|
Educational Course Machine Learning in Cardiovascular Disease |
Machine Learning in Cardiovascular Disease
Weekday Course
ORGANIZERS: Sebastian Kozerke, Tim Leiner, Reza Nezafat
Monday, 18 June 2018
N02 |
13:45 - 15:45 |
Moderators: Sebastian Kozerke, Tim Leiner |
Skill Level: Intermediate
Session Number: M-05
Overview
This session will review some of the techniques currently implemented for machine learning using MRI with a special focus to cardiovascular diseases. In addition, the clinical role of machine learning and future perspectives will be described.
Target Audience
This session is targeted to imaging scientists and physicians who are interested in expanding their knowledge of machine learning in cardiovascular diseases.
Educational Objectives
As a result of attending this course, participants should be able to:
-Describe current and future techniques for machine learning in cardiovascular MRI;
-Discuss potential clinical applications of machine learning in cardiovascular MRI; and
-Explain some of the challenges and future perspectives of machine learning in cardiovascular MRI.
13:45
|
|
Nuts & Bolts of Machine Learning
Daniel Rueckert
This talk will give an overview of machine learning techniques for medical image analysis. We will describe both supervised and supervised machine learning approaches. A particular focus will be on deep learning approaches, including Convolutional Neural Networks (CNN) and how these can be used in cardiovascular MR imaging. We will demonstrate machine learning applications for the fast reconstruction of cardiac MR images from undersampled k-space data, image super-resolution as well segmentation of the cardiovascular anatomy in cine cardiac MRI.
|
14:15
|
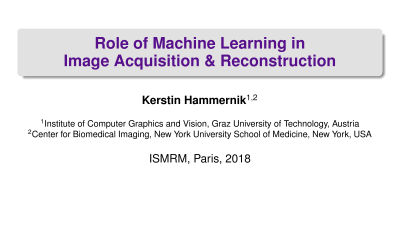 |
Role of Machine Learning in Image Acquisition & Reconstruction
Kerstin Hammernik
In this educational, we give an overview how deep learning is currently used in static and dynamic MRI reconstruction of undersampled k-space data. While we observe large improvements in terms of image quality and artifact removal for learning-based approaches compared to traditional approaches, we have to consider also several challenges. We will discuss both advantages and challenges using examples of current deep learning-based approaches for reconstruction of undersampled k-space data, focusing on the design of network architectures and loss functions.
|
14:45
|
|
Machine Learning: A Clinical Perspective on CV Disease
Bharath Ambale Venkatesh
Machine learning methods are better suited for meaningful risk prediction in extensively phenotyped large-scale epidemiological studies than traditional methods or risk scores. This strategy could yield insights about specific use of variables for specific event prediction and guiding strategies to prevent cardiovascular disease outcomes. Potentially, these techniques could be applied retrospectively to analyze large data sets for identifying disease mechanisms, and as a means of hypothesis generation, without prior assumptions.
|
15:15
|
|
Will ML Replace Radiologists?
Declan O'Regan
In this presentation the current state of the art of Machine Learning in medical image analysis will be discussed and what impact this may have on the role of clinical radiologists.
|
15:45
|
|
Adjournment & Meet the Teachers |
|
Back |
The International Society for Magnetic Resonance in Medicine is accredited by the Accreditation Council for Continuing Medical Education to provide continuing medical education for physicians. |