Poster: Quantitation, Prediction & Machine Learning in the Brain
Electronic Power Pitch Poster
Neuro
Monday, 24 April 2017
Exhibition Hall |
17:15 - 18:15 |
|
|
Plasma # |
|
0252.
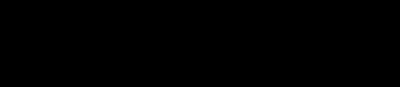 |
16 |
Machine Learning Based Diagnosis of Early Parkinson's Disease using QSM 
Seon Lee, Joon Yul Choi, Jeehun Kim, Sun Won Park, Jongho Lee
This study proposed a support vector machine model to classify early PD patients from healthy controls using QSM. The results validated better performance of SVM than conventional logistic regression based on statistical ordering of backward feature selection. This computer aided technique may help to reduce misdiagnosis rate of early-PD patients.
|
|
0253.
 |
17 |
Reproduciblity of advanced MR metrics in a multi-site, multi-vendor study of mild traumatic brain injury 
Andrew Nencka, Timothy Meier, Yang Wang, Yu-Chien Wu, Brad Swearingen, Robin Karr, Melissa Koschnitzke, Andy Saykin, Michael McCrea, Kevin Koch
The Advanced Research Core of the Concussion Assessment Research and Education consortium includes a multi-site, multi-vendor, multi-contrast imaging acquisition protocol with T2 FLAIR, quantitative T2*, task free fMRI, ASL, and DTI. This abstract describes the variance of measurements made in the first year and a half of the study from these imaging methods across a cohort of non-contact sport control athletes. With the variance of these measurements in the colligate athlete population known, the sensitivities of the individual and combined contrasts to diagnose injury and recovery can be assessed.
|
|
0254.
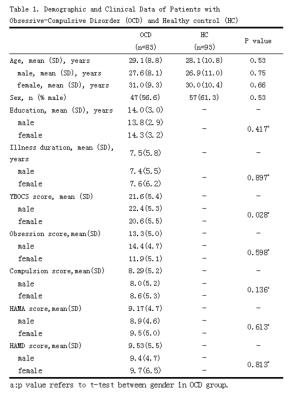 |
18 |
Characteristic Changes of Volume and Shape of Subcortical Structures in Obsessive-Compulsive Disorder 
Lianqing Zhang, Xinyu Hu, Ming Zhou, Lu Lu, Xiaoxiao Hu, Xiaoqi Huang
We analysis subcortical nucleus volume and shape alterations in a relatively large sample of drug-naive adult patients with Obsessive-Compulsive disorder by using an automatically segmentation and vertex-based shape analysis protocol. Our findings indicated that (i)Gender effects were found in OCD, male patients seems are affected in reward system whereas females might have more disrupted stratum-pallidum-thalamus pathway which might be related to chronic stress. (ii)Shape analysis may provide more anatomical change information from a different perspective. Future structural research should consider shape and volume alterations together when explore brain changes.
|
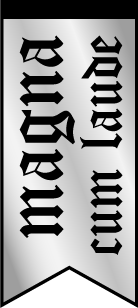 |
0255.
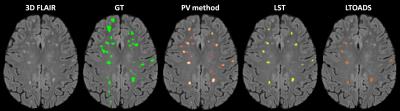 |
19 |
PARTIAL VOLUME ESTIMATION IN MULTIPLE SCLEROSIS LESION SEGMENTATION 
Mário Joăo Fartaria , Alexandra Sorega, Tobias Kober, Gunnar Krueger, Cristina Granziera, Alexis Roche, Meritxell Bach Cuadra
Partial volume (PV) is the effect of having a mixture of tissues present within a voxel. This effect occurs in tissue borders and affects small structures such as small multiple sclerosis (MS) lesions. Ignoring PV effects in volumetry may lead to significant estimation errors. Here, we propose a novel automated MS lesion segmentation technique that takes PV effects into account. The proposed method shows higher accuracy in terms of lesion volume estimation compared to a manually segmented ground truth as well as significant improvement in detection of small lesions, also in comparison to two software packages for MS lesion segmentation.
|
|
0256.
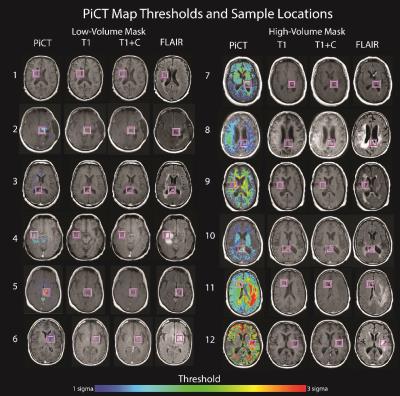 |
20 |
Predictive cytological topography highlights regions of pathologically confirmed non-enhancing hypercellular tumor in glioblastoma patients 
Sarah Hurrell, Elizabeth Cochran, Sean McGarry, Amy Kaczmarowski, Jennifer Connelly, Wade Mueller, Scott Rand, Kathleen Schmainda, Peter LaViolette
This study combines clinical brain cancer imaging and pathological microscopy with machine learning to generate predictive maps off pathological features (i.e. new contrasts) based on segmented histological cellularity. Predictive cytological topography (PiCT) maps of cellularity were utilized to detect additional pathologically confirmed infiltrative glioblastoma cellularity beyond margins of contrast enhancement.
|
|
0257.
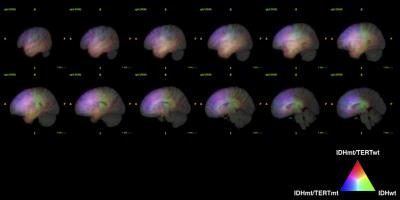 |
21 |
Radiogenomics of 201 WHO grade 2 and 3 gliomas 
Manabu Kinoshita, Hideyuki Arita, Masamishi Takahashi, Yoshitaka Narita, Yuzo Terakawa, Naohiro Tsuyuguchi, Yoshiko Okita, Masahiro Nonaka, Shusuke Moriuchi, Junya Fukai, Shuichi Izumoto, Kenichi Ishibashi, Yoshinori Kodama, Kanji Mori, Koichi Ichimura, Yonehiro Kanemura
Genetic alterations found in WHO grade 2 and 3 gliomas include IDH1/2 and TERT promoter mutations and 1p19q co-deletion. In this research, the authors attempted to test the hypothesis that genetic alterations could contribute to the locations and texture of the tumor by analyzing 201 WHO grade 2 and 3 gliomas using radinogenomic analysis. The authors were able to confirm the hypothesis that genetic alterations do contribute to the locations and textures of the tumor. 15 radionomic features were identified and those features were able to identify 3 genetic subgroups of WHO grade 2 and 3 gliomas.
|
|
0258.
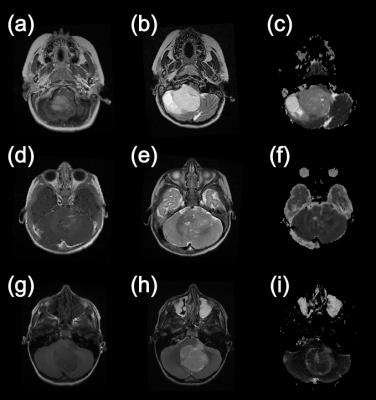 |
22 |
Classification of Pediatric Brain Tumours using Apparent Diffusion Coefficient – a Multi-Centre Study 
Jan Novak, Niloufar Zarinabad, Theodoros Arvanitis, Lesley MacPherson, Daniel Rodriguez , Richard Grundy, Dorothee Auer, Tim Jaspan, Shivaram Avula, Laurence Abernethy, Patrick Hales, Ramneek Kaur, Darren Hargrave, Dipayan Mitra, Simon Bailey, Nigel Davies, Christopher Clark, Andrew Peet
The purpose of the study was to investigate the potential of apparent diffusion coefficient (ADC) to discriminate between paediatric cerebellar tumours on a multicentre basis using histograms. A total of 117 paediatric patients with cerebellar tumours (55 Medulloblastomas, 36 Pilocytic Astrocytomas and 26 Ependymomas) were imaged using diffusion weighted imaging across 12 different hospitals using a total of 18 different scanners. Overall classification accuracies for the ADC histogram metrics were 86 % using Naďve Bayes and 84 % for Random Forest classifier.
|
|
0259.
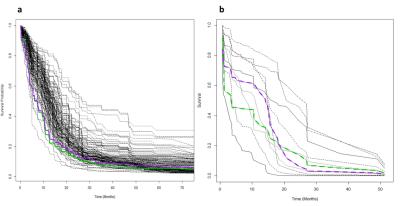 |
23 |
Exploiting radiogenomics data for personalised prediction of glioblastoma - permission withheld
Paul Blakeley, Chia-Feng Lu, Fei-Ting Hsu, Li-Chun Hsieh, Yu-Chieh Jill Kao, Huai-Lu Chen, Ping-Huei Tsai, Hua-Shan Liu, Gilbert Aaron Lee, Cheng-Yu Chen
The present study demonstrates the feasibility of machine learning in radiogenomics to predict patient outcome. The Random Forest Survival model is able to predict patient survival based on apparent diffusion coefficients or gene expression data without any prior knowledge.
|
|
0260.
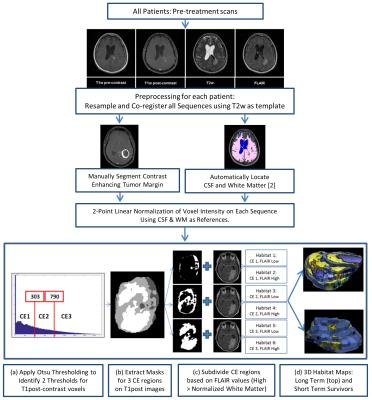 |
24 |
Multiparameter MRI Predictors of Extreme Survival in Glioblastoma Multiforme 
Natarajan Raghunand, Olya Stringfield, John Arrington, Robert Gatenby
We retrospectively analyzed initial MRI scans in two cohorts diagnosed with Glioblastoma Multiforme (GBM). The Long-Term Survival (LTS) cohort included 13 subjects who survived >36 months post-diagnosis, while the Short-Term Survival (STS) cohort included 12 subjects who survived ≤18 months. Tumor voxels were clustered by the normalized signal intensities on post-contrast T1w and FLAIR sequences into 6 distinct “habitats”. The LTS cohort were found to have a significantly higher fraction of Habitat 6 (high signal on T1w and FLAIR sequences) and lower fraction of Habitat 2 (low signal on T1w and high signal on FLAIR) compared with the STS cohort.
|
|
0261.
 |
25 |
Multi-Site Concordance of DSC-MRI Analysis for Brain Tumors: Results of a NCI Quantitative Imaging Network DSC-MRI Collaborative Project 
Kathleen Schmainda, Melissa Prah, Scott Rand, Mark Muzi, Swati Rane, Xiao Da, Yi-Fen Yen, Jayashree Kalpathy-Cramer, Thomas Chenevert, Dariya Malyarenko, Benjamin Hoff, Brian Ross, Yue Cao, Madhava Aryal, Bradley Erickson, Panagiotis Korfiatis, Laura Bell, Leland Hu, Christopher Quarles
Though DSC-MRI perfusion is of well-known benefit for the evaluation of brain tumors, clinical translation has been hampered by a lack of confidence in the consistency of the derived RCBV (relative cerebral blood volume) and cerebral blood flow (CBF) values across sites and platforms. This multi-site and multi-platform study, for which the same patient data set was analyzed, demonstrated substantial consistency in RCBV across software sites and platforms and the ability of each to distinguish low-grade from high-grade tumor. In addition, a single RCBV threshold was identified for which all platforms maintained good accuracy.
|
|
0262.
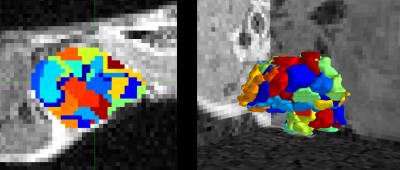 |
26 |
Perfusion-supervoxels for DCE-MRI based tumor subregion assessment - permission withheld
Benjamin Irving , Jolanta Mirecka, Ana Gomes, Danny Allen, Paul Kinchesh, Veerle Kersemans, Stuart Gilchrist, Sean Smart, Julia Schnabel, Sir J Michael Brady, Michael Chappell
Tumors exhibit chaotic and leaky vasculature, which leads to variations in perfusion, and regions of edema, hypoxia and necrosis. We develop a method to extract perfusion-supervoxels, regions of locally similar perfusion, and use these regions with k-means clustering to define tumor subregions that are robust to noise and outliers. This method offers a number of advantages over both mean tumor parameters and voxelwise clustering.
|
|
0263.
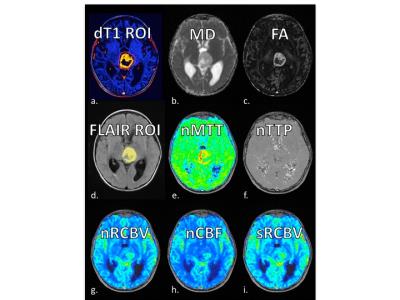 |
27 |
A New Combined Perfusion and Diffusion MRI Biomarker to Distinguish Pediatric High-Grade Glioma from Pilocytic Astrocytoma 
Kathleen Schmainda, Melissa Prah, Jose Palomares, Mohit Maheshwari, Sean Lew, Teresa Kelly
Pediatric high-grade glioma (HGG) and pilocytic-astrocyoma/optic-pathway gliomas (PA+OPG) are each very vascular tumors but vary substantially in their treatment, clinical course and long term prognosis. In this study we demonstrate that measurements that work in adults to distinguish tumor grades (eg RCBV) cannot distinguish between pediatric HGG and PA+OPG, but a new MRI biomarker, the ratio of standardized RCBV (sRCBV) to mean diffusivity (MD) can cleanly making this distinction. This finding has significant implications for treatment management in the pediatric brain tumor population.
|
|
0264.
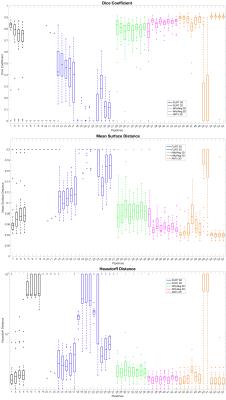 |
28 |
A ranking of pipelines for optimal co-registration of anatomical and diffusion weighted images of the cervical spinal cord 
Stephanie Alley, Francesco Grussu, Marios Yiannakas, Hugh Kearney, Olga Ciccarelli, Ferran Prados, Sébastien Ourselin, Claudia Gandini Wheeler-Kingshott
We conduct the first systematic evaluation of the performance of three widely used registration software toolkits (FLIRT from FSL, NiftyReg and ANTs employing Spinal Cord Toolbox) in an effort to outline a method for reliable co-registration between anatomical and quantitative (EPI-based) spinal cord MRI. We generate a diverse set of registration pipelines and rank them according to quality of co-registration metrics. We find that ANTs and NiftyReg outperform FLIRT, and we report heterogeneity of specifications for optimal co-registration among software toolkits.
|
|
0265.
 |
29 |
Deep-Neural-Network based image diagnosis: comparing various image preprocessing strategies to achieve higher accuracy and understanding of the decision 
Yasuhiko Tachibana, Takayuki Obata, Jeff Kershaw, Yoko Ikoma, Tokuhiko Omatsu, Riwa Kishimoto, Tatsuya Higashi
The purpose of this study was to investigate how image preprocessing might help overcome two problems for deep-neural-network (DNN) based image diagnosis: the need for a large training database to achieve high accuracy and the difficulty humans have in understanding the internal decision process. Five DNNs were trained with a brain image series (preprocessed in five different ways), to judge the age-range of a volunteer. The performance of the DNNs was then compared statistically. The results suggested that image preprocessing may facilitate higher accuracy, and also make it easier to understand how and why a judgement was made.
|
|
0266.
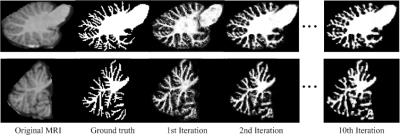 |
30 |
Cerebellum Tissue Segmentation with Ensemble Sparse Learning 
Jiawei Chen, Li Wang, Dinggang Shen
Accurate segmentation of cerebellum is important in studying the structural changes in brain and the alert in different neuro-developmental disorders. However, cerebellum has received relatively little attention in the image processing literature, compared with cerebrum segmentation. In fact, cerebellum tissue segmentation is also very challenging due to severe partial volume effect and low contrast. In this study, an ensemble sparse learning is proposed for cerebellum tissue segmentation, where the goal is to segment the tissues in cerebellum into white matter (WM), gray matter (GM) and cerebrospinal fluid (CSF). The experiment results demonstrate that our proposed method show advantages in cerebellum tissue segmentation.
|
|