Segmentation & Parcellation in the Brain
Oral
Neuro
Wednesday, 20 June 2018
N03 |
08:15 - 10:15 |
Moderators: Anders Dale, Timothy Shepherd |
08:15
|
0693.
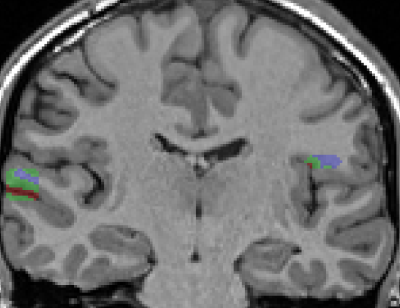 |
Self-Organising Maps Enable Global Searching For Patch Based Segmentations
Lee Reid, Alex Pagnozzi, Suzannah Cooper, Stephen Rose, Jurgen Fripp
Most automated MRI tissue-segmentation techniques require whole-brain atlases, which are extremely time-consuming to manually delineate. This inhibits utilisation of complementary structural sequences during segmentation as matching atlases rarely exist. We demonstrate a means of reducing the computational complexity of patch-based segmentation using self-organising maps. This overcomes the need for local-only searching of matching patches and, in turn, the need for complete atlases. We demonstrate this technique’s ability to perform registration-free tissue-segmentation of target images using an atlas that is less than 2% complete. The technique may be used as-is, or for rapidly generating novel whole-brain atlases for other techniques.
|
08:27
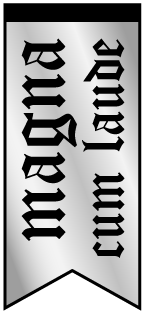 |
0694.
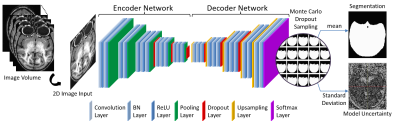 |
Bayesian Deep Learning for Uncertainty Generation in MR Image Segmentation
Gengyan Zhao, Fang Liu, Mary Meyerand, Rasmus Birn
The ability of generating model uncertainty for a predictive system on each prediction is crucial for decision-making, especially in the field of medicine, but it has been a missing part in conventional deep learning models. We propose the utilization of Bayesian deep learning, which combines Monte Carlo dropout layers with the original deep neural network at testing time to enable model uncertainty generation. Its prediction accuracy and the behavior of uncertainty were studied on MRI brain extraction. Its segmentation accuracy outperforms 6 popular methods, and the uncertainty’s reactions to different training set sizes and inconsistent training labels meet the expectation well.
|
08:39
|
0695.
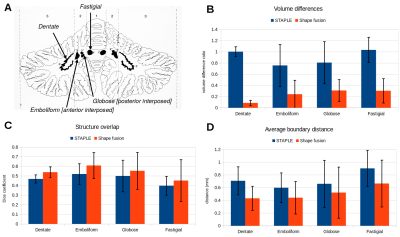 |
Automated Segmentation of Cerebellar Nuclei from Ultra-High-Field Quantitative Susceptibility Maps with Multi-Atlas Shape Fusion
Pierre-Louis Bazin, Andreas Deistung, Andreas Schäfer, Robert Turner, Jürgen Reichenbach, Dagmar Timmann
Multi-atlas segmentation techniques fail to properly represent very small nuclei because of their low overlap in the fusion stage. We present a shape modeling approach that recovers more accurately such small structures, which we apply to the segmentation of the deep cerebellar nuclei.
|
08:51
|
0696.
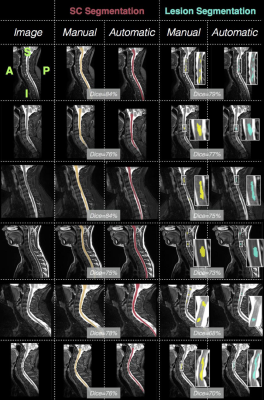 |
Automatic MS lesion segmentation in the spinal cord using deep learning
Charley Gros, Atef Badji, Josefina Maranzano, Ren Zhuoquiong, Yaou Liu, Elise Bannier, Anne Kerbrat, Gilles Edan, Pierre Labauge, Virginie Callot, Jean Pelletier, Bernard Audoin, Henitsoa Rasoanandrianina, Paola Valsasina, Massimo Filippi, Rohit Bakshi, Shahamat Tauhid, Ferran Prados, Marios Yiannakas, Hugh Kearney, Olga Ciccarelli, Sridar Narayanan, Julien Cohen-Adad
Detection of multiple sclerosis (MS) lesions in the spinal cord is clinically important for diagnosis and disease progression assessment. Although several automatic segmentation methods have been proposed for brain lesions, these methods cannot be directly applied to spinal lesions. We propose a fully automatic pipeline based on deep learning to segment the spinal cord and spinal MS lesions, and validate it against a dataset of T2-w images (265 patients from 5 centers). The proposed cord segmentation achieved better results than the current state-of-the-art, and lesion segmentation yielded a median Dice of 63.4%. The pipeline will be available as open-source.
|
09:03
|
0697.
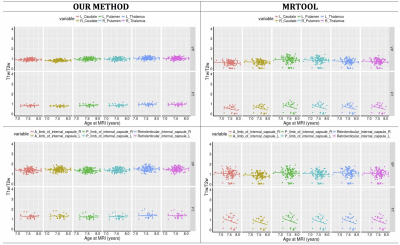 |
Automated segmentation of calibration regions for pediatric whole brain T1- and T2-weighted ratio myelin mapping
Jian Chen, Joseph Yang, Michelle Wu, Simone Mandelstam, Richard Leventer, Deanne Thompson, Bonnie Alexander, Michael Kean, Peter Anderson, Marc Seal, Richard Beare
Delineation of calibration regions is a key component of the T1w/T2w ratio myelin mapping approach. The existing method implemented in SPM was not designed for pediatric MRI application. We have developed an automated approach that is able to reliably segment appropriate calibration regions in the pediatric populations- the CSF and scalp fat layer. Using two pediatric MRI datasets, we demonstrated reliability of our segmentation method and the low variance of regional T1w/T2w ratios than the existing method. Our proposed calibration method has potential to be implemented in pediatric myelination studies using the whole brain T1w/T2w ratio technique.
|
09:15
|
0698.
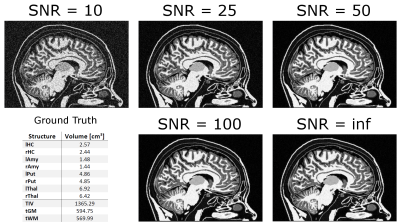 |
Brain Tissue Segmentation and Subcortical Parcellation: How Reliable Are Different Tools?
Ariane Fillmer, Andre Kuehne, Laura Goeschel, Theresa Köbe, Agnes Flöel, Bernd Ittermann
Volume based morphometry is a well-established tool to investigate changes of brain structures in neurodegenerative diseases, and a variety of implementations is available. With MRI investigations as an indispensable instrument for diagnosis and disease progress monitoring, the question about reliability and robustness of these tools arises. This work examines the accuracy of CAT 12 and FreeSurfer by comparing a selection of their calculated subcortical volumes to a ground truth.
|
09:27
|
0699.
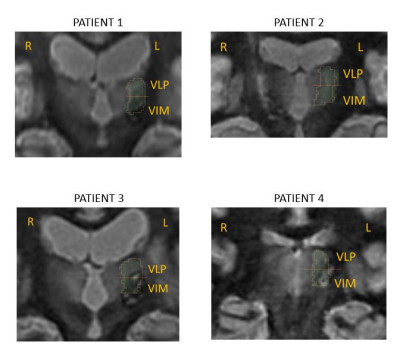 |
A novel strategy for automated near-real-time segmentation of the ventral-intermediate (VIM) nucleus for deep brain stimulation (DBS) surgery
Francis Tyson Thomas, Willard Kasoff, Manojkumar Saranathan
Direct visualization of thalamic nuclei using MRI has been challenging for applications such as Deep Brain Stimulation (DBS) surgery for treatment of essential tremor, where the ventral intermediate (VIM) nucleus is targeted. Current methods are mainly based on Diffusion Tensor Imaging (DTI) which is limited by low spatial resolution and distortion of EPI as well as manual post processing such as seeding specific areas to identify the cerebro thalamic tract. We have developed a novel strategy that combines a white-matter nulled MPRAGE sequence followed by fast near-real time automated segmentation of thalamic nuclei, which can be tailored for each patient for accurate targeting of the VIM nucleus.
|
09:39
|
0700.
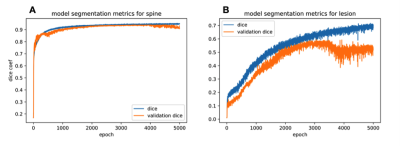 |
Convolutional neural network based segmentation of the the spinal cord and intramedullary injury in acute blunt spinal cord trauma
David McCoy, Sara Dupont, Charley Gros, Jared Narvid, Julien Cohen-Adad, Jason Talbott
This study aims to develop and validate a convolutional neural network for automatic segmentation of the spinal cord (SC) and intramedullary injury in acute blunt SC trauma patients. Using image augmentation of the axial slice cross section and U-net architecture, we were able to achieve a dice coefficient for SC segmentation of 0.92. The same network architecture was also able to identify areas of intramedullary injury. This is the first study to accurately segment the acute blunt trauma SC. Automatic segmentation of the SC in this population makes automatic biomarker analysis and quantitative prognostication of outcomes possible for SC injury.
|
09:51
|
0701.
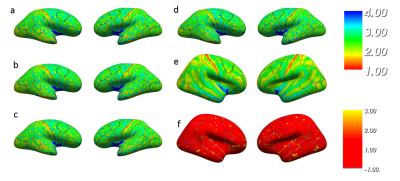 |
High resolution T1-weighted brain imaging and segmentation at 7T: a travelling head study
Olivier Mougin, William Clarke, Ian Driver, Catarina Rua, Adrian Carpenter, Susan Francis, Keith Muir, Richard Wise, Stuart Clare, Richard Bowtell
Ultra-high magnetic field (7T) MRI scanners can provide high spatial resolution images and excellent contrast for classifying brain tissue in vivo, but imaging reproducibility and tissue segmentation between sites is key for multi-site studies. Here, we present a travelling-head study focusing on the harmonized acquisition and segmentation of T1-weighted images acquired on three subjects at 0.7mm3 isotropic resolution at four different 7T sites. The aim of the study is to assess the harmonisation and robustness of the MPRAGE and MP2RAGE sequence across sites, by focusing on segmentation reproducibility and T1 estimation.
|
10:03
|
0702.
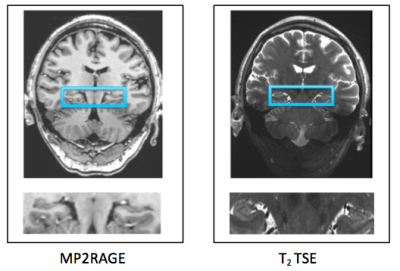 |
First Application of Automated Hippocampal Subfield Segmentation using 7T MRI in Patients with Major Depressive Disorder
Judy Alper, Rui Feng, Hadrien Dyvorne, Long Xie, Marin Kautz, Hung-Mo Lin, Bradley Delman, Patrick Hof, James Murrough, Priti Balchandani
Major depressive disorder (MDD) is a debilitating illness, which is widely prevalent. There is a need to elucidate MDD pathophysiology to better target treatment. Studies have shown association between hippocampal subfield volumes and MDD, making the subfields potential biomarkers. We use high-resolution 7T-MRI to perform effective subfield delineations and evaluate subfield volume differences between sixteen patients and sixteen controls. Using automatic segmentation of hippocampal subfields software revealed a trend towards reduced right-CA1 and right-DG subfield volumes in patients compared to controls. Identifying hippocampal subfield volumes as imaging biomarkers for MDD may help design more targeted treatments for the disease.
|
|