David Atkinson1
1Centre for Medical Imaging, UCL, London,
United Kingdom, 2Centre
for Medical Image Computing, UCL, London, United Kingdom
Dynamic Contrast Enhanced (DCE) MRI is a technique used
in oncology to extract quantitative information about
tumours. However, when it comes to image organs in the
thorax or the abdomen, several types of motion (e.g.
patient’s breathing, heartbeat and bowel peristalsis)
can lead to errors in the estimation of that
quantitative information. The study we present proposes
a new technique aimed at compensating
deformations/displacements due to motion in DCE images
acquired using a repeat breath-hold protocol or a free
breathing protocol.
|
11:18 |
0750. |
AN AUTOMATED INTENSITY
CORRECTION, REGISTRATION AND VOLUME STITCHING ALGORITHM FOR
RECONSTRUCTION OF THE WHOLE SPINE FROM 7 TESLA MR DATA 
Oleh Dzyubachyk1, Boudewijn Lelieveldt1,2,
Jorik Blaas1, Monique Reijnierse1,
Andrew Webb1, and Rob van der Geest1
1Department of Radiology, Leiden University
Medical Center, Leiden, Netherlands, 2Mediamatics
Department, Delft University of Technology, Delft,
Netherlands
Initial feasibility studies have shown the promise of 7T
MRI for studying spine disorders. However, diagnosis
based on such data is compromised by the presence of
high intensity inhomogeneities and the need to analyze
several separately acquired image stacks. In practice,
both intensity inhomogeneity correction and volume
stitching must be performed manually. Here we present
our novel fully automated algorithm for reconstructing a
whole spine volume from a set of image stacks acquired
on a 7T MR scanner.
|
11:30 |
0751. |
Validation of Fully
Automatic Adipose Tissue Segmentation and Volume
Quantification 
Bryan T Addeman1, Melanie Beaton2,
Robert A Hegele3, Abraam S Soliman3,4,
Curtis N Wiens5, and Charlies A McKenzie1,5
1Department of Medical Biophysics, University
of Western Ontario, London, ON, Canada, 2Department
of Medicine, University of Western Ontario, London, ON,
Canada,3The Robarts Research Institute,
London, ON, Canada, 4Biomedical
Engineering, University of Western Ontario, London, ON,
Canada, 5Department
of Physics, University of Western Ontario, London, ON,
Canada
The distribution of adipose tissue is associated with
the long-term development of type 2 diabetes and
cardiovascular disease. Most adipose tissue volume
quantification techniques require manual input, are
susceptible to human error, and are time consuming. We
propose a novel automated process for the quantification
and segmentation of Total Adipose Tissue, Subcutaneous
Adipose Tissue, and Intra-Abdominal Adipose Tissue using
quantitative fat fraction maps. Segmentation is robust
and requires no prior knowledge or complex machine
learning. Results show that automated fat volume
measurements are similar to manual segmentation
techniques and can be calculated very rapidly.
|
11:42 |
0752. |
Computer-Aided Detection
of Metastatic Brain Tumors: Comparison between MP-RAGE and
Black-Blood Imaging 
Seungwook Yang1, Eung Yeop Kim2,
Min-Oh Kim1, Yoonho Nam1, Jaeseok
Park3, and Dong-Hyun Kim1
1Electrical and Electronic Engineering,
Yonsei University, Sinchon-dong, Seoul, Korea, Republic
of, 2Radiology
and Research Institute of Radiological Science, Yonsei
University College of Medicine, Seoul, Korea, Republic
of, 3Department
of Brain and Cognitive Engineering, Korea University,
Seoul, Korea, Republic of
The purpose of this study was to develop a
computer-aided detection (CAD) system for brain
metastases detection from magnetic resonance (MR) black
blood images and to assess the applicability of MR
black-blood imaging to CAD. Twenty-six patients with
brain metastases of various sizes were imaged with a
contrast-enhanced, three dimensional, whole brain MR
black blood pulse sequence. The CAD system uses 3D
template matching which measures normalized
cross-correlation coefficient (NCCC) to generate
possible metastases candidates from the patient data.
Various image features were extracted from each
candidate, then principal component analysis (PCA) was
performed to determine dominant features. Artificial
neural network (ANN) training and testing scheme were
incorporated for classification.
|
11:54 |
0753.
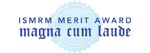 |
Atlas-free Brain Tissue
Segmentation Using a Single T1-weighted MRI Acquisition 
Tobias Kober1, Alexis Roche1,2,
Oscar Esteban3,4, Subrahmanyam Gorthi4,
Delphine Ribes5, Meritxell Bach-Cuadra2,4,
Reto Meuli2, and Gunnar Krueger1,2
1Advanced Clinical Imaging Technology,
Siemens Healthcare Sector IM&WS S, Lausanne,
Switzerland, 2Centre
Hospitalier Universitaire Vaudois and University of
Lausanne, Switzerland, 3Biomedical
Image Technology (BIT), Universidad Politécnica de
Madrid, Madrid, Spain, 4Signal
Processing Laboratory (LTS5), École Polytechnique
Fédérale de Lausanne, Lausanne, Switzerland, 5ARTORG
Center for Computer Aided Surgery, University of Bern,
Switzerland
Typical brain tissue segmentation algorithms use prior
knowledge in the form of pre-segmented templates, i.e.
atlases. This can lead to erroneous segmentation results
if the actual image differs too much from the template,
e.g. due to a too big age difference or a disease. In
this work, the homogenous T1-contrast of the MP2RAGE
sequence is combined with a Dixon water/fat imaging
approach. It is shown that a single MP2RAGE-Dixon
acquisition provides enough information for reproducible
atlas-free brain tissue segmentation.
|
12:06 |
0754. |
Automated quality control
in MR-based brain morphometry 
Bénédicte Maréchal1, Tobias Kober1,
Tom Hilbert2, Delphine Ribes3,
Nicolas Chevrey4, Alexis Roche1,
Jean-Philippe Thiran5, Reto Meuli4,
and Gunnar Krueger1
1Advanced Clinical Imaging Technology,
Siemens Healthcare Sector - CIBM, Renens, Switzerland, 2Universität
Heidelberg, Germany, 3ARTORG
Center for Computer Aided Surgery, Univ. of Bern,
Switzerland, 4Centre
Hospitalier Universitaire Vaudois and Univ. of Lausanne, 5Signal
Processing Laboratory (LTS5) Ecole Polytechnique
Fédérale de Lausanne
Normal aging and a wide range of neurologic,
inflammatory or psychiatric diseases lead to changes in
the brain tissue over time. In the interest of
diagnosis, prognosis and treatment monitoring, it is
highly desirable to have robust tools that reliably
measure brain morphometry. We explore the ability of an
automated MR image quality assessment technique to
predict the accuracy of subsequent algorithms for brain
quantitative analysis. The approach proofs to be a very
promising candidate to objectively assess quality prior
to any post-processing in order to attribute tissue
changes to a potential pathology rather than to image
degradation.
|
12:18 |
0755. |
Are two samples of
parametric images statistically different? Novel significane
tests on samples of density estimates, with application to
ventilation-to-perfusion mapping of the lung in COPD using
oxygen-enhanced MRI 
Chris J Rose1,2, Penny L Hubbard1,2,
Tim F Cootes1,2, Chris J Taylor1,2,
Josephine H Naish1,2, Geoff J Parker1,2,
Simon S Young3, and John C Waterton1,4
1University of Manchester Biomedical Imaging
Institute, Manchester, Greater Manchester, United
Kingdom, 2University
of Manchester Academic Health Science Centre,
Manchester, Greater Manchester, United Kingdom, 3AstraZeneca
R&D, Charnwood, Loughborough, Leicestershire, United
Kingdom, 4AstraZeneca,
Alderley Park, Macclesfield, Cheshire, United Kingdom
MRI is used in natural history and intervention studies
to spatially map parameters throughout organs or tumors.
However, the problem of choosing the best method for
drawing inferences about the populations being studied
is unsolved. Conventionally, a significance test is
applied using averaged parameter values, but this method
neglects heterogeneity. We developed significance tests
for application to a sample of density estimates (smooth
histograms). Using ventilation-to-perfusion (V/Q)
ratio data from an oxygen-enhanced MRI study of COPD
patients and age-matched controls, we can identify
biologically meaningful significant differences in V/Q where
the conventional method fails.
|
|