|
Electronic Poster Session Acquisition, Reconstruction & Analysis |
Monday, 18 June 2018
Electronic PosterAcquisition, Reconstruction & Analysis
3362 -3385 Machine Learning for Image Reconstruction
3386 -3408 RF Pulses & Sequences
3409 -3432 Image Analysis
3481 -3504 Machine Learning for Image Analysis
3505 -3528 Image Reconstruction Potpourri
3529 -3552 Compressive MRI |
|
Machine Learning for Image Reconstruction
Electronic Poster
Acquisition, Reconstruction & Analysis
Monday, 18 June 2018
Exhibition Hall |
13:45 - 14:45 |
|
|
Computer # |
|
3362.
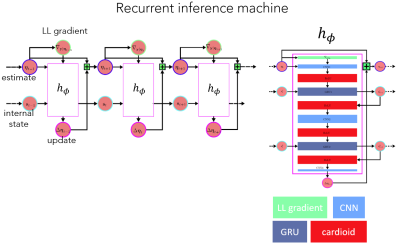 |
49 |
A Recurrent Inference Machine for accelerated MRI reconstruction at 7T
Kai Lønning, Patrick Putzky, Max Welling, Matthan Caan
Accelerating high resolution brain imaging at 7T is needed to reach clinically feasible scanning times. Deep learning applies multi-layered neural networks as universal function approximators and is able to find its own compression implicitly. We propose a Recurrent Inference Machine (RIM) that is designed to be a general inverse problem solver. Its recurrent architecture can acquire great network depth, while still retaining a low number of parameters. The RIM outperforms compressed sensing in reconstructing 0.7mm brain data. On the reconstructed phase images, Quantitative Susceptibility Mapping can be performed.
|
|
3363.
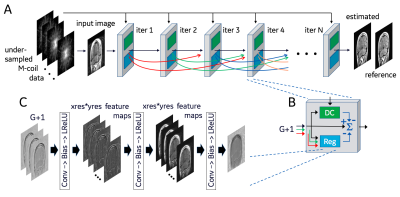 |
50 |
Densely Connected Iterative Network for Sparse MRI Reconstruction
Itzik Malkiel, Sangtae Ahn, Zac Slavens, Valentina Taviani, Christopher Hardy
We propose a densely connected deep convolutional network for reconstruction of highly undersampled MR images. Eight-channel 2D brain data with fourfold undersampling were used as inputs, and the corresponding fully-sampled reconstructed images as references for training. The algorithm produced notably higher-quality images than state-of-the-art parallel imaging and compressed sensing methods, both in terms of reconstruction error and perceptual quality. The dense architecture was found to significantly outperform a similar network without dense connections.
|
|
3364.
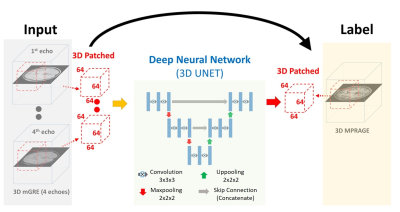 |
51 |
Reconstruction of synthetic T1 MPRAGE via Deep Neural Network from Multi Echo Gradient Echo images.
Kanghyun Ryu, Yoonho Nam, Na-young Shin, Jinhee Jang, Jiyong Park, Dong-Hyun Kim
We propose to use deep learning to reconstruct synthetic T1-weighted Magnetization prepared rapid gradient echo (MPRAGE) image from multi echo gradient echo (mGRE) images. With our method, high tissue contrast can be achieved without actual MPRAGE scan, which could be utilized for post processing methods, such as tissue segmentation or volumetric quantification. We validated our method’s accuracy by comparing the result of synthetic images with the true image via segmentation and volumetry. Additionally, we tested our method on clinical images containing pathologies not seen in the training set.
|
|
3365.
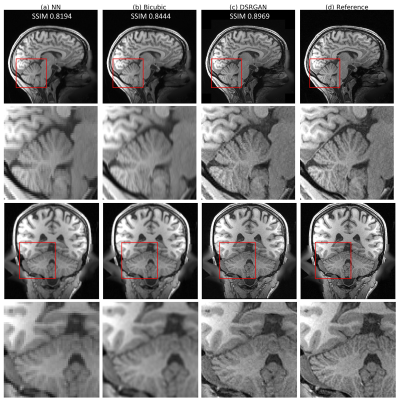 |
52 |
Fast and Realistic Super-Resolution in Brain Magnetic Resonance Imaging using 3D Deep Generative Adversarial Networks
Yuhua Chen, Feng Shi, Yibin Xie, Zhengwei Zhou, Anthony Christodoulou, Debiao Li
High-resolution magnetic resonance image (MRI) are favorable by clinical application thanks to its detailed anatomical information. However, high spatial resolution typically comes at the expense of longer scan time, less spatial coverage, and lower signal to noise ratio (SNR). Single Image Super-Resolution (SISR), a technique aimed to restore high-resolution (HR) details from one single low-resolution (LR) input image, has been improved dramatically by the recent invention of deep Generative Adversarial Networks(GAN). In this paper, we introduce a new neural networks structure, 3D Densely Connected Super-Resolution GAN (DSRGAN) to realistic restore HR features of structural brain MR images. Through experiments on a dataset with 1,113 subjects, we demonstrate that our network outperforms bicubic interpolation in restoring 4x resolution-reduced images.
|
|
3366.
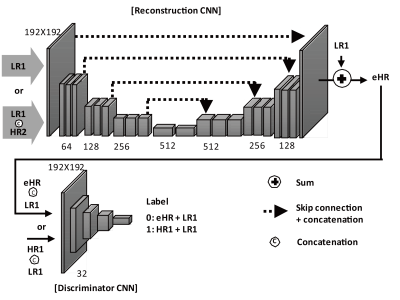 |
53 |
MR Image Generation with Deep Learning Incorporating Anatomical Prior Knowledge Video Permission Withheld
Ki Hwan Kim, Won-Joon Do, Sung-Hong Park
We proposed a new convolutional neural network (CNN) to generate high resolution (HR) MR images from highly down-sampled MR images, incorporating HR images in another contrast. Anatomical information from another HR images and adversarial loss functions allowed the proposed model to restore details and edges clearly from the down-sampled images, proved in normal and brain tumor regions. Pre-training with a public database improved performance in real human applications. The proposed methods outperformed several CS algorithms in both pseudo-k-spaces from public data and real k-spaces from human brain data. CNNs can be a good alternative for accelerating routine MRI scanning.
|
|
3367.
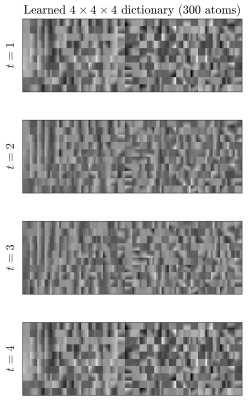 |
54 |
Image Reconstruction of Accelerated Dynamic MRI using Spatiotemporal Dictionaries with Global Sparsity Regularization
Valery Vishnevskiy, Sebastian Kozerke
Adaptive spatiotemporal dictionaries offer improved reconstruction accuracy for dynamic cardiac MRI. However, most modern methods perform local encoding of image patches treating them independently. In order to increase reconstruction quality, we present a convex model that allows global control of encoding sparsity. The proposed method has a single tunable parameter and delivers 9% peak signal-to-noise ratio improvement of reconstruction compared to the state-of-the-art dictionary-based approach. Moreover, the implemented numerical scheme allowed 3-fold reconstruction time reduction.
|
|
3368.
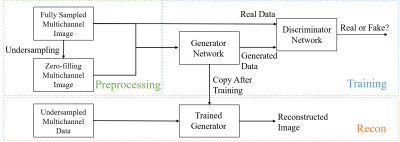 |
55 |
Parallel Imaging Reconstruction with a Conditional Generative Adversarial Network
Pengyue Zhang, Fusheng Wang, Yu Li
This work presents a parallel imaging reconstruction framework based on deep neural networks. A conditional generative adversarial network (conditional GAN) is used to learn how to recover anatomical image structure from undersampled data for imaging acceleration. The new approach is shown to be suitable for image reconstruction with high undersampling factors when conventional parallel imaging suffers from a g-factor increase.
|
|
3369.
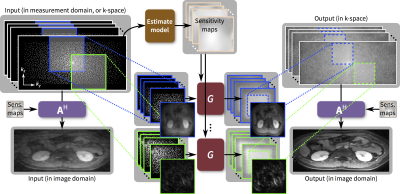 |
56 |
Highly-Scalable Image Reconstruction using Deep Neural Networks with Bandpass Filtering
Joseph Cheng, Feiyu Chen, Marcus Alley, John Pauly, Shreyas Vasanawala
To increase the flexibility and scalability of deep convolution neural networks in the context of MRI reconstruction, a framework is proposed using bandpass filtering. The introduction of bandpass filtering enables us to leverage imaging physics while ensuring that the final reconstruction is consistent with known measurements to maintain diagnostic accuracy. We demonstrate this architecture for reconstructing subsampled datasets of contrast-enhanced T1-weighted volumetric scans of the abdomen. Additionally, we demonstrate the generality of the framework through the reconstruction of wave-encoded 2D single-shot fast-spin-echo scans of the abdomen. The proposed technique performs comparably with state-of-the-art techniques while offering the ability for simple parallelization and increase computational speed.
|
|
3370.
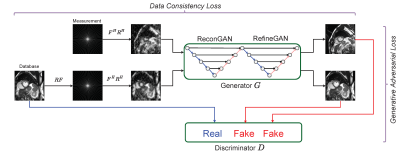 |
57 |
Compressed Sensing MRI Reconstruction using Generative Adversarial Networks with Cyclic Loss.
Tran Minh Quan, Thanh Nguyen-Duc, Won-Ki Jeong
Compressed Sensing MRI (CS-MRI) has provided theoretical foundations upon which the time-consuming MRI acquisition process can be accelerated. However, it primarily relies on iterative numerical solvers which still hinders their adaptation in time-critical applications. In addition, recent advances in deep neural networks have shown their potential in computer vision and image processing, but their adaptation to MRI reconstruction is still at an early stage. Therefore, we propose a novel compressed sensing MRI reconstruction algorithm based on a deep generative adversarial neural network with cyclic data consistency constraint. The proposed method is fast and outperforms the state-of-the-art CS-MRI methods by a large margin in running times and image quality, which is demonstrated via evaluation using several open-source MRI databases.
|
|
3371.
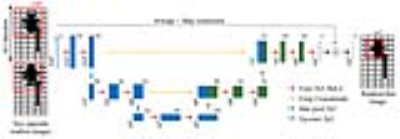 |
58 |
A Learning-based Metal Artifacts Correction Method using Dual-Polarity Readout Gradients
Kinam Kwon, Jaejin Cho, Seohee So, Byungjai Kim, Namho Jeong, HyunWook Park
Metallic implants induce large field perturbations, which generate various types of artifacts according to the spatial encoding mechanisms in MRI. Especially, a frequency encoding dimension is influenced by bulk displacements with off-resonance frequencies and the pixel sizes are distorted in the frequency encoding dimension. In the abstract, a new learning-based method is proposed to map two metal-induced-artifacts images with positive and negative-polarity readout gradients into a metal-induced-artifacts-free image. Simulated data was utilized for training the network instead of real MR data that requires many resources to be collected.
|
|
3372.
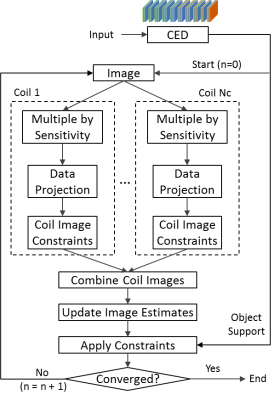 |
59 |
DL-POCS: Deep Learning Augmented POCS Reconstruction for Vastly Undersampled MR Data
Fang Liu, Julia Velikina, Richard Kijowski, Alexey Samsonov
We introduced a novel reconstruction framework by combining deep learning (DL) neural network with the Projections Onto Convex Sets (POCS) algorithm, termed DL-POCS. The image restoration from undersampled images was first performed by a convolutional encoder-decoder network. Then the output from deep learning was used as initialization and extra constraints were imposed to promote the POCS reconstruction. We evaluated this approach on vastly undersampled knee MR data and found that this combined approach is superior to each of individual components alone. Our study suggests that deep learning regularized image reconstruction will have a substantial impact on data-driven accelerated MR imaging.
|
|
3373.
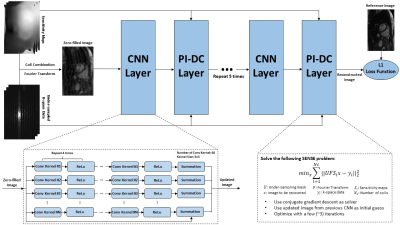 |
60 |
Parallel Imaging and Convolutional Neural Network Combined Fast Image Reconstruction for Low Latency Accelerated 2D Real-Time Imaging
Ziwu Zhou, Fei Han, Vahid Ghodrati, Yu Gao, Yingli Yang, Peng Hu
Real-time imaging is a powerful technique to exam multiple physiological motions are the same time. Previous literature has described methods to accelerate the real-time imaging acquisition down to 20ms with the help of compressed sensing. However, reconstruction time remains relatively long, preventing its wide clinical use. Recent developments in deep learning have shown great potential in reconstructing high-quality MR images with low-latency reconstruction. In this work, we proposed a framework that combines the parallel imaging, which is a unique feature in MR imaging, with convolution neural network to reconstruct 2D real-time images with low-latency and high-quality.
|
|
3374.
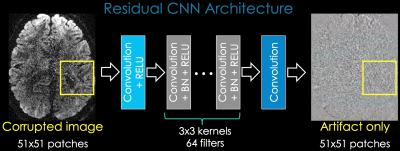 |
61 |
Combining MR-Physics and Machine Learning to Address Intractable Reconstruction Problems
Berkin Bilgic, Stephen Cauley, Itthi Chatnuntawech, Mary Kate Manhard, Fuyixue Wang, Melissa Haskell, Congyu Liao, Lawrence Wald, Kawin Setsompop
We are combining Machine Learning (ML) with MR-physics based image reconstruction to tackle intractable problems. We address open problems that are either too stochastic to be modeled (e.g. shot-to-shot phase variations in multi-shot EPI due to physiological noise), or that admit a computationally prohibitive model (e.g. motion correction with simultaneous estimation of motion parameters and image content). Using ML to jumpstart physics-based non-convex reconstructions dramatically improve their efficiency and helps avoid local minima. In return, MR-physics reconstruction keeps ML in check, and avoids using it as a blackbox. Such synergistic combination also provides >2x reduction in RMSE over conventional reconstruction.
|
|
3375.
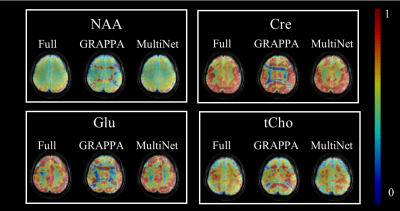 |
62 |
MultiNet PyGRAPPA: A Novel Method for Highly Accelerated Metabolite Mapping
Sahar Nassirpour, Paul Chang, Anke Henning
In this work, a novel acceleration method (MultiNet PyGrappa) is introduced which enables high in-plane acceleration factors for non-lipid suppressed 1H MRSI data. By using a variable density undersampling scheme and reconstructing the missing data points with multiple neural networks, this method enables a more robust reconstruction of highly undersampled data. High resolution metabolite maps acquired at 9.4T in the human brain using the proposed method are presented.
|
|
3376.
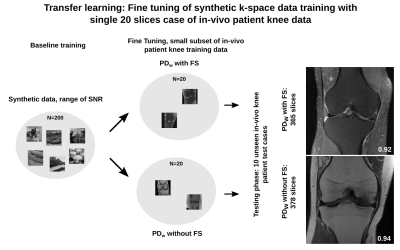 |
63 |
Assessment of the generalization of learned image reconstruction and the potential for transfer learning
Florian Knoll, Kerstin Hammernik, Thomas Pock, Daniel Sodickson, Michael Recht
The goal of this study is to assess the influence of image contrast, SNR and image content on the generalization of machine learning in MR image reconstruction. Experiments are performed with patient data from clinical knee MR exams as well as synthetic data created from a public image database. It shows that while SNR is a critical parameter, trainings can be generalized towards a range of SNR values. It also demonstrates that transfer learning can be used successfully to fine-tune trainings from synthetic data to a particular target application using only a very small number of training cases.
|
|
3377.
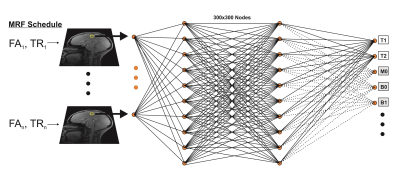 |
64 |
Characterization of Sparsely Trained Deep Learning Reconstruction of Noisy MR Fingerprinting Data
Ouri Cohen, Bo Zhu, Matthew Rosen
MR Fingerprinting offers the ability to obtain simultaneous tissue (T1,T2…) and hardware (B1, B0…) parameter maps in a fast acquisition time but is limited by the size of the reconstruction dictionary. In previous work we demonstrated that these issues can be overcome by reconstructing the data using a properly trained neural network. Here we characterize the accuracy of a neural network trained on sparse dictionaries for reconstruction of noisy data.
|
|
3378.
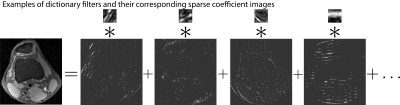 |
65 |
k-space Aware Convolutional Sparse Coding: Learning from Undersampled k-space Datasets for Reconstruction
Frank Ong, Michael Lustig
Learning from existing datasets has the potential to improve reconstruction quality. However, deep learning based methods typically require many clean fully-sampled datasets as ground truths. Such datasets can be hard to come by, especially in applications where rapid scans are desired. Here, we propose a method based on convolutional sparse coding that can learn a convolutional dictionary from under-sampled datasets for sparse reconstruction. Recent works have shown close connections between deep learning and convolutional sparse coding. The benefit of convolutional sparse coding is that it has a well-defined forward model, and can be easily extended to incorporate physical models during training. We extend convolutional sparse coding to incorporate the under-sampling forward model. We show that the dictionary learned from under-sampled datasets is similar to the dictionary learned from fully-sampled datasets, and improves upon wavelet transform for l1 regularized reconstruction in terms of mean-squared error.
|
|
3379.
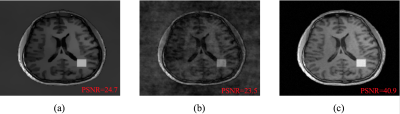 |
66 |
Constrained Image Reconstruction Using a Kernel+Sparse Model
Yudu Li, Zhi-Pei Liang
Constrained image reconstruction incorporating prior information has been widely used to overcome the ill-posedness of reconstruction problems. In this work, we propose a novel "kernel+sparse" model for constrained image reconstruction. This model represents the desired image as a function of features "learned" from prior images plus a sparse component that captures localized novel features. The proposed method has been validated using multiple MR applications as example. It may prove useful for solving a range of image reconstruction problems in various MR applications where both prior information and localized novel features exist.
|
|
3380.
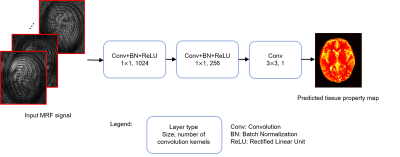 |
67 |
Quantification of relaxation times in MR Fingerprinting using deep learning
Zhenghan Fang, Yong Chen, Weili Lin, Dinggang Shen
MRF is a new quantitative MR imaging technique, which can provide rapid and simultaneous measurement of multiple tissue properties. Compared to the fast speed for data acquisition, the post-processing to extract tissue properties with MRF is relatively slow and often requires a large memory for the storage of both image dataset and MRF dictionary. In this study, a convolutional neural network was developed, which can provide rapid estimation of multiple tissue properties in 0.1 sec. The T1 and T2 values obtained in white matter and gray matter are also in a good agreement with the results from pattern matching.
|
|
3381.
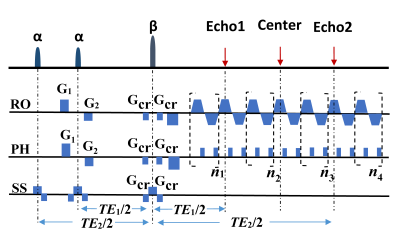 |
68 |
High efficient reconstruction of overlapping-echo detachment (OLED) planar imaging based on deep residual network
Congbo Cai, Chao Wang, Xinghao Ding, Shuhui Cai, Zhong Chen, Jianhui Zhong
Overlapping-echo detachment (OLED) planar imaging sequence can provide reliable T2 mapping within milliseconds even under continuous object motion. A detachment algorithm based on the sparsity and structure similarity constraints has been used to separate the echo signals to form T2 map. However, the effectiveness of separation is limited and the reconstruction is time consuming. Here, an end-to-end deep convolutional network based on deep residual network was introduced. The results of simulation and in vivo human brain show that it can reconstruct T2 mapping efficiently and reduce the reconstruction time from minutes to milliseconds after deep residual network is trained.
|
|
3382.
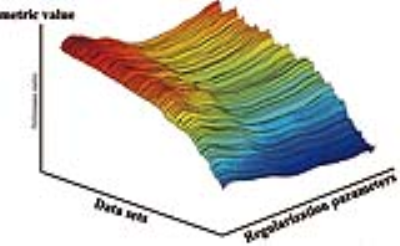 |
69 |
Optimal Regularization Parameter Selection for Constrained Reconstruction Using Deep Learning
Xi Peng, Fan Lam, Yudu Li, Bryan Clifford, Brad Sutton, Zhi-Pei Liang
Regularization is widely used for solving ill-posed image reconstruction problems and an appropriate selection of the regularization parameter is critical in ensuring high-quality reconstructions. While many methods have been proposed to address this problem, selecting a regularization parameter for optimal performance (under a specific metric) in a computationally efficient manner is still an open problem. We propose here a novel deep learning based method for regularization parameter selection. Specifically, a convolutional neural network is designed to predict the optimal parameter from an “arbitrary” initial parameter choice. The proposed method has been evaluated using experimental data, demonstrating its capability to learn the optimal parameter for two different L1-regularized reconstruction problems.
|
|
3383.
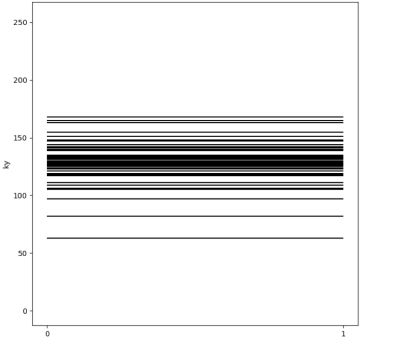 |
70 |
A Machine Learning Approach for Mitigating Artifacts in Fetal Imaging due to an Undersampled HASTE Sequence
Sayeri Lala, Borjan Gagoski, Jeffrey Stout, Bo Zhao, Berkin Bilgic, Ellen Grant, Polina Golland, Elfar Adalsteinsson
This work investigates using deep learning to mitigate artifacts in fetal images resulting from accelerated acquisitions. We applied an existing deep learning framework to reconstruct undersampled HASTE images of the fetus. The deep learning architecture is a cascade of two convolutional neural networks combined with data consistency layers. Training and evaluation were performed on coil-combined and reconstructed HASTE images with retrospective undersampling. The datasets derived from imaging of ten pregnant subjects, GA 19-37 weeks, yielding 3994 HASTE slices. This approach mitigates artifacts from incoherent aliasing with residual reconstruction errors in high spatial frequency features in the phase encoding direction.
|
|
3384.
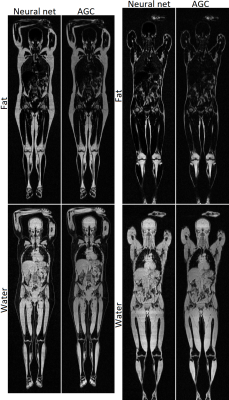 |
71 |
Single Point Dixon Reconstruction of Whole-Body Scans Using a Convolutional Neural Network
Jonathan Andersson, Håkan Ahlström, Joel Kullberg
Reconstructions of water and fat images are clinically useful for removing obscuring fat signal. It can also be useful in for example obesity related research, measuring for example different adipose depot volumes. Normally reconstructions would be performed using at least two echos, which requires about twice as much time as collecting a single echo. Therefore, using only a single echo would reduce the required scan time drastically. In this abstract a method for reconstruction of water and fat images from a single echo is introduced, using a convolutional neural network. We conclude from visual evaluation that the results are promising.
|
|
3385.
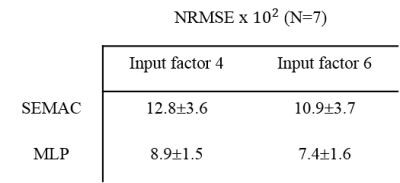 |
72 |
Artificial Neural Network for Suppression of Metal Artifacts with Slice Encoding for Metal Artifact Correction (SEMAC) MRI
Sunghun Seo, Ki Hwan Kim, Seung Hong Choi, Sung-Hong Park
We present a new method of artificial neural network (ANN) to suppress metal artifacts in MR Imaging with Slice Encoding for Metal Artifact Correction (SEMAC). Seven titanium-embedded phantoms were imaged using different SEMAC factors. The acquired data with low and high SEMAC factors were separated into input and label images, respectively, for training. The trained model was tested on separate phantoms. Metal artifacts in low SEMAC factors could be further suppressed visually and quantitatively using the implemented ANN, with the performance being comparable to that of label images. The proposed method reduces scan time necessary for high-quality SEMAC imaging.
|
|
RF Pulses & Sequences
Electronic Poster
Acquisition, Reconstruction & Analysis
Monday, 18 June 2018
Exhibition Hall |
13:45 - 14:45 |
|
|
Computer # |
|
3386.
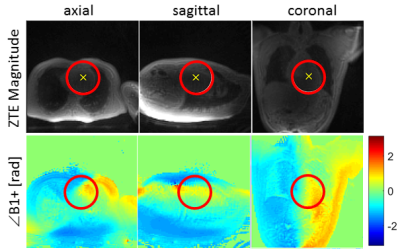 |
73 |
Cardiac B1+ Shimming using ZTE Transmit Phase Mapping
Rolf Schulte, Haonan Wang, Anja Brau, Martin Janich
Two different transmit B1+ mapping techniques were implemented and investigated for cardiac B1+ shimming at 3T: (1) 2D cardiac-triggered spiral-Bloch-Siegert B1+ mapping; (2) 3D Zero-Echo-Time (ZTE) B1+ phase mapping. B1+ homogeneity was optimised and performance assessed by evaluating the cardiac black-blood fast spin-echo sequence performance in healthy volunteers.
|
|
3387.
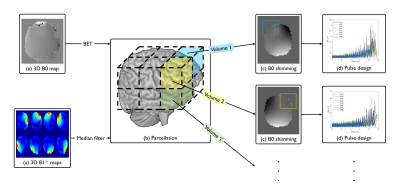 |
74 |
Parcellated shimming for brain imaging with 3D EPI at 7T
Christopher Mirfin, Simon Shah, Paul Glover, Richard Bowtell
A novel acquisition strategy to improve the overall B0 field homogeneity by utilising 2D RF selection with acceleration via parallel transmission in conjunction with parcellated sub-volume shimming is proposed. The method has been demonstrated for brain imaging using a 3D EPI sequence at 7T.
|
|
3388.
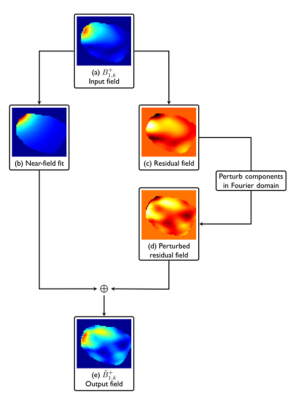 |
75 |
Optimisation of parallel transmission radiofrequency pulses using neural networks
Christopher Mirfin, Paul Glover, Richard Bowtell
Developing fast accurate large-tip-angle radiofrequency pulses and gradient trajectories suitable for ‘online’ use is a challenging problem. In this work we propose a novel method for the sub-second design of RF pulses and gradient trajectories through use of a suitably trained artificial neural network which attempts to learn the required pulse and gradient spoke parameters from B1+ field spatial variations. A method for synthesising a large training database is also described. Our initial results highlight some of the challenges of this approach but suggest areas for future development.
|
|
3389.
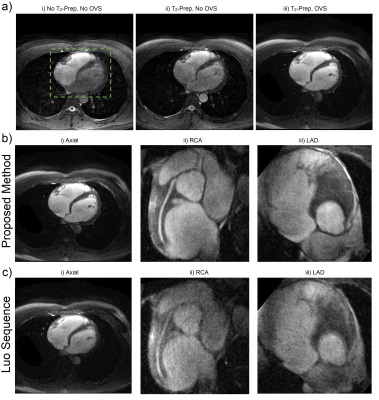 |
76 |
Empirical Sequence Design for Combined T2-Preparation and Outer Volume Suppression Preparation Sequence
David Zeng, Mario Malavé, Corey Baron, Adam Kerr, Phillip Yang, Bob Hu, Dwight Nishimura
A new combined T2-prepared, multidimensional OVS pulse sequence was designed by an empirically-driven method. We defined a T2-prepared OVS module as a tip-down pulse, refocusing sequence, and a selective tip-up pulse. Candidate pulses were proposed for each portion of the module and all possible modules were evaluated by a metric based on Bloch simulations. Multidimensional OVS was achieved by concatenation of modules. The proposed sequence had the lowest metric and was compared against an existing T2-prepared OVS sequence for in vivo (n=5) coronary imaging. The proposed sequence had superior vessel sharpness, SNR, OVS, and qualitative reader scores.
|
|
3390.
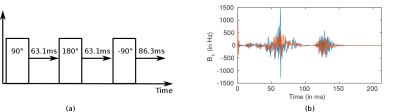 |
77 |
Contrast Preparation Pulses Robust to B1 and B0 Inhomogeneities: an Optimal Control Approach
Eric Van Reeth, Hélène Ratiney, Kevin Tse Ve Koon, Michael Tesch, Denis Grenier, Olivier Beuf, Steffen Glaser, Dominique Sugny
This abstract proposes an optimal control strategy for the computation of contrast preparation pulses robust to B1 (+/- 35%) and B0 (+/- 400Hz) inhomogeneities. The problem formulation allows to optimize the compromise between contrast performance and preparation time. An in vitro short-T2 enhancing contrast experiment validates the robustness superiority of the proposed preparation compared to a block pulse-based scheme, and shows a good match with simulations.
|
|
3391.
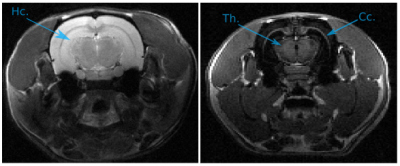 |
78 |
A Simplified Framework for Contrast Optimization in MRI
Eric Van Reeth, Hélène Ratiney, Kevin Tse Ve Koon, Michael Tesch, Denis Grenier, Olivier Beuf, Steffen Glaser, Dominique Sugny
This abstract details the implementation and interest of an adapted parameterization for the computation of contrast preparation schemes in an optimal control framework. It optimally balances the effect of T1 and T2 relaxation, penalizes long preparation sequences in order to improve the compromise between contrast performance and preparation time, and significantly reduces the computation time. As an example, an in vitro experiment validates the contrast benefit over an inversion-recovery scheme. Finally, it offers a huge flexibility in terms of achievable contrasts, which is demonstrated in vivo by a white-matter enhancement experiment on a rat brain.
|
|
3392.
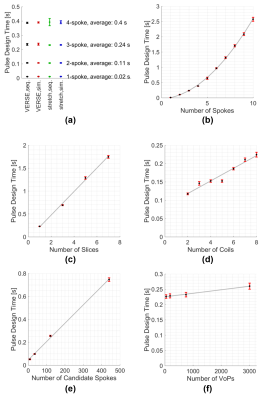 |
79 |
Near real-time parallel-transmit pulse design
Emre Kopanoglu
With many MRI scans lasting several minutes, patient motion is a common problem, especially with uncooperative subjects such as paediatric patients or patients with dementia or Parkinson’s. Realizing the finer-resolution that higher field strengths offer through the availability of increased SNR necessitates even longer scans, exacerbating this problem. While prospective motion correction techniques can compensate for motion at lower field strengths, such techniques are not directly applicable at higher field strengths, when more complicated parallel-transmit pulses are used. This study proposes a pulse design technique that can design multi-spoke and simultaneous multi-slice parallel-transmit pulses in less than one second, while adhering to peak-voltage limits, local and global SAR.
|
|
3393.
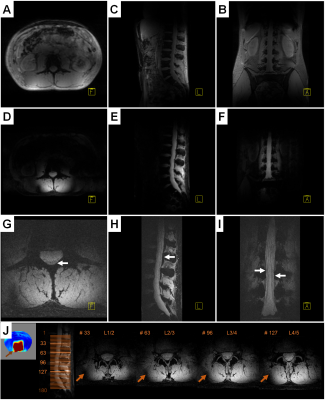 |
80 |
16-channel pTx body MRI for reduced field of view lumbar spine and kidney imaging at 7 Tesla
Sascha Brunheim, Stephan Orzada, Sören Johst, Marcel Gratz, Jessica Kohl, Mark Ladd, Harald Quick
In this work we present in-vivo pTx excitation results in the low flip angle regime with a 16-channel transceiver body array at 7 Tesla. The pTx pulse calculation was based on a jointly fast acquired B0 and single-channel B1+ dataset (B01TIAMO) of the central abdomen. The pTx pulse enabled us to acquire high-resolution reduced field of view images of the distal spinal cord and the unilateral left kidney. The results of the established workflow for abdominal pTx provide promising perspectives, especially for neuroradiological spine imaging.
|
|
3394.
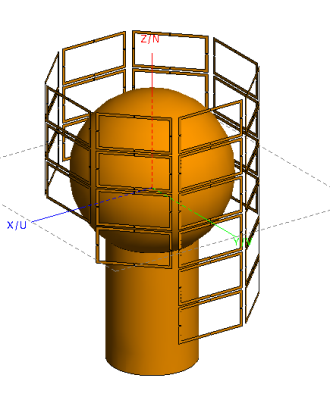 |
81 |
pTx-PINS pulses for simultaneous multislice excitation using 32 ch Tx array and insertable head gradient
Mihir Pendse, Riccardo Stara, Joshua deBever, Brian Rutt
We describe a method for combining the PINS method for SMS excitation with pTx to design pulses that achieve both good B1+ uniformity (15% inhomogeneity over whole volume) and significant multiband factors (MB = 16) at ultra high field strengths (7T, 10.5T). This is enabled by the use of advanced hardware including a 6-row 32 channel parallel transmit array and a high performance head gradient (130 mT/m strength, 1500 T/m/s slew rate). We optimized RF shimming over the whole volume and applied the optimized shim weights at each point in the PINS trajectory. We satisfied very demanding pulse requirements (0.4 mm slice thickness, MB=16, total flip angle inhomogeneity = 15%) with a practical pulse duration (<12 ms) which is >2 times shorter compared to using conventional hardware
|
|
3395.
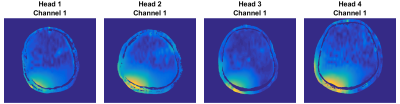 |
82 |
Universal Parallel Transmit Pulse Design for Local Excitation
Ole Geldschläger, Tingting Shao, Anke Henning
This study investigates different parallel transmission (PTx) pulse design methods to find a universal PTx-pulse that excites the same local pattern with a 90 degree flip-angle across different heads. Thus, it abandons prospective the need for time-consuming subject specific B1+mapping and PTx-pulse calculation, during the scan session. The best results were achieved by solving a minimax optimization problem were the maximum normalized root mean square error (NRMSE) over all subjects was minimized. The resulting pulse created magnetization profiles with a maximum NRMSE of around 0.049 across all volunteers.
|
|
3396.
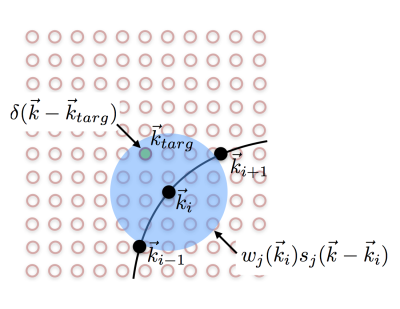 |
83 |
k-Space Domain Parallel Transmit Pulse Design
William Grissom
Current parallel transmit pulse design methods are based on a spatial domain formulation that has prohibitive memory and computational requirements when the number of coils or the number of dimensions is large. We describe a k-space domain parallel transmit pulse design method that directly solves for the columns of a sparse design matrix with a much smaller memory footprint than existing methods, and is highly parallelizable. The method is validated with phantom and in vivo 7T 8-channel spiral excitations.
|
|
3397.
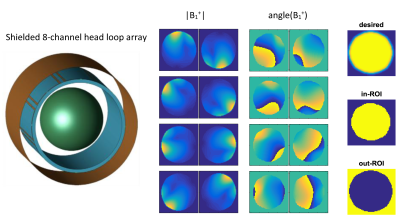 |
84 |
Optimal control based design of parallel transmission RF pulses with minimum local SAR
Armin Rund, Christoph Aigner, Lena Nohava, Roberta Frass-Kriegl, Elmar Laistler, Karl Kunisch, Rudolf Stollberger
An optimal control framework for designing parallel transmission RF pulses and gradient shapes is introduced. The optimal control model includes technical constraints and a local SAR model based on the Q-matrix formalism. Second-order optimization methods give RF pulses with enhanced homogeneity of the excitation pattern and/or decreased local SAR. The optimized results are tested in numerical experiments and validated with numerical electromagnetic simulations.
|
|
3398.
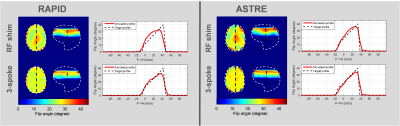 |
85 |
Z-segmentation of a transmit array head coil improves RF ramp pulse design for TOF MRA at 7T
Gaël Saïb, Raphaël Tomi-Tricot, Franck Mauconduit, Vincent Gras, Nicolas Boulant, Alexandre Vignaud, Edouard Chazel, Eric Giacomini, Guillaume Ferrand, Michel Luong, Denis Le Bihan, Laurent Le Brusquet, Alexis Amadon
In Time-Of-Flight sequences, ramp pulses such as TONE are frequently used to compensate for thru-slab blood saturation in cerebral MRA. At Ultra High Field, parallel transmit fast-kz spokes can be used to greatly mitigate B1+ heterogeneities in the slab selection process. Here we use this technique to design TONE pulses with improved flip angle ramp fidelity and compare the performance achieved with a homemade z-segmented head coil versus a non-segmented commercial array.
|
|
3399.
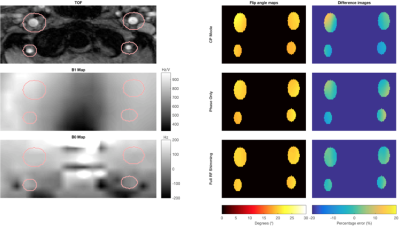 |
86 |
Improving arterial spin labelling at ultra-high field using parallel transmission: a simulation study
Yan Tong, Peter Jezzard, Thomas Okell, William Clarke
Implementing ASL at ultra-high field is challenging due to increased B1+ and B0 inhomogeneity. Parallel transmission (pTx) provides additional degrees of freedom to mitigate B1+ inhomogeneity. Among various pTx strategies, RF shimming is a simple formulation that modulates the complex weights of each RF channel. RF shimming is particularly robust for applications involving small regions-of-interest. In this study, we explored the possibility of using RF shimming for ASL via simulation, and RF shimming is shown to achieve improved lower NRSME and improved labeling homogeneity over CP mode through simulation.
|
|
3400.
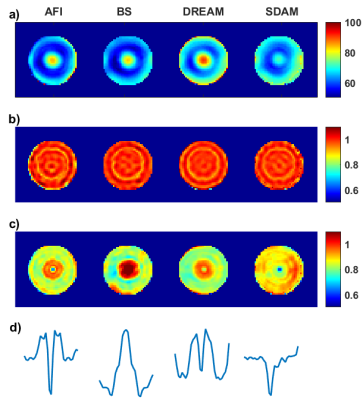 |
87 |
Investigating the effect of B1 map inaccuracies on advanced pulse design
Marjolein Piek, Nam Lee, Anouk Marsman, Vincent Boer, Esben Petersen
B1 inhomogeneities at high field lead to undesired variation of contrast over the images. With advanced RF pulse design, the effect of B1 inhomogeneities on the excitation pattern can be restored. Bloch simulations in combination with advanced pulse design were performed to study the B1 mapping robustness. The results show that there is relatively high variation between four well established B1 mapping methods. From the results it is clear that next to acquisition speed and SNR, the robustness of B1 estimation is also an important factor if the B1 mapping is to be used for advanced RF pulse design.
|
|
3401.
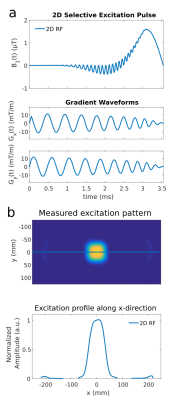 |
88 |
2D selective excitation with UNFOLD for 4D Flow Imaging
Clarissa Wink, Jean Pierre Bassenge, Giulio Ferrazzi, Sebastian Schmitter
4D flow MRI allows to quantify the velocity vector field non-invasively in-vivo. However, it still suffers from long acquisition times and low temporal and spatial resolution. Here, we accelerate acquisition time and increase temporal resolution without loss of spatial resolution by combining 2D selective excitation and UNFOLD. 2D selective excitation allows to limit the field-of-view in phase encoding direction and thus acquisition time, whereas UNFOLD grants to increase temporal resolution.
|
|
3402.
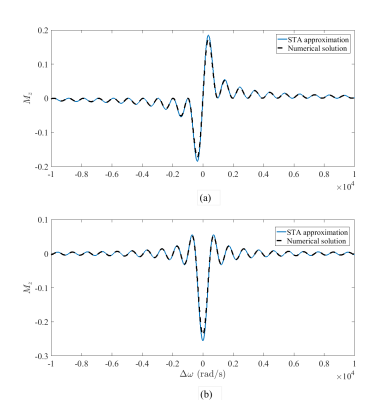 |
89 |
Extending the small tip angle approximation to the non-equilibrium initial condition
Bahman Tahayori, Zhaolin Chen, Gary Egan, N. Jon Shah
We have applied Volterra series expansion to the Bloch equation and have calculated the kernels for an arbitrary initial condition. We have shown that small tip angle approximation can be extended to the non-equilibrium initial condition. Simulation results illustrated the validity of the extended small tip angle approximation.
|
|
3403.
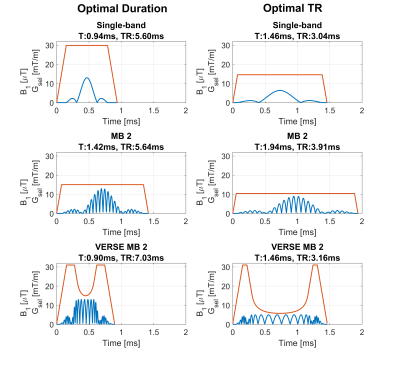 |
90 |
Minimum-TR pulse design for rapid gradient echo sequences
Samy Abo Seada, Arian Beqiri, Anthony Price, Jo Hajnal, Shaihan Malik
Use of Multiband and high-TBW RF pulses in SSFP sequences is limited due to their long pulse duration and high RF energy. These two properties are not independent of each other, as shorter RF pulses lead to higher RF energy and often violate SAR limitations when using short repetition times (TR). We show how Time-optimal VERSE can be used to produce pulses that are optimized for minimum TR performance with both single and multi-band examples.
|
|
3404.
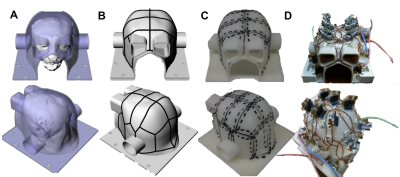 |
91 |
Translating the Human Connectome Project to Marmoset Imaging: 16-Channel Multi-Array Coil and HCP-Style MRI Protocols and Preprocessing
Yuki Hori, Joonas Autio, Masahiro Ohno, Yoshihiko Kawabata, Yuta Urushibata, Katsutoshi Murata, Masataka Yamaguchi, Akihiro Kawasaki, Chiho Takeda, Chihiro Yokoyama, Matthew Glasser, Takuya Hayashi
The common marmoset is increasingly used as a non-human primate model to understand the organization of the brain. Better cross species comparisons can be achieved by adapting methods from the Human Connectome Project. Here, we show a customized 16-channel receiver coil designed for the marmoset brain and present the initial imaging results on a 3T MRI scanner with powerful gradients. The coil had high signal-to-noise ratio and B1 transmit homogeneity. In-vivo marmoset data, acquired and preprocessed using HCP-style methods, provided high-resolution images, allowing cortical mapping of myelin, thickness, and structural and functional connectivity, enabling high quality cross-species comparisons.
|
|
3405.
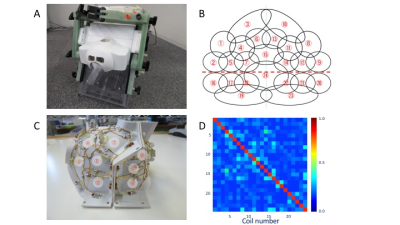 |
92 |
Development of a 24-Channel 3T Multi-Array Coil for functional MRI in awake monkeys
Atsushi Yoshida, Yoki Hori, Kantaro Nishigori, Masahiro Ohno, Yoshihiko Kawabata, Yuta Urushibata, Katsutoshi Murata, Masataka Yamaguchi, Joonas Autio, Matthew Glasser, Takuya Hayashi
High-resolution fMRI in awake macaques may address compelling questions for how the brain is dynamically organized to create behaviors. Here, we developed a 24-channel multi-array receive coil for awake macaques and a 3T MRI scanner. High performance of the coil was confirmed by assessing noise correlation, B0/B1 field and SNR. Preliminary resting-state fMRI data, preprocessed with the Human Connectome Project pipeline, revealed a number of functional network components, some of which replicated previous findings. Our system may be useful for multi-modal cortical mapping of task-dependent and resting functional activity.
|
|
3406.
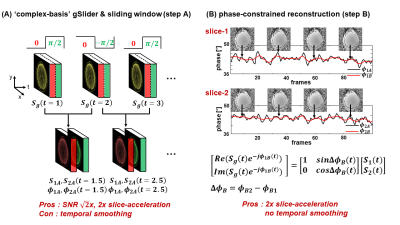 |
93 |
Accelerated spin-echo fMRI using generalized SLIce Dithered Enhanced Resolution Simultaneous MultiSlice (gSlider-SMS) with 'complex-basis' RF-encoding
SoHyun Han, Congyu Liao, Mary Kate Manhard, Berkin Bilgic, Fuyixue Wang, Anna I. Blazejewska, Maaike van den Boomen, William Grissom, Jonathan R. Polimeni, Kawin Setsompop
High spatiotemporal resolution spin-echo (SE) fMRI acquisition is challenging due to the longer repetition times (TR) compared to conventional gradient-echo (GE) fMRI. In this study, we developed a new method, dubbed ‘complex-basis’ gSlider, which utilizes the spatiotemporal phase-smoothness of SE-fMRI time frames to accelerate the slice coverage of SE-fMRI acquisitions. We further combined ‘complex-basis’ gSlider with conventional SMS to boost the slice-acceleration as well. The proposed method showed comparable tSNR and a two-fold increase in slice-acceleration when compared with standard SE-SMS-EPI. This method would be beneficial for applications requiring high resolution SE-fMRI with whole-brain coverage
|
|
3407.
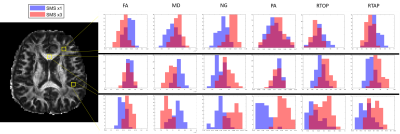 |
94 |
A Bootstrap Analysis of Diffusion MRI Parameters Derived from Simultaneous Multislice Diffusion MRI
Adam Bernstein, Loi Do, Nan-kuei Chen, Theodore Trouard
In this study, we design a unique bootstrapping method to approximate the distributions of diffusion MRI parameters derived from scans that utilize simultaneous multislice techniques compared to the distribution of parameters fit from a single slice EPI sequence. While there are no statistically significant differences between accelerated and non-accelerated datasets, there are some subtle differences that may warrant closer inspection.
|
|
3408.
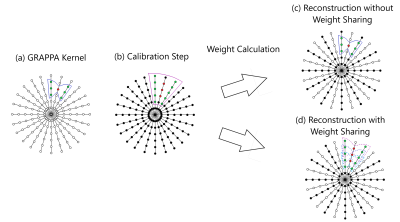 |
95 |
Sharing Radial GRAPPA Weight Sets Across k-Space to Decrease Memory Requirements for Real-Time Imaging
Evan Cummings, Dominique Franson, Jesse Hamilton, Nicole Seiberlich
This study examines the feasibility of reconstructing multiple neighboring k-space points from a single non-Cartesian GRAPPA weight set. This approach reduces both the time to calibrate the GRAPPA weights and the memory needed to store the weights with minimal loss of image quality in the reconstructed images.
|
|
Image Analysis
Electronic Poster
Acquisition, Reconstruction & Analysis
Monday, 18 June 2018
Exhibition Hall |
13:45 - 14:45 |
|
|
Computer # |
|
3409.
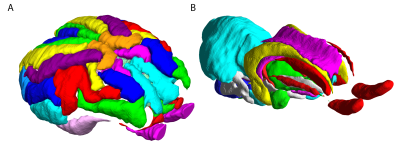 |
97 |
The average sheep: multi-modal population atlases and variability maps
Stephen Sawiak, Nicholas Perentos, Lucy Johnson, A Jennifer Morton
We present a sheep atlas from 160 subjects from high-resolution MRI images. Aided by histology, cortical and subcortical regions were labelled for surgical planning and anatomical localisation. Templates for voxel-based morphometry were produced for SPM/DARTEL approaches. To demonstrate the use of the atlas and software, we analysed post mortem volumetric changes in repeatedly scanned brains from in vivo to 12 weeks post mortem.
|
|
3410.
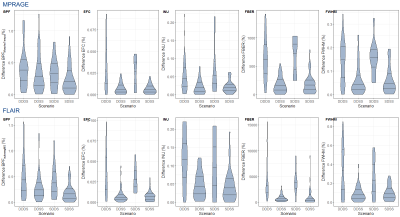 |
98 |
Assessing the Relation between Image Quality Metrics and Brain Volume in a Scan-Rescan Dataset
Ricardo Corredor-Jerez, Jonas Richiardi, Mário Fartaria, Bénédicte Maréchal, Adrian Tsang, Robert Bermel, Stephen Jones, Izlem Izbudak, Ellen Mowry, Yvonne Lui, Lauren Krupp, Elizabeth Fisher, Tobias Kober
Satisfactory image quality is essential to accurately assess brain volume using automated methods for evaluating neurodegenerative diseases. Variations in image quality may cause volume estimation errors hard to distinguish from disease-induced changes. We studied the relationship between brain volume estimations and image quality metrics in a scan-rescan study. Two segmentation methods were used to quantify brain volume in FLAIR and MPRAGE images. Volume estimations on MPRAGE varied less with hardware, compared to the estimations on FLAIR. We found a significant correlation between hardware and several image quality metrics, suggesting that these can be used to render volume estimations more hardware-independent.
|
|
3411.
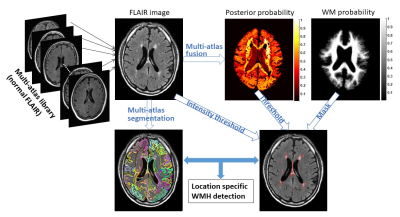 |
99 |
Multi-atlas based Detection and Localization (MADL) of White Matter Hyperintensities: Relationship with Amyloid Accumulation and Vascular Risks
Dan Wu, Kenichi Oishi, Anja Soldan, Corinne Pettigrew, Chenfei Ye, Michael Miller, Marilyn Albert, Susumu Mori
Recent findings suggest white matter hyperintensities (WMH) that appear on FLAIR images may play a role in the evolution of Alzheimer’s disease (AD). Here, we developed a novel algorithm that simultaneously detects and locates WMH, based on a FLAIR atlas database and a multi-atlas fusion algorithm. The method showed a respectful WMH detection accuracy. We also investigated region-specific WMH load in participants for whom amyloid imaging and vascular data were available. The results suggested that posterior WMH is related to amyloid deposition; whereas anterior and parietal WMH is associated with vascular risk factors.
|
|
3412.
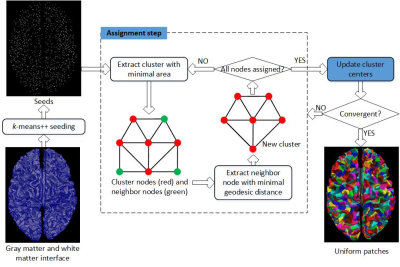 |
100 |
Surface Uniform Random Partition for Atlas-free Brain Network Analysis
Teng Zhang, Pan Sun, Lin Shi, Queenie Chan, Defeng Wang
Random partition is the cornerstone of atlas-free brain network analysis which can be used for multiscale analysis and comparison of cohorts with different brain sizes. The random parcels should be uniform to avoid additional variability from different parcel areas. In this study a uniform random partition of meshed surface is proposed considering geodesic distances and parcel areas. The partition results showed that proposed method can partition surface into any given number of parcels with similar areas. With repeating network analysis using proposed uniform parcels, results showed low intra-subject variations of global network measures.
|
|
3413.
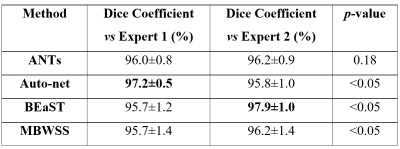 |
101 |
Can brain MRI skull-stripping methods be further improved using manual segmentation as ground-truth for validation?
Roberto Souza, Oeslle Lucena, Letícia Rittner, Roberto Lotufo, Richard Frayne
Automatic skull-stripping (SS) methods have reached a high level of accuracy compared to expert manual segmentation (typically defined as the “ground-truth”), but SS is still an active research area with many methods being proposed every year. In this work, we use twelve T1-weighted brain magnetic resonance (MR) images with each image having two different manual segmentations performed by experts, and four state-of-the-art SS methods to assess if it is possible to evaluate further accuracy improvements to SS. Our results indicate that at the current level of SS accuracy, this is not possible using single expert manual segmentation.
|
|
3414.
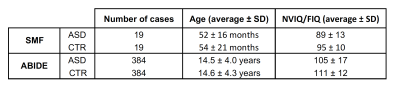 |
102 |
Lifespan study by cross-sectional case-control comparisons in sliding age windows: test of ASD heterogeneity with One-Class Classifiers
Piernicola Oliva, Alessia Giuliano, Paolo Bosco, Elisa Ferrari, Michela Tosetti, Filippo Muratori, Calderoni Sara, Alessandra Retico
Cross-sectional studies reported inconsistent findings on distinctive neuroanatomical characteristics of Autism Spectrum Disorders (ASD). We set up a lifespan study through a series of machine-learning-based case-control comparisons made on sub-cohorts obtained by partitioning a large structural MRI data sample (age range: 2-25 years) in subsamples with partially-overlapping narrower age ranges (3-4 years). We implemented One-Class Support Vector Machines on these sub-cohorts, obtaining the temporal evolution of the case-control separation ability, which is related to the detectability of neuroimaging-based biomarkers. Distinctive common features characterize children with ASD under 5 years of age; the heterogeneity of the ASD condition dominates from adolescence.
|
|
3415.
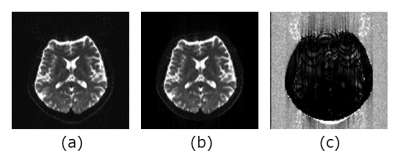 |
103 |
An image-based method for undistorted image estimation from distorted brain EPI image with field inhomogeneity
Seiji Kumazawa, Takashi Yoshiura, Takumi Tanikawa, Yuji Yaegashi
Our purpose was to develop an image-based method for undistorted image estimation from the distorted EPI image using T1 weighted image. Our basic idea to estimate the field inhomogeneity map is to reproduce the distorted EPI image, and estimates the undistorted image using the estimated field inhomogeneity map based on the signal equation in a single-shot EPI k-space trajectory. The value of the NRMSE between the measured EPI and synthesized EPI was 0.017, and both images were in good agreement. Results demonstrate that our proposed method was able to perform a reasonable estimation of the field inhomogeneity map and undistorted EPI image.
|
|
3416.
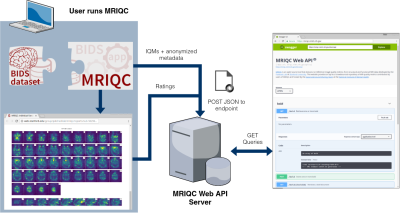 |
104 |
MRIQC Web-API: Crowdsourcing image quality metrics and expert quality ratings of structural and functional MRI
Oscar Esteban, Ross Blair, Dylan Nielson, Jan Varada, Sean Marrett, Adam Thomas, Russell Poldrack, Krzysztof Gorgolewski
The MRIQC Web-API is a resource for scientists to train new automatic quality classifiers. The MRIQC Web-API has collected more than 30K sets of image quality measures automatically extracted from BOLD and T1-weighted scans using MRIQC. MRIQC is an automated MRI Quality Control tool, and here we present an extension to crowdsource these quality metrics along with anonymized metadata and manual quality ratings. This new resource will allow a better understanding of the normative values and distributions of these quality metrics, help determine the relationships between image quality and metadata such as acquisition parameters and finally, provide a cost-effective, easy way to annotate the quality of a large number of cross-site MR scans.
|
|
3417.
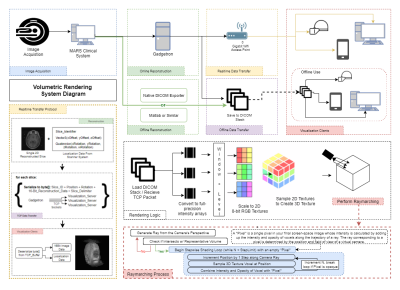 |
105 |
Collaborative volumetric magnetic resonance image rendering on consumer-grade devices
Andrew Dupuis, Dominique Franson, Yun Jiang, Jeff Mlakar, Henry Eastman, Vikas Gulani, Nicole Seiberlich, Mark Griswold
We present a system for intra- or post-acquisition 3D rendering of volumetric MRI datasets for independent or collaborative use on AR/VR and mobile platforms. Consumer-grade head mounted displays, phones, and computers are used to provide 3D visualizations. Datasets can be windowed and leveled in the same manner as classic visualizations, and arbitrary slices can be selected and viewed in real time in the context of the whole volume. Real world dimensionality and spatialization is retained. Using this system, multiple users can interact with a dataset collaboratively using current AR/VR platforms or any modern cellphone, tablet, or laptop.
|
|
3418.
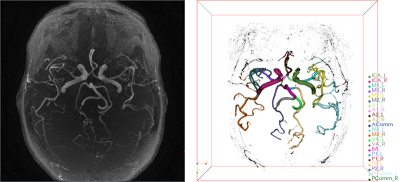 |
106 |
Quantification of Morphometry and Intensity Features of Intracranial Arteries from 3D TOF MRA: A Reproducibility Study
Li Chen, Mahmud Mossa-Basha , Daniel Hippe , Jie Sun, Niranjan Balu, Kristi Pimentel, Thomas Hatsukami, Jenq-Neng Hwang, Chun Yuan
The aim is to evaluate the reproducibility of intracranial artery feature extraction (iCafe) technique for quantitative analysis of intracranial arteries from 3D time-of-flight (TOF) magnetic resonance angiography (MRA). Twenty-four patients with known intracranial artery stenosis were recruited and underwent two separate MRA scans within 2 weeks. Each dataset was processed blindly using iCafe. Eight morphometry and intensity features were acquired from each artery. The inter-scan reproducibility of iCafe was excellent with intra-class correlation coefficients between 0.92-0.98 and within-subject coefficients of variation between 3.2-8.6% across all features, showing iCafe is a reliable technique for intracranial artery feature quantification from TOF MRA.
|
|
3419.
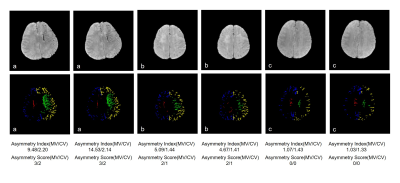 |
107 |
An automatic system for asymmetry vein analysis in patients with acute ischemic stroke
Hanjing Kong, Wenjian Huang, Mei Yang, Weihai Xu, Yining Huang, Jue Zhang
Asymmetry veins in patients with acute ischemic stroke are associated with infarct growth and clinical outcome. However, due to the lack of effective segmentation and quantification methods, these studies focus only on medullary or cortical veins. In this study, an automatic image processing system was developed for asymmetry analysis in medullary and cortical veins using the magnitude data of SWI.
|
|
3420.
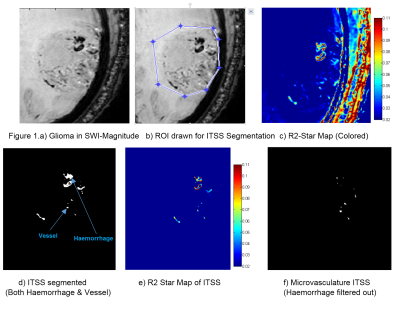 |
108 |
Quantitative Micro-Vasculature Volume Assessment of Intra Tumoral Susceptibility Signal (ITSS) in differentiating Grade-III from IV glioma
Rupsa Bhattacharjee, Prashant Budania, Pradeep Kumar Gupta, Rakesh Kumar Gupta, Sunita Ahlawat, Anup Singh
Angiogenesis transforms gliomas from low-to-high-grade. Vasculature-properties are of essential prognostic-value within grade-III and IV glioma as compared to grade-II. High-resolution susceptibility-weighted imaging (SWI) improves the diagnostic accuracy1. Existing Semi-quantitative methods are user-dependent which manually counts intra-tumoral-susceptibility-signal-intensities (ITSS); a combination of haemorrhage and vasculature. Haemorrhage contributes to false ITSS-count and subsequently to misclassification of tumor-grading. We propose a non-invasive segmentation-based-quantitative approach that calculates the R2-Star relaxivity maps of ITSS, automatically removes haemorrhages from ITSS based on high-R2-Star relaxivity of haemorrhage and finally calculate microvasculature volume within glioma. The proposed-method scores over the existing semi-quantitative method in-terms-of ITSS-estimation and grading-accuracy.
|
|
3421.
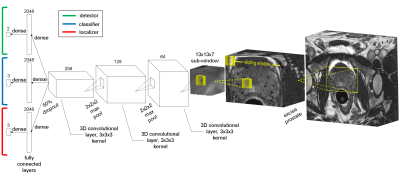 |
109 |
SeedNet: a sliding-window convolutional neural network for radioactive seed detection and localization in MRI
Jeremiah Sanders, Steven Frank, Jingfei Ma
Radioactive seed localization is an essential step in quantifying the dose delivered to the prostate and surrounding anatomy after low-dose-rate prostate cancer brachytherapy. Currently, dosimetrists spend hours manually localizing the radioactive seeds in postoperative images. In this work, we investigated a novel sliding-window convolutional neural network approach for automatically identifying and localizing the seeds in MR images. The method doesn’t rely on prior knowledge of the number of seeds implanted, strand placements, or needle-loading configurations. In initial testing, the proposed approach achieved a recall of 100%, precision of 97%, and processing time of ~0.5-1.5 minutes per patient.
|
|
3422.
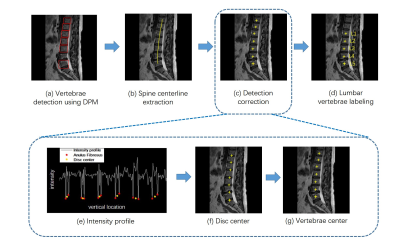 |
110 |
An improved automatic localization method for abnormal lumbar vertebrae using MR Images
Fei Gao, Shui Liu, Xiaodong Zhang, Jue Zhang, Xiaoying Wang, Jing Fang
In this study, we provide an automatic lumbar localization method efficient for abnormal vertebrae based on the local context information of lumbar MR images. The localization results indicate the efficiency of the proposed method for lumbar vertebrae with various abnormalities.
|
|
3423.
 |
111 |
An objective tool for diagnosing Prostate Cancer and Benign Prostatic Hyperplasia: Radiomics Featuresextracted from Diffusion-weighted Imaging Video Permission Withheld
Lihua Chen, Ailian Liu, Yan Guo, Xin Li, Dan Guo
Prostate cancer is the second most common cancer for men, and it has high leading cause of cancer death among men. The term radiomics has attracted increased attention in recent years, and it is the process of the conversion of medical images into high-dimensional, mineable data via high-throughput extraction of quantitative features, followed by subsequent data analysis for decision support.The aim of this study was to evaluate radiomics as a tool to distinguish PCa from BPH based on diffusion-weighted imaging (DWI) sequence without subjective factors.
|
|
3424.
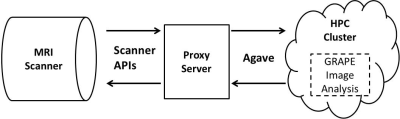 |
112 |
User-defined, scanner-integrated, and real-time MRI image analysis in a cloud-based computing environment
Refaat Gabr, William Allen, Getaneh Tefera, Xiaojun Sun, Renjie He, Manickam Kumaravel, Matthew Vaughn, Ponnada Narayana
To enhance the utility of quantitative MRI, we propose a flexible platform for high-performance cloud computing integrated with the MRI scanner. Jetstream, an NSF-sponsored open science platform for high-performance computing resources, was integrated into a clinical 3.0T MRI system for executing user-defined image analysis using the graphical pipeline environment (GRAPE) tool. Integration was achieved through the Agave platform. This framework was used for real-time quantitative T1 mapping for cartilage tissue assessment. Seamless scanner integration enabled immediate access to the results to the interpreting clinician, providing valuable quantitative information which can be incorporated in clinical practice.
|
|
3425.
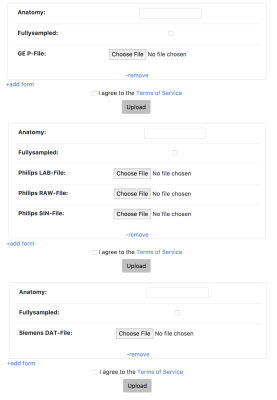 |
113 |
mridata.org: An Open Archive for Sharing MRI Raw Data
Frank Ong, Shahab Amin, Shreyas Vasanawala, Michael Lustig
Current machine learning techniques for image reconstruction require large number of datasets for training, yet the number of public MRI raw datasets is limited. We present mridata.org as an open archive for researchers to share their MRI raw data. The website is designed to facilitate sharing MRI datasets, with features including automatic ISMRMRD conversion from uploaded vendor specific files. We hope that with contributions from many researchers, this website can provide more datasets to train and validate machine learning models for MRI reconstruction.
|
|
3426.
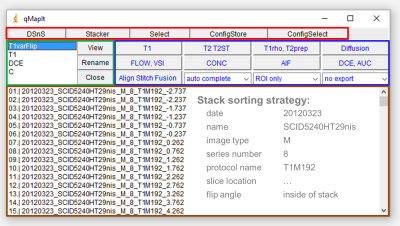 |
114 |
qMapIt, a multi-parametric analysis platform for ImageJ
Michael Kaul, Gerhard Adam
Workstations are expensive and the access is often limited. We developed several ImageJ-plugins to build the basis for a multi-parametric imaging platform for ImageJ. ImageJ is a flexible and extendable image processing software that runs with Windows, MacOS and Linux. With qMapIt the researcher has a graphical user interface to selectively import DICOM files and to perform multi-core supported data analysis. Whether relaxation time analysis for T1, T2, T2*, T1ρ, or various diffusion models, or velocity mapping, or vessel size imaging, or pharmacokinetic modelling you name it. Every step can also be addressed in a macro script to automate the workflow. To reduce programming overhead and to speed up the plugin development a fitting and visualisation framework is embedded.
|
|
3427.
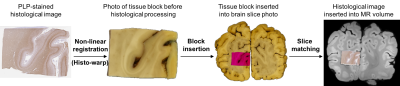 |
115 |
Pipeline for Registering Histological Sections to MRI Volumes
Istvan Huszar, Karla Miller, Menuka Pallebage-Gamarallage, Olaf Ansorge, Christopher Mirfin, Mattias Heinrich, Mark Jenkinson
Post-mortem MRI–histology comparisons provide great potential to advance our understanding of disease, and validating the source of MRI signals, that is necessary for the development of novel imaging methods to study neurodegeneration. A semi-automated prototype of a registration pipeline is reported, that was designed for conventional sparse histological sampling. Use of the pipeline is demonstrated by inserting individual 25 x 25 mm histological sections to their respective locations in whole-brain MRI data. The registration accuracy is approximately 1 mm.
|
|
3428.
 |
116 |
Automated breast segmentation with high reproducibility of MR-based breast density measurement
Jie Ding, Arjun Anilkumar, Patricia Thompson, Maria Altbach, Jean-Philippe Galons, Cynthia Thomson, Alison Stopeck, Chuan Huang
Breast density(BD) is a significant risk factor for breast cancer and serves as a biomarker of risk in clinical trials. Breast segmentation is the first and an important step for accurate and reproducible BD estimation. However, the conventional manual segmentation is labor-intensive and bias-prone. Based on fat-water decomposition MRI, we developed an automated breast segmentation method and validated it against manual segmentation using 50 test-retest scans. The BD measures using our automated segmentation were very comparable to results from manual segmentation, and exhibited extremely high test-retest reproducibility. Our automated segmentation yielded more reproducible BD measures than the manual segmentation method.
|
|
3429.
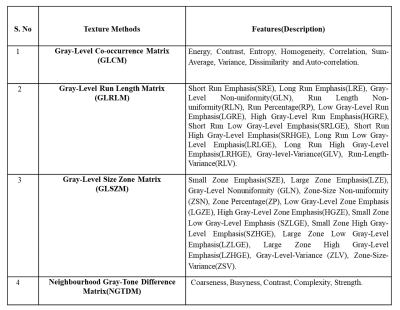 |
117 |
Feature Extraction and Analysis for Characterization of Breast Lesion Type using Multi-parametric MRI Video Permission Withheld
Snekha Thakran, Subhajit Chatterjee, Rakesh Gupta, Anup Singh
Quantitative analysis of T1-perfusion data provides estimation of hemodynamic and physiological parameters of tissue. Texture analysis uses mathematical approach to distinguish the spatial distribution of signal intensity variations. In this study, we computed different texture and quantitative parameters in terms of characterizing histological types (lobular and ductal) of invasive breast cancer. Experimental results revealed that combination of texture and quantitative features provided highest sensitivity and specificity to differentiate IDC and ILC breast lesions.
|
|
3430.
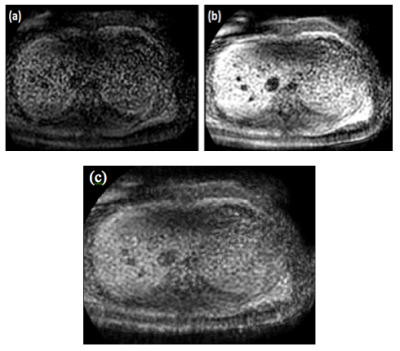 |
118 |
Detection of Liver Fibrosis using Strain-Encoding MRI and Support Vector Machine
Inas Yassine, Mai Wael, Mohamed Elmahdy, Tamer Basha, Ahmed Fahmy, Ralph Sinkus, Theo Heller, Ahmed Gharib, Khaled Abd-Elmoniem
This study proposes a device-free semi-automatic liver fibrosis identification system based on Strain Encoded (SENC) MRI. SENC-MRI was applied to quantify liver deformation induced by the heart motion over the cardiac cycle. Twenty-two patients with different stages of biopsy proven liver fibrosis and ten healthy subjects were imaged using SENC-MRI. A Support Vector Machine (SVM) classification system was used to classify the strain and strain rate for both the patients and healthy subjects. Based on leave-one-out cross validation. Strain and strain rate were more robust than the peak-to-peak value based classification, which has bias towards the sensitivity. The proposed method showed classification accuracy of 87.5% with sensitivity and specificity of 90.0% and 90%, respectively.
|
|
3431.
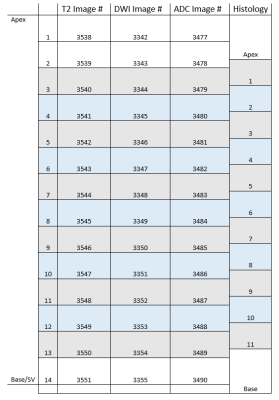 |
119 |
A Methodology Towards Registering Prostate Histology and Radiologic Imaging to Validate Prostate Cancer Detection in 2D
Brandon Caldwell, Meltem Uyanik, Michael Abern, Virgilia Macias, Cristian Luciano, Richard Magin
In-vivo radiological imaging is used globally to detect possible cancers and inform treatment decisions, but difficulties arise when attempting to compare radiological findings to the gold-standard of diagnosis, histopathology. Standard imaging protocols have documented success but to determine the reliability of new imaging sequences and modalities, correlation to histopathology must be made. Several methods have been proposed for registration in both 2D and 3D, but these have shown limited effectiveness and often require unique equipment or proprietary algorithms. In this study, we attempt to complete an accurate registration in 2D in order to validate different imaging modalities.
|
|
3432.
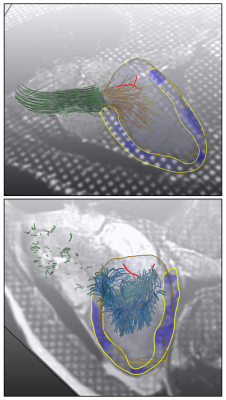 |
120 |
Combined Visual Analysis of Myocardial Strain and Intra-Ventricular Blood Flow
Chitiboi Teodora, Anja Hennemuth, Lennart Tautz, Leon Axel
Intra-ventricular blood flow dynamics are closely related to both ventricle and valve geometry and to the contraction pattern of the myocardial wall. The interaction mechanisms between contraction forces inside the myocardium and hydrodynamic forces are highly complex and have not yet been fully understood, especially in the presence of pathology. We propose a visual analysis framework for the integrated assessment of ventricle and valve morphology, blood flow and local myocardial function. This is a first step to enable better understanding of the different mechanical factors involved in hypertrophic cardiomyopathy (HCM) and their combined contributions to the formation of obstructive HCM.
|
|
Machine Learning for Image Analysis
Electronic Poster
Acquisition, Reconstruction & Analysis
Monday, 18 June 2018
Exhibition Hall |
14:45 - 15:45 |
|
|
Computer # |
|
3481.
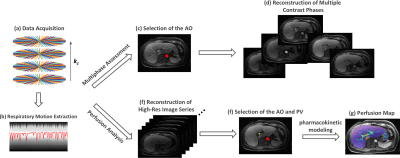 |
49 |
AUTO-DCE-MRI: A Deep-Learning Augmented Liver Imaging Framework for Fully-Automated Multiphase Assessment and Perfusion Mapping
Li Feng, Fang Liu, Henry Rusinek, Bari Dane, Henry Brody, Teodora Chitiboi, Daniel Sodickson, Ricardo Otazo, Hersh Chandarana
This work proposes and tests a novel dynamic contrast-enhanced liver MRI framework called AUTO-DCE-MRI, which allows for simultaneous multiphase assessment and automated perfusion mapping from a single continuous free-breathing data acquisition. A deep convolutional neural network is trained to automatically select the abdominal aorta and the main portal vein. For low temporal-resolution multiphase assessment, the contrast bolus information is extracted from the aorta to guide image reconstruction of desired contrast phases. For high temporal-resolution perfusion analysis, the arterial/venous input functions are generated from the automatically selected regions in the aorta and main portal vein for pharmacokinetic modeling.
|
|
3482.
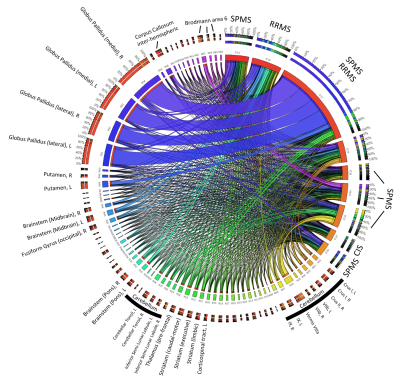 |
50 |
Supervised Machine Learning with Blind Source Separation (BSS) reveals distinct networks of pathological changes in brain magnetic susceptibility (QSM): Application to multiple sclerosis.
Ferdinand Schweser, Juliane Damm, Niels Bergsland, Michael Dwyer, Akshay Dhamankar, Bianca Weinstock-Guttman, Robert Zivadinov
Conventional region-of-interest (ROI) or voxel-based analyses of quantitative susceptibility maps (QSM) do not provide insights on the mechanistic and temporal independence of tissue alterations between subjects. In this study, we combined Blind Source Separation (BSS) with a Machine Learning strategy to reveal specific, independent disease-related networks of tissue alterations. Our analysis identified anatomically localized independent networks of pathological susceptibility alterations in multiple sclerosis (MS) without a priori information on age, sex, disease, or anatomy.
|
|
3483.
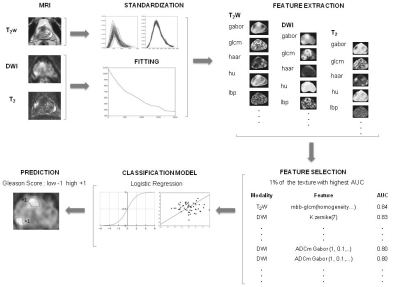 |
51 |
Machine learning for prostate cancer Gleason score prediction using radiomics of T2-weighted imaging, diffusion weighted imaging and T2-mapping Video Permission Withheld
Jussi Toivonen, Ileana Montoya Perez, Parisa Movahedi, Harri Merisaari, Janne Verho, Pekka Taimen, Peter Boström, Tapio Pahikkala, Hannu Aronen, Ivan Jambor
We extensively evaluated large number radiomics of prostate T2-weighted imaging, diffusion weighted imaging and T2-mapping. The highest overall performance estimate (AUC = 0.88) we obtained for the model utilizing a small subset of texture features from the ADCm, K, and T2w parameters. These features included texture descriptors based on gray-level co-occurrence matrix, Gabor transform, and the Zernike and Hu moments.
|
|
3484.
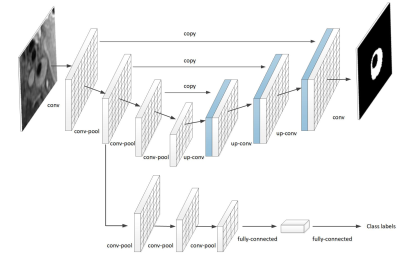 |
52 |
An integrative deep learning model to distinguish between normal and atherosclerotic carotid arteries on black-blood vessel wall MRI
Jiayi Wu, Jingmin Xin, Jie Sun, Zechen Zhou, Baocheng Chu, Dongxiang Xu, Chun Yuan
Vessel wall (VW) MRI has been used to characterize atherosclerotic plaques but the review process is complex. To facilitate the translation of VWMRI into clinical application, we utilized deep convolutional neural networks (CNN) to distinguish between normal and atherosclerotic carotid arteries automatically in black-blood (BB) VWMRI. Trained with a dataset that contains both normal and diseased carotid arteries with expert labeling, an integrative deep CNN model was developed and yielded better automatic diagnosis accuracy of carotid atherosclerosis (85.18%) compared with other existing methods. This model may be used as an initial screening to separate normal from diseased arteries.
|
|
3485.
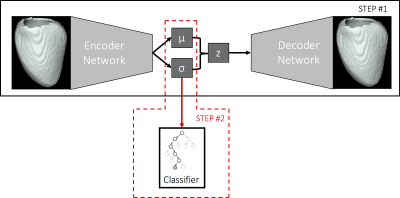 |
53 |
Deep learning analysis of cardiac MRI for unsupervised classification of heart disease
Carlo Biffi, Ozan Oktay, Wenjia Bai, Giacomo Tarroni, Antonio De Marvao, Martin Rajchl, Stuart Cook, Declan O'Regan, Daniel Rueckert
Magnetic resonance imaging provides detailed assessment of cardiac structure and function. However, conventional manual phenotyping reduces the rich biological information to few global metrics. A learning-based approach providing more complex phenotypic features could offer an objective data-driven means of disease classification. In this work, we exploit a convolutional variational autoencoder model to learn low-dimensional representations of cardiac remodelling which are easily visualisable on a template shape and readily applicable in classification models. This approach yielded 91,7% accuracy in the discrimination among healthy, hypertrophic and dilated cardiomyopathy subjects, and shows promise for unsupervised classification of pathologies associated with ventricular remodelling.
|
|
3486.
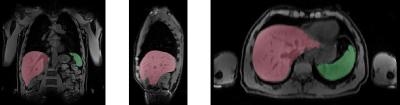 |
54 |
Automated segmentation of abdominal organs in T1-weighted MR images using a deep learning approach: application on a large epidemiological MR study Video Permission Withheld
Thomas Küstner, Marc Fischer, Sarah Müller, Daniel Guttmann, Konstantin Nikolaou, Fabian Bamberg, Bin Yang, Fritz Schick, Sergios Gatidis
In this study we implemented and validated an automated method for segmentation of T1-weighted MR images using a deep learning approach. We applied the algorithm two 80 training and 20 validation data sets drawn from an epidemiological MR study and observed high accuracy compared to manual tumor segmentation. This approach can potentially contribute to efficient analysis of large epidemiological MR studies in the future.
|
|
3487.
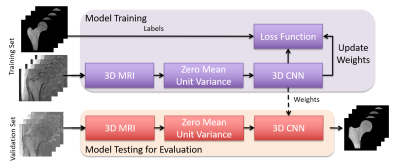 |
55 |
Fully Automatic Proximal Femur Segmentation in MR Images using 3D Convolutional Neural Networks
Siyuan Xiang, Gregory Chang, Stephen Honig, Kyunghyun Cho, Cem Deniz
MRI has been successfully used in structural imaging of trabecular bone micro architecture in vivo. In this project, we develop supervised convolutional neural network for automatically segmental proximal femur from structural MR images. We found that the proposed method provides accurate segmentation without any post-processing, bringing trabecular bone micro architecture analysis closer to clinical practice.
|
|
3488.
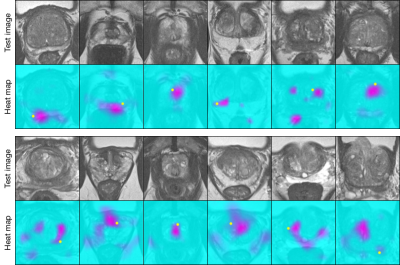 |
56 |
Explanatory Auxiliary Generative Adversarial Network for Prostate Cancer Lesion Awareness with Very-Weak Supervision
Ruiming Cao, Xinran Zhong, Kyunghyun Sung
Although supervised deep convolutional neural network has shown good performance regarding lesion detection and classification using multi-parametric MRI, it is still limited by high data label requirement. In this work, we proposed a model called explanatory auxiliary generative adversarial network (ExpA-GAN), which generates heatmap for object detection under very-weak supervision (no ground truth location). The model was trained and evaluated in a public TCIA prostate dataset. Among 50 testing slices enclosing the whole prostate, the proposed model achieves 0.169 normalized distance for lesion detection, showing the potential to improve lesion detection using limited labeled data.
|
|
3489.
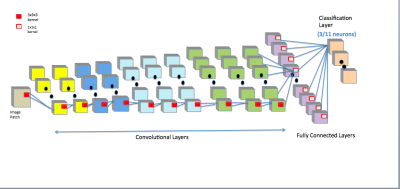 |
57 |
Cascaded 3D fully convolutional neural network for segmenting amygdala and its subnuclei
Yilin Liu, Brendon Nacewicz, Gregory Kirk, Andrew Alexander, Nagesh Adluru
We address the problem of segmenting subcortical brain structures that have small spatial extent but are associated with many neuropsychiatric disorders and neurodegenerative diseases. Specifically, we focused on the segmentation of amygdala and its subnuclei. Most existing methods including deep learning based focus on segmenting larger structures and the existing architectures do not perform well on smaller structures. Hence we designed a new cascaded fully convolutional neural network with architecture that can perform well even on small structures with limited training data. Several key characteristics of our architecture: (1) 3D convolutions (2) deep network with small kernels (3) no pooling layers.
|
|
3490.
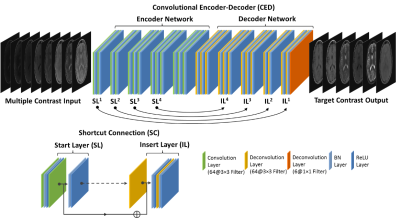 |
58 |
MR Image Synthesis Using A Deep Learning Based Data-Driven Approach
Fang Liu, Alan McMillan
In this study, we demonstrate MR image synthesis using deep learning networks to generate six image contrasts (T1- and T2-weighted, T1 and T2 FLAIR, STIR, and PD) from a single multiple-dynamic multiple-echo (MDME) sequence. A convolutional encoder-decoder (CED) network was used to map axial slices of the MDME acquisition to the six different image contrasts. The synthesized images provide highly similar contrast and quality in comparison to the real acquired images for a variety of brain and non-brain tissues and demonstrate the robustness and potential of the data-driven deep learning approach.
|
|
3491.
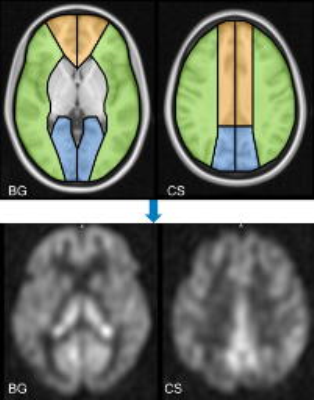 |
59 |
Arterial spin labeling (ASL)-based radiomics features for predicting perfusion territory changes after carotid endarterectomy: a pilot study Video Permission Withheld
Tianye Lin, Chencui Huang, Jianxun Qu, Bing Wu, Panli Zuo, Xiangfei Chai, Feng Feng
To investigate whether radiomics can be apply to cerebrovascular disease and to develop features based on ASL for predicting perfusion territory change after carotid endarterectomy (CEA). A total of 1029 features were derived from ASL images, and 14 features were selected when comparing the differences between the two groups (select K best P<0.05). The selected features in difference are in agreement with visual inspection of collateral flow based on arterial transit artifact (ATA) on ASL.
|
|
3492.
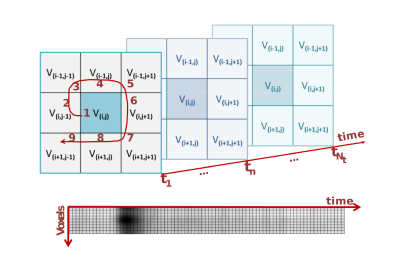 |
60 |
Perfusion MRI in stroke as a regional spatio-temporal texture
Noëlie Debs, Mathilde Giacalone, Pejman Rasti, Tae-Hee Cho, Carole Frindel, David Rousseau
We tackle the clinical issue of predicting the final lesion in stroke from early perfusion magnetic resonance imaging. We demonstrate here the value of exploiting directly the raw perfusion data by encoding the local environment of each voxel as a spatio-temporal texture. As an illustration for this approach, the textures are characterized with Haralick coefficients computed on co-occurrence matrices and a standard support vector machine classifier is used for the classification. This simple machine learning classification scheme demonstrates good results while working on raw perfusion data.
|
|
3493.
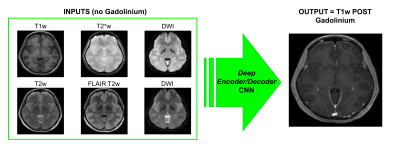 |
61 |
Predicting Contrast Agent Enhancement with Deep Convolution Networks
Thomas Christen, Enhao Gong, Jia Guo, Michael Moseley, Greg Zaharchuk
In this study, we tested whether deep convolutional neural networks (CNNs) could predict what an image would look like if a contrast agent was injected in the body. We trained a network to use information contained in a non-contrast MR brain exam and create a synthetic T1w image acquired after gadolinium injection. Multiple datasets including patients with tumors were used for training. Great similarities were found between the predicted and the actual images acquired after contrast agent injection. If further validated, this approach could have great clinical utility in patients who cannot receive contrast.
|
|
3494.
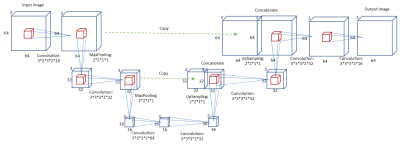 |
62 |
Automatic Segmentation of Carotid Vessel Wall Using Convolutional Neural Network
Li Chen, Jie Sun, Wei Zhang, Thomas Hatsukami, Jianrong Xu, Jenq-Neng Hwang, Chun Yuan
Accurate vessel wall segmentation on black-blood MRI is an important but difficult task. Using previously annotated carotid vessel wall contours by human reviewers, a convolutional neural network (CNN) was trained to predict vessel wall region from the combination of T1-weighted and time-of-flight images. Compared with human segmentation results, the CNN-based model achieved a Dice similarity coefficient of 0.86±0.06 and a correlation coefficient of 0.96 (0.94, 0.97) in measuring vessel wall area. Fast and accurate vessel wall segmentation may help fully realize the potential of vessel wall MRI in monitoring atherosclerosis progression or regression in serial studies and clinical trials.
|
|
3495.
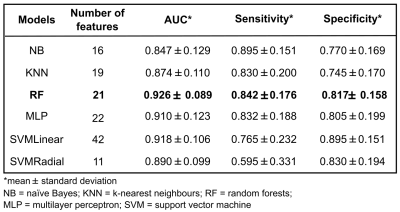 |
63 |
3D Texture Analysis on fMRI to Detect Alterations in the Striatal Network of an Alcohol-Preferring Rat Model
Silvia Ruiz-España, Rafael Ortiz-Ramón, Úrsula Pérez-Ramírez, Antonio Díaz-Parra, Roberto Ciccocioppo, Santiago Canals, David Moratal
We propose an approach that uses 3D texture features extracted from fMRI to detect changes in the striatal network induced by alcohol drinking. Scans of eighteen alcohol-preferring rats before and after 30 days of alcohol consumption were analyzed. Data were preprocessed and a group independent component analysis was performed to identify striatal network; in total 36 volumes of interest were studied. Texture analysis was performed using 43 texture features and six predictive models. An AUC of 0.927±0.089 (sensitivity=84.25%, specificity=81.75%) was obtained for the best model (random forests). The proposed method was able to accurately identify subjects with alcohol use disorders.
|
|
3496.
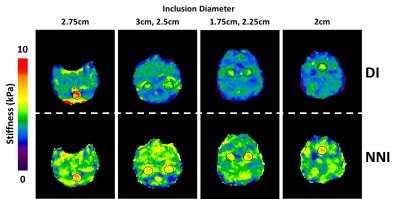 |
64 |
Estimating Inclusion Stiffness with Artificial Neural Networks in Magnetic Resonance Elastography
Jonathan Scott, Matthew Murphy, Arvin Arani, Christopher Schwarz, Armando Manduca, John Huston III, Richard Ehman
Magnetic Resonance Elastography stiffness estimates in intracranial tumors correlate with intraoperative assessment of tumor consistency, but the spatial kernel-based stiffness calculation of Direct Inversion (DI) creates challenges for small or heterogeneous tumors. The objective of this study is to evaluate an artificial neural network based inversion technique (NNI) in the assessment of small stiff inclusions in a brain phantom. This study shows that NNI can resolve inclusions as small as 1.75cm in diameter with a contrast to noise ratio higher than that of DI. Furthermore, preliminary clinical results show agreement with intraoperative findings.
|
|
3497.
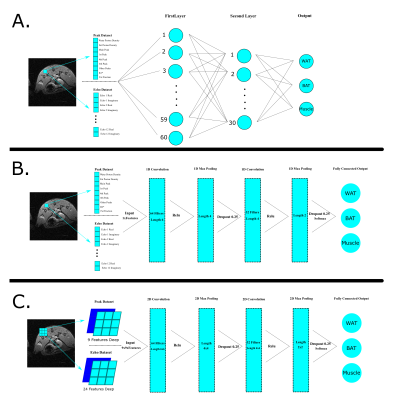 |
65 |
Classification of Adipose Tissues using Machine Learning
Brandon Campbell, Gregory Simchick, Hang Yin, Qun Zhao
Previous classification techniques for determining the quantification of white adipose tissue and brown adipose tissue have relied on using fat fraction and proton relaxation times using fixed peak spectroscopic models. Machine learning algorithms have proven to be highly accurate for image segmentation but their accuracies rely heavily on input datasets. By using the recently proposed Multi-Varying MR Spectroscopy model an increase in dataset specificity can be applied to each voxel by addition of varying fat peak intensity values. Using this new dataset, four machine learning models were compared.
|
|
3498.
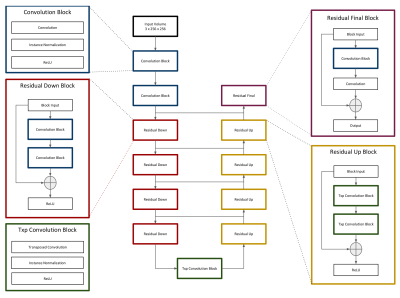 |
66 |
Automated Zonal Prostate Segmentation with 2.5D Convolutional Neural Networks
Alex Bratt, Kevin Seals, Daniel Margolis
Accurate delineation of anatomical boundaries on prostate MR is crucial for cancer staging and standardized assessment. Unfortunately, manual prostate segmentation is time consuming and prone to inter-rater variability while existing automated segmentation software is expensive and inaccurate. We demonstrate a novel fully-automated zonal prostate segmentation method that is fast and accurate using a convolutional neural network. The network is trained using a dataset of 149 T2-weighted prostate MR volumes that were manually annotated by radiologists. Our method improves upon prior related work, achieving a full-gland Dice score of 0.92 and zonal Dice score of 0.88.
|
|
3499.
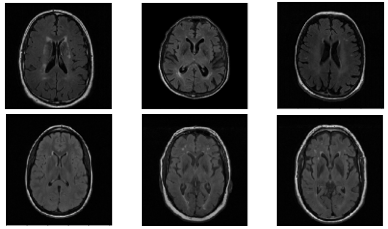 |
67 |
MR Intensity Normalization: Influence on Supervised Machine Learning Algorithms using Textural and Convolutional Features
Mariana Bento, Marina Salluzzi, Leticia Rittner, Richard Frayne
Supervised machine learning techniques have been used in medical imaging and aim to reduce subjectivity and improve quantitative results. When handling heterogeneous MR datasets, most algorithms require pre-processing, such as intensity normalization. Here, the influence of MR normalization techniques on supervised classification is evaluated using handcrafted textural and convolutional features. These features combined can differentiate control subjects from atherosclerosis patients using only imaging scans. Non-significant statistical difference in classification was found across intensity normalization methods, demonstrating little influence of this pre-processing step on the supervised classification outcome.
|
|
3500.
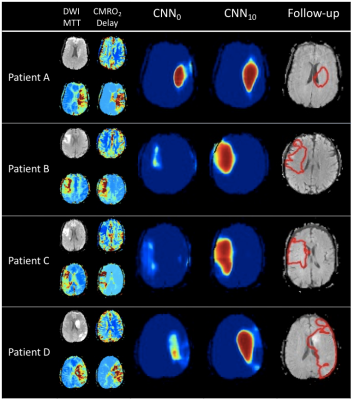 |
68 |
How easily can an existing stroke outcome deep learning model become attuned to new acquisition protocols and patient cohorts?
Anne Nielsen, Mikkel Hansen, Soren Christensen, Maarten Lansberg, Greg Zaharchuk, Kim Mouridsen
Acute ischemic stroke is a major disease and one of the leading causes of adult death and disability. Final outcome prediction is hampered by the heterogeneity and physiological complexity of stroke progression. Convolutional neural networks have shown promising results in final outcome predictions. However, less attention has been paid to the generalizability of the results across patient cohorts. We test the applicability of an existing neural network trained on two clinical studies to completely independent cohort from the DEFUSE 2 trial. We examine how a few additional patients can be used to obtain performance comparable to the original studies.
|
|
3501.
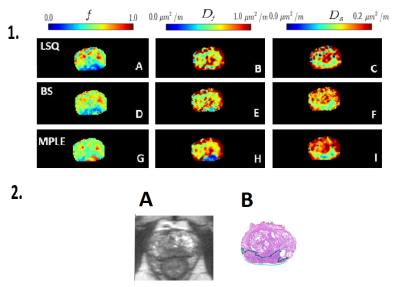 |
69 |
Bi-exponential modeling of prostate diffusion weighted MR imaging acquired using high b values: clinical evaluations of advanced post-processing methods
Parisa Movahedi, Harri Merisaari, Ileana Montoya Perez, Jussi Toivonen, Pekka Taimen, Peter Boström, Janne Verho, Hannu Aronen, Tapio Pahikkala, Ivan Jambor
The aim of this study was to evaluate various mathematical methods for enhanced parameter estimation of bi-exponential DWI (12 b values 0-2000 s/mm2) of prostate cancer. Least Squares (LSQ), Bayesian Shrinkage (BS) and Maximum Penalized Likelihood Estimation (MPLE) fitting methods were evaluated in the terms of Coefficients of Variation (CV), Contrast to Noise Ratio (CNR) and the Area under the curve (AUC) between tumor and non-tumor prostate tissue. BS and MPLE methods improved AUC and CNR values of bi-exponential model parameters and also decreased CV values in comparison with the commonly used LSQ fitting method.
|
|
3502.
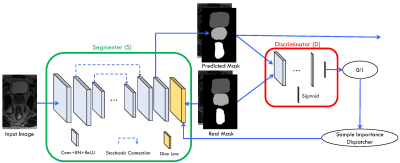 |
70 |
Pelvic Organ Segmentation with Sample Attention based Stochastic Connection Networks
Dong Nie, Li Wang, Jun Lian, Dinggang Shen
Accurate segmentation of pelvic organs is important for prostate radiation therapy. Modern radiation therapy starts to use magnetic resonance image (MRI) as an alternative to CT image, because of the superior soft tissue contrast of MRI and also no risk of radiation exposure. In this abstract, we propose a novel deep network architecture, called “Sample Attention based Stochastic Connection Networks” (SASCNet), to delineate pelvic organs from MRI in an end-to-end fashion. Our proposed network has two main contributions: 1) We propose a novel randomized connection module and adopt it as a basic unit to combine the shallower and deeper layers in the fully convolutional networks (FCN); 2) We propose a novel adversarial attention mechanism to automatically dispatch sample importance so that we can avoid the domination of easy samples in training the network. Experimental results show that our SASCNet achieves competitive segmentation accuracy.
|
|
3503.
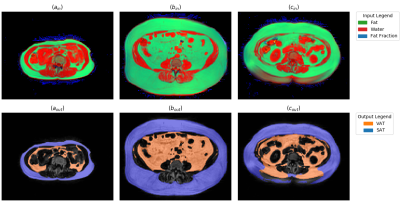 |
71 |
Fully Convolutional Networks for Automated Segmentation of Abdominal Adipose Tissue Depots in Water-Fat MRI
Taro Langner, Anders Hedström, Håkan Ahlström, Joel Kullberg
The segmentation and quantification of human adipose tissue depots offers new insights into the development of metabolic and cardiovascular disease but is often hindered by the need for time-consuming and subjective manual input. We propose an automatic method that uses a convolutional neural network for the segmentation of both visceral adipose tissue (VAT) and subcutaneous adipose tissue (SAT). The network was applied to two-dimensional slices of 90 water-fat MRI scans of the abdomen. In a 10-fold cross-validation it reached average dice scores of 0.979 (VAT) and 0.987 (SAT), with average absolute quantification errors of 0.8% (VAT) and 0.5% (SAT).
|
|
3504.
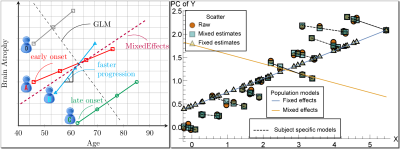 |
72 |
Analysis of longitudinal MRI changes using mixed effects models on deformation tensors
Nagesh Adluru, Hyunwoo Kim, Molly Prigge, Nicholas Lange, Erin Bigler, Janet Lainhart, Andrew Alexander, Vikas Singh
Mixed effects models that include fixed and factor-specific (also known as random) effects offer a natural framework for studying longitudinal MRI data. This work extends mixed effects models to the setting where the responses lie on curved spaces such as the manifold of symmetric positive definite matrices. By treating the subject-wise diffeomorphic deformations between consecutive time points as a field of Cauchy deformation tensors, our framework can facilitate longitudinal analysis that respects the geometry of such data. While the existing body of work dealing with regression models on manifold-valued data is inherently restricted to cross-sectional studies, the proposed mixed effects formulation significantly expands the operating range of longitudinal analyses.
|
|
Image Reconstruction Potpourri
Electronic Poster
Acquisition, Reconstruction & Analysis
Monday, 18 June 2018
Exhibition Hall |
14:45 - 15:45 |
|
|
Computer # |
|
3505.
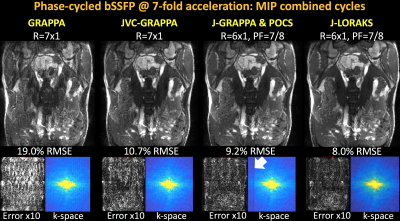 |
73 |
Improving Parallel Imaging by Jointly Reconstructing Multi-Contrast Data
Berkin Bilgic, Tae Kim, Congyu Liao, Mary Manhard, Lawrence Wald, Justin Haldar, Kawin Setsompop
We propose a general joint reconstruction framework to accelerate multi-contrast acquisitions further than currently possible with conventional parallel imaging. Our joint parallel imaging techniques simultaneously exploit similarities between echoes/phase-cycles/contrasts, virtual coil concept, partial Fourier acquisition, complementary sampling across images along with limited support and smooth phase constraints. These permit highly accelerated 2D, Simultaneous MultiSlice and 3D acquisitions as well as improved calibrationless parallel imaging from multiple contrasts. Our algorithms, JVC-GRAPPA and J-LORAKS, provide over 2-fold improvement in reconstruction error compared to conventional GRAPPA, with improved mitigation of artifacts and noise amplification.
|
|
3506.
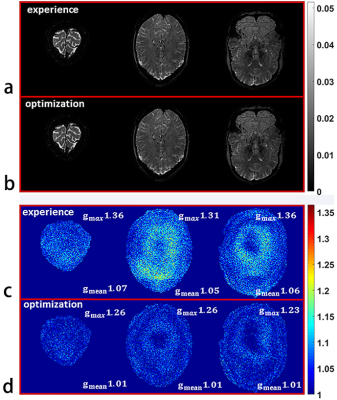 |
74 |
Parameter Optimization of Wave-CAIPI Based on Theoretical Analysis
Zhilang Qiu, Haifeng Wang, Leslie Ying, Xin Liu, Dong Liang
Wave-CAIPI is an novel 3D imaging technique with corkscrew trajectory in k-space to reduce g-factor penalty and speed up MRI acquisitions. The sinusoidal gradient parameters of Wave-CAIPI, amplitude and cycles, play an important role since they determine the point spread function of the trajectory and thus the final reconstruction. However, how to choose the optimal sinusoidal gradient parameters which leads to the minimal g-factor has not been exploited. In this work, we theoretically analyzed the influence of the sinusoidal gradient parameters on g-factor. An optimization algorithm which can be automatically conducted is then proposed to optimize these parameters for achieving minimal g-factor penalty. The simulations show that using the optimized sinusoidal gradient parameters can achieve lower g-factor penalty in Wave-CAIPI reconstructions.
|
|
3507.
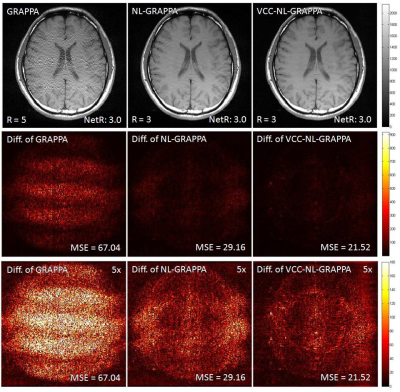 |
75 |
Nonlinear GRAPPA Reconstruction with Virtual Coil Conception
Haifeng Wang, Yuchou Chang, Leslie Ying, Xin Liu, Dong Liang
Nonlinear GRAPPA is a kernel-based approach for improving parallel imaging reconstruction, by reducing noise-induced error. Virtual coil conception has been applied into the reconstruction process for parallel acquisitions, by generating virtual coils containing conjugate symmetric k-space signals from actual multiple-channel coils. In this work, we proposed a hybrid method to combine nonlinear GRAPPA and virtual coil conception for incorporating additional image- and coil-phase information into the reconstruction process. The experiments of in vivo human brain data show that the proposed method can reduce more noise and artifacts than the traditional GRAPPA and original Nonlinear GRAPPA methods.
|
|
3508.
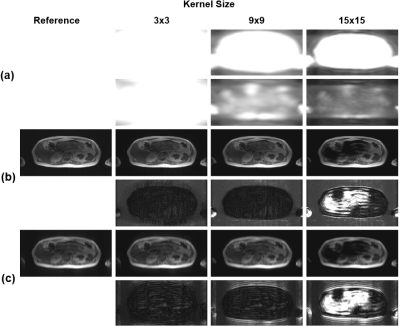 |
76 |
A Method for Automatically Determining an Optimal Kernel Size in ESPIRiT Reconstruction
Jong Bum Son, Colleen Costelloe, Tao Zhang, Jingfei Ma
ESPIRiT is a hybrid-domain parallel imaging method which can estimate the coil-sensitivity information from the k-space calibration matrix. In ESPIRiT, the calibration matrix is constructed by sliding a window through the fully sampled data region of auto-calibrating signals. Presently, the kernel size of the sliding window determining the performance of ESPIRiT reconstruction is empirically chosen, even though an optimal value may vary depending on a combination of scan parameters and scan configurations. In this work, we developed an automatic data-driven method for determining an optimal kernel size in ESPIRiT to reduce the performance variation of ESPIRiT reconstructions.
|
|
3509.
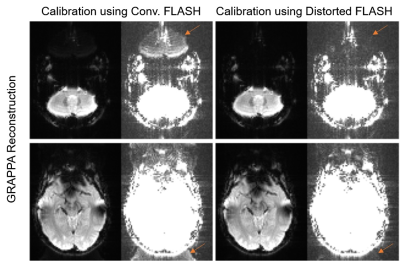 |
77 |
Improved Parallel Imaging Reconstruction of EPI using Inversely Distortion Corrected FLASH as Calibration Data
Mengye Lyu, Yilong Liu, Ed Wu
For parallel imaging reconstruction of EPI, EPI based calibration scan may suffer from ghost artifacts, whereas non-EPI based calibration scan such as FLASH cannot provide consistent geometric distortion. In this study, we propose to employ dual-echo FLASH as the calibration scan, such that B0 field maps can be derived to match FLASH images to EPI images and the reconstruction artifact related to inconsistent distortion can be minimized.
|
|
3510.
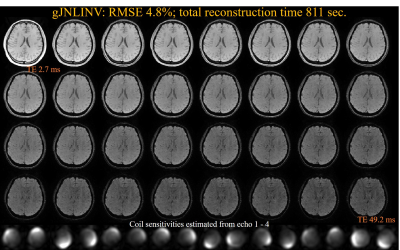 |
78 |
Accelerated reconstruction for calibrationless parallel imaging using grouped joint nonlinear inversion and its application in myelin water imaging
Zhe Wu, Hongjian He, Yi Sun, Jianhui Zhong
The simultaneous estimation of images and coil sensitivities using joint nonlinear inversion (JNLINV) has been shown to be effective for calibrationless parallel imaging for multi-echo data. However, the number of unknowns grows with increasing number of echoes, so the reconstruction procedure could be lengthy. This study proposes an improved method called grouped JNLINV (gJNLINV) to enhance the reconstruction efficiency. Its reconstruction time is ~1/3 of that with JNLINV while preserving a similar root-mean-square error (RMSE) and increasing the fidelity of the coil sensitivities. We further demonstrate the application of gJNLINV on a 32-echo GRE data set for myelin water imaging.
|
|
3511.
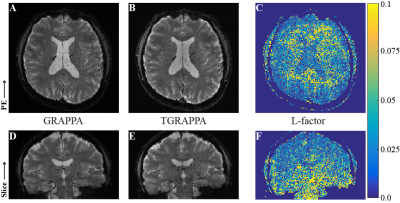 |
79 |
In-Plane Signal Leakage (L-factor) Maps from TGRAPPA
R. Allen Waggoner, Kenichi Ueno, Hideto Kuribayashi, Keiji Tanaka
Residual aliasing is a well-documented problem for multiband reconstructions, but it can be an important issue with in-plane acceleration methods as well. With GRAPPA in particular, the residually aliased signal can be distributed fairy randomly, making it appear as g-factor noise. We demonstrate that the use of TGAPPA permits not only the elimination of the residually aliased signal but also the determination of L-factor maps, which can be a potentially useful tool in understanding how to minimize residual aliasing.
|
|
3512.
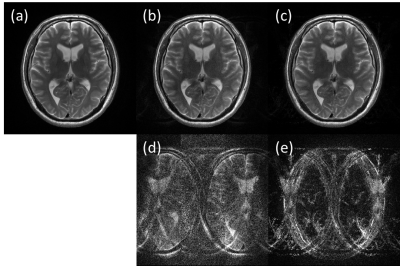 |
80 |
Parallel Imaging Reconstruction Algorithm Mitigating SNR Loss Using Phase Distribution for Fast Spin Echo Sequence
Kosuke Ito, Masahiro Takizawa
Parallel imaging is widely used in clinical routine practice. However, SNR degradation occurs due to undersampling and higher g-factor in higher acceleration factor. In this study, a new algorithm of parallel imaging reconstruction mitigating noise enhancement for fast spin echo sequence was proposed. The algorithm uses information of phase distribution of unaliased image, aliasing image, and folded image. SNR was compared in vivo T2 weighted image between full sampling, conventional parallel imaging, and proposed method. And higher SNR was demonstrated.
|
|
3513.
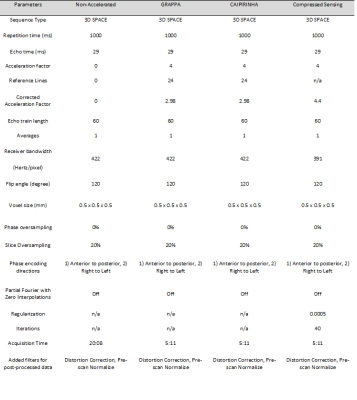 |
81 |
Whole-Volume, High-Resolution, In-Vivo Signal-to-Noise Ratio and G-factor Superiority, and Structural Similarity Index Differences, of Compressed Sensing SPACE and CAIPIRINHA SPACE over GRAPPA SPACE
Neil Kumar, Sheil Kumar, Jan Fritz
Compressed Sensing, CAIPIRINHA, and GRAPPA techniques reduce MRI acquisition times. We used a 3-dimensional sliding region-of-interest analysis tool to perform parameter-controlled, whole-volume average signal-to-noise ratio and g-factor comparison, and g-factor structural similarity index measurements (SSIM) of the above techniques in the setting of 3 Tesla knee MRI. We demonstrate g-factor superiority of CS SPACE over CAIPIRINHA SPACE and g-factor superiority of CAIPIRINHA SPACE over GRAPPA SPACE in living subjects. Post-processing, including pre-scan normalize and distortion correction, improves g-factors and causes variation in the g-factor SSIM results between the techniques.
|
|
3514.
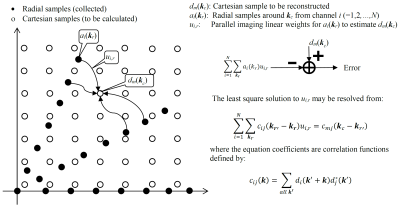 |
82 |
Reduced-FOV k-space Variant Radial Parallel Imaging Reconstruction for Real-time Cardiac MR
Yu Li, Shams Rashid, Yang Cheng, William Schapiro, Kathleen Gliganic, Ann-Marie Yamashita, Marie Grgas, Michelle Maragh, Jie Cao
Radial imaging is k-space variant, but mostly uses k-space invariant methods in image reconstruction. This permits reconstructing images with lower computation complexity at a cost of performance. Here a k-space variant parallel imaging reconstruction technique is developed to reconstruct Cartesian data directly from multi-channel radial samples with affordable computation. It is demonstrated that this technique offers the ability to collect real-time images with a temporal resolution of 40ms and a spatial resolution of 1.7mm. The new technique outperforms those gridding-based methods with k-space invariant algorithms in a stress cardiac test.
|
|
3515.
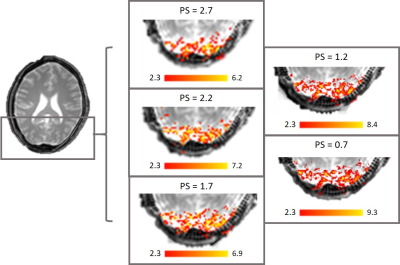 |
83 |
Radial acquisition and PFT reconstruction allow for retrospective selection of spatial resolution in fMRI studies
Banfshe Shafiei Zargar, Abbas Moghaddam
Aiming for fine resolution is always a challenging compromise between various parameters. We have investigated a method for retrospective adjustment of resolution in reconstruction step. Our study of fMRI data indicates that an adjustable pixel size is obtainable in a selected central region during the PFT (Polar Fourier Transform) reconstruction of a radially acquired K-space. Preserving the functional sensitivity, this improvement of resolution results in finer activation detection and higher functional CNR.
|
|
3516.
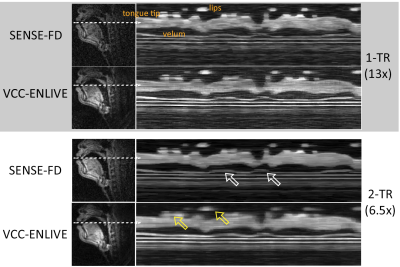 |
84 |
Comparison of leading reconstruction techniques for real-time speech MRI
Weiyi Chen, Yongwan Lim, Yannick Bliesener, Shrikanth Narayanan, Krishna Nayak
Real-time MRI (RT-MRI) has revolutionized the study of human speech production. Two state-of-the-art reconstruction techniques have been adopted by different groups to accelerate real time imaging, constrained SENSE, and regularized nonlinear inversion. In this study, we describe our best performing implementations of both classes of reconstructions, and compare performance on common data from spiral RT-MRI of human speech at 1.5T.
|
|
3517.
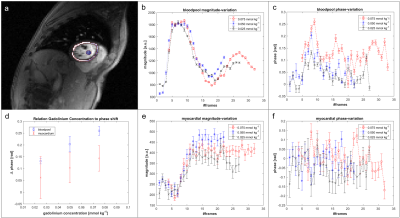 |
85 |
Partial Fourier Acquisitions in Myocardial First Pass Perfusion Revisited
Tobias Hoh, Jonas Walheim, Mareike Gastl, Alexander Gotschy, Sebastian Kozerke
The inflow of a paramagnetic contrast agent (CA) in cardiac dynamic contrast-enhanced (DCE) MRI effects the local phase of magnetization. In this work the impact of phase variations on Partial Fourier (PF) reconstruction is simulated for k-space zero filling, homodyne (HR) and projections onto convex sets (POCS) reconstruction and consequently assessed in in-vivo first-pass perfusion. CA induced phase variations in DCE MRI are seen to compromise HR and POCS reconstruction of PF data to an extent where they do not convey any benefit over simple zero-filling reconstruction.
|
|
3518.
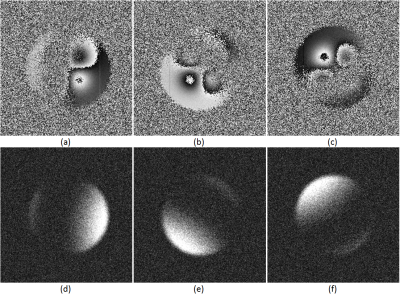 |
86 |
Optimization-Based Simultaneous Combination and Unwrapping for MR Phase Imaging
John Baxter, Zahra Hosseini, Olivia Stanley, Ravi Menon, Maria Drangova, Terry Peters
MRI phase allows for the extraction of inherent tissue contrasts arising from differences in magnetic susceptibility. However, in order to enhance signal-to-noise ratio and accelerate acquisition, modern MRI uses multiple receiver coils. Extracting susceptibility information relies on combining phase information from these multiple channels. Once combined, phase unwrapping beyond the [$$$-\pi$$$, $$$\pi$$$] range allows for further processing and visualization. These processes can be sensitive to noise and errors which are compounded during serial processing, motivating more robust integrated algorithms. This paper introduces simultaneous combination and unwrapping (SCAU) that simultaneously estimates channel phase offset images and a combined unwrapped image.
|
|
3519.
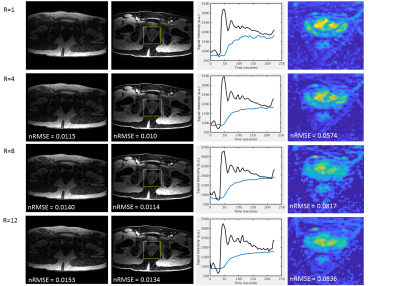 |
87 |
Reconstruction of Accelerated DCE-MRI Guided by Image Quality Metrics
James Rioux, Nathan Murtha, Chris Bowen, Sharon Clarke, Steven Beyea
Golden-angle sampling allows arbitrary retrospective selection of temporal resolution in dynamic MRI scans. To select the fastest temporal resolution that preserves time course fidelity, we propose the use of image quality metrics (IQMs). We demonstrate multiple IQMs that correlate strongly with the accuracy of fitted pharmacokinetic parameters up to at least an acceleration factor of R=12. For a fixed undersampling factor, these metrics can also inform the selection of reconstruction parameters such as regularization weights for compressed sensing. This approach may enable rational, individual-level tuning of temporal resolution following a prospectively accelerated DCE-MRI scan.
|
|
3520.
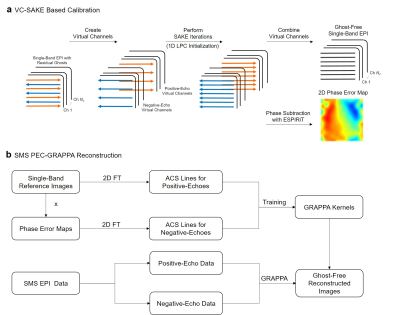 |
88 |
PEC-GRAPPA Reconstruction of Simultaneous Multislice EPI with Slice-Dependent 2D Nyquist Ghost Correction
Zheyuan Yi, Yilong Liu, Mengye Lyu, Ed Wu
Nyquist ghost correction is challenging for simultaneous multislice (SMS) EPI due to the slice-dependent 2D phase error between positive and negative echoes. For this problem, phase error correction SENSE (PEC-SENSE) has been proposed recently, which incorporates slice-dependent 2D phase error maps into coil sensitivity maps. In this study, we extend the concept of PEC-SENSE to k-space based implementation termed as PEC-GRAPPA. It outperforms 1D LPC based GRAPPA reconstruction and requires less tuning than PEC-SENSE such as excluding background areas.
|
|
3521.
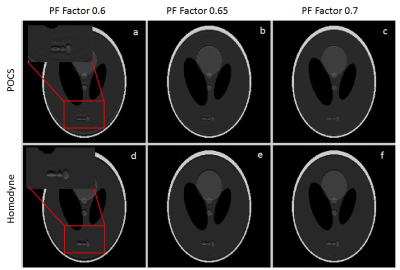 |
89 |
Optimal Partial Fourier MRI reconstruction: Homodyne vs POCS
Venkata kadimesetty, Harsh Agarwal
Partial Fourier MRI (PF-MRI) is a common fast MRI technique to reduce the scan time. While POCS PF-MRI is known to produce MRI images with least amount of RMSE error, homodyne PF-MRI is popularly used in clinical practice. In this abstract we did digital phantom experiments to show that for smoothly varying phase, such as for FSE, POCS localises the error while an over-/under-estimation in image intensity is observed for Homodyne PF-MRI technique. However for fast varying phase such as for GRE, error is localised for Homodyne compared to POCS PF-MRI technique.
|
|
3522.
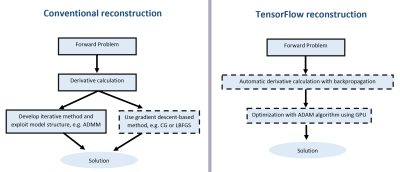 |
90 |
Easy-to-Implement and Rapid Image Reconstruction of Accelerated Cine and 4D Flow MRI Using TensorFlow
Valery Vishnevskiy, Jonas Walheim, Hannes Dillinger, Sebastian Kozerke
Many MR image reconstruction algorithms can be formulated as optimization problems and solved with gradient-based optimization methods of choice. In this work, we present and analyze the performance of the TensorFlow framework for modeling and solving MR image reconstruction problems. We test our approach on undersampled cine cardiac and 4D flow datasets. It is demonstrated that MR image reconstruction is easy to implement in TensorFlow, TensorFlow performs comparably to sophisticated optimization algorithms with theoretical convergence guarantees, and that TensorFlow is as fast as or faster compared to standard MR reconstruction toolboxes.
|
|
3523.
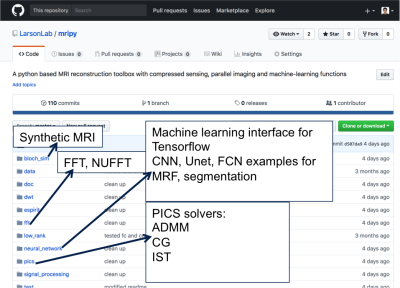 |
91 |
A Python-based MRI Reconstruction Toolbox, “MRIPY”, for Compressed Sensing, Parallel Imaging and Machine Learning
Peng Cao, Xucheng Zhu, Jing Liu, Yan Wang, Peder Larson
A python-based open-source package, “MRIPY” combines the existing MRI reconstruction methods, i.e. compressed sensing and parallel imaging, with deep neural networks that are implemented in the Tensorflow software.
|
|
3524.
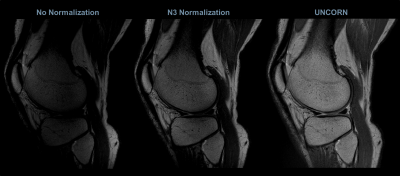 |
92 |
Uniform Combined Reconstruction (UNICORN) of Multi-channel Surface-coil Data at 7T without use of a Reference Scan Video Permission Withheld
Venkata Veerendranadh Chebrolu, Peter Kollasch, Vibhas Deshpande, John Grinstead, Thomas Benner, Robin Heidemann, Daniel Spence, Joel Felmlee, Matthew Frick, Kimberly Amrami
An algorithm for correcting the intensity non-uniformity in MR images without the use of a calibration/reference scan was proposed and its efficacy was demonstrated at ultra-high-field in musculoskeletal MRI. The algorithm was shown to provide better sensitivity in the inferior/superior regions of the knee compared to state-of-the-art inhomogeneity correction filters. Without the use of a reference scan, the algorithm was also shown to provide image uniformity equivalent to calibration based methods.
|
|
3525.
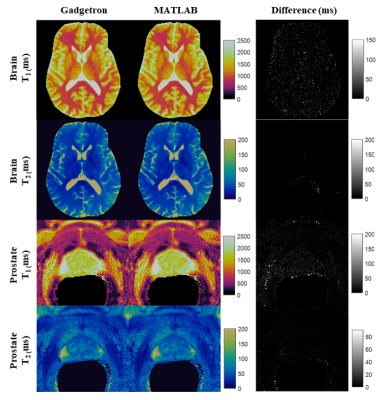 |
93 |
MR Fingerprinting using a Gadgetron-based reconstruction
Wei-Ching Lo, Yun Jiang, Dominique Franson, Mark Griswold, Vikas Gulani, Nicole Seiberlich
Gadgetron-based online MRF reconstruction enables rapid generation of quantitative tissue property maps directly at the scanner before completing acquisition of the following slice. This technique can facilitate multicenter clinical studies and facilitate easier and direct comparisons of quantitative maps from different scanners.
|
|
3526.
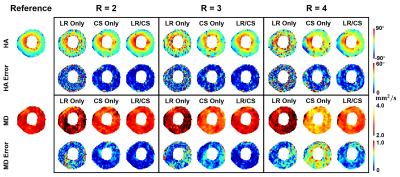 |
94 |
Sparsely Sampled Cardiac Diffusion Tensor Imaging Using Phase-Corrected Joint Low-Rank and Sparsity Constraints
Sen Ma, Christopher Nguyen, Anthony Christodoulou, Sang-Eun Lee, Hyuk-Jae Chang, Debiao Li
We propose to sparsely sample in vivo cardiac diffusion tensor imaging (CDTI) by combining a phase-corrected low-rank model and sparsity constraint. The proposed method was evaluated on 7 hypertrophic cardiomyopathy patients. Helix angle and mean diffusivity maps were compared against employing single constraint, and changes in helix angle transmurality and mean diffusivity were evaluated using Wilcoxon signed rank test to statistically determine the highest achievable acceleration factors preserving CDTI measurements with no significant difference. Our framework shows promise in accelerating acquisition window while preserving myofiber architecture features, and may allow higher spatial resolution or shorter temporal footprint in the future.
|
|
3527.
 |
95 |
Minimum-variance weighted image reconstruction and the application to MRI Video Permission Withheld
Jyh-Miin Lin, Philippe Ciuciu
Non-stationary MRI noise occurs in sparse and non-uniform k-space. Weighted least squares regression has been used to handle data with non-stationary noise. A minimum-variance weighting function may reduce the variance (image noise) of the image, and it may also relax the regularization needed for MRI reconstruction. To obtain the optimal weighting in non-uniform MRI reconstruction, this study uses the Monte Carlo method to determine the minimum-variance weighting function in Shepp-Logan phantom and breast MRI. The parameter $$$\alpha=-0.5$$$ provides a weighting function with the minimum-variance in the reconstructed images.
|
|
3528.
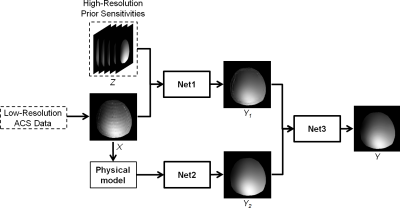 |
96 |
Deep-SENSE: Learning Coil Sensitivity Functions for SENSE Reconstruction Using Deep Learning
Xi Peng, Kevin Perkins, Bryan Clifford, Brad Sutton, Zhi-Pei Liang
Parallel imaging is an essential tool for accelerating image acquisition by exploiting the spatial encoding effects of RF receiver coil sensitivity functions. In practice, the coil sensitivity functions are often estimated from low-resolution auto-calibration signals (ACS) which limits estimation accuracy and in turn results in aliasing artifacts in the final reconstructions. This paper presents a novel deep learning based method for coil sensitivity estimation which exploits empirical and physics-based prior information to produce high-accuracy estimates of coil sensitivity functions from low-resolution ACS. Results are given which demonstrate the proposed method provides a significant reduction in aliasing over standard methods.
|
|
Compressive MRI
Electronic Poster
Acquisition, Reconstruction & Analysis
Monday, 18 June 2018
Exhibition Hall |
14:45 - 15:45 |
|
|
Computer # |
|
3529.
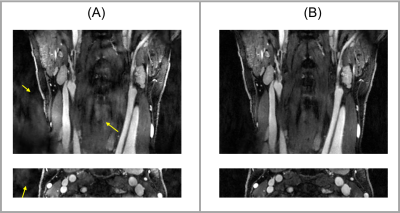 |
97 |
Joint Reconstruction of Images with Different Temporal Basis in Carotid Vessel Wall Imaging
Nan Wang, Anthony Christodoulou, Yibin Xie, Zixin Deng, Zhaoyang Fan, Debiao Li
We recently proposed two techniques, qMATCH and DCE, based on Low Rank Tensor (LRT) framework. qMATCH is a single 8-min scan for carotid T1 T2 mapping and LRT DCE is a 10-min scan evaluating inflammatory status of carotid atherosclerosis. The LRT DCE has many advantages over conventional DCE protocols, but has a scan time longer than typical 5-6 minutes. In this work, we proposed a new protocol combining qMATCH and 5-min DCE. In vivo studies have demonstrated the feasibility of the joint reconstruction. Results of joint reconstruction showed improved image quality with shortened scan time.
|
|
3530.
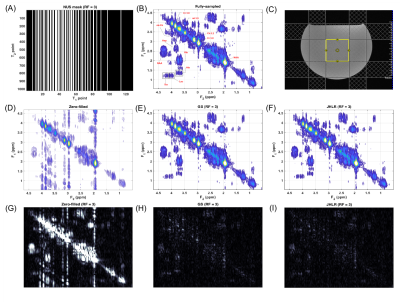 |
98 |
Accelerated Localized Correlated Spectroscopy with Compressed Sensing Reconstruction Using Joint Hankel Low Rank Regularization and Group Sparsity
Andres Saucedo, Manoj Sarma, M. Albert Thomas
Compressed sensing (CS) combined with non-uniform undersampling, such as the low-rank Hankel matrix completion method, have accelerated the acquisition time of 2D magnetic resonance spectroscopy (MRS). This technique relies on reconstructing the vector of all t1 points separately for each F2 point. We introduce a CS-based method that implements joint Hankel low rank regularization, which enforces the low-rankness of all Hankel matrices formed from the entire F2-t1 data simultaneously. We compare this method with group sparsity CS reconstruction of retrospectively undersampled localized correlated spectroscopy (COSY) acquisitions in a brain phantom and calf muscle.
|
|
3531.
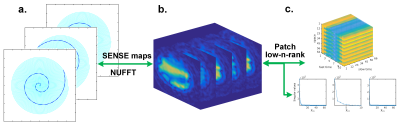 |
99 |
Patch-Tensor Low-n-Rank Reconstruction for Oscillating Steady State fMRI Acceleration
Shouchang Guo, Douglas Noll
Oscillating steady-state imaging is a new acquisition method for T2*-weighted functional MRI that offers very high SNR, but longer acquisition times. The oscillations are highly reproducible, which make low-rank models suitable. In this work, a sparse sampling scheme combined with a patch-based low-rank tensor reconstruction is introduced to speed the image acquisitions. The low-n-rank algorithm was applied to oscillating steady state data to demonstrate the utility of this approach for functional MRI, demonstrating a 17-fold speed up with error levels less than 3%.
|
|
3532.
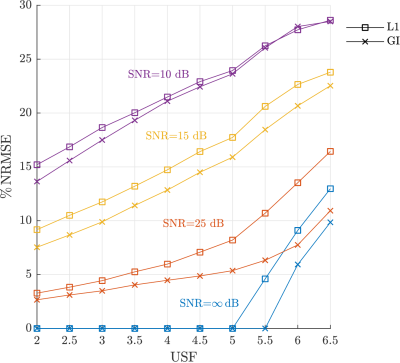 |
100 |
Gini reweighted l1 minimization for rapid MRI
Carlos Castillo-Passi, Claudia Prieto, Gabriel Varela-Mattatall, Carlos Sing-Long, Pablo Irarrazaval
Under-sampling acquisition is oftenly used to reduce the scan time. Compressed Sensing allows the reconstruction of these data by solving a convex optimization problem. This is done to exploit the sparsity of the signals using the l1-norm. We propose to use the Gini Index as a sparsity measure. In this work we demonstrate that this index allow to further increase the under-sampling factor. Interestingly this non-linear index can be computed by solving iteratively reweighted l1 problems, without excessive computational load.
|
|
3533.
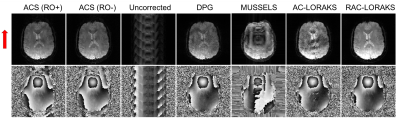 |
101 |
Robust Autocalibrated LORAKS for Improved EPI Ghost Correction with Structured Low-Rank Matrix Models
Rodrigo Lobos, Ahsan Javed, Krishna Nayak, W Hoge, Justin Haldar
The presence of ghost artifacts is a recurrent problem in EPI images, which has been recently addressed using structured low-rank matrix (SLM) methods. In this work we propose a new SLM ghost correction method called Robust Autocalibrated LORAKS (RAC-LORAKS). RAC-LORAKS considers autocalibrated k-space constraints (similar to GRAPPA) to deal with the ill-posedness of existing SLM EPI ghost correction methods. RAC-LORAKS additionally adapts these constraints to enable robustness to possible imperfections in the autocalibration data. We illustrate the capabilities of RAC-LORAKS in two challenging scenarios: highly accelerated EPI of the brain, and cardiac EPI with double-oblique slice orientation.
|
|
3534.
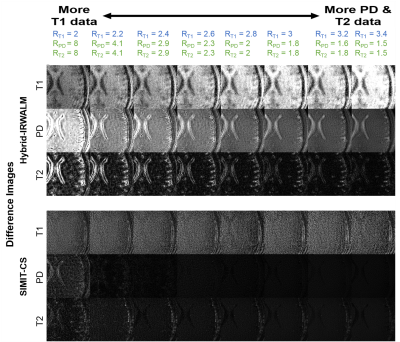 |
102 |
Compressive Sensing Reconstruction for Multi-Contrast Data with Unequal Acceleration Rates
Emre Kopanoglu, Alper Güngör, Toygan Kilic, Emine Saritas, Tolga Çukur, H. Guven
In multi-contrast acquisitions, a critical concern is whether to distribute undersampling uniformly or unequally across contrasts, as scan times and SNR typically vary among sequences. This study investigates a compressive sensing framework in jointly reconstructing multi-contrast data with unequal acceleration rates. Using in-vivo and numerical datasets, the total scan time was fixed and acceleration factors were varied between protocols. The results suggest using lower acceleration rates for protocols with higher-SNR and shorter duration, and higher rates for protocols with lower-SNR and longer duration improves image quality, even in the highly accelerated contrast. The method was also compared to seven state-of-the-art methods from the literature.
|
|
3535.
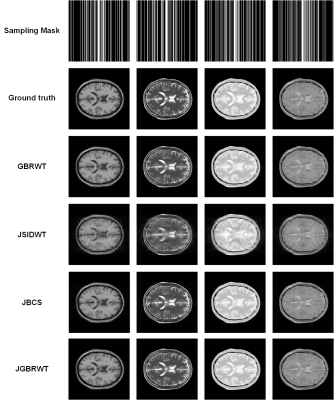 |
103 |
Joint Sparse Reconstruction of Multi-contrast MRI Images with Graph Wavelets
Zongying Lai, Xinlin Zhang, Di Guo, Xiaofeng Du, Zhong Chen, Xiaobo Qu
Multi-contrast images in magnetic resonance imaging (MRI) are widely applied in clinical applications, since an abundant contrast information reflects the characteristics of the internal tissue of human body, providing an effective reference for clinical diagnosis. However, long acquisition time limits the application of magnetic resonance multi contrast imaging. Under-sampling the k-space data and reconstructing images with sparsity constraint is one efficient way to accelerate magnetic resonance imaging sampling. In this work, multi-contrast undersampled MRI images are jointly reconstructed under the sparse representation using graph wavelets. Experiment results demonstrate that the proposed method outperforms the compared state-of-the-art methods.
|
|
3536.
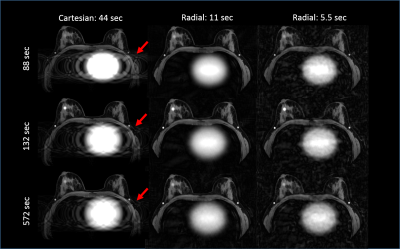 |
104 |
Feasibility of High Spatial and Temporal Resolution Breast DCE-MRI using Radial Acquisition with Data-Driven Model Consistency Condition Reconstruction
Pingni Wang, Roberta Strigel, Julia Velikina, Alexey Samsonov, Leah Henze Bancroft, Kang Wang, Ty Cashen, Kevin Johnson, James Holmes
Dynamic contrast-enhanced (DCE) MRI using conventional Cartesian sampling is used in routine clinical practice due to its high sensitivity for breast cancer. However, ghosting artifacts caused by cardiac motion can obscure the axilla, making interpretation of this area more difficult and potentially obscuring findings. Radial acquisitions are less motion sensitive due to more frequent sampling of the center of k-space and prior work has suggested these methods for breast MRI. In this study, we report results from a reader study to assess image quality of a 3D stack-of-stars radial acquisition compared with Cartesian imaging for breast MRI.
|
|
3537.
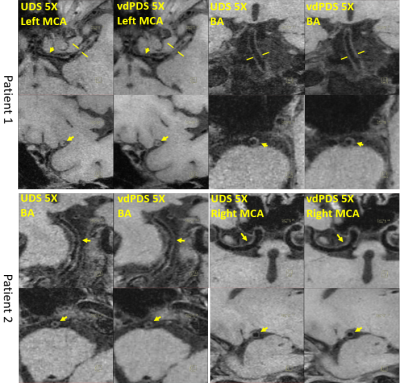 |
105 |
The influence of Sampling on Compressed Sensing accelerated high resolution Vessel Wall Imaging
Sen Jia, Jing Cheng, Lei Zhang, Xin Liu, Dong Liang
This study aims to accelerate high resolution whole brain and neck vessel wall imaging using combined Compressed Sensing and Parallel Imaging. The influence of sampling choices with different control of sampling density and randomness on CSPI reconstruction were investigated on retrospectively and prospectively accelerated in vivo datasets, with emphasis on the sharpness of vessel wall borders which was critically demanded by detecting potential vessel wall thickening and atherosclerotic plaques for ischemic stroke patients.
|
|
3538.
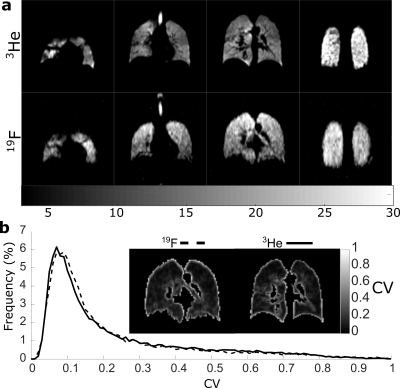 |
106 |
Evaluation of Sparse Sampling for Improved Image Quality of 19F Fluorinated Gas Lung Ventilation MRI
Adam Maunder, Guilhem Collier, Fraser Robb, Madhwesha Rao, Jim Wild
In an effort to improve image SNR per unit time and effective resolution in 19F-fluorinated gas ventilation imaging the application of compressed sensing was investigated. Simulations of sparse sampling were performed using a 3D 3He ventilation imaging dataset as a gold standard. Sparse and fully sampled image fidelity was quantified by the mean-square error and coefficient of variation of signal intensity. Simulations of low resolution and sparsely sampled images with equivalent acceleration factor were also compared. Based on the simulations prospective lung images using sparse sampling with C3F8 gas were then acquired in a healthy volunteer with acceleration factor of 4.
|
|
3539.
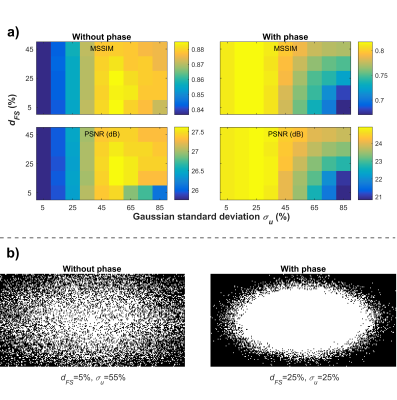 |
107 |
On the importance of adapting compressed sensing for images with significant spatial phase variations
Jérémie Fouquet, Michael Paquette, Réjean Lebel, Maxime Descoteaux, Martin Lepage
We explore the limits of compressed sensing (CS) in the practical setting of T2*-weighted imaging of the brain. Surprisingly, those limits are rapidly reached due in part to the presence of spatial phase variations. While conventional CS performs well for synthetic phase-free images, it is equally performant to or even outperformed by a simple zero-padding of the k-space center for acquired complex-valued images. Clearly, CS must be adapted to images including spatial phase variations, and our results point toward new solutions to achieve this adaptation.
|
|
3540.
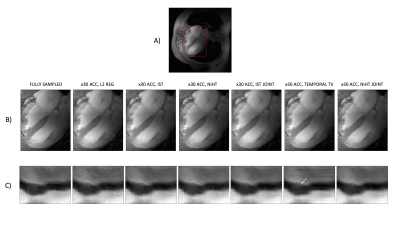 |
108 |
Evaluating the Normalised Iterative Hard Thresholding Algorithm for Compressed Sensing Reconstruction on 7T Cardiac cine MRI.
Sofia Dimoudi, Matthew Robson, Jared Tanner, Aaron Hess
We present our updated results in the evaluation of the Normalised Iterative Hard Thresholding Algorithm (NIHT) for parallel imaging and compressed sensing reconstructions of highly accelerated Cardiac cine MRI at 7 Tesla. We compare imaging performance with three other parallel imaging and compressed sensing methods, including regularisation in the temporal dimension.
|
|
3541.
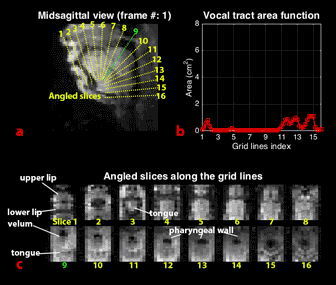 |
109 |
3D Real-Time MRI of Vocal Tract Shaping
Yongwan Lim, Yinghua Zhu, Sajan Lingala, Dani Byrd, Shrikanth Narayanan, Krishna Nayak
We demonstrate a new three-dimensional (3D) real-time MRI technique for the study of dynamic vocal tract shaping during human speech production. This, for the first time, enables a comprehensive assessment of vocal tract area function dynamics. We used a minimum-phase 3D slab excitation, stack-of-spirals gradient echo sequence, pseudo golden-angle view order in kx-ky, linear Cartesian order along kz, and sparse SENSE image reconstruction with spatiotemporal finite difference constraints. This provides 2.4 x 2.4 x 5.8 mm3 spatial resolution, 72 ms temporal resolution, and a 200 x 200 x 70 mm3 field-of-view, which covers the entire adult human vocal tract.
|
|
3542.
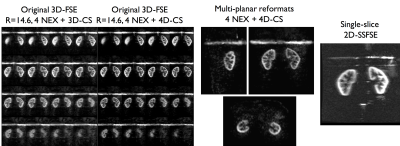 |
110 |
Accelerated volumetric renal perfusion using pseudo-continuous ASL and a 3D Fast-Spin-Echo readout with Compressed Sensing
Manuel Taso, Li Zhao, Arnaud Guidon, Daniel Litwiller, David Alsop
While ASL is a promising technique to measure renal perfusion in multiple applications, its translation into clinical practice is still challenged by its motion-sensitivity and limited spatial coverage. In the current work, we propose an implementation of an undersampled Cartesian 3D-FSE readout with pseudo-continuous ASL labeling and Compressed-sensing reconstruction for fast whole kidney perfusion measurement. Results show that even at high acceleration factors (≈15), acceptable quality whole kidney ASL images could be obtained in less than a minute with increased motion-robustness. Furthermore, the CS acceleration enables acquiring multiple averages, providing increased coverage with similar SNR than mostly used 2D readouts.
|
|
3543.
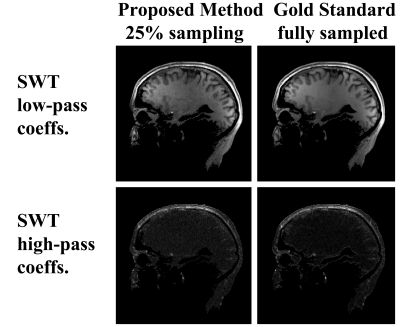 |
111 |
Rapid Parallel MRI Reconstruction Utilizing the Wavelet Filter Bank
Efrat Shimron, Andrew Webb, Haim Azhari
A novel method for reconstruction from highly undersampled parallel MRI data is proposed. The method computes the Stationary Wavelet Transform (SWT) of the unknown MR image directly from sub-sampled k-space measurements, and then recovers the image using the Inverse SWT filter bank. Experiments with in-vivo data show that this method produces high quality reconstructions, comparable to Compressed Sensing (CS) reconstructions. However, unlike CS, the proposed method is non-iterative. Moreover, it is simple, fast, and allows flexible (random or ordered) k-space undersampling schemes.
|
|
3544.
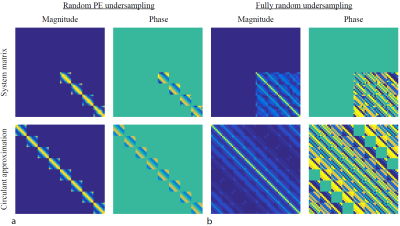 |
112 |
Accelerating Compressed Sensing in Cartesian Parallel Imaging Reconstructions using an Efficient and Effective Circulant Preconditioner
Jeroen Gemert, Kirsten Koolstra, Peter Börnert, Andrew Webb, Rob Remis
Reconstruction methods in parallel imaging and compressed sensing problems are generally very time consuming, especially for a large number of coil elements. In this work, the image is reconstructed using the Split Bregman algorithm (SB). We present an efficient and effective preconditioner that reduces the number of iterations in the linear least squares step of SB by almost a factor of 5 as alternative to extra variable splitting. The designed preconditioner works for Cartesian sampling schemes and for different coil configurations. It has negligible initialization time and leads to an overall speedup factor of 2.5.
|
|
3545.
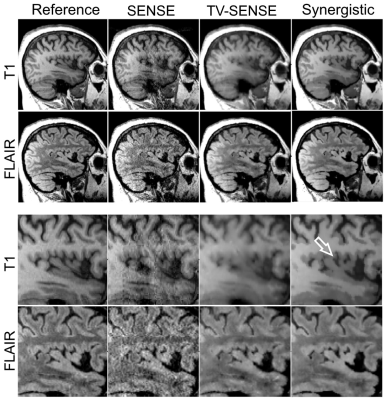 |
113 |
Synergistic reconstruction of undersampled multi-contrast MRI using weighted quadratic priors
ABOLFAZL MEHRANIAN, Claudia Prieto, Radhouene Neji, Colm McGinnity, Alexander Hammers, Andrew Reader
We propose a simple and robust methodology for synergistic multi-contrast MR image reconstruction to improve image quality of undersampled MR data beyond what is achieved from conventional independent reconstruction methods. The advantages of the proposed methodology are threefold: i) it exploits quadratic priors that are mutually weighted using all available MR images, leading to preservation of unique features, ii) the weighting coefficients are independent of the relative signal intensity and contrast of different MR images and iii) the algorithm is based on a well-established parallel imaging iterative reconstruction, which makes the synergistic reconstruction of undersampled MR data clinically feasible
|
|
3546.
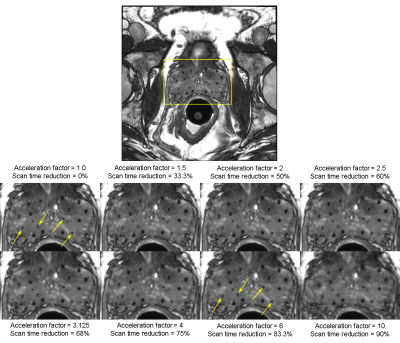 |
114 |
Parallel imaging compressed sensing for MRI-only radiation dosimetry of post-implant prostate cancer brachytherapy
Jeremiah Sanders, Steven Frank, Hao Song, Paula Berner, Aradhana Venkatesan, Jingfei Ma
Researchers recently demonstrated that both anatomical structures and implanted radioactive seeds can be visualized with high-resolution balanced steady-state free precession (bSSFP) imaging using positive-MRI-signal seed markers and an endorectal coil (ERC). However, ERC use is limited by cost, patient intolerance, and low clinical throughput. A previous preliminary study demonstrated that imaging without an ERC resulted in reduced image signal-to-noise ratio and reduced seed detection. In the current study, we investigated the feasibility of using parallel imaging compressed sensing to substantially accelerate the bSSFP acquisition and potentially enable MRI-only dosimetry of post-implant prostate cancer brachytherapy without an ERC.
|
|
3547.
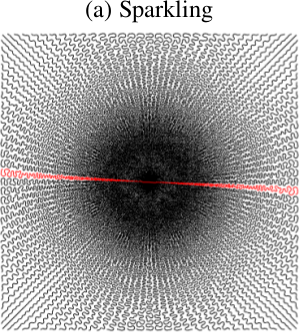 |
115 |
Self-calibrating nonlinear MR image reconstruction algorithms for variable density sampling and parallel imaging
Loubna EL GUEDDARI, Carole LAZARUS, Hanaé CARRIE, Alexandre VIGNAUD, Philippe CIUCIU
Compressed Sensing has allowed a significant reduction of acquisition times in MRI. However, to maintain high signal-to-noise ratio during acquisition, CS is usually combined with parallel imaging (PI). Here, we propose a new self-calibrating MRI reconstruction framework that handles non-Cartesian CS and PI. Sensitivity maps are estimated from the data in the center of k-space while MR images are iteratively reconstructed by minimizing a nonsmooth criterion using the proximal optimized gradient method, which converges faster than FISTA. Comparison with L1-ESPIRiT suggests that our approach performs better both visually and numerically on 8-fold accelerated Human brain data collected at 7 Tesla.
|
|
3548.
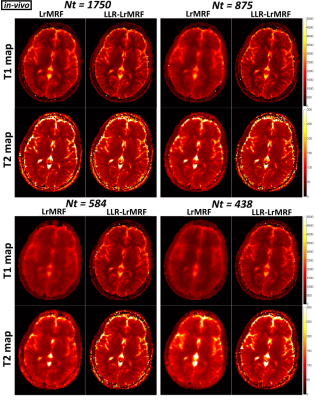 |
116 |
Locally Low Rank Regularization for Magnetic Resonance Fingerprinting
Gastao Cruz, Aurelien Bustin, Olivier Jaubert, Torben Schneider, René Botnar, Claudia Prieto
Magnetic Resonance Fingerprinting (MRF) estimates simultaneous, multi-parametric maps from a dynamic series of highly undersampled time-point images. At very high undersampling factors, some of these artefacts may propagate into the parametric maps leading to errors. Here we propose the use of locally low rank regularization for a low rank approximation reconstruction to enable highly accelerated MRF. The proposed approach was evaluated in simulations and in-vivo brain acquisitions. Results show that the proposed approach enables accurate MRF reconstructions from ~600 time-point images with one radial spoke per time-point.
|
|
3549.
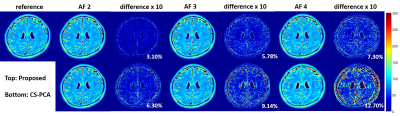 |
117 |
Accelerating T2 Mapping Using a Self-trained Kernel PCA Model
Chaoyi Zhang, Ukash Nakarmi, Hongyu Li, Yihang Zhou, Dong Liang, Leslie Ying
Kernel Principal component analysis(KPCA) model has recently been proposed to accelerate dynamic cardiac imaging. In this abstract, we study the effectiveness of KPCA for MR T2 mapping from highly under-sampled data acquired at different echo time. Different from dynamic cardiac imaging where only morphological information is needed, the quantitative values are highly important in parameter mapping. Here we use a self-trained KPCA model to guarantee the accuracy of the reconstructed T2 maps. The experimental results show that the proposed method can recover the T2 map with high fidelity at high acceleration factors.
|
|
3550.
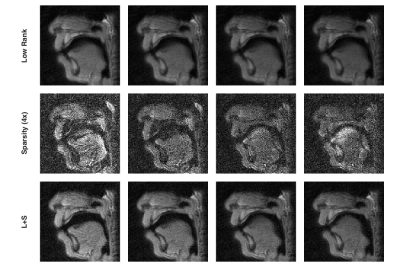 |
118 |
Accelerated real-time spiral MRI for high-resolution velum imaging using low rank and sparse decomposition and Chebyshev based off-resonance correction
Xue Feng, Catherine Pelland, Silvia Blemker, Craig Meyer
In this study, an accelerated real-time spoiled spiral GRE sequence was developed for high-resolution velum imaging during speech to evaluate VPI. The low rank plus sparse decomposition was used to reconstruction highly undersampled (6x) dynamic image series. Chebysheve based off-resonance correction was used to reduce local blurring around velum after L+S reconstruction. The developed method generated high quality dynamic images with minimal temporal blurring and reduced blurring at 1.5 T, which can then be used to track velum movements for further analysis.
|
|
3551.
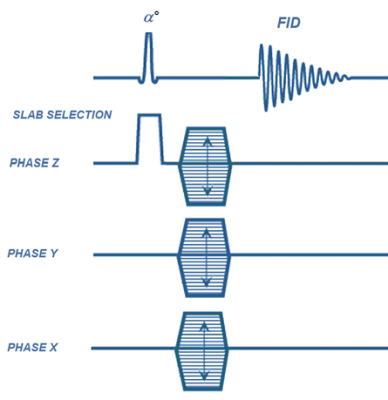 |
119 |
Highly Accelerated 3D Chemical-Shift Magnetic Resonance Imaging Using 4D Compressed Sensing
Jian-xiong Wang, Xiaodong Wen, Crystal Harrison, A Sherry, Craig Malloy
Compressed Sensing method using 4D operators and functions to treat the entire 4D data as unity can perform high reduction rate of acceleration and preserve excellent data fidelity. This work demonstrated its effectiveness on 3D chemical-shift-imaging with reduction rate R=16 or 6.25% sampling ratio and up to R=32 or 3.125% sampling ratio. The method has been implemented onto MRI scanner for real-time sparse 3D CSI acquisition. The excellent data fidelity are demonstrated with 3D CSI images of phantom and in-vivo hyperpolarized [1-13C]pyruvate and its production metabolites.
|
|
3552.
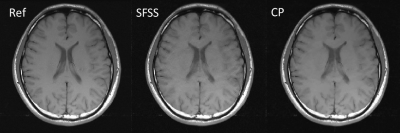 |
120 |
MR image reconstruction using the Chambolle-Pock algorithm
Jing Cheng, Sen Jia, Haifeng Wang, Dong Liang
The combination of Parallel imaging (PI) and compressed sensing (CS) allow high quality MR image reconstruction from partial k-space data. However, most CS-PI MRI methods suffer from detail loss with large acceleration and complicated parameter selection. In this work, we describe and evaluate an efficient and robust algorithm to overcome these limitations. The experimental results on in vivo data show that, the proposed method using a first-order primal-dual algorithm can successfully remove undersampling artifacts while keeping the details with little parameter tuning compared with the existing advanced method.
|
|
Back |
The International Society for Magnetic Resonance in Medicine is accredited by the Accreditation Council for Continuing Medical Education to provide continuing medical education for physicians. |