10:30 |
0602. |
Reference Histogram
Constrained Artifact Suppression (RHiCA) for Incoherently
Undersampled Magnetic Resonance Imaging 
Thomas Gaass1, Guillaume Potdevin2,
Grzegorz Bauman3,4, Peter Noël5,
and Axel Haase1
1Zentralinstitut für Medizintechnik,
Technische Universität München, Garching, Germany, 2Department
of Physics, Technische Universität München, Garching,
Germany, 3Department
of Medical Physics, German Cancer Research Center,
Heidelberg, Germany, 4Department
of Medical Physics, University of Wisconsin, Madison,
Wisconsin, United States, 5Department
of Diagnostic and Interventional Radiology, Technische
Universität München, Munich, Germany
We introduce a novel reconstruction technique (RHiCA),
based on the specific signature of incoherent
undersampling artifacts in histogram space and relying
on a minimization algorithm. Utilizing the histogram of
a low resolution reference image we propose to correct
the impaired image via its altered histogram. The
performance of the Reference Histogram Constrained
Artifact (RHiCA) reduction is successfully presented on
a numerical phantom and a 3T head MRI. We successfully
illustrate, that applying RHiCA leads to an effective
suppression of undersampling artifacts in radial MRI
acquisitions up to undersampling factors of 6.
|
10:42 |
0603.
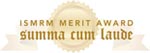 |
A Unified Tensor Regression
Framework for Calibrationless Dynamic, Multi-Channel MRI
Reconstruction 
Joshua D. Trzasko1 and
Armando Manduca1
1Mayo Clinic, Rochester, MN, United States
Previously, low-rank matrix regression methods have been
used to enable "calibrationless" parallel and
"training-free" dynamic MRI reconstruction. In this
work, we present a novel low n-rank tensor regression
framework for calibrationless reconstruction of dynamic
and multi-channel MRI data, and demonstrate that
previously image-domain strategies arise as instances of
this unifying model.
|
10:54 |
0604.
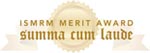 |
Accelerating Encoded
Simultaneous Multi Slice MRI with Compressed Sensing 
Sagar Mandava1, Jean-Philippe Galons2,
and Ali Bilgin1,3
1Electrical and Computer Engineering,
University of Arizona, Tucson, AZ, United States, 2Medical
Imaging, University of Arizona, Tucson, AZ, United
States, 3Biomedical
Engineering, University of Arizona, Tucson, AZ, United
States
Simultaneous Multislice Acquisition (SIMA) by Hadamard-encoded
excitation has been proposed as an alternative to 3D
volume imaging (3DFT) when acquiring fewer than 64
slices to avoid ringing and leakage artifacts. As slices
are excited simultaneously, SIMA enjoys SNR benefits
over slice-by-slice imaging similar to 3DFT. In this
work, we investigate the use of compressive sampling
strategies within the SIMA framework. In addition to
Hadamard and complex Hadamard encoding, we introduce the
use of Noiselet encoding in SIMA.
|
11:06 |
0605.
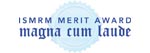 |
Compressed Sensing ASL
Perfusion Imaging Using Adaptive Nonlinear Sparsifying
Transforms 
Yihang Zhou1,2, Yanhua Wang1,2,
Jie Zheng3, and Leslie Ying1,2
1Department of Electrical Engineering,
University at Buffalo, Buffalo, NY, United States, 2Department
of Biomedical Engineering, University at Buffalo,
Buffalo, NY, United States, 3Department
of Radiology, Washington University, St. Louis, MO,
United States
In this study, a broader family of nonlinear transforms
is investigated for sparse representation of dynamic
images in compressed sensing (CS). We propose a novel
kernel-based CS method that implicitly and adaptively
sparsifies the dynamic image series of interest using
nonlinear transforms. The proposed method is evaluated
using accelerated arterial spin labeled perfusion data.
It is shown to be able to better preserve the spatial
and temporal information than the conventional CS method
with linear transforms.
|
11:18 |
0606.
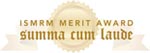 |
Compressed Sensing
Reconstruction with an Additional Respiratory-Phase
Dimension for Free-Breathing Imaging 
Li Feng1,2, Jing Liu3, Kai Tobias
Block4, Jian Xu5, Leon Axel1,2,
Daniel K. Sodickson1,2, and Ricardo Otazo1,2
1Center for Biomedical Imaging, New York
University, School of Medicine, New York, New York,
United States, 2Sackler
Institute of Graduate Biomedical Sciences, New York
University, School of Medicine, New York, New York,
United States, 3Radiology
and Biomedical Imaging, University of California, San
Francisco, San Francisco, CA, United States, 4Center
for Biomedical Imaging, NYU Langone Medical Center, New
York, New York, United States, 5Siemens
Medical Solutions, New York, New York, United States
Respiratory motion reduces the temporal sparsity and
thus the performance of compressed sensing
reconstruction. In this work, we propose a respiratory
motion compensation method for compressed sensing
reconstruction using golden-angle radial sampling by
creating an extra respiratory-phase dimension estimated
from the acquired data with self-gating. The additional
respiratory-phase dimension improves the performance of
compressed sensing for free-breathing imaging due to (a)
additional correlation and thus increased overall
multidimensional sparsity and (b) higher incoherence,
since the dimension is formed by sorting complementary
golden-angle radial data. We demonstrate the feasibility
of the technique for accelerated free breathing cardiac
cine and liver imaging.
|
11:30 |
0607. |
High-Frame-Rate
Full-Vocal-Tract Imaging Based on the Partial Separability
Model and Volumetric Navigation 
Maojing Fu1,2, Bo Zhao1,2, Joseph
Holtrop2,3, Jamie Perry4, David
Kuehn5, Zhi-Pei Liang1,2, and
Bradley P. Sutton2,3
1Department of Electrical and Computer
Engineering, University of Illinois at Urbana-Champaign,
Urbana, Illinois, United States, 2Beckman
Institute for Advanced Science and Technology,
University of Illinois at Urbana-Champaign, Urbana,
Illinois, United States, 3Department
of Bioengineering, University of Illinois at
Urbana-Champaign, Urbana, Illinois, United States, 4Department
of Communication Sciences and Disorders, East Carolina
University, Greenville, North Carolina, United States, 5Department
of Speech and Hearing Science, University of Illinois at
Urbana-Champaign, Urbana, Illinois, United States
Dynamic MRI can provide quantitative assessment on the
anatomy and dynamics of the vocal tract, but usually
suffers from poor spatial or temporal resolution or poor
spatial coverage. This work presents full-vocal-tract
dynamic imaging at a frame rate of 102.2 fps with a 2.2
mm × 2.2 mm × 6.5 mm spatial resolution. It is performed
by incorporating an optimized 3D volumetric navigation
scheme into a Partial Separability (PS) model-based
imaging method. Subtle temporal behaviors of the
articulator motion and fine 3D anatomy of the vocal
tract are well captured and analyzed.
|
11:42 |
0608. |
Ultra-Fast Dynamic MRI for
Lung Tumor Tracking Based on Compressed Sensing 
Manoj K. Sarma1, M. Albert Thomas1,
Peng Hu2, Daniel B. Ennis2, and Ke
K. Sheng3
1Radiological Sciences, UCLA School of
Medicine, Los Angeles, CA, United States, 2Radiological
Sciences, University of California Los Angeles, Los
Angeles, CA, United States, 3Radiation
Oncology, UCLA School of Medicine, Los Angeles, CA,
United States
Radiotherapy guided by MRI has afforded the hardware
potential to treat a moving tumor more accurately but
existing imaging speed is inadequate for 3D real-time
lung and lung tumor imaging. By exploiting the intrinsic
coherence of the patient anatomy during time, we adapted
a k-t SLR compressed sensing method to dramatically
reduce the amount of data that is needed to update a new
dynamic imaging without losing details. We were able to
accurately track moving tumors of nine patients based on
images reconstructed with very high data under-sampling
ratio up to 5% of the original data.
|
11:54 |
0609. |
Whole Heart Motion
Corrected Compressed Sensing for 3D Free Breathing Dynamic
Cardiac MRI 
Muhammad Usman1, Mariya Doneva2,
Tobias Schaeffter1, and Claudia Prieto1,3
1King's College London, London, United
Kingdom, 2Philips
Research Europe, Hamburg, Germany, 3Pontificia
Universidad Catolica de Chile, Santiago, Chile
|
12:06 |
0610. |
Compressive Diffusion MRI –
Part 1: Why Low-Rank? 
Hao Gao1,2, Longchuan Li3, and
Xiaoping P. Hu3
1Department of Mathematics and Computer
Science, Emory University, Atlanta, Georgia, United
States, 2Department
of Radiology and Imaging Sciences, Emory University,
Atlanta, Georgia, United States, 3Department
of Biomedical Engineering, Emory University and Georgia
Institute of Technology, Atlanta, Georgia, United States
This work compares several sparsity models for dynamic
MRI with the focus on diffusion MRI. The low-rank model,
a global sparsification of diffusion images via SVD,
generally was found to be the best model, while the
rank-sparsity decomposition was shown to be the best
when the diffusion images are non-low-rank.
|
12:18 |
0611. |
Accelerating
Compressed-Sensing-Based DCE-MR Image Reconstruction with
GPU 
Jiangsheng Yu1, Yiqun Xue2, and
Hee Kwon Song2
1Toshiba Medical Research Institute USA,
Cleveland, Ohio, United States, 2University
of Pennsylvania, Philadelphia, Pennsylvania, United
States
Synopsis: Temporally constrained reconstruction based on
compressed sensing (CS) has recently been developed for
dynamic MR imaging to obtain high temporal resolution
without losing image quality. The intensive computation
overhead in CS reconstruction has limited the
application for clinical data processing where large
data sets are generated from multi-slice and
multi-channel acquisition. The current work presents a
parallelized GPU implementation to accelerate the
CS-based image reconstruction in DCE-MRI. The forward
and backward gridding operations, which are the
most-time consuming part of the conjugate gradient
searching, is addressed with a radial-point driven
parallelization approach by assigning a thread for each
radial point operation. A comparison with the C++
sequential implementation shows an acceleration factor
of ~15 was achieved on a moderately GPU-powered laptop
computer.
|
|