Machine Learning in Neuroimaging
Oral
Neuro
Wednesday, 20 June 2018
N02 |
16:15 - 18:15 |
Moderators: Dinggang Shen, Greg Zaharchuk |
16:15
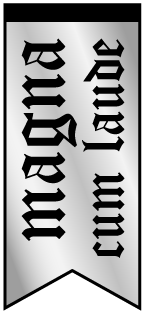 |
0893.
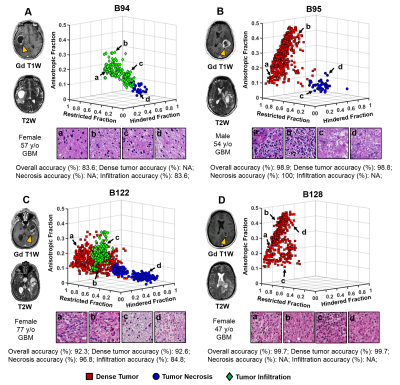 |
Classification of Dense Tumor, Tumor Necrosis and Tumor Infiltration in Glioma: Machine Learning and Diffusion MRI
Zezhong Ye, Xiran Liu, Joshua Lin, Liang Wang, Richard Price, Peng Sun, Jeff Viox, Sonika Dahiya, Albert Kim, Jr-Shin Li, Sheng-Kwei Song
Here we introduce a diffusion MR-based imaging technique - Diffusion MRI Histology (D-Histo), to detect and differentiate various co-existing tumor pathologies including high-cellularity tumor (tumor), tumor necrosis (necrosis) and tumor infiltration (infiltration) within high grade glioma. We incorporated a support vector machine algorithm to generate an automation framework to predict locations of tumor lesion, necrosis and infiltration. The mean predictive accuracy of the D-Histo SVM classifier for tumor lesion, necrosis and infiltration were 91.9%, 93.7% and 87.8%. DTI-based prediction under the same framework resulted in 44.4%, 56.0% and 43.0% accuracy for the three pathologies.
|
16:27
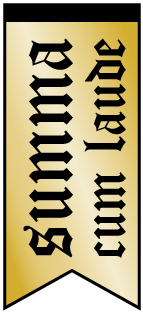 |
0894.
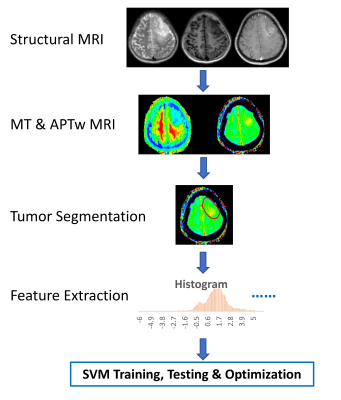 |
Machine learning with amide proton transfer and magnetization transfer MRI for predicting IDH mutation status in diffuse gliomas
Shanshan Jiang, Hye-Young Heo, Qihong Rui, Hao Yu, Yu Wang, Charles Eberhart, Peter Van Zijl, Zhibo Wen, Jinyuan Zhou
The current diagnostic criterion for IDH mutation status is based on lab tests via surgical tissue samples. APTw and MT imaging contrast mechanisms can detect low-concentration mobile proteins and semi-solid macromolecules, respectively. We implemented a support vector machine (SVM)-based method to predict IDH1/2 genotype in gliomas using APTw and MT MRI features. 105 WHO Grade II and III glioma patients with complete imaging and genetic data were enrolled. Within the supervised classification framework, our results suggest that the use of APTw and MT features enabled the SVM to reach the accurate diagnosis of the IDH genotype in gliomas.
|
16:39
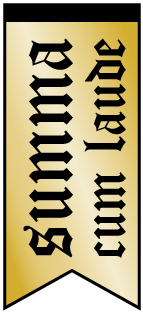 |
0895.
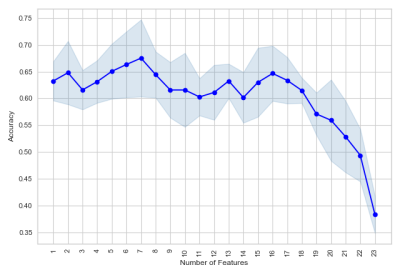 |
Biomarkers for CTE diagnosis in retired NFL player using Machine learning
Marcia Louis, Michael Alosco, Benjamin Rowland, Huijin Liao, Joseph Wang, Ajay Joshi, Robert Stern, Alexander Lin
Multiple concussions have the potential to develop Chronic Traumatic Encephalopathy (CTE), a neurodegenerative disease that is currently diagnosed only in postmortem by tau protein deposition in the brain. Since repetitive head trauma alters brain morphology and metabolism, magnetic resonance imaging and spectroscopy could be suitable candidates for CTE diagnosis. Therefore, we propose machine learning-based approach to identify CTE-related biomarkers. The model achieves 80% prediction accuracy with AUC of 0.72 using creatine, macromolecules and brain volume as features for the machine learning model.
|
16:51
|
0896.
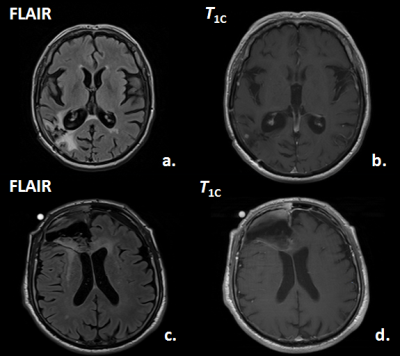 |
Prediction of Tumor Progression Time Interval in Malignant Glioma Using Textural Information derived from FLAIR and T1c MR-images and Machine Learning
Johannes Slotboom, Urspeter Knecht, Nuno Pedrosa de Barros, Timo Nannen, Martin Zbinden, Ekkehard Hewer, Erik Vassella, Alessia Pica, Philippe Schucht, Jürgen Beck, Andreas Raabe, Jan Gralla, Roland Wiest, Marwan El-Koussy
Glioma-patients get regular neuroradiological MRI-follow-ups to evaluate the tumor-progression-status. In this study it was investigated whether it is possible to predict tumor progression within the next follow-up period, from progression on a longer time-scale. The T1c- and FLAIR-images of two times 20 patients were investigated; one group having stable-disease at two subsequent follow-ups (ST-ST), the second group showed stable-disease during the first but progressive-disease during the second follow-up (ST-PR). By applying machine-learning (random-forests) on textural MRI-information, short-term progression could be predicted with an accuracy of 77.5%. This novel type of information can have an impact on improved personalized-treatment of glioma-patients.
|
17:03
|
0897.
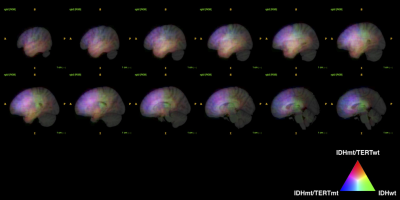 |
Radiogenomics of 154 WHO grade 2 and 3 gliomas via machine learning and the impact of texture analysis
Manabu Kinoshita, Hideyuki Arita, Atsushi Kawaguchi, Masamichi Takahashi, Yoshitaka Narita, Yuzo Terakawa, Naohiro Tsuyuguchi, Yoshiko Okita, Masahiro Nonaka, Shusuke Moriuchi, Junya Fukai, Shuichi Izumoto, Kenichi Ishibashi, Yoshinori Kodama, Kanji Mori, Koichi Ichimura, Yonehiro Kanemura
In this research, the authors performed radiomics for 154 LrGG and attempted to build a MRI based predictive model to classify clinically relevant 3 LrGG subgroups using machine learning algorithm. The impact of texture analysis such as GLCM and GLRLM on building the model was also investigated. Accuracy for predicting 3 molecular subgroups were 0.587 without and 0.546 with texture analysis. Although radiomics was shown to be a powerful tool to identify genetic subgroups of LrGG, little improvement is expected from texture analysis.
|
17:15
|
0898.
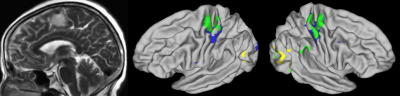 |
Identifying Individual Motor Function Using Machine Learning Predication Based on Resting-State fMRI for Presurgical Mapping in Patients with Brain Tumor
Chen Niu, Elizabeth Zakszewski, Alexander Cohen, Xiao Ling, Ming Zhang, Maode Wang, Yang Wang
A novel machine learning model was developed using resting-state and task fMRI on healthy subjects. This study applied this novel model to clinical patients. Preliminary data on 25 patients with space-occupying brain tumors suggested our approach could accurately predict hand functional area at the individual level in patients with brain tumors, even in cases where patients had displacement of brain tissue and reorganization of brain motor functional network. Our methods implicated the great potential for clinical application of presurgical mapping.
|
17:27
|
0899.
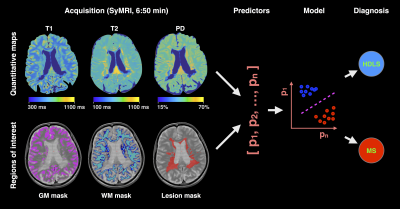 |
Machine learning and rapid multi-parametric relaxometry can differentiate demyelinating disorders with high accuracy
Gabriel Mangeat, Russell Ouellette, Maxime Wabartha, Virginija Karrenbauer, Nikola Stikov, Marcel Warntjes, Nikola Stikov, Caterina Mainero, Julien Cohen-Adad, Tobias Granberg
Hereditary diffuse leukoencephalopathy with spheroids (HDLS) and multiple sclerosis (MS) are both demyelinating and neurodegenerative disorders that can be hard to distinguish clinically and radiologically. Here, we present a machine learning method that relies on rapid multi-parametric relaxometry and volumetry to achieve a robust classification of HDLS vs. MS. Linear discriminant analysis was shown to be a favorable approach compared to non-linear options. A leave-one-out cross-validation show a detection rate of 100% and 0% false positives for both conditions, which suggests that computer-assistance maybe helpful in accurately diagnosing these disorders.
|
17:39
|
0900.
 |
Early Prediction of Language Deficits in Very Preterm Infants Using Functional Connectome Data and Machine Learning
Lili He, Hailong Li, Nehal Parikh
Children who are born prematurely are at an increased risk for impaired neurodevelopmental outcomes, including language deficits. Earlier identification, soon after birth, of infants who are experiencing difficulties with complex language function is urgently needed to take advantage of critical windows of brain development so that targeted delivery of Early Intervention therapies can be undertaken during this optimal period. We propose to develop a robust machine learning framework that can analyze functional brain connectome data obtained at term corrected age to make an individual-level prediction about language outcomes at two years corrected age in very preterm infants.
|
17:51
|
0901.
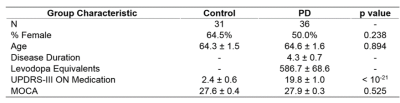 |
Machine learning classification of Parkinson’s disease using brainstem MRI and demographic features
Daniel Huddleston, Babak Mahmoudi, Jason Langley, Mark Connolly, Stewart Factor, Bruce Crosson, Xiaoping Hu
Objective biomarkers for Parkinson’s disease (PD) are needed, and a PD MRI diagnostic could have high impact in clinical and research applications. 3T MRI sequences sensitive to neuromelanin loss and iron accumulation in substantia nigra pars compacta and locus coeruleus robustly detect PD effects. We hypothesized that a multivariate MRI classifier can differentiate PD from controls with high accuracy. A machine learning classifier was developed using data from PD and controls (n=67) with brainstem MRI and demographic features as model inputs. Using 5-fold cross-validation the model demonstrated 86% accuracy, which is in a clinically useful range and warrants further development.
|
18:03
|
0902.
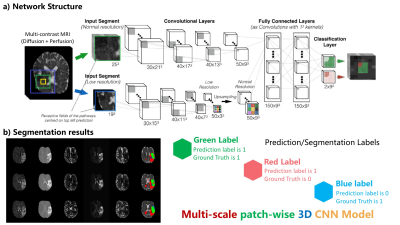 |
Improved Prediction of the Final Infarct from Acute Stroke Neuroimaging Using Deep Learning
Yilin Niu, Enhao Gong, Junshen Xu, Thoralf Thamm, John Pauly, Greg Zaharchuk
Magnetic Resonance Imaging (MRI) is a widely-used technique for clinics. Its advantages in providing multiple complimentary contrasts make it the best image tool for detecting presenting lesions in the brain. A lot methods have been proposed for lesion detection and segmentations using machine learning techniques. It is more sophisticated than common computer vision tasks since the estimation of treatment outcomes are not merely determined by lesions captured by current MR images. We targeted to develop an algorithm, based on 3D Convolutional Neural Network, to predict the final lesion shown on day-90 scans by processing the day-0 acute stroke images.
|
|