|
10:30
|
0866.
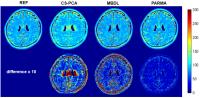 |
Compressive Parametric Manifold Recovery (PARMA) from
Multi-channel Acquisition for Fast Parameter Mapping - Permission Withheld
Chaoyi Zhang1, Yihang Zhou1, Jingyuan
Lyu1, Ukash Nakarmi1, and Leslie Ying1,2
1Electrical Engineering, State University at
buffalo,SUNY, Buffalo, NY, United States, 2Biomedical
Engineering, State University at Buffalo,SUNY, Buffalo, NY,
United States
MR parameter mapping has shown great potential but is still
limited in clinical application due to the lengthy
acquisition time. To address this issue, we proposed a
novel image reconstruction method(PARMA) to accelerate
parameter mapping with reduced multi-channel acquisition
using alternating projections on the single-exponential
parametric manifold, the subspace data consistancy, and the
convex of the regularized coil sensitivities. The
experimental results show the potential of highly
accelerated quantitative imaging by the proposed method.
|
|
10:42
|
0867.
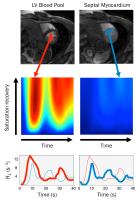 |
A general low-rank tensor framework for high-dimensional cardiac
imaging: Application to time-resolved T1 mapping 
Anthony G. Christodoulou1,2, Jaime L. Shaw2,3,
Behzad Sharif2,4, and Debiao Li2,3
1Heart Institute, Cedars-Sinai Medical Center,
Los Angeles, CA, United States, 2Biomedical
Imaging Research Institute, Cedars-Sinai Medical Center, Los
Angeles, CA, United States, 3Department
of Bioengineering, University of California, Los Angeles,
Los Angeles, CA, United States, 4Department
of Biomedical Sciences, Cedars-Sinai Medical Center, Los
Angeles, CA, United States
We present a general low-rank tensor framework for
high-dimensional cardiac imaging, modeling the underlying
image as partially separable in all relevant dimensions:
space, cardiac phase, respiratory phase, wall-clock time
(e.g., for contrast agent dynamics), variable sequence
parameters (e.g., inversion time), etc. An explicit-subspace
variant of the framework is demonstrated, with subspaces
estimated from navigator data and a signal recovery
dictionary of solutions to the Bloch equations (similar to
MR fingerprinting). This variant is used to perform ECG-less
cardiac- and time-resolved T1 mapping
during first-pass perfusion, as well as free-breathing, ECG-less
native T1 mapping
at multiple cardiac phases. The framework shows promise for
time-resolved T1 mapping
and other high-dimensional applications.
|
|
10:54
|
0868.
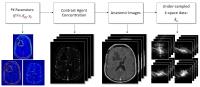 |
Direct Reconstruction of Kinetic Parameter Maps in Accelerated
Brain DCE-MRI using the Extended-Tofts Model 
Yi Guo1, Sajan Goud Lingala1, Yinghua
Zhu1, R. Marc Lebel2, and Krishna S
Nayak1
1Electrical Engineering, University of Southern
California, Los Angeles, CA, United States, 2GE
Healthcare, Calgary, AB, Canada
Pharmacokinetic (PK) parameter maps derived from DCE-MRI
provide quantitative physiological information that aids in
cancer diagnosis and assessment of treatment response.
Recently, direct reconstruction of PK maps from
under-sampled k,t-space has shown great potential to provide
optimal detection of kinetic parameter maps from an
information theoretic perspective. We build on prior work
(using the Patlak model) and demonstrate direct
reconstruction of kinetic parameter maps using the
extended-Tofts model, which is a more appropriate model in
brain tumor. We demonstrate convergence behavior,
computational efficiency, and application to brain DCE-MRI.
|
|
11:06
|
0869.
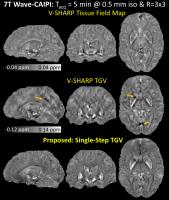 |
TGV-Regularized Single-Step Quantitative Susceptibility Mapping 
Itthi Chatnuntawech1, Patrick McDaniel1,
Stephen F. Cauley2,3, Borjan A. Gagoski3,4,
Christian Langkammer5, Adrian Martin6,
Ellen Grant3,4, Lawrence L. Wald2,3,7,
Kawin Setsompop2,3, Elfar Adalsteinsson1,7,8,
and Berkin Bilgic2
1Electrical Engineering and Computer Science,
Massachusetts Institute of Technology, Cambridge, MA, United
States, 2Department
of Radiology, A. A. Martinos Center for Biomedical Imaging,
Charlestown, MA, United States, 3Harvard
Medical School, Boston, MA, United States, 4Fetal-Neonatal
Neuroimaging & Developmental Science Center, Boston
Children’s Hospital, Boston, MA, United States, 5Department
of Neurology, Medical University of Graz, Graz, Austria, 6Applied
Mathematics, Universidad Rey Juan Carlos, Madrid, Spain, 7Harvard-MIT
Health Sciences and Technology, Cambridge, MA, United
States, 8Institute
for Medical Engineering and Science, Cambridge, MA, United
States
To directly estimate tissue magnetic susceptibility
distribution from the raw phase of a gradient echo
acquisition, we propose a single-step quantitative
susceptibility mapping (QSM) method that benefits from its
three components: (i) the single-step processing that
prevents error propagation normally encountered in
multiple-step QSM algorithms, (ii) multiple spherical mean
value kernels that permit high fidelity background removal,
and (iii) total generalized variation regularization that
promotes a piecewise-smooth solution without staircasing
artifacts. A fast solver for the proposed method, which
enables simple analytical solutions for all of the
optimization steps, is also developed. Improved image
quality over conventional QSM algorithms is demonstrated
using the SNR-efficient Wave-CAIPI and 3D-EPI acquisitions.
|
|
11:18
|
0870.
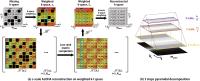 |
In vivo accelerated MR parameter mapping using annihilating
filter-based low rank Hankel matrix (ALOHA) 
Dongwook Lee1, Kyong Hwan Jin1,
Eung-yeop Kim2, Sunghong Park1, and
Jong Chul Ye1
1Bio and Brain Engineering, Korea Advanced
Institute of Science and Technology, Daejeon, Korea,
Republic of, 2Radiology,
Gachon University Gil Medical Center, Inchoen, Korea,
Republic of
The purpose of this study is to develop an accelerated MR
parameter mapping technique. For accelerated T1 and T2
mapping, spin-echo inversion recovery and multi-echo spin
echo pulse sequences were redesigned to perform
undersampling along phase encoding direction. The highly
missing k-space were then interpolated by using recently
proposed annihilating filter based low-rank Hankel matrix
approach (ALOHA). By exploiting the duality between the
transform domain sparsity and the low-rankness of weighted
Hankel structured matrix in k-space, ALOHA provided
outperforming reconstruction results compared to the
existing compressed sensing methods.
|
|
11:30
|
0871.
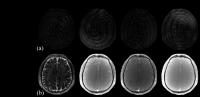 |
A Model-Based Approach to Accelerated Magnetic Resonance
Fingerprinting Time Series Reconstruction 
Bo Zhao1, Kawin Setsompop1, Borjan
Gagoski2, Huihui Ye1, Elfar
Adalsteinsson3, P. Ellen Grant2, and
Larry L. Wald1
1Athinoula A. Martinos Center for Biomedical
Imaging, Chalestown, MA, United States, 2Boston
Children's Hospitial, Boston, MA, United States, 3EECS,
MIT, Cambridge, MA, United States
A new model-based approach using low-rank and sparsity
constraints is presented for reconstructing the accelerated
magnetic resonance fingerprinting (MRF) time-series images.
By enabling high-quality reconstructions of
contrast-weighted images from highly-undersampled data, the
proposed method produces more accurate estimates of tissue
parameter maps compared to the conventional gridding based
reconstruction of the time-series. Ultimately, the goal is
to reduce imaging time for MRF acquisitions and improve
spatial resolution.
|
|
11:42
|
0872.
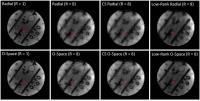 |
Low-Rank O-Space Reconstruction 
Haifeng Wang1, Emre Kopanoglu1, R.
Todd Constable1,2, and Gigi Galiana1
1Department of Radiology and Biomedical Imaging,
Yale University, New Haven, CT, United States, 2Department
of Neurosurgery, Yale University, New Haven, CT, United
States
Low-Rank O-Space presents a scheme to incorporate O-Space
imaging with Low-Rank matrix recovery. The Low-Rank
reconstruction based on iterative nonlinear conjugate
gradient algorithm is applied to substitute the previous
Kaczmarz and Compressed Sensing (CS) reconstructions to
recover highly undersampled O-Space data. The simulations
and experiments illustrate the proposed scheme can remove
artifacts and noise in O-Space imaging at high reduction
factors, compared to results recovered by Kaczmarz and CS.
Moreover, the proposed method does not need to modify the
conventional O-Space pulse sequences, and reconstruction
results are better than those in radial imaging recovered by
Kaczmarz, CS, or Low-Rank methods.
|
|
11:54
|
0873.
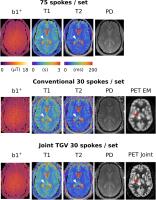 |
Simultaneous multi-modality/multi-contrast image reconstruction
with nuclear-norm TGV 
Florian Knoll1, Martin Holler2, Thomas
Koesters1, Martijn Cloos1, Ricardo
Otazo1, Kristian Bredies2, and Daniel
K Sodickson1
1Center for Advanced Imaging Innovation and
Research (CAI2R) and Bernard and Irene Schwartz Center for
Biomedical Imaging, Department of Radiology, NYU School of
Medicine, New York, NY, United States,2Mathematics
and Scientific Computing, University of Graz, Graz, Austria
A typical clinical imaging protocol covers a large number of
different image contrasts and, in the era of multi-modality
systems, even different imaging modalities. While the
resulting datasets share a substantial amount of structural
information, they consist of fundamentally different
contrasts and signal values and show unique features and
image content. We propose a reconstruction framework based
on nuclear-norm second-order Total Generalized Variation
that exploits structural similarity both between different
contrasts and modalities while still being flexible with
respect to signal intensity and unique features. Numerical
simulations and in vivo MR-Fingerprinting experiments
demonstrate improved PET resolution and improved depiction
of quantitative values. The proposed approach allows a 6
minute whole brain coverage exam that provides both
quantitative PET and MR-relaxation parameters.
|
|
12:06
|
0874.
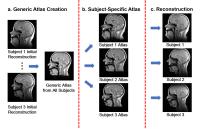 |
Spatiotemporal-atlas-based High-resolution Dynamic Speech MRI 
Maojing Fu1, Jonghye Woo2, Marissa
Barlaz3, Ryan Shosted3, Zhi-Pei Liang1,
and Bradley Sutton4
1Electrical and Computer Engineering, University
of Illinois at Urbana-Champaign, Urbana, IL, United States, 2CAMIS
(Center for Advanced Medical Imaging Sciences),
Massachusetts General Hospital, Boston, MA, United States, 3Linguistics,
University of Illinois at Urbana-Champaign, Urbana, IL,
United States, 4Bioengineering,
University of Illinois at Urbana-Champaign, Urbana, IL,
United States
Dynamic speech MRI holds great promise for visualizing
articulatory motion in the vocal tract. Recent work has
enabled accelerated imaging speed, resulting in the need to
integrate mechanisms to enable interpretation of the dynamic
images that contain great amounts of movement information.
This work integrates a spatiotemporal atlas into a partial
separable (PS) model-based imaging framework and uses the
atlas as prior information to improve reconstruction
quality. This method not only captures high-quality dynamics
at 102 frames per second, but also enables quantitative
characterization of articulatory variability utilizing the
residual component from the atlas-based sparsity constraint.
|
|
12:18
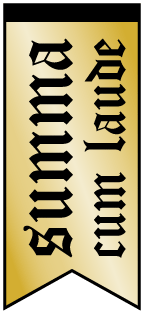 |
0875.
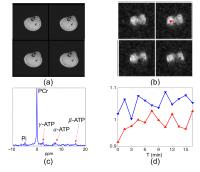 |
High-Resolution Dynamic 31P-MRSI Using High-Order Partially
Separable Functions 
Chao Ma1, Fan Lam1, Qiang Ning1,2,
Bryan A. Clifford1,2, Qiegen Liu1,
Curtis L. Johnson1, and Zhi-Pei Liang1,2
1Beckman Institute, University of Illinois
Urbana-Champaign, Urbana, IL, United States, 2Electrical
and Computer Engineering, University of Illinois
Urbana-Champaign, Urbana, IL, United States
Dynamic MRSI measures the temporal changes of metabolite
concentrations by acquiring a time series of MRSI data.
These data can be used in a range of applications, including
the study of the response of a metabolic system to a
perturbation. However, high-resolution dynamic MRSI is
challenging due to poor SNR resulting from the low
concentrations of metabolites. This work presents a new
method for high-resolution dynamic 31P-MRSI using high-order
partially separable functions. The method has been validated
using in vivo dynamic 31P-MRSI experiments, producing
encouraging results.
|
|