Digital Poster Session Acquisition, Reconstruction & Analysis Back to Program-at-a-Glance
|
Thursday, 16 May 2019
Digital PosterAcquisition, Reconstruction & Analysis
4719 -4743 Segmentation 1
4744 -4768 Image Reconstruction I
4769 -4793 Machine Learning for Image Reconstruction: Optimised
4794 -4818 Segmentation 2
4819 -4843 Software & Tools
4844 -4868 Machine Learning for Image Enhancement, Quality Assessment & Synthetic Image Generation
4869 -4893 Machine Learning for Prediction & Image Analysis |
|
Segmentation 1
Digital Poster
Acquisition, Reconstruction & Analysis
Thursday, 16 May 2019
Exhibition Hall |
13:45 - 14:45 |
|
|
Computer # |
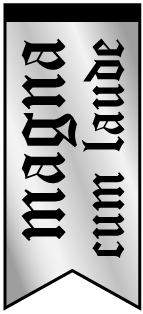 |
4719.
 |
1 |
Automated femoro-tibial cartilage segmentation of OA patients with and without bone abnormality

Rafeek Thaha, Sandeep Jogi, Sriram Rajan, Vidur Mahajan, Vasantha Venugopal, Amit Mehndiratta, Anup Singh, Dharmesh Singh, Neha Vats
The study of knee cartilage under subchondral abnormality is important in osteoarthritis (OA) progression studies. However, cartilage segmentation for patients with Bone-Marrow-Edema (BME) lesion, particularly using radial-search based approach, is erroneous. In this study, a framework for automatic segmentation of femoro-tibial cartilage of OA patients with and without bone abnormality, based on modified radial-search approach and T2-map values is developed. A 2D projected view of T2-map and thickness values of cartilage was generated. Proposed method was successfully applied on 23 MRI patient data. Dice-coefficient for cartilage segmentation was ~82% for OA patients with and without BME lesions.
|
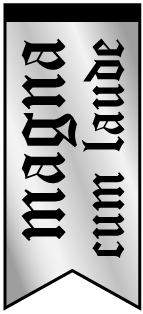 |
4720.
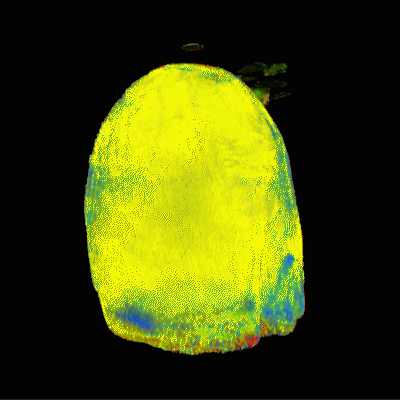 |
2 |
Performant summative 3D rendering of voxel-wise MRF segmentation data

Andrew Dupuis, Debra McGivney, Rasim Boyacioglu, Dan Ma, Anagha Deshmane, Mark Griswold
Visualization of Magnetic Resonance Fingerprinting segmented data presents significant difficulty because of the abstraction from the usual appearance and contrast of MR images. We present a method of rendering any probability-based tissue fraction partial volume ROIs in three dimensions using additive voxelized volumetric rendering as a form of segmentation. Datasets consist of n groups of segmented maps with each voxel representing the probability of a given tissue converted into 3D textures usable by the GPU to perform raymarched additive rendering. This allows for different tissue classifications within the dataset to be faded in and out with minimal human involvement.
|
|
4721.
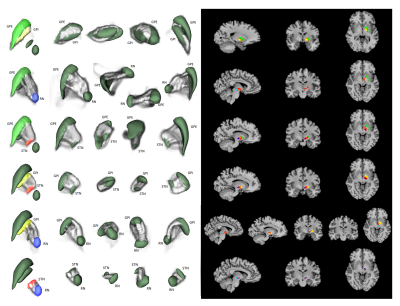 |
3 |
Segmentation and probabilistic tractography of GPi, GPe, STN and RN using Lead-DBS and FSL

Jae-Hyuk Shim, Hyeon-Man Baek
Lead-DBS toolbox is used to segment globus pallidus internal, globus pallidus external, subthalamic nucleus and red nucleus, all of which are structures not automatically segmented by popular toolboxes such as FSL and Freesurfer. In addition, FSL's diffusion toolbox was used to generate probabilistic tractography between each segmented structure as well as compare the level of connectivity between each segmented structure.
|
|
4722.
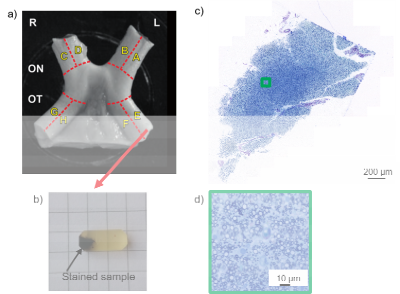 |
4 |
Deep learning segmentation (AxonDeepSeg) to generate axonal-property map from ex vivo human optic chiasm using light microscopy

Thibault Tabarin, Maria Morozova, Carsten Jaeger, Henriette Rush, Markus Morawski, Stefan Geyer, Siawoosh Mohammadi
Development of in-vivo histology using MRI needs validation strategies with gold standard methods. Ex-vivo histology combined with microscopy could become such a strategy; however, for comparing larger field-of-views automatic segmentation of axons and myelin will be required. State-of-the-art segmentation has recently involved deep learning (DL). In this work, we investigated the recently published AxonDeepSeg deep learning algorithm (ADS). We successful applied ADS on light microscopy images of an optical chiasm sample, improved the segmentation of myelin to access the full properties of individual fibers, and finally created microstructural maps such as the histology g-ratio map.
|
|
4723.
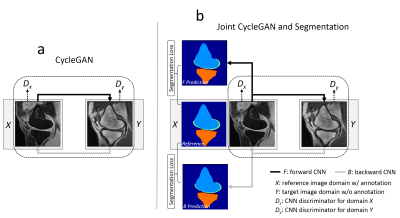 |
5 |
Segment Unannotated MR Image Dataset using Joint Image Translation and Segmentation Adversarial Network

Fang Liu
The purpose of our study was to develop and evaluate a generalized CNN-based method for fully-automated segmentation of different MR image datasets using a single set of annotated training data. A technique called cycle-consistent generative adversarial network (CycleGAN) is applied as the core of the proposed method to perform image-to-image translation between MR image datasets with different tissue contrasts. A joint segmentation network is incorporated into the adversarial network to obtain additional segmentation functionality. The proposed method was evaluated for segmenting bone and cartilage on two clinical knee MR image datasets acquired at our institution using only a single set of annotated data from a publicly available knee MR image dataset. The new technique may further improve the applicability and efficiency of CNN-based segmentation of medical images while eliminating the need for large amounts of annotated training data.
|
|
4724.
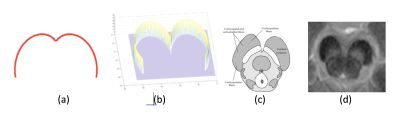 |
6 |
Automated Segmentation of Substantia Nigra in Neuromelanin-Sensitive Magnetic Resonance Imaging

Touseef Ahmad Qureshi, Cody Lynch, Elliot Hogg, Tina Wu, Michele Tagliati, Debiao Li, Zhaoyang Fan
Accurate segmentation of Substantia Nigra (SN) in Neuromelanin-Sensitive MRI (NM-MRI) is a prerequisite for efficient quantification and evaluation of severity of Parkinson disease. We present a fully automated algorithm for localization and segmentation of SN in NM-MRI. The localization algorithm uses a new specialized template matching model consisting of a resizable cardioid plane. The segmentation of SN is performed using freeform active contour segmentation model. The system is tested on 19 NM-MRI scans (10 healthy volunteers and 9 patients with Parkinson disease), acquired using 3T MRI system. The success rate for localization is 98.2%, whilst dice coefficient for segmentation reaches 0.89.
|
|
4725.
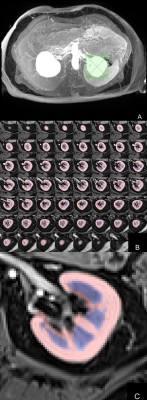 |
7 |
Rapid virtually automated technique for renal corticomedullary segmentation from volumetric arterial phase imaging: Initial experience

Kane Nicholls, Julia Williams, Lucy McKenna, Julie Smith, Emma Hornsey, Elif Ekinci, Leonid Churilov, Henry Rusinek, Artem Mikheev, Ruth Lim
Efficient, reproducible and accurate corticomedullary renal segmentation is challenging but important for MR renography and disease monitoring. We assessed segmentation time, reproducibility and accuracy of a virtually automated (VA) approach (<5 second user interaction), compared to gold standard (GS) manual segmentation. Segmentation time per subject (n=11) was 78.6±7.0s for VA and 60-120min for GS. VA intra- and inter-rater agreement was near perfect for cortex, medullary and whole kidney segmentation (concordance correlation coefficient all ≥0.99), with excellent concordance with GS segmentation (CCC all >0.80). VA is a rapid, accurate and highly reproducible corticomedullary segmentation tool which has promising clinical potential.
|
|
4726.
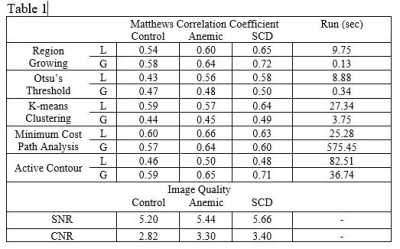 |
8 |
Performance of Automatic Cerebral Arterial Segmentation of MRA Images Improves in Patients with Anemia and Sickle Cell Disease Compared with Healthy Volunteers.

Alexander Saunders, John Wood, Matthew Borzage
Sickle cell disease (SCD) and chronic anemia cause morphological abnormalities in the cerebral arterial vasculature that are observable using time-of-flight magnetic resonance angiography (MRA). We seek to evaluate the accuracy of automatic vessel segmentation algorithms in extracting vessel data from these images for further analysis. Five segmentation algorithms were applied to three MRA images (one control, one anemic, and one SCD patient) and performance was measured against manually segmented ground truth data. We found that automatic segmentation performs better in anemic and SCD patients over healthy controls.
|
|
4727.
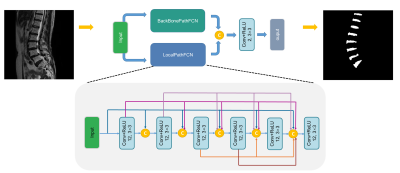 |
9 |
Automated intervertebral disc segmentation using a two-pathway network Presentation Not Submitted
Fei Gao, Shui Liu, Xiaodong Zhang, Jue Zhang, Xiaoying Wang
We developed a two-pathway fully convolutional network for refined intervertebral disc segmentation. The proposed pooling free subbranch can capture more local fine-grained features. The quantitative results indicate its priority for disc segmentation.
|
|
4728.
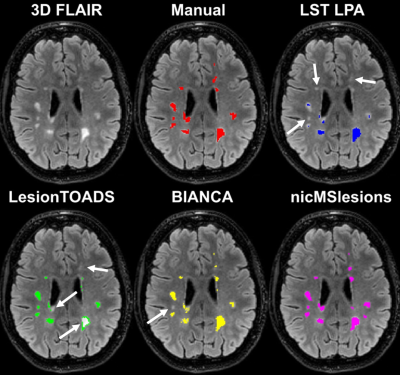 |
10 |
The deep learning lesion segmentation method nicMSlesions only needs one manually delineated subject to outperform commonly used unsupervised methods

Merlin Weeda, Iman Brouwer, Marlieke de Vos, Myrte de Vries, Frederik Barkhof, Petra Pouwels, Hugo Vrenken
Automatic lesion segmentation is important for measurements of atrophy and lesion load in subjects with multiple sclerosis (MS). Although supervised methods perform overall better than unsupervised methods, they are not widely used since they are more labor-intensive due to the need for great amounts of manual input. Our research showed increased performance of supervised methods over unsupervised methods. In addition, when using a deep learning based supervised method, training on only one subject already outperformed the commonly used unsupervised methods. We therefore recommend using deep learning lesion segmentation methods in MS research.
|
|
4729.
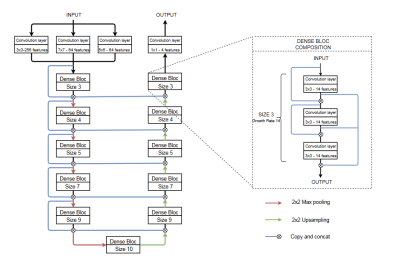 |
11 |
Propagation Neural Network for cardiac segmentation

Benjamin Roussel, Julien Oster, Mattias Heinrich
To perform a fully-automated segmentation of cardiac volumes, current Convolutional Neural Networks (CNNs) process each slice independently, not taking the depth information into consideration. Networks using 3D convolutions being memory-hungry, we propose a CNN model with a low memory demand and processing the whole volume. The network is based on propagating the redundant depth information from slice to slice. Following a 4-fold cross validation on the MICCAI/ACDC challenge dataset, our network obtained better results than a standard 2D network, improving the average DICE score of 1.7% computed over three cardiac structures (myocardium, left and right ventricle).
|
|
4730.
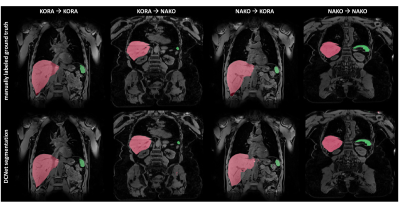 |
12 |
Automated organ segmentation of liver and spleen in whole-body T1-weighted MR images: Transfer learning between epidemiological cohort studies

Thomas Kuestner, Sarah Müller, Marc Fischer, Martin Schwartz, Petros Martirosian, Bin Yang, Fritz Schick, Sergios Gatidis
Automated segmentation of organs and anatomical structures is a prerequisite for efficient analysis of MR data in large cohort studies with thousands of participants. The feasibility of deep learning approaches has been shown to provide good solutions. Since all these methods are based on supervised learning, labeled ground truth data is required which can be time- and cost-intensive to generate. This work examines the feasibility of transfer learning between similar epidemiological cohort studies to derive possibilities in reuse of labeled training data.
|
|
4731.
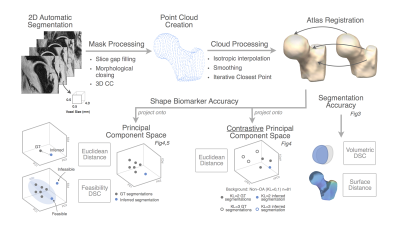 |
13 |
Beyond Dice Coefficient: Evaluating Shape Biomarker Preservation in Neural Network Segmentations

Claudia Iriondo, Valentina Pedoia, Michael Girard, Sharmila Majumdar
High accuracy scores in volumetric overlap metrics, such as Dice Similarity Coefficient, have not been proven to be reliable indicators of shape biomarker preservation. This study proposes a novel approach towards quantitative evaluation of segmentations from neural networks using PCA and contrastive PCA.
|
|
4732.
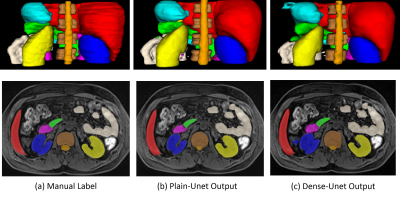 |
14 |
Fully Automatic Learning-based Multi-Organ Segmentation(ALMO) in abdominal MRI for Radiotherapy Planning using Deep Neural Networks

Yuhua Chen, Yujin Xie, Lixia Wang, Jiayu Xiao, Zixin Deng, Yi Lao, Richard Tuli, Debiao Li, Wensha Yang, Zhaoyang Fan
Precise dose measurement is critical in radiotherapy planning, which involves accurate and fast segmentation of the organ for estimation of the region at risk. Segmentation Magnetic Resonance Imaging (MRI), as it is gaining more favor against CT in radio therapy, is new for multi-organ segmentation task. In this work, we proposed a fast, accurate, and fully automatic technique (ALMO) that reliefs the intense human labor from manual segmentation in a timing fashion. On our 51-subject dataset, our proposed method achieves an average dice score of 0.76 in the test set in seconds.
|
|
4733.
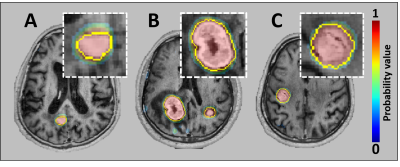 |
15 |
Automatic Detection and Segmentation of Brain Metastases using Deep Learning on Multi-Modal MRI: A Multi-Center Study

Endre Grøvik, Darvin Yi, Michael Iv, Elizabeth Tong, Kyrre Emblem, Line Nilsen, Cathrine Saxhaug, Kari Jacobsen, Åslaug Helland, Daniel Rubin, Greg Zaharchuk
In recent years, many deep learning approaches have been developed and tested for automatic segmentation of gliomas. However, few studies have shown its potential for use in patients with brain metastases. Deep learning may ultimately aid radiologists in the tedious and time-consuming task of lesion segmentation. The objective of this work is to assess the clinical potential and generalizability of a deep learning technique, by training and testing a convolutional neural network for segmenting brain metastases using multi-center data.
|
|
4734.
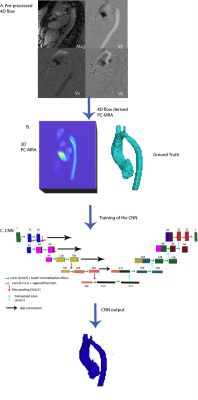 |
16 |
3D U-Net for Automated Segmentation of the Thoracic Aorta in 4D-Flow derived 3D PC-MRA

Haben Berhane, Michael Scott, Joshua Robinson, Cynthia Rigsby, Michael Markl
We developed a 3D convolutional neural network for the automatic segmentation of the thoracic aorta in 4D Flow-derived 3D PC-MRAs. Using 100 testing datasets, we obtained an average dice score of 0.94±0.03 and an average voxel-wise accuracy of 0.99. Additionally, our algorithm is robust enough to accurately segment a wide array of aortic geometries and disease, such as bicuspid aortic value, coarctation, and interrupted aortic arches.
|
|
4735.
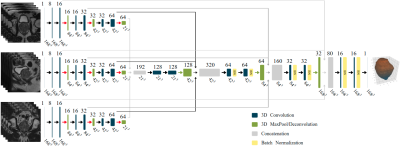 |
17 |
Prostate and peripheral zone segmentation on multi-vendor MRIs using Deep Learning

Olmo Zavala-Romero, Adrian Breto, Nicole Gautney, Yu-Cherng Chang, Alan Dal Pra, Mattew Abramowitz, Alan Pollack, Radka Stoyanova
A Deep Learning algorithm for automatic segmentation of the prostate and its peripheral zone (PZ) is investigated across MR images from two MRI vendors. The proposed architecture is a 3D U-net that uses axial, coronal, and sagittal MRI series as input. When trained with Siemens MRI, the network achieves a Dice similarity coefficient (DSC) of .91 and .76 for the segmentation of the prostate and the PZ respectively. However, the network performs poorly on a GE dataset. Combining images from different MRI vendors is of paramount importance to pursue a universal algorithm for prostate and PZ segmentation.
|
|
4736.
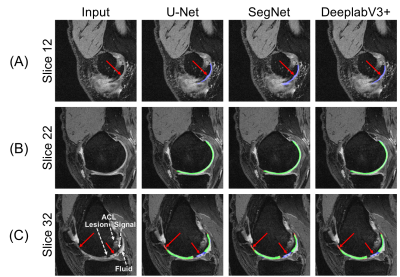 |
18 |
Technical Considerations for Semantic Segmentation in Magnetic Resonance Imaging using Deep Convolutional Neural Networks: A Case Study in Femoral Cartilage Segmentation

Arjun Desai, Garry Gold, Brian Hargreaves, Akshay Chaudhari
Deep convolutional neural networks (CNNs) have shown promise in challenging tissue segmentation problems in medical imaging. However, due to the large size of these networks and stochasticity of the training process, the factors affecting CNN performance are difficult to analytically model. In this study, we numerically evaluate the impact of network architecture and characteristics of training data on network performance for segmenting femoral cartilage. We show that extensive training of several common network architectures yields comparable performance and that somewhat optimal network generalizability can be achieved with limited training data.
|
|
4737
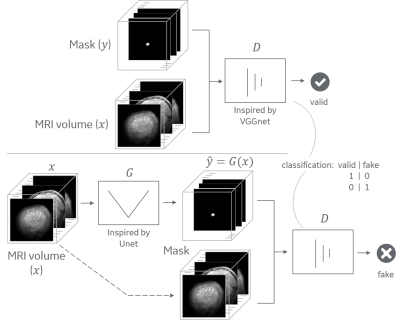 |
19 |
Conditional adversarial network for segmentation with simple loss function Video Permission Withheld
Andre Maximo, Chitresh Bhushan
Most deep-learning approaches require defining a loss function that is appropriate for the task. The choice of the loss function generally substantially affects the accuracy of the trained model and often requires hand-tuning. For example, some segmentation tasks work well with Dice loss while other work well with mean squared error (MSE). In this work we show how conditional adversarial network (cGAN) can be used to avoid defining a specialized loss function for each task and, instead, use a simple approach to achieve comparable or even superior results in context of segmentation of MRI images.
|
|
4738.
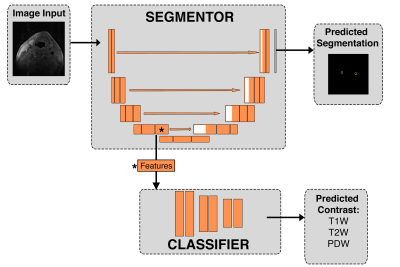 |
20 |
Towards Domain-invariant Carotid Artery Lumen-wall Segmentation Using Adversarial Networks

Anna Danko, Roberto Souza, Richard Frayne
Magnetic resonance (MR) imaging is frequently used for carotid artery wall imaging. The capacity for multi-contrast imaging allows MR scanners to resolve the lumen and wall, as well as multiple plaque components. Combined this information can provide evidence of increased stroke risk. Quantitative analysis of carotid artery MR images regularly begins with the manual segmentation of wall and plaque. This process is time-consuming and costly, and suggests the need for automated methods. Developing a robust segmentation tool is challenging because of the domain shift due to different image contrasts and/or scanners. Here, we demonstrate that a deep learning network including an adversarial component is capable of learning domain-invariant features, thus producing a generalizable segmentation model.
|
|
4739.
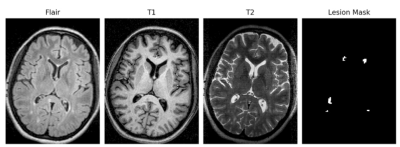 |
21 |
Deep convolutional neural networks for brain lesion segmentation in multiple sclerosis using clinical MRI scans

Sunny Nagam, Glen Pridham, Yunyan Zhang
Machine learning opens up a new opportunity for advancing our image pattern recognition abilities in medical imaging. In this study, we tested the potential of 3 new deep convolutional neural network-based learning methods for detecting brain MRI lesions in multiple sclerosis (MS). Using clinical scans available online from 10 patients, we found that the ResNet and SegNet achieved a promising dice score of 0.65 and 0.61 respectively, better than the generative adversarial network. Deep learning methods may be novel tools for optimal detection of brain MRI lesions, improving the management of patients with MS and similar disorders.
|
|
4740.
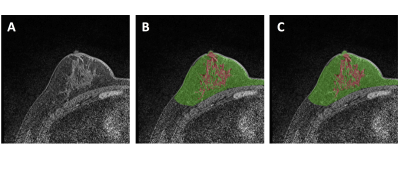 |
22 |
Development of U-Net Breast Density Segmentation Method for Fat-Sat T1-Weighted Images Using Transfer Learning from Model for Non-Fat-Sat Images

Yang Zhang, Jeon-Hor Chen, Kai-Ting Chang, Siwa Chan, Huay-Ben Pan, Jiejie Zhou, Ouchen Wang, Meihao Wang, Min-Ying Su
The U-Net deep learning is a feasible method for segmentation of breast and fibroglandular tissue on non-fat-suppressed (non-fat-sat) T1-weighted images. Whether it can work on fat-sat images, which are more commonly used for diagnosis, is studied. Three datasets were used: 126 Training, 62 Testing Set-A, and 41 Testing Set-B. The model was developed without and with transfer learning based on parameters in the previous model developed for non-fat-sat images. The results show that U-Net can also achieve a high segmentation accuracy for fat-sat images, and when training case number is small, transfer learning can help to improve accuracy.
|
|
4741.
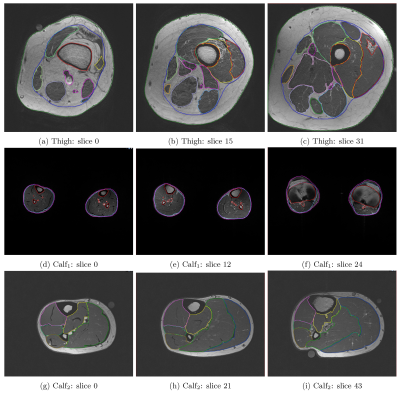 |
23 |
Complete Segmentation of Human Thigh and Calf Muscles/Tissues with Convolutional Neural Network and Partially Segmented Training Images

Chun Kit Wong, Tian Siew Yap, Serene Shi Hui Teo, Maria Kalimeri, Mary Charlotte Stephenson
Quantitative analysis of lower extremity images typically require manual or semi-automated segmentation of regions of interest. This can be extremely time consuming. Here, we utilise DeepLearning and a database of previously segmented thigh and calf t1-weighted images to automatically segment the images into different tissue types and various muscle groups. Dice scores greater than 0.85 were achieved on average across the classes with as few as 40 training images (3D). In addition, we demonstrate a method for training the model with partially labelled images, enabling access to potentially much larger training datasets.
|
|
4742.
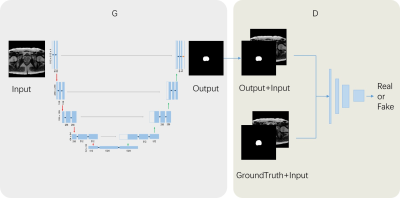 |
24 |
An fully automatic prostate segmentation based on generative adversarial networks

Yi Zhu, Rong Wei, Ge Gao, Jue Zhang, Xiaoying Wang
Automatic prostate segmentation in MR images is essential in many clinical applications. Generative adversarial networks(GAN) have recently gained interests due to their promising ability in generating images which are difficult to distinguish from real images. In this paper, we propose an automatic and efficient algorithm base on GAN to segment the prostate contour and make the prostate segmentation shape more realistic. Our restult shows that the mean segmentation accuracy in test dataset is 90.3%±5.5. It indicates that the proposed strategy is feasible for segmentation of prostate MR images.
|
|
4743.
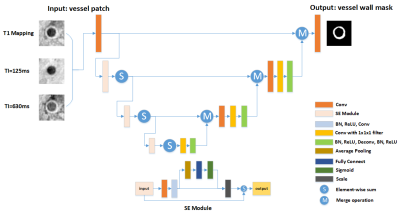 |
25 |
Automatic Segmentation of Carotid Vessel Wall on GOAL-SNAP Images using SE-UNet

Yuze Li, Haikun Qi, Huiyu Qiao, Hualu Han, Xihai Zhao, Chun Yuan, Huijun Chen
In this work, we proposed a deep learning structure called SE-UNet for carotid vessel wall segmentation on 3D golden angle radial k-space sampling simultaneous non-contrast angiography and intraplaque hemorrhage (GOAL-SNAP) images. The structure of network consisted of an encoder path for feature extraction and a decoder path for precise localization. The squeeze-and-excitation (SE) module was introduced to the encoder part to learn the context between channels. The proposed SE-UNet achieved high IOU of 0.786, and high pixel-wise sensitivity of 0.976, specificity of 0.850.
|
|
Top |
Image Reconstruction I
Digital Poster
Acquisition, Reconstruction & Analysis
Thursday, 16 May 2019
Exhibition Hall |
13:45 - 14:45 |
|
|
Computer # |
|
4744.
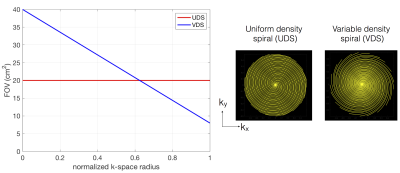 |
26 |
Fast dynamic speech MRI at 3 Tesla using variable density spirals and constrained reconstruction

Sajan Goud Lingala, Douglas Blake, Stanley Kruger, David Meyer, Eileen Finnegan, Ingo Titze, Eric Hoffman
We propose an ultra fast dynamic 3 T MRI scheme for imaging the vocal tract dynamics during speech production. Our approach synergistically exploits efficiency of variable density spirals for motion robustness, artifact suppression, and a sparse SENSE based temporal constrained reconstruction scheme. We realize time resolution of upto 6.2 ms/frame and a spatial resolution of 2.4x2.4 mm2. We demonstrate the utility of this scheme in capturing rapidly varying articulators during fast speech stimuli.
|
|
4745.
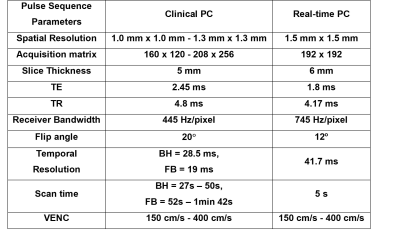 |
27 |
Highly-Accelerated, Real-Time, Phase-Contrast MRI using Radial k-space Sampling and Cartesian GRASP Reconstruction: A Feasibility Study in Pediatric Patients

Hassan Haji-valizadeh, Joshua Robinson, Michael Markl, Cynthia Rigsby, Daniel Kim
Iterative compressed sensing reconstruction of real-time phase-contrast MR images acquired with highly-accelerated radial k-space sampling produces considerable image blurring. We propose a Cartesian Golden-angle radial sparse parallel (GRASP) framework that achieves a good balance between image reconstruction speed and data fidelity. The performance of the proposed reconstruction framework is compared with the original GRASP and GROG-GRASP frameworks using 38.4-fold accelerated phase-contrast MRI data acquired from pediatric patients.
|
|
4746.
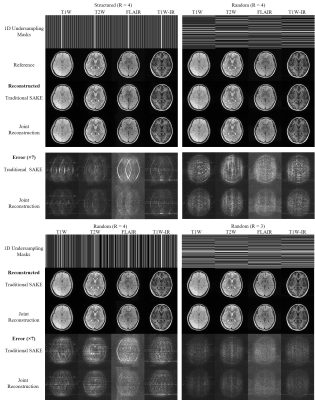 |
28 |
Joint Calibrationless Reconstruction of Highly Undersampled Multi-Contrast MR Datasets Using A Novel Low-Rank Completion Approach

Zheyuan Yi, Yilong Liu, Yujiao Zhao, Fei Chen, Ed X. Wu
Routine clinical MRI session often requires multi-contrast imaging with identical geometries but different contrasts, and these images of different contrasts are independently reconstructed despite ubiquitous similarities. Simultaneous autocalibrating and k-space estimation (SAKE) provides a powerful calibrationless parallel imaging approach to reduce scanning time through undersampling. However, traditional SAKE reconstruction does not utilize redundant information embedded in multi-contrast datasets. In this study, we propose to advance SAKE by jointly reconstructing concatenated multi-contrast datasets using a novel low-rank completion approach. Our new method explicitly exploits the correlations in multi-contrast datasets and outperforms the traditional SAKE, leading to higher acceleration factors.
|
|
4747.
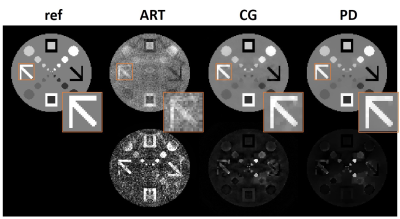 |
29 |
Non-smooth Convex Optimization for O-Space Reconstruction

Jing Cheng, Haifeng Wang, Yuchou Chang, Dong Liang
Non-linear spatial encoding magnetic (SEM) fields can accelerate data acquisitions and improve the image quality. O-Space imaging generates a radially varying SEM field for spatial encoding in order to achieve more efficient encoding. In this work, we introduce and evaluate a novel primal dual algorithm which can handle the inverse problems of non-smooth convex optimization with non-linear forward operators to reconstruct O-Space images from undersampled data. The experimental results on simulated data show that the proposed method can achieve better image quality compared with the existing methods.
|
|
4748.
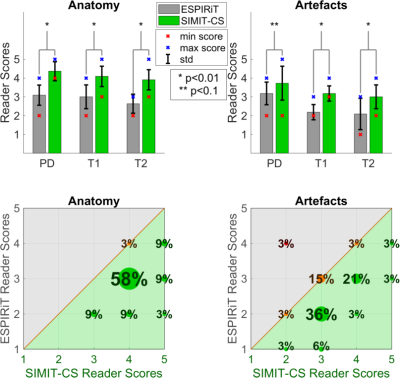 |
30 |
Multi-channel multi-contrast reconstructions via simultaneous use of individual and joint regularization terms

Emre Kopanoglu, Alper Güngör, Toygan Kilic, Emine Saritas, Kader Oguz, Tolga Çukur, H. Güven
Multi-contrast images of the same anatomy are commonly acquired together to maximize diagnostic information. We demonstrate a multi-channel multi-contrast compressed sensing – parallel imaging (CS-PI) technique that simultaneously uses joint and individual regularization terms to exploit anatomical similarities across contrasts without leakage of distinct features across contrasts and that incorporates coil sensitivities to further improve image quality. The method is compared in-vivo to the single-contrast multi-channel CS-PI method l1-ESPIRiT for PD-/T1-/T2-weighted images of N=11 participants using signal-to-noise ratio calculations as well as neuroradiologist reader studies. The proposed method yields superior performance than l1-ESPIRiT both quantitatively and qualitatively.
|
|
4749.
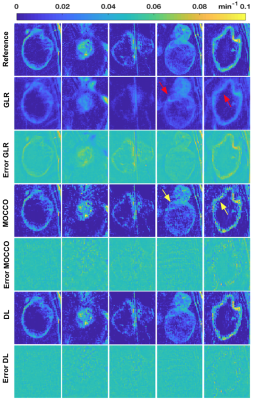 |
31 |
Sparse DCE-MRI using a Temporal Constraint Learned from Clinical Data

Sreedevi Gutta, Yannick Bliesener, Jay Acharya, Meng Law, Krishna Nayak
Dynamic contrast enhanced MRI has benefitted substantially from developments in sparse sampling and constrained reconstruction. Thus far, temporal constraints have proven to be the most powerful. In this work, we explore the use of temporal dictionaries that are learned from a clinical database. We demonstrate that this method provides improved reconstruction quality compared to state-of-the-art TK-model-based constraints or low-rank constraints. The inclusion of spatial information while constructing dictionaries is also explored.
|
|
4750.
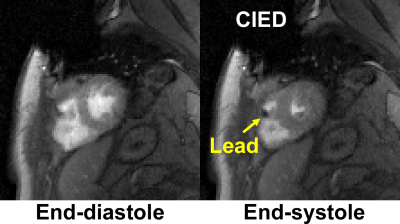 |
32 |
Rapid, Free-Breathing, Cine MRI for Patients with a Cardiac Implantable Electronic Device: A Preliminary Study

KyungPyo Hong, Jeremy Collins, Daniel Lee, Daniel Kim
Standard ECG-gated, breath-held cardiac cine MRI often produces poor image quality in patients with a cardiac implantable electronic device (CIED) due to off-resonance effects, high prevalence of arrhythmia, and/or difficulty in breath-holding. This study seeks to develop a 16-fold accelerated, free-breathing cine MRI pulse sequence using a combination of a gradient echo readout, compressed sensing, and optimal Cartesian k-space sampling. The results from this study shows that an optimal k-space sampling scheme produces superior results compared to random and Poisson disc k-space sampling patterns in imaging phantoms and patients.
|
|
4751.
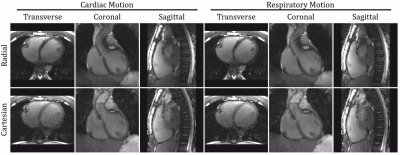 |
33 |
Cardiac and Respiratory Motion-Resolved 5D Imaging Using a Free-Running Framework: Comparison of Cartesian and Radial Trajectories

Christopher Roy, Jerome Yerly, Jessica Bastiaansen, Nemanja Masala, Lorenzo Di Sopra, Jens Wetzl, Christoph Forman, Davide Piccini, Matthias Stuber
Recent advances have enabled high resolution cardiac imaging using continuous acquisitions that do not require external gating devices and can be reconstructed in arbitrary dimensions. Here, we extend the use of this Free-running framework to a fully self-gated free-breathing 3D Cartesian trajectory with spiral profile ordering for cardiac and respiratory motion resolved 5D imaging. We demonstrate the feasibility of this Cartesian approach by reconstructing and comparing images from both radial and Cartesian sequences with matching scan parameters in healthy volunteers. Overall, Cartesian images demonstrated comparable cardiac and respiratory motion albeit with more residual artifacts present in the Cartesian images.
|
|
4752.
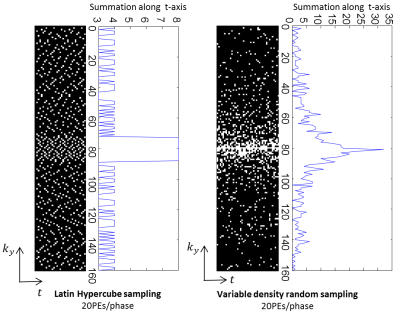 |
34 |
Toward single breath-hold whole-heart coverage compressed sensing MRI using VAriable spatial-temporal LAtin hypercube and echo-Sharing (VALAS)

Jingyuan Lyu, Yu Ding, Jiali Zhong, Zhongqi Zhang, Lele Zhao, Jian Xu, Qi Liu, Ruchen Peng, Weiguo Zhang
The main goal is to design and implement a sampling and reconstruction strategy that enables full heart coverage in a single breath-hold, with a relatively high spatial resolution (2.5 × 2.5 mm2) and temporal resolution (40 ms). The challenge in sampling pattern design is how to sample most efficiently. In this work, we present a 10 fold accelerated real-time cardiac cine MRI pulse sequence using a combination of compressed sensing and parallel imaging.
|
|
4753.
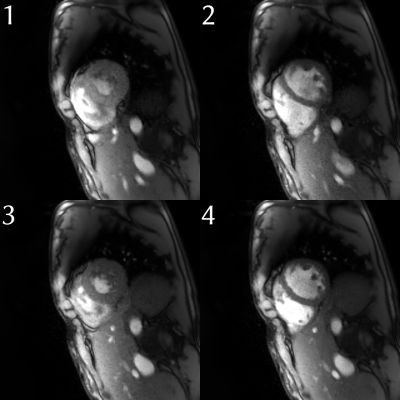 |
35 |
Non-linear Inverse Compressed-Sensing Reconstruction for Self-Gated Multidimensional Cardiac MRI: XD-NLINV

H. Christian M. Holme, Sebastian Rosenzweig, Xiaoqing Wang, Martin Uecker
Motion is a perpetual challenge in cardiac MRI: for comfortable free-breathing exams, both cardiac and breathing motion need to be resolved. Self-gating approaches have been proposed to automatically bin MRI data into appropriate motion states. Here, we propose a new combined parallel imaging/compressed sensing reconstruction for such multi-dimensional datasets. This method, termed XD-NLINV, solves the non-linear parallel imaging problem, simultaneously estimating images and coil sensitivities. This assures efficient use of the available data and removes the need for pre-calculating the coil profiles. We present initial results showing high image quality for self-gated cardiac short-axis data, resolving both breathing and cardiac motion.
|
|
4754.
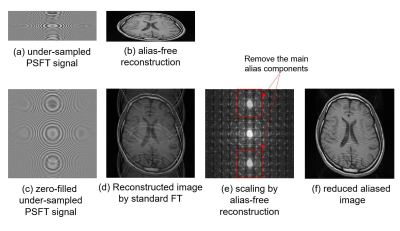 |
36 |
De-Aliasing for Under-sampling in Phase Scrambling Fourier Transform Imaging using Alias-free Reconstruction and Deep Convolutional Neural Network

Satoshi ITO, Tsukasa SAITO
Alias-free image reconstruction is feasible in phase scrambling Fourier transform imaging. When small down-scaling factor is used in that method, the size of reconstructed images become small and aliased image are separated in the scaled space. In this work, a new fast imaging method in which aliasing artifacts due to under-sampling of signal is removed 2-steps; one is down-scaled space introduced by alias-free reconstruction and the second is the denoising using deep convolution network. It was shown that proposed method provide higher PSNR images compared to random sampling compressed sensing and has an advantage in low sampling rate image acquisition.
|
|
4755.
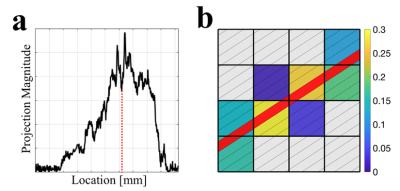 |
37 |
Highly Accelerated Simultaneous Multislice Projection Imaging

Nikolai Mickevicius, L. Tugan Muftuler, Andrew Nencka, Eric Paulson
Projection imaging has many advantages over Cartesian sampling. The unique point spread function makes it particularly useful for highly accelerated parallel imaging and compressed sensing reconstructions[1]. In this study, a projection-domain sensitivity encoding algorithm is developed for highly accelerated simultaneous multislice radial imaging. Since it operates in the projection-domain, no time expensive gridding, de-gridding, and FFT operations are required within each iteration of the solving algorithm. From an in vivo experiment, two slices were reconstructed from only 34 radial spokes.
|
|
4756
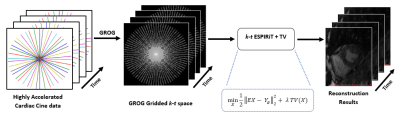 |
38 |
Reconstruction of Highly Accelerated Radial Cardiac Cine MRI using GROG based k-t ESPIRiT with TV Constraint Video Permission Withheld
Ibtisam Aslam, Lindsey CROWE , Miklos KASSAI, Jean-Paul VALLEE , Hammad Omer
Breath-hold cardiac cine MRI requires fast data acquisition with good spatio-temporal resolution. Accelerated non-Cartesian trajectories accelerate data acquisition but lead to artifacts. This work proposes a GROG-based k-t ESPIRiT approach with TV to recover the unaliased MR real-time cine images with good spatio-temporal quality. The proposed method was tested on 8 patients with single breath-hold, short-axis, real-time cardiac cine whole-heart stack with under-sampled radially acquired data using trueFISP. The efficiency of the proposed reconstruction was clinically assessed for automated segmentation, CNR & SNR and compared with the standard image reconstruction available on Siemens 3T PRISMA and 1.5T AERA scanners.
|
|
4757.
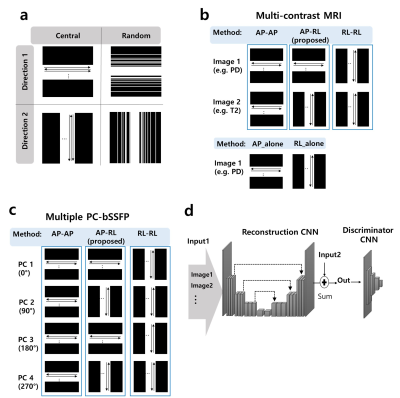 |
39 |
Varying Undersampling Dimension for Accelerating Multiple-Acquisition Magnetic Resonance Imaging

Ki Hwan Kim, Won-Joon Do, Sung-Hong Park
We proposed a new sampling strategy for efficiently accelerating multiple acquisition MRI. The new sampling strategy is to obtain data along different phase encoding directions across multiple acquisitions. The proposed sampling strategy was evaluated in multi-contrast MR imaging (T1, T2, proton density) and multiple phase cycled (PC) balanced steady-state free precession (bSSFP) imaging by using compressed sensing (CS) algorithms and convolutional neural networks (CNNs) with central and/or random sampling pattern. Sampling along different phase encoding directions across multiple acquisitions was advantageous for accelerating multi-acquisition MRI, irrespective of reconstruction method, sampling pattern or datasets, with further improvement through transfer learning.
|
|
4758.
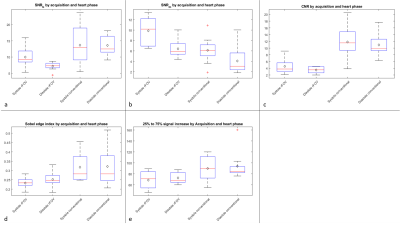 |
40 |
Sliding Window Reduced FOV Reconstruction in EPI for Real-Time Cardiac Imaging

Patrick Metze, Tobias Speidel, Kilian Stumpf, Volker Rasche
In this work we present a reconstruction technique based on kk-space
subtraction of static image parts to acquire real-time cardiac images. The static part is estimated with a sliding window reconstruction of the region outside of the heart to account for respiratory motion. The reduced field of view, i.e. the region of interest, is then reconstructed using a standard SENSE reconstruction, resulting in a temporal resolution of under 40 ms. The image quality is sufficient to estimate functional values in accordance with the BH-CINE reference standard.
|
|
4759.
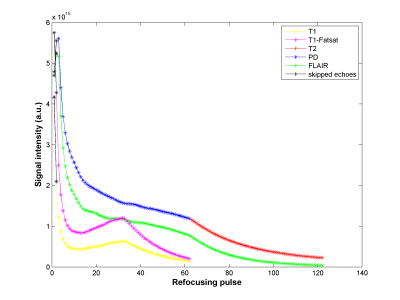 |
41 |
High Resolution 3D Isotropic Multi-Contrast Brain Imaging using APIR4EMC

Chaoping Zhang, Alexandra Cristobal-Huerta, Juan Antonio Hernandez-Tamames, Stefan Klein, Dirk Poot
The long scan time of the brain MRI limits its applicability in high resolution 3D isotropic imaging. By using the recent Autocalibrated Parallel Imaging Reconstruction for Extended Multi-Contrast (APIR4EMC) method, we propose a high resolution (1 mm) 3D isotropic multi-contrast (T1, T1-Fatsat, T2, PD, FLAIR) brain imaging method with scan time around 10 min on a 3T MR scanner with an 8-channel brain coil. Experimental results demonstrate the effectiveness of this method.
|
|
4760
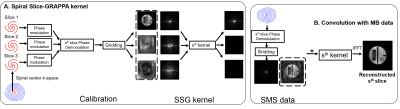 |
42 |
Simultaneous multislice reconstruction using spiral slice-GRAPPA Video Permission Withheld
Changyu Sun, Yang Yang, Daniel Weller, Xiaoying Cai, Craig Meyer, Michael Salerno, Frederick Epstein
Spiral trajectories provide efficient data acquisition and favorable motion properties for cardiac MRI. We developed multiband (MB) methods to accelerate spiral cardiac cine imaging including a non-iterative spiral slice-GRAPPA (SSG) reconstruction and a temporal SSG (TSSG). Using 25-35% of k-space for single-band calibration data, experiments in phantoms and five volunteers show 18.7% lower mean artifact power than CG-SENSE when imaging three slices simultaneously. TSSG incorporating CAIPIRINHA with temporal alternation and a temporal filter in reconstruction further reduced rRMSE by 11.2% compared to SSG.
|
|
4761.
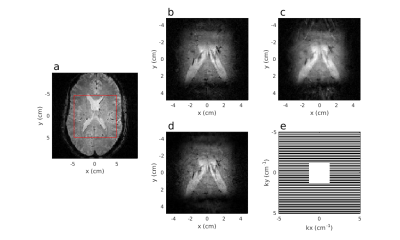 |
43 |
Accelerated Image Acquisition Using 2D Pulse Segments as Virtual Receivers for GRAPPA

Michael Mullen, Alexander Gutierrez, Jarvis Haupt, Michael Garwood
When based on a k-space description, 2D RF pulses can be applied in segments to increase the excitation bandwidth relative to a single-shot implementation, at a cost of increased imaging time. The increased imaging time can be overcome by undersampling the acquisition in one phase-encoded dimension, where data from each segment are viewed as originating from “virtual receive coils” rather than multiple physical coils. The undersampled data are reconstructed using parallel imaging techniques (e.g. as in GRAPPA). The method was tested in vivo with brain imaging, and the GRAPPA-like reconstruction was comparable in quality to a fully sampled reconstruction.
|
|
4762.
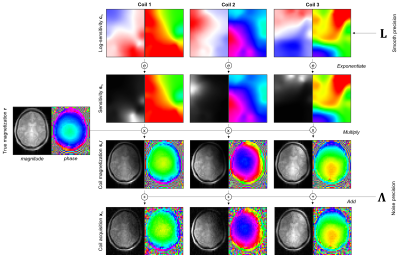 |
44 |
A Generative Approach to Estimating Coil Sensitivities from Autocalibration data

Yael Balbastre, Julio Acosta-Cabronero, Nadège Corbin, Oliver Josephs, John Ashburner, Martina Callaghan
We present an algorithm for inferring sensitivities from low-resolution data, acquired either as an external calibration scan or as an autocalibration region integrated into an under-sampled acquisition. The sensitivity profiles of each coil, together with an unmodulated image common to all coils, are defined as penalised-maximum-likelihood parameters of a generative model of the calibration data. The model incorporates a smoothness constraint for the sensitivities and is efficiently inverted using Gauss-Newton optimization. Using both simulated and acquired data, we demonstrate that this approach can successfully estimate complex coil sensitivities over the full (FOV), and subsequently be used to unfold aliased images.
|
|
4763.
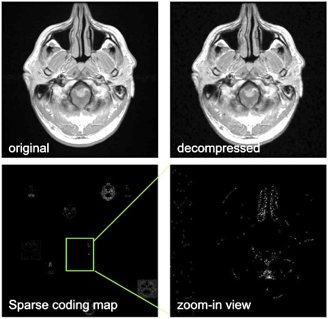 |
45 |
Efficient MR Image Compression using Deep Learning Models for Multi-contrast MRI Presentation Not Submitted
Enhao Gong, Xiaofan Lin, John Pauly
As more and more medical imaging dataset is created, efficient and high-rate data compression is in demand for applications such as data transfer, storage and cloud based MR image analysis. However, conventional compression options do not provide the efficiency and compression performance needed for real-time applications such as image query and computer-aided diagnosis. In this work we demonstrated the applicability of the DL based compression algorithm for MRI to improve the compression performance and efficiency. Trained on natural images and fine-tuned on multi-contrast brain MRI, the proposed method provide significantly (~2x) higher compression rate compared with conventional method. Additionally, the end-to-end deep learning compression/de-compression is also several magnitude's faster than conventional methods. This technique can directly benefit industrial and clinical applications, and can provide new model in applications such as multi-contrast fusion and reconstruction.
|
|
4764.
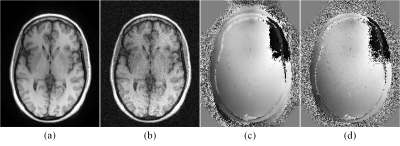 |
46 |
NAPALM: An Algorithm for MRI Reconstruction with Separate Magnitude and Phase Regularization

Yunsong Liu, Justin Haldar
We describe a new algorithm for model-based MRI reconstruction with separate magnitude and phase regularization. The algorithm, named NAPALM, combines the existing proximal alternating linearized minimization (PALM) algorithm for nonsmooth and nonconvex optimization with Nesterov's acceleration and adaptive gradient (AdaGrad) acceleration methods. Results demonstrate the advantages of NAPALM over existing state-of-the-art algorithms.
|
|
4765.
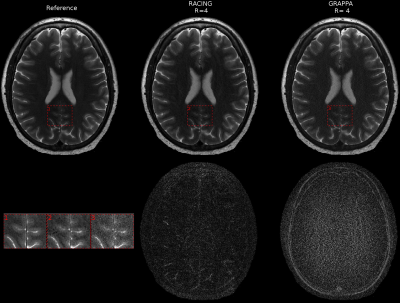 |
47 |
Reconstruction Augmentation by Constraining with Intensity Gradients (RACING)

Ali Pour Yazdanpanah, Onur Afacan, Simon Warfield
Conventional parallel imaging exploits coil sensitivity profiles to enable image reconstruction from undersampled data acquisition. The extent of undersampling that preserves high quality images is limited in part by the total reduction in signal associated with undersampling, and in part by an additional geometry factor that arises from the position of the coil array with respect to the anatomy being imaged. We propose to further constrain the image reconstruction in order to reduce the geometry factor artifact that limits the use of high acceleration factors. We derive equality constraints from the acquisition model that induce a coupling between the signal intensity at a voxel, and the signal intensity at other neighboring voxels. These additional constraints improve the conditioning of the parallel imaging reconstruction by helping to disambiguate aliasing artifact. Consequently, increased undersampling factors have higher image quality. This in turn enables rapid acquisition of 2D and 3D images. Furthermore, these additional constraints are entirely compatible with alternative sources of information to better condition or regularize the image reconstruction. We propose a SENSE formulation of our augmented image reconstruction equations, derive the equality constraints that reduce the g-factor artifact, and solve for the final image using an Augmented Lagrangian numerical formulation. We also indicate how our formulation can be extended to incorporate image prior models by adding regularized reconstruction or sparsity constraints.
|
|
4766.
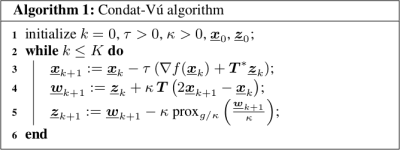 |
48 |
OSCAR-based reconstruction for compressed sensing and parallel MR imaging

Loubna El Gueddari, Emilie Chouzenoux, Jean-Christophe Pesquet, Alexandre Vignaud, Philippe Ciuciu
Compressed sensing combined with parallel imaging has allowed significant reduction in MRI scan time. However, image reconstruction remains challenging and common methods rely on a coil calibration step. In this work, we focus on calibrationless reconstruction methods that promote group sparsity. The latter have allowed theoretical improvements in CS recovery guarantees. Here, we compare the performances of several regularization terms (group-LASSO, sparse group-LASSO and OSCAR) that define with the data consistency term the convex but nonsmooth objective function to be minimized. The same primal-dual algorithm can be used to perform this minimization. Our results demonstrate that OSCAR-based reconstruction is competitive with state-of-the-art ℓ1ℓ1-ESPIRiT.
|
|
4767.
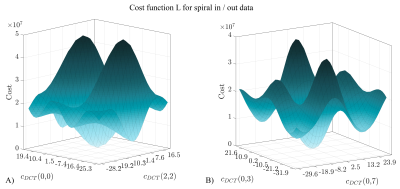 |
49 |
An Advanced Optimization Strategy for Joint Estimation of Object and B0

Franz Patzig, Bertram Wilm, Kasper Lars, Maria Engel, Klaas Pruessmann
A major problem of single-shot acquisition techniques are distortions due to local offsets of the static magnetic B0 field. To avoid relying on separately acquired field maps, the object and the B0 map can be jointly estimated, which usually involves updating object and B0 map in an alternating fashion. A new optimization strategy to solve the non-convex B0 sub-problem is suggested. The number of unknowns is significantly reduced by modelling the B0 maps by a smaller basis and a modified version of the simulated annealing algorithm is implemented to better handle the non-convexity. First in-vivo results are presented.
|
|
4768.
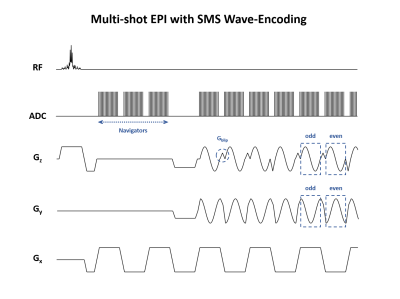 |
50 |
Multi-shot Echo-planar Imaging with Simultaneous MultiSlice Wave-Encoding

JaeJin Cho, HyunWook Park, Kawin Setsompop, Berkin Bilgic
We propose an imaging sequence for Simultaneous MultiSlice Multi-Shot EPI (SMS-MS EPI) with wave-CAIPI controlled aliasing, to significantly reduce the imaging time and geometric distortion in gradient echo imaging. We extend the MUSSELS low-rank constrained parallel imaging technique to SMS acceleration and exploit the similarities among the EPI shots for improved reconstruction. In simulations, we demonstrate the capability of our sequence to incorporate wave-CAIPI encoding, which allows higher acceleration rates by fully harnessing the three-dimensional encoding capability of multi-channel receive arrays. Using MUSSELS with wave-SMS, whole-brain T2*-weighted images at 1 mm isotropic resolution can be obtained at the total acceleration of Rtotal=24 (RinplanexRSMS=8x3), corresponding to an acquisition with high image quality and geometric fidelity.
|
|
Top |
Machine Learning for Image Reconstruction: Optimised
Digital Poster
Acquisition, Reconstruction & Analysis
Thursday, 16 May 2019
Exhibition Hall |
13:45 - 14:45 |
|
|
Computer # |
|
4769.
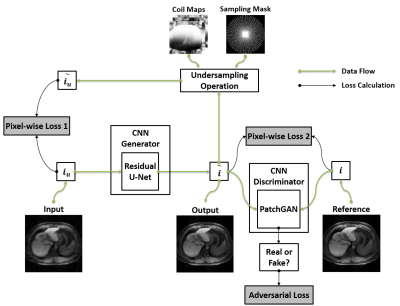 |
51 |
SANTIS: Sampling-Augmented Neural neTwork with Incoherent Structure for efficient and robust MR image reconstruction

Fang Liu, Lihua Chen, Richard Kijowski, Li Feng
The purpose of this work was to develop and evaluate a new deep-learning based image reconstruction framework, termed as Sampling-Augmented Neural neTwork with Incoherent Structure (SANTIS) for MR image reconstruction. Our approach combines efficient end-to-end CNN mapping with k-space consistency using the concept of cyclic loss to enforce data fidelity. Adversarial training is implemented for maintaining high quality perceptional image structure and incoherent k-space sampling is used to improve reconstruction accuracy and robustness. The performance of SANTIS was demonstrated for reconstructing vast undersampled Cartesian knee images and golden-angle radial liver images. Our study demonstrated that the proposed SANTIS framework represents a promising approach for efficient and robust MR image reconstruction at vast acceleration rate.
|
|
4770.
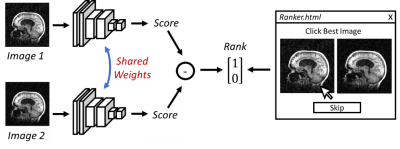 |
52 |
Crowdsourced Quality Metrics for Image Reconstruction using Machine Learned Ranking

Kevin Johnson, Laura Eisenmenger, Patrick Turski, Leonardo Rivera-Rivera
In this work, we investigate a scheme for crowd sourcing image quality using machine learned metrics from user rankings of corrupted images. Using an HTML application, experienced observers ranked pairs of corrupted images with respect to image quality. A convolution neural network (CNN) was then trained to produce a quality score that was higher in the preferred images. The trained CNN was found to be more sensitive to artifacts from image blurring and wavelet compression than mean square error. Finally, preliminary use in training a machine learned image reconstruction is demonstrated.
|
|
4771.
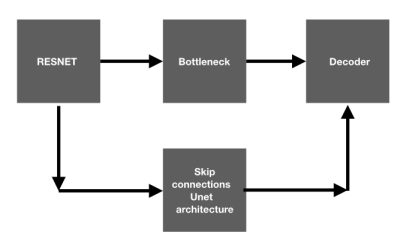 |
53 |
Virtual Imaging Using Generative Adversarial Networks for Image Translation (VIGANIT): Deep Learning based Prediction of Diffusion-Weighted Images from T2-Weighted Brain MR Images Presentation Not Submitted
Vidur Mahajan, Aravind Upadhyaya, Vasantha Kumar Venugopal, Abhishek Venkataram, Mukundhan Srinivasan, Murali Murugavel, Harsh Mahajan
100 whole brain MRI scans of patients with no abnormality and 30 with acute infarcts, comprising of 25 T2-weighted and Diffusion-Weighted (b=1000) images each, were fed into a Deep Learning model with a 75-25 training-validation split. The T2W image was assigned as the input to predict DW images. Binary Cross entropy of 0.15 for normal and 0.11 for infarct cases was obtained and the predicted images were able to successfully delineate acute and chronic infarcts in all test cases.
|
|
4772
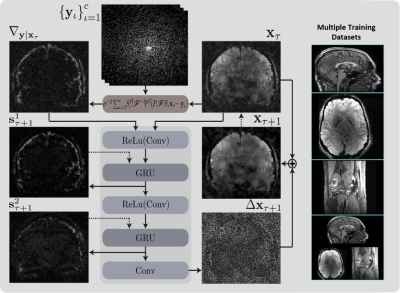 |
54 |
A Deep Learning Accelerated MRI Reconstruction Model's Dependence on Training Data Distribution Video Permission Withheld
Dimitrios Karkalousos, Kai Lønning, Serge Dumoulin, Jan-Jakob Sonke, Matthan Caan
Recurrent Inference Machines (RIM) are deep learning inverse problem solvers that have been shown to generalize well to anatomical structures and contrast settings it was not exposed to during training. This makes RIMs ideal for accelerated MRI reconstruction, where the variation in acquisition settings is high. Using T1- and T2*-weighted brain scans and T2-weighted knee scans, we compare the RIM's performance when trained on only a single type of data against the case where all three data types are present in the training set. We present results that show an overall model robustness, but also indicate a slight preference for training on all three types of data.
|
|
4773.
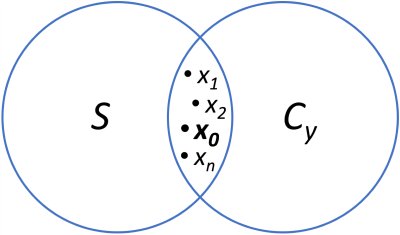 |
55 |
Exploring the Hallucination Risk of Deep Generative Models in MR Image Recovery

Vineet Edupuganti, Morteza Mardani, Joseph Cheng, Shreyas Vasanawala, John Pauly
The hallucination of realistic-looking artifacts is a serious concern when reconstructing highly undersampled MR images. In this study, we train a variational autoencoder-based generative adversarial network (VAE-GAN) on a dataset of knee images and conduct a detailed exploration of the model latent space by generating extensive admissible reconstructions. Our preliminary results indicate that factors such as sampling rate and trajectory as well as loss function affect the risk of hallucinations, but with a reasonable choice of parameters deep learning schemes appear robust in recovering medical images.
|
|
4774.
 |
56 |
DCTV-Net: Model based Convolutional Neural Network for dynamic MRI

Shanshan Wang, Yanxia Chen, Leslie Ying, Cheng Li, Ziwen Ke, Taohui Xiao, Xin Liu, Dong Liang, Hairong Zheng
Compressive sensing MRI (CS-MRI) is a popular technique to accelerate MR dynamic imaging. Nevertheless, the reconstruction is normally time-consuming and its parameters have to be hand-tuned To address this challenge, we solve a CS-based dynamic MR imaging problem by adopting the Alternating Direction Method of Multipliers (ADMM) iteration method with the most popular deep learning technique. Specifically, we introduce a deep network structure, dubbed as DCTV-NET, for dynamic magnetic resonance image reconstruction from highly under-sampled k-t space data. Experimental results demonstrate that our method is superior to the state-of-the-art dynamic MRI methods.
|
|
4775.
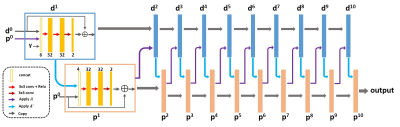 |
57 |
Learning Primal Dual Network for Fast MR Imaging

Jing Cheng, Haifeng Wang, Leslie Ying, Dong Liang
We introduce a novel deep learning network which combines elements of model and data driven approaches for fast MR imaging, termed modified Learned PD. The network is inspired by the first-order primal dual algorithm, where the convolutional neural network blocks are used to learn the proximal operators. Learned PD network works directly from undersampled k-space data and reconstructs MR images by updating in k-space and image domain alternatively. This approach has been evaluated by in vivo MR datasets and achieves accurate MR reconstructions, outperforming other comparing methods across various quantitative metrics.
|
|
4776.
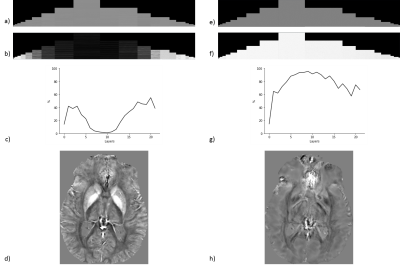 |
58 |
Fidelity Imposing Network Edit (FINE) for Solving Ill-Posed Image Reconstruction

Jinwei Zhang, Zhe Liu, Shun Zhang, Pascal Spincemaille, Thanh Nguyen, Mert Sabuncu, Yi Wang
A Fidelity Imposing Network Edit (FINE) method is proposed for solving inverse problem that edits a pre-trained network's weights with the physical forward model for the test data to overcome the breakdown of deep learning (DL) based image reconstructions when the test data significantly deviates from the training data. FINE is applied to two important inverse problems in neuroimaging: quantitative susceptibility mapping (QSM) and undersampled multi-contrast reconstruction in MRI.
|
|
4777.
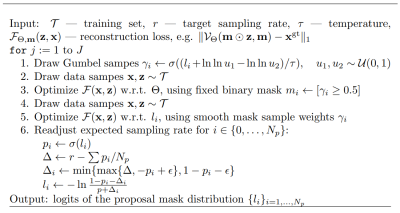 |
59 |
Probabilistic Optimization of Cartesian k-Space Undersampling Patterns for Learning-Based Reconstruction

Valery Vishnevskiy, Jonas Walheim, Sebastian Kozerke
Learning-based methods offer improved reconstruction accuracy for compressed Sensing MRI. However, most modern methods assume the sampling trajectory to be predefined. In order to further increase reconstruction quality, we present a method for adaptive design of Cartesian undersampling masks. The proposed method delivers sampling trajectories that allow to improve reconstruction accuracy by 26% and 6% compared to the random and state-of-the-art interleaved variable density patterns, respectively.
|
|
4778.
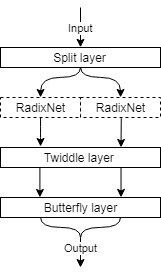 |
60 |
Deep transform networks for scalable learning of MR reconstruction

Anatole Moreau, Florent Gbelidji, Boris Mailhe, Simon Arberet, Xiao Chen, Marcel Dominik Nickel, Berthold Kiefer, Mariappan Nadar
In this work we introduce RadixNet, a fast, scalable, transform network architecture based on the Cooley-Tukey FFT, and use it in a fully-learnt iterative reconstruction with a residual dense U-Net image regularization. Results show that fast transform networks can be trained at 256x256 dimensions and outperform the FFT.
|
|
4779.
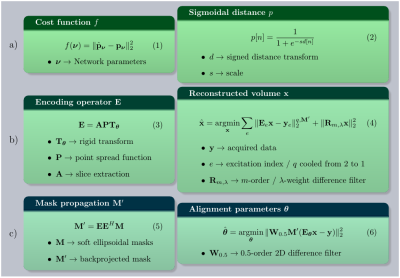 |
61 |
Automating fetal brain reconstruction using distance regression learning

Lucilio Cordero-Grande, Anthony Price, Emer Hughes, Robert Wright, Mary Rutherford, Joseph Hajnal
We describe a method for automated fetal brain reconstruction from stacks of 2D single-shot slices. Brain localization is performed by a deep distance regression network. Slice alignment is accomplished by a global search in the rigid transform space followed by registration using a fractional derivative metric. An outlier robust hybrid 1,2-norm
and linear high order regularization are used for reconstruction. Brain localization has achieved competitive results without requiring annotated segmentations. The method has produced acceptable reconstructions in 129 out of 133 3T fetal examinations tested so far.
|
|
4780.
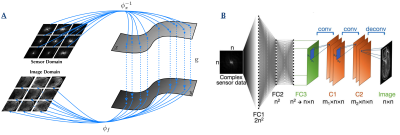 |
62 |
AUTOMAP Image Reconstruction of Ultra-Low Field Human Brain MR Data

Koonjoo Neha, Bo Zhu, Matthew Christensen, John Kirsch, Matthew Rosen
Due to very low Boltzmann polarization, MR images acquired at ultra-low field (ULF), MR images require significant signal averaging to overcome low signal-to-noise, which results in longer scan times. Here, we apply the deep neural network image reconstruction technique, AUTOMAP (Automated Transform by Manifold Approximation), to 50% under-sampled low SNR in vivo datasets acquired at 6.5 mT. The performance of AUTOMAP on this data was compared to the conventional 3D Inverse Fast Fourier Transform (IFFT). The results for AUTOMAP reconstruction show a significant improvement in image quality and SNR.
|
|
4781.
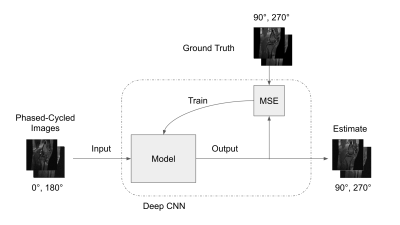 |
63 |
Synthetic Banding for bSSFP Data Augmentation

Michael Mendoza, Nicholas McKibben, Grayson Tarbox, Neal Bangerter
Balanced Steady State Free Precession (bSSFP) MRI is a highly-efficient MRI pulse sequence but suffers from banding artifacts caused by its high sensitivity to magnetic field inhomogeneity. Many algorithms exist that can effectively remove these banding artifacts, typically by requiring multiple phase-cycled acquisitions, which increase scan time. While some of the algorithms can suppress banding to some degree with two sets of phase-cycled acquisitions, much more accurate band suppression is typically achieved with at least four phase-cycled acquisitions. In this work, we present a deep learning method for synthesizing additional phase-cycled images from a set of at least two phase-cycled images that can then be used with existing band reduction techniques in order to reduce scan time.
|
|
4782.
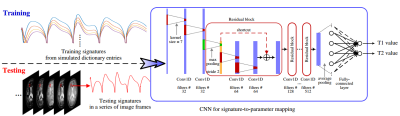 |
64 |
Magnetic Resonance Fingerprinting Using a Residual Convolutional Neural Network Presentation Not Submitted
Pingfan Song, Yonina Eldar, Gal Mazor, Migue Rodrigues
Dictionary matching based MR Fingerprinting (MRF) reconstruction approaches suffer from inherent quantization errors, as well as time-consuming parameter mapping operations that map temporal MRF signals to quantitative tissue parameters. To alleviate these issues, we design a residual convolutional neural network to capture the mappings from temporal MRF signals to tissue parameters. The designed network is trained on synthesized MRF data simulated with the Bloch equations and fast imaging with steady state precession (FISP) sequences. After training, our network is able to take a temporal MRF signal as input and directly output corresponding tissue parameters, playing the role of a dictionary and look-up table used in conventional approaches. However, the designed network outperforms conventional approaches in terms of both inference speed and reconstruction accuracy, which has been validated on both synthetic data and phantom data generated from healthy subjects.
|
|
4783.
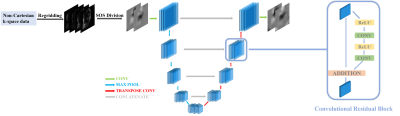 |
65 |
A Deep Learning Algorithm for Non-Cartesian Coil Sensitivity Map Estimation

Zihao Chen, Yuhua Chen, Debiao Li, Anthony Christodoulou
The use of parallel imaging (PI) to exploit the encoding power of multiple coil sensitivity patterns is essential for any modern method for accelerating MRI. In practice, the need to estimate sensitivity maps when using an image-space PI formulation delays the image reconstruction process, particularly for non-Cartesian acquisitions. This paper presents a deep learning method to estimate sensitivity maps from non-Cartesian dynamic imaging data. Results show that this algorithm provide a significant reduction in the time (from 42s to 2.5s for 12 coils) for generating high-quality coil sensitivity maps from non-Cartesian MR data compared to the conventional algorithms.
|
|
4784.
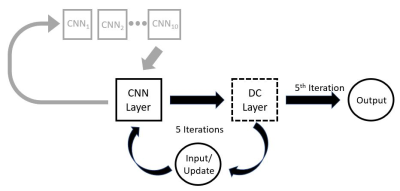 |
66 |
Real-time MR image reconstruction using Convolutional Neural Networks

Bryson Dietz, Gino Fallone, Keith Wachowicz
There has been an increasing interest for systems that combine a linear accelerator with a MRI. The goal of such systems is to allow for real-time adaptive radiotherapy; to have the ability to track a region of interest for the purpose of accurate radiation delivery. This requires the ability to image in real-time. We investigated the use of convolution neural networks (CNNs) for the purpose of real-time imaging. The reconstruction time of our preliminary data was 150 ms using a NVIDIA 1080Ti GTX GPU. Further optimization of the CNN parameters may decrease the reconstruction time below 100 ms.
|
|
4785.
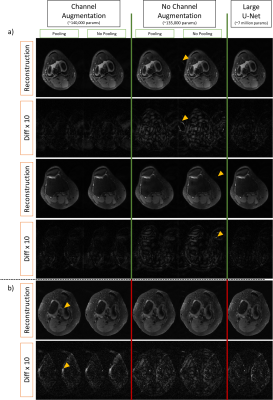 |
67 |
ShiftNets: Deep Convolutional Neural Networks for MR Image Reconstruction & the Importance of Receptive Field of View

Philip Lee, Makai Mann, Brian Hargreaves
Deep learning has been applied to the Parallel Imaging problem of resolving coherent aliasing in image domain. Convolutional neural networks have finite receptive FOV, where each output pixel is a function of a limited number of input pixels. For uniformly undersampled data, a simple hypothesis is that including the aliased peak in the receptive FOV would improve suppression of aliasing. We show that a simple channel augmentation scheme allows us to resolve aliasing using 50x fewer parameters than a large U-Net with millions of parameters and a global receptive FOV. This method was tested on retrospectively undersampled knee volumes.
|
|
4786.
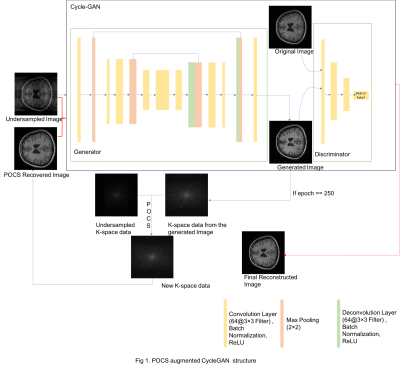 |
68 |
POCS Augmented CycleGAN for MR Image Reconstruction

Hanlu Yang, Yiran Li, Danfeng Xie, Wang Ze
Traditional MRI reconstruction depends heavily on solving nonlinear optimization problems, which could be highly time-consuming and sensitive to noise. We proposed a hybrid DL-based MR image reconstruction method by combining two state-of-art deep learning networks, U-Net and CycleGAN (Generative adversarial network with cycle loss) and a traditional method: projectiononto convex set (POCS). Our result shows a high reconstruction accuracy and this method can be further used to increase the sample size, which may find many applications in situations where the training samples are limited such as medical images.
|
|
4787.
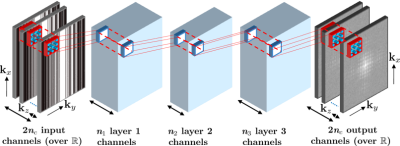 |
69 |
Accelerated Targeted Coronary MRI Using Sparsity-Regularized SPIRiT-RAKI

Seyed Amir Hossein Hosseini, Steen Moeller, Sebastian Weingärtner, Kâmil Ugurbil, Mehmet Akçakaya
Long scan duration remains a challenge in coronary MRI. A scan-specific machine learning technique, called Robust Artificial-neural-network for k-space Interpolation (RAKI) has recently shown promising results in accelerating MRI. However, RAKI was originally designed for uniform undersampling patterns. In this study, we propose a technique, called SPIRiT-RAKI that enables RAKI with arbitrary undersampling using scan-specific convolutional neural networks to enforce self-consistency among coils. Regularization terms are also incorporated in the new formulation. Our results indicate that SPIRiT-RAKI can successfully accelerate 3D targeted coronary MRI.
|
|
4788.
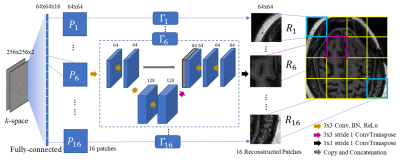 |
70 |
A divide-and-conquer strategy to overcome memory limitations of current GPUs for high resolution MRI reconstruction via a domain transform deep learning method

Chengzhu Zhang, Dalton Griner, Yinsheng Li, Yijing Wu, Guang-hong Chen
Direct learning of a domain transform to reconstruct images with flexible data acquisition schemes represents a step to achieve intelligence in image reconstruction. However, a technical challenge that is encountered with the domain transform type of learning strategy is that current network architectures and training strategies are GPU memory hungry. As a result, given the currently available GPUs with memory on the order of 24 GB, it is very difficult to achieve high resolution (beyond 128x128) MRI reconstruction. The main purpose of this paper is to present a divide-and-conquer strategy to reconstruct high resolution (better than 256x256) MRI images via domain transform learning while staying within the current GPU memory restrictions.
|
|
4789
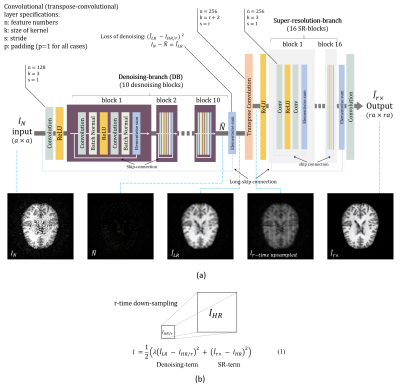 |
71 |
A New Deep Learning Structure for Improving Image Quality of a Low-field Portable MRI System Video Permission Withheld
WENCHUAN MU, Liang Zheng, Danial C. Alexander, Jia Gong, Wenwei Yu, Shao Ying Huang
A permanent magnet based low-field MRI system provides portability and affordability. However, the quality of the image is low due to a low signal-to-noise ratio (SNR). We propose a new deep learning structure which effectively integrates denoising-networks end-to-end to super-resolution-networks, to transfer the rich information available from one-o? experimental imaging from a mid-field MRI scanner (1.5T) to the lower-quality data from a portable system. The procedure uses matched pairs to learn mappings from low-quality to the corresponding high-quality images. Using the proposed method, the quality and resolution of an image from a low-field MRI system is significantly improved.
|
|
4790.
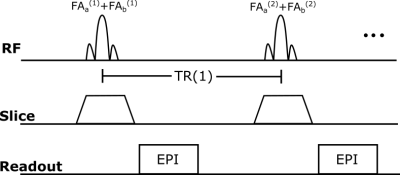 |
72 |
Simultaneous Multi-Slice Deep RecOnstruction NEtwork (SMS-DRONE)

Ouri Cohen
Recently, MR fingerprinting (MRF) has been proposed as a means of disentangling simultaneously excited slices by exciting each slice with a distinct acquisition schedule. A notable drawback of this approach, which is particularly acute for multi-parametric dictionaries, is the linear increase in reconstruction time with the number of slices and the potential reduction in accuracy. Here we describe an extension to our previously described MRF-DRONE method that can overcome these issues. Our method can enable larger acceleration factors and faster reconstruction of multi-parametric data.
|
|
4791.
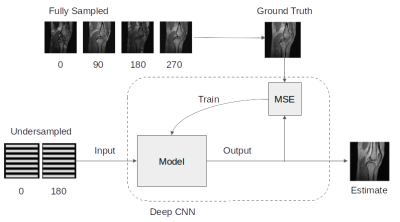 |
73 |
Deep Learning Super-FOV for Accelerated bSSFP Banding Reduction

Nicholas McKibben, Michael Mendoza, Edward DiBella, Neal Bangerter
We present a technique for bSSFP band removal using two undersampled phase-cycled bSSFP image acquisitions.
|
|
4792.
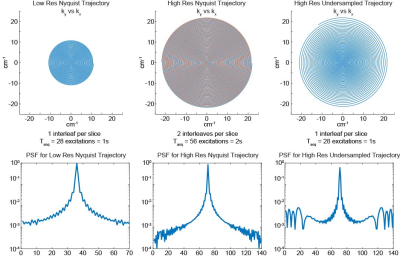 |
74 |
Convolutional Neural Network for Real-Time High Spatial Resolution Functional Magnetic Resonance Imaging

Cagan Alkan, Zhongnan Fang, Jin Hyung Lee
We propose a convolutional neural network (CNN) based real-time high spatial resolution fMRI method that can reconstruct a 3D volumetric image (140x140x28 matrix size) in 150 ms. We achieved 4x spatial resolution improvement using variable density spiral (VDS) trajectory design. The proposed method achieves similar reconstruction performance as our earlier compressed sensing reconstructions while achieving 17x faster reconstruction time. We demonstrate that this method accurately detects cortical layer specific activity.
|
|
4793.
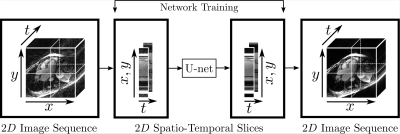 |
75 |
Spatio-Temporal Undersampling Artefact Reduction with Neural Networks for Fast 2D Cine MRI with Limited Data

Andreas Kofler, Marc Dewey, Tobias Schaeffter, Christian Wald, Christoph Kolbitsch
A well-known bottleneck of neural networks is the requirement of large datasets for successful training. We present a method for reduction of 2D radial cine MRI images which allows to properly train a neural network on limited datasets. The network is trained on spatio-temporal slices of healthy volunteers which are previously extracted from the image sequences and is tested on patients data with known heart dysfunction. The image sequences are reassembled from the processed spatio-temporal slices. Our method is shown to have several advantages compared to other Deep Learning-based methods and achieves comparable results to a state-of-the-art Compressed Sensing-based method.
|
|
Top |
Segmentation 2
Digital Poster
Acquisition, Reconstruction & Analysis
Thursday, 16 May 2019
Exhibition Hall |
13:45 - 14:45 |
|
|
Computer # |
|
4794.
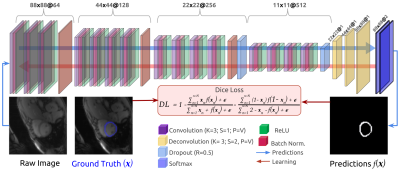 |
76 |
Automatic Segmentation Of The Myocardium in Cardiac Arterial Spin Labelling Images Using a Deep Learning Model Facilitates Myocardial Blood Flow Quantification

Pedro Gordaliza, Verónica Aramendía-Vidaurreta, Juan José Vaquero, Gorka Bastarrika, María Fernández-Seara, María Arrate Muñoz-Barrutia
Arterial Spin Labelling (ASL) allows to quantify Myocardial Blood Flow (MBF) by averaging over multiple ASL pairs. However, the procedure heavily depends on the manual segmentation of the myocardium. In this work, we introduce a Deep Learning model to segment this region and build a completely automatic pipeline for the MBF estimation. The accomplished evaluation results prove the success of the proposed method, which presents: 1) high overlap between the automatically extracted masks and those manually segmented by an expert (Dice Similarity Coefficient around 90%) and 2) good agreement of the MBF estimations with those obtained from the manual annotations.
|
|
4795.
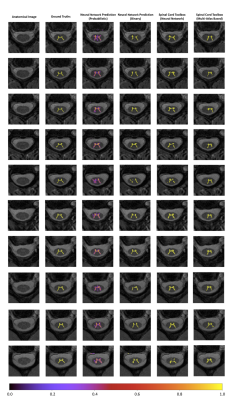 |
77 |
Spinal Cord Grey Matter Segmentation using a Light-Weight Off-The-Shelf Neural Network

Jackie Yik, Roger Tam, John Kramer, Cornelia Laule, Hanwen Liu
Spinal cord grey matter segmentation is typically done manually. Automatic segmentation methods exist but are generally highly customized. We used an off-the-shelf neural network (LinkNet) to segment the grey matter in the spinal cord to assess the performance of a method with a generic architecture, which may be easier to replicate on different machine learning frameworks. Manual segmentation was used as training data. The performance of our trained network was compared to an automatic segmentation method in the Spinal Cord Toolbox (SCT), and both networks produced similar results, demonstrating the viability of the off-the-shelf approach.
|
|
4796.
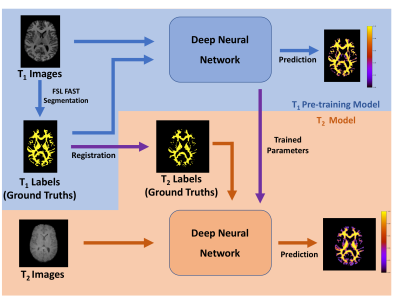 |
78 |
Feasibility of brain white matter segmentation on multi-echo T2-weighted images without registration: a Neural Network approach.

Jackie Yik, Roger Tam, Cristina Rubino, Lara Boyd, David Li, Cornelia Laule, Hanwen Liu
Most current methods of human brain white matter segmentation require registration to T1 image space. Artificial intelligence can reduce potential errors in, and speed up, this process by segmenting white matter from T2-weighted images directly. A neural network was pre-trained using T1-weighted images and FSL’s FAST followed by T2-weighted images using transfer learning. The network could then segment new T2-weighted images directly. T1- and T2-weighted image segmentations using the neural network were comparable to FSL’s FAST. Our work shows the feasibility of multi-echo T2-weighted images for brain white matter segmentation without initial segmentation and registration of T1-weighted images.
|
|
4797.
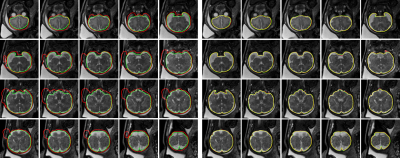 |
79 |
Automated Fetal Brain Segmentation Using Deep Convolutional Neural Network

Bin Chen, Liming Wu, Bing Zhang, Simin Liu, Hua Guo
Recent advances show promising fetal brain reconstruction results through image motion correction and super resolution from a stack of unregistered images consisting of in-plane motion free snapshot slices acquired by fast imaging methods. Most motion correction and super resolution techniques for 3D volume reconstruction require accurate fetal brain segmentation as the first step of image analysis. In this study, a customized U-Net based deep learning method was implemented for automatic fetal brain segmentation. The high accuracy of deep learning based semantic segmentation improves the performance in volume registration as well as quantitative studies of brain development and group analysis.
|
|
4798.
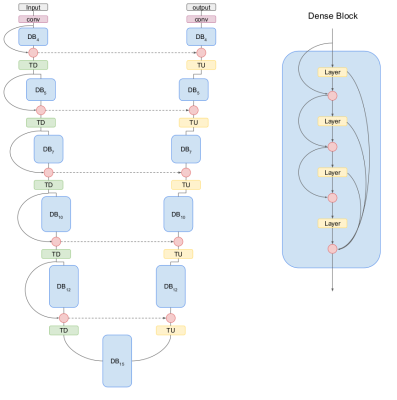 |
80 |
Random forests and DenseNet: a comparative study of brain gliomas segmentation

Marco Castellaro, Gianmario Battista, Alessandra Bertoldo
Machine Learning techniques can provide useful automatic tools. Segmentation of brain tumors is a time consuming task that could potentially beneficiate from its automation. This work investigate and compare the performances of two frameworks: Random forest and DenseNet. The former is a well known framework and the latter is a novel technique based on deep learning.
|
|
4799.
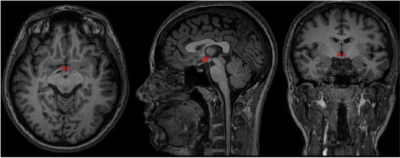 |
81 |
Hypothalamus semi-automatic segmentation from MR images using Convolutional Neural Networks

Lívia Rodrigues, Thiago Rezende, Ariane Zanesco, Ana Hernandez, Marcondes França, Letícia Rittner
Hypothalamus is a small structure of the brain with important role in sleep, body temperature regulation and emotion. Some diseases as schizophrenia can be attributed to volumetric change on hypothalamus, usually measured through Magnetic Resonance Imaging (MRI). However, hypothalamic morphological landmarks are not always clear and manual segmentation can become variable, leading to inconsistent data on literature. On this project, hypothalamus was automatically segmented using convolutional neural networks (CNNs) . Three independent CNNs were trained, one for each view of volumetric MRI, obtaining final dice of 0.787 for axial view, 0.781 for sagittal and 0.747 for coronal view.
|
|
4800.
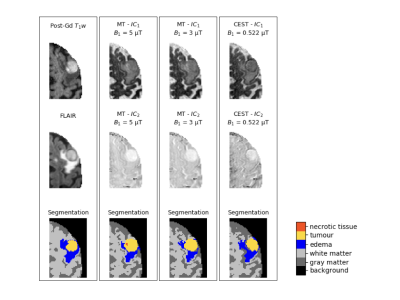 |
82 |
Automatic Segmentation of Brain Metastases Using Saturation Transfer Magnetic Resonance Imaging

Elham Karami, Wilfred Lam, Wendy Oakden, Margaret Koletar, Leedan Murray, Stanley Liu, Ali Sadeghi Naini, Hany Soliman, Arjun Sahgal, Greg Stanisz
Chemical exchange saturation transfer (CEST) and magnetization transfer (MT) are MR contrast mechanisms that have been shown to correlate with cancer metabolism. Given that CEST does not require exogenous contrast agents, the goal of this study was to investigate the potential of CEST for segmenting the images of brain metastasis. As such, the tumour, and edema were segmented on CEST images and compared with segmentation performed on FLAIR and post-gadolinium T1-weighted images. The results indicate that the Dice similarity coefficient ranges between 0.78 to 0.84, suggesting that CEST can potentially be used for segmentation of brain metastases.
|
|
4801.
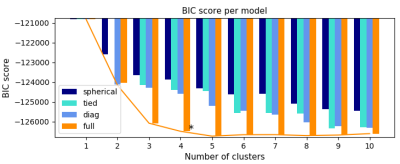 |
83 |
Segmentation of Intra-Tumour Distinct Metabolic Regions Using Chemical Exchange Saturation Transfer Imaging

Elham Karami, Wilfred Lam, Wendy Oakden, Margaret Koletar, Leedan Murray, Stanley Liu, Ali Sadeghi Naini, Greg Stanisz
Chemical exchange saturation transfer (CEST) is a promising MR contrast mechanism that has been shown to correlate with cancer metabolism and reveal regions of active tumour metabolism. However, the acquisition of CEST-weighted images is time consuming. In this study, computational methods including unsupervised learning were adapted to find the minimum number of CEST images required to segment the intra-tumour distinct metabolic regions accurately, and to find the number of different cell groups existing within a tumour. The results indicate that four intra-tumour regions can be segmented accurately using only CEST images acquired at 3.5 ppm and 2.0 ppm.
|
|
4802.
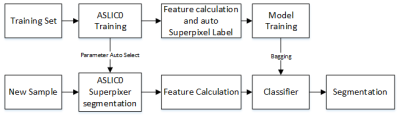 |
84 |
Automatic Glioma Segmentation Algorithm Based on Superpixel Features

Yaping Wu, Yusong Lin, Guohua Zhao, Longfei Li, Meiyun Wang
This study proposes an algorithm to locate and segment Glioma tumor automatically. The algorithm contains three main steps. Firstly, a self-adaptation simple linear iterative clustering (ASLIC0) algorithm was executed to segment T2 weighted MRI images to superpixels images. Then, 52 features including fractal features, curvature feature and higher order derivative map Haralick texture features was calculated on each superpixel. Finally, a Support Vector Machine was trained as a classifier to select superpixels belong to tumor lesion or not. The Dice overlap measure for the segmented Glioma is 0.87 on the data set from the Henan Provincial People’s Hospital.
|
|
4803.
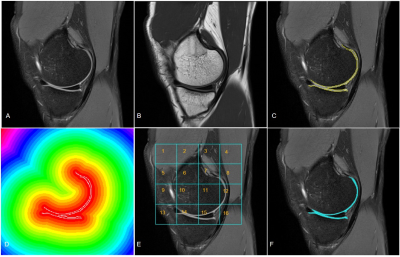 |
85 |
Machine Learning-based Human Knee Cartilage Segmentation on MRI

Siddhi Munde, Melissa Manzer, Wellsandt Elizabeth, Jessica Emory, Balasrinivasa Sajja
Accurate knee cartilage segmentation on MRI is essential to obtain quantitative measures from cartilage that help in the assessment of knee pathology and therapeutic response in patients with diseases such as Osteoarthritis. Segmentation of cartilage on routine clinical MRI is challenging due to image intensity variation across the structure and low image contrast. In this study, we obtained an accurate cartilage segmentation on PD and T1 weighted images using Support Vector Machine (SVM) classifier with a spatial indexing feature which accounts for regional signal variations.
|
|
4804.
|
86 |
Automated Segmentation of Thalamic Nuclei using Convolutional Neural Networks

Mohammad Majdi, Mahesh Keerthivasan, Natalie Zahr, Jeffrey Rodriguez, Manoj Saranathan
parcellation of thalamic nuclei is critical step in targeting for deep brain surgery, volumetry for longitudinal tracking of diseases such as Alzheimer’s and multiple sclerosis,. However, thalamic nuclei are mostly indistinguishable in conventional T1 or T2 weighted MRI. In this study, we propose a deep neural network based method to achieve a fast and accurate segmentation of thalamic nuclei, taking advantage of high contrast characteristics of a white matter nulled MPRAGE sequence at both 3T and 7T.
|
|
4805.
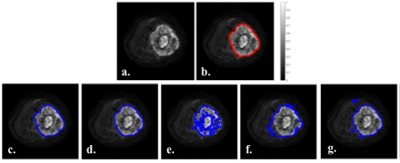 |
87 |
Machine Learning Techniques for Bone Tumor Segmentation using Diffusion MRI

Sneha Patil, Esha Baidya Kayal, Sameer Bakhshi, Raju Sharma, Devasenathipathy Kandasamy, Amit Mehndiratta
Automatic and accurate segmentation of osteosarcoma region in MRI images can assist doctor to prepare a feasible treatment plan, hence resulting in improved cure rate. The purpose of this study was to evaluate and compare the performance of automated and semi-automated algorithms that might be effective in segmenting bone tumor in MRI data, with reasonable accuracy, speed and minimal manual input. The results are very conclusive for efficient performance.
|
|
4806.
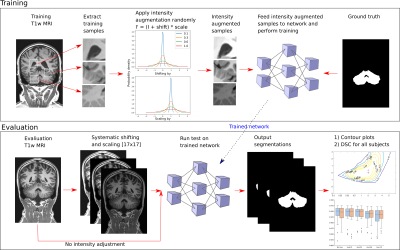 |
88 |
Can Intensity Augmentation Improve Generalizability of CNN-based Image Segmentation?

Nina Jacobsen, Andreas Deistung, Dagmar Timmann, Sophia Luise Goericke, Jürgen Reichenbach, Daniel Güllmar
As a strategy to achieve higher generalizability of a convolutional neural network (CNN), data augmentation may be used to introduce a higher degree of variability within the training sample. In this study, five different intensity augmentation strategies were compared and analyzed by means of the CNN segmentation performance. The results indicate how intensity augmentation improves the robustness, and thereby the generalizability, of the CNN but in some cases also compromise the segmentation performance in terms of accuracy.
|
|
4807.
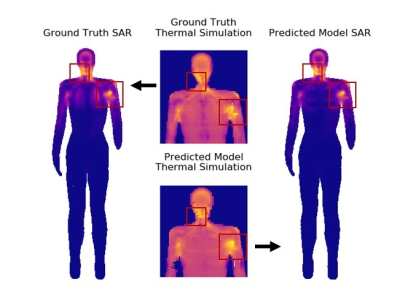 |
89 |
U-Net Segmentation for Human Body Models for SAR Simulations

Isabelle Heukensfeldt Jansen, Matthew Tarasek, Johan Reimann, Desmond Teck Beng Yeo
RF power absorption during MRI, expressed in terms of specific absorption rate (SAR), is an important safety issue, especially in multi-channel transmit MRI. To reduce uncertainties of local SAR estimates due to subject antatomical variations, patient-specific human body models can be applied in EM simulations of the RF transmit coil. In this work, we trained a U-net neural network on simulated CT scans to quickly create HBMs with four primary tissue classes (bone, lungs, fat, and water-based). Local SAR results using HBMs created with the U-net showed good agreement with those from ground truth models.
|
|
4808.
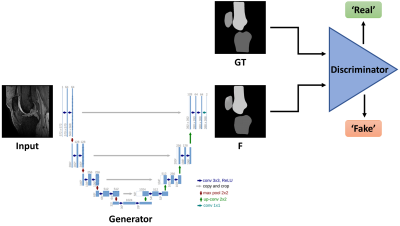 |
90 |
Automated Knee MRI Semantic Segmentation with Generative Adversarial Networks

Dimitri Kessler, James MacKay, Martin Graves, Fiona Gilbert, Joshua Kaggie
We describe a fully automated deep learning approach for generating semantic segmentation maps of the knee joint. A conditional Generative Adversarial Network (cGAN) was trained on 3D fat-saturated spoiled gradient recalled-echo MRIs of the knee from nine individuals (nimages=778) to generate segmentation maps containing the patella, femur and tibia. The trained network was tested with a separate dataset of one individual (nimages=80). The mean Sørensen–Dice Similarity Coefficient (DSC) was 0.959 and Jaccard Index was 0.985 for all three compartments. These results suggest that cGANs can perform accurate bony segmentation of the knee.
|
|
4809.
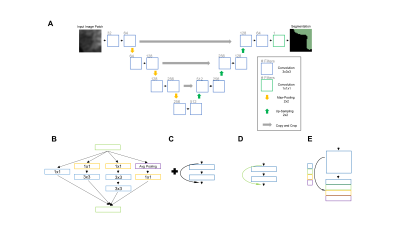 |
91 |
Volumetric Segmentation of Acute Brain Infarcts on Diffusion-Weighted Imaging using Deep Learning

Ken Chang, James Brown, Andrew Beers, Katharina Hoebel, Jay Patel, Otto Rapalino, Bruce Rosen, Hakan Ay, Jayashree Kalpathy-Cramer
Rapid and accurate evaluation of stroke is imperative as currently available treatments are constrained by a narrow time window. Diffusion Weighted Magnetic Resonance (DWI) is a key imaging modality in stroke evaluation as it allows for assessment of the extent of acute ischemic brain injury. Nonetheless, manual delineation of stroke regions is expensive, time-consuming, and subject to inter-rater variability. In this study, we sought to develop a deep learning approach for ischemic stroke volumetric segmentation in a large clinical dataset of 1,205 patients from the NIH-funded Heart-Brain Interactions in Human Acute Ischemic Stroke Study utilizing only DWI imaging.
|
|
4810.
 |
92 |
Real-time Ultrafast Fetal Brain Localization using Convolutional Neural Networks

Dhineshvikram Krishnamurthy, Wonsang You, Kushal Kapse, Catherine Limperopoulos
The advent of fetal magnetic resonance imaging has provided innovative approaches to study in-vivo brain development in the womb. One of the major challenges in the quantification of fetal brain growth and development is regional and tissue-specific segmentation. An automated brain localization algorithm can reduce the time and facilitate accurate segmentation. In this study, we propose an ultra-fast and robust method for fetal brain localization from SSFSE anatomical images using a minimally modified object localization algorithm called You Only Look Once (YOLO). YOLO provides not only the enhanced accuracy of brain localization by differentiating brain from maternal tissues but also fast computation time for brain detection compared to the other algorithms.
|
|
4811.
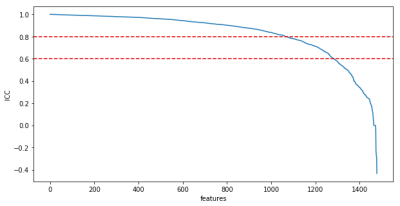 |
93 |
Target-class-agnostic feature rejection for radiomics analyses based on variations of tumor segmentation mask

Balthasar Schachtner, Michael Ingrisch, Gresser Eva, Moritz Schneider, Andrea Schreier, Olga Solyanik, Guiseppe Magistro, Dominik Nörenberg
Feature selection is a key aspect to radiomics analyses. An approach to remove features which are not stable with respect to small variations of the segmented mask is presented. The rejection works target-class agnostic and can be used in combination with target-class-based selections. An increase of about 5 percentage points can be seen when using the proposed approach in a simple machine learning setup on prostate MRI of prostate cancer patients.
|
|
4812.
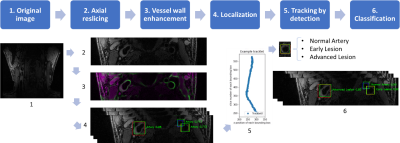 |
94 |
Carotid Artery Localization and Lesion Classification on 3D-MERGE MRI using Neural Network and Object Tracking methods

Li Chen, Huilin Zhao, Niranjan Balu, Xihai Zhao, Rui Li, Jianrong Xu, Thomas Hatsukami, Jenq-Neng Hwang, Chun Yuan
Carotid vessel wall imaging (VWI) with MRI provides additional prognostic value for cerebro/cardiovascular ischemic events, beyond current clinical diagnostic imaging methods. While fast 3D carotid MRI is possible, manual review of the large 3D dataset is time consuming. Automatic identification of artery locations and lesion categories are therefore required for VWI screening protocols. With neural network and object tracking methods, we developed a fully automated analysis tool to find common/internal/external carotid arteries and flag possible high-risk lesion locations. The tool achieved 0.782 Intersection over Union (IoU) for artery localization, and 0.895 sensitivity for high-risk lesion classification.
|
|
4813.
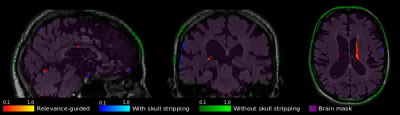 |
95 |
Relevance-guided Feature Extraction for Alzheimer's Disease Classification

Christian Tinauer, Stefan Heber, Lukas Pirpamer, Anna Damulina, Maximilian Sackl, Edith Hofer, Marisa Koini, Reinhold Schmidt, Stefan Ropele, Christian Langkammer
Using FLAIR images we separated Alzheimer's patients (n=106) from controls (n=173) by using a deep convolutional neural network and found that the classifier might learn irrelevant features e.g. outside the brain. Preprocessing of MRI plays a crucial but often neglected role in classification and therefore we have developed a method enforcing the relevant features to be within brain tissue and, thus, eliminated the influence of precomputed brain masks. While our relevance-guided training method reached the same classification accuracy, incorporating relevance improved feature identification in an anatomically more reasonable manner.
|
|
4814.
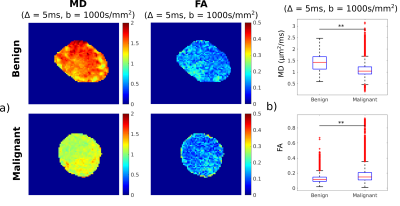 |
96 |
Classification of benign and malignant lymph nodes based on ex-vivo diffusion MRI data

Andrada Ianus, Inês Santiago, Daniele Ravi, Celso Matos, Daniel Alexander, Noam Shemesh
Developing non-invasive imaging technique for detection and characterisation of lymph nodes is an important topic in cancer research. Diffusion MRI (dMRI) appears to be a promising modality for this task. This work investigates the ability of dMRI to differentiate benign and malignant lymph nodes based on a rich, ex-vivo dataset, and aims to find which measurements provide the most differentiation power.
|
|
4815.
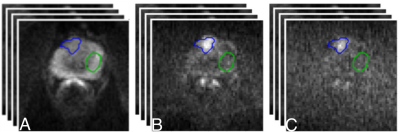 |
97 |
Automatic classification of benign and malignant prostate lesions: A comparison using VERDICT DW-MRI and ADC maps

Eleni Chiou, Edward Johnston, Francesco Giganti, Elisenda Bonet-Carne, Shonit Punwani, Iasonas Kokkinos, Eleftheria Panagiotaki
Currently, many studies exploit deep learning and mp-MRI data to enhance the diagnostic accuracy of prostate cancer characterisation. In this study, we focus on VERDICT DW-MRI data and compare its diagnostic performance to those of the ADC map and the raw DW-MRI from the mp-MRI. Specifically, we compare the performance obtained by a fully convolutional neural network (CNN) when training and test is performed on the raw VERDICT DW-MRI, the ADC maps and the DW-MRI data from the mp-MRI acquisition. The results indicate that the CNN performs better when it is trained and tested on VERDICT DW-MRI.
|
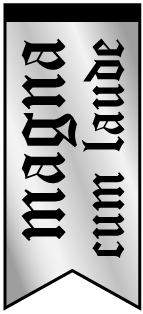 |
4816.
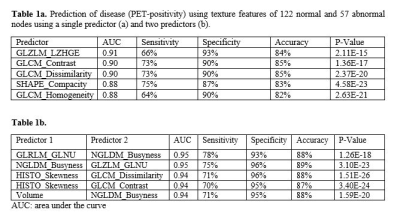 |
98 |
MRI texture analysis for detection of axillary lymph node metastasis in breast cancer patients

Renee Cattell, Vincent Zhang, Pauline Huang, Meghan Italo, James Kang, Jason Ha, Haifang Li, Jules Cohen, Lea Baer, Dinko Franceschi, Cliff Bernstein, Sean Clouston, Timothy Duong
We tested the hypothesis whether texture analysis of axillary lymph node (aLN) MRI can reliably detect cancer metastasis in the aLN. Comparison was made with ground truth based on pathology and clinical reports. The top single-feature predictor yielded an area under the curve (AUC) of 0.91 and the top two-feature combination yielded an AUC of 0.95. These findings showed that texture analysis of aLN MRI can accurately predict disease status in the nodes associated with breast cancer.
|
|
4817.
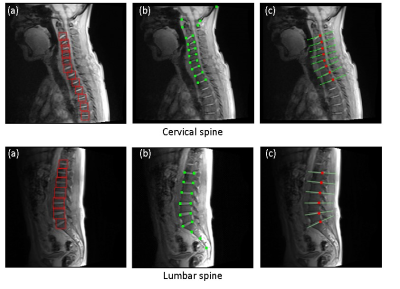 |
99 |
Robust detection of anatomical landmark by combining adaptive boosting and active shape model for automated scan plane planning of spine MRI

Suguru Yokosawa, Yoshimi Noguchi, Kenta Sakuragi, Kuniaki Harada, Masatomo Yokose, Hisako Nagao, Hisaaki Ochi
Automated scan plane planning is expected to improve MRI scanner usability and provide consistent scan plane prescriptions which are useful for follow-up examinations. However, a landmark degenerated by formation of a lesion such as an intervertebral disc in the case of hernia patient is difficult to detect because shape and properties of tissue greatly deviate from normal cases. In this study, we have proposed combining adaptive boosting and active shape model to detect intervertebral discs robustly for automated scan plane planning of spine MRI.
|
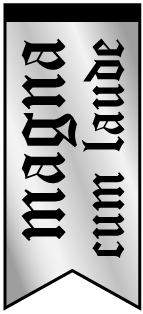 |
4818.
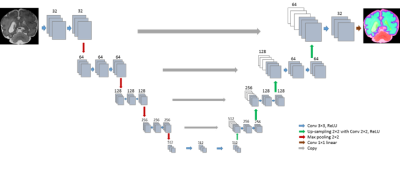 |
100 |
Brain tissue segmentation in fetal MRI using convolutional neural networks with simulated intensity inhomogeneities

Nadieh Khalili, Nikolas Lessmann, Elise Turk, Max Viergever, Manon Benders, Ivana Isgum
Automatic brain tissue segmentation in fetal MRI is a challenging task due to artifacts such as intensity inhomogeneity, caused in particular by spontaneous fetal movements during the scan. Unlike methods that estimate the bias field to remove intensity inhomogeneity as a preprocessing step in segmentation process, we aim at improving the robustness of the segmentation method by introducing an intensity inhomogeneity augmentation (IIA). The IIA simulates various patterns of intensity inhomogeneity during the training of the segmentation network. The segmentation results demonstrate an improvement in segmentation performance when the training data is augmented with IIA.
|
|
Top |
Software & Tools
Digital Poster
Acquisition, Reconstruction & Analysis
Thursday, 16 May 2019
Exhibition Hall |
13:45 - 14:45 |
|
|
Computer # |
|
4819.
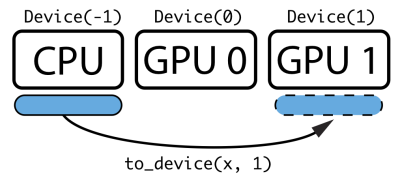 |
101 |
SigPy: A Python Package for High Performance Iterative Reconstruction

Frank Ong, Michael Lustig
We present SigPy, a Python package designed for high performance iterative reconstruction. Its main features include: - A unified CPU and GPU Python interface to signal processing functions, including convolution, FFT, NUFFT, wavelet transform, and thresholding functions.
- Convenient classes (Linop, Prox, Alg, App) to build more complicated iterative reconstruction algorithms.
- Commonly used MRI reconstruction methods as Apps, including SENSE, L1-wavelet regularized reconstruction, total-variation regularized reconstruction, and JSENSE.
- MRI-specific functions, including poisson-disc sampling, ESPIRiT calibration, and non-Cartesian preconditioners.
- Simple installation via pip and conda.
|
|
4820.
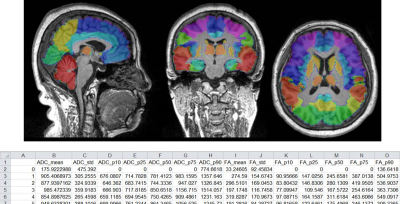 |
102 |
BrainQuan: An integrated tool for automated and region-specific analysis of multi-parametric brain MRI data Presentation Not Submitted
Xiang Feng, Meng Xiao Liu, Guang Yang, Xu Yan
This abstract presents an integrated tool, namely BrainQuan, developed in Python to automatically segment the brain MRI into sub-regions, align the multi-parametric MRI data into the same space, and then extract the region-specific information from quantitative MRI data, such as quantitative susceptibility maps and diffusion parameters in these brain sub-regions. This tool provides an easy and comprehensive solution for several pilot studies spanning a range of applications: infant brain, brain morphology analysis and neuro-degenerative diseases. BrainQuan might be helpful to establish potential biomarkers from many different quantitative brain MRI data.
|
|
4821.
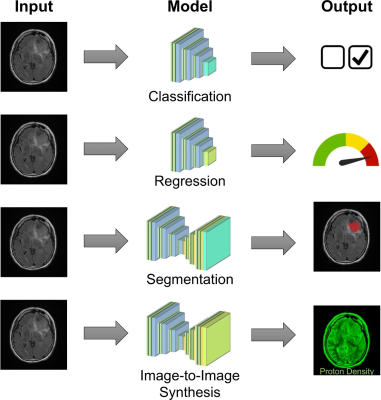 |
103 |
DeepRad: An Accessible, Open-source Tool for Deep Learning in Medical Imaging

Jinnian Zhang, Samuel Hurley, Varun Jog, Alan McMillan
Deep learning has shown incredible potential as a powerful tool in medical imaging, however accessibility to deep learning is still limited for users who lack expertise in computer programming, machine learning, or data science. Existing tools to perform deep learning have not been designed to be user friendly. We have developed a powerful, flexible, and easy-to-use software specifically tailored to medical imaging for biomedical researchers and physicians with limited programming skills to utilize deep learning for many common tasks.
|
|
4822.
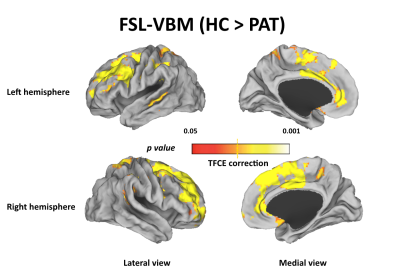 |
104 |
Voxel-based morphometry results in first-episode schizophrenia: a comparison of publicly available software packages Presentation Not Submitted
Yuanqiang Zhu, Xingrui Wang, Xiaocheng Wei, Yibin Xi
Investigations of brain structure in schizophrenia using magnetic resonance imaging (MRI) have identified variations in regional grey matter (GM) volume throughout the brain but the results are mixed. This study aims to investigate whether the inconsistent voxel-based morphometry (VBM) findings in schizophrenia are due to the use of different software packages. our data indicate that the GM volume differences between FESZ and HCs depend on which software are used(FSL, SPM), algorithms of GM tissue segmentation and image registration might contribute to these disparate results.
|
|
4823.
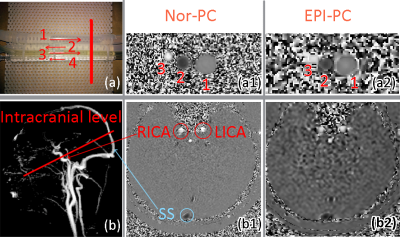 |
105 |
Post Processing Software for Echo Planar Imaging Phase Contrast Sequence

Pan LIU, Armelle LOKOSSOU, Sidy FALL, Malek MAKKI, Olivier BALEDENT
The new sequence Echo Planar Imaging Phase Contrast (EPI-PC) allows real-time imaging of blood flow and can be used to study the effect of breathing unlike to the normal Phase Contrast Magnetic Resonance Imaging sequence (Nor-PC). However, there is no software for the processing of EPI-PC data. We developed new software to visualize, segment and analyze EPI-PC data. We implemented in the software functions as filtering, denoising, segmentation, reconstruction, and extraction that can be applied on EPI-PC signal. This software was easy to use and gave promising results for the quantification of blood flow and the study of breathing effect.
|
|
4824
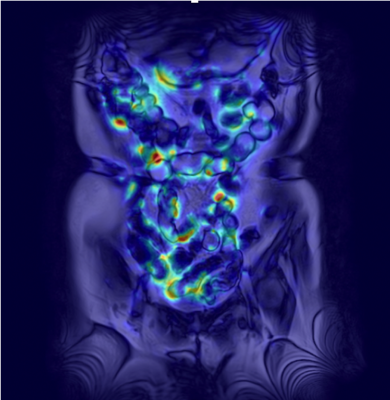 |
106 |
Development of a computer software to quantify bowel motility shown on cine MR imaging by using classical Horn-Schunck approach Video Permission Withheld
Yoshio Kitazume, Kento Takenaka, Kazuo Ohtsuka, Ukihide Tateishi
We developed a computer software which quantifies the small bowel motility shown on cine MR imaging using optical flow algorithm with Horn-Schunck approach, by adding a preprocessing step for analyzing cine MR images. A high Pearson’s correlation coefficient was obtained between direct measurement on cine MR and motility map value (r= 0.83 [95% confidence interval: 0.83 – 0.95, P<0.0001]).
|
|
4825.
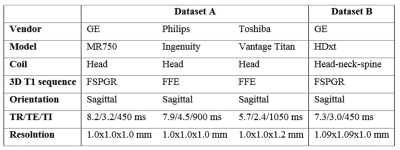 |
107 |
High reproducibility and robustness to lesions, but large software and scanner effects for mean upper cervical cord area (MUCCA) measurement in MS

Merlin Weeda, Sander Middelkoop, Martijn Steenwijk, Marita Daams, Houshang Amiri, Iman Brouwer, Joep Killestein, Bernard Uitdehaag, Iris Dekker, Carsten Lukas, Barbara Bellenberg, Frederik Barkhof, Petra Pouwels, Hugo Vrenken
Atrophy of the spinal cord is known to occur in multiple sclerosis (MS). To measure such atrophy, the mean upper cervical cord area (MUCCA) can be assessed. We tested five different (semi-)automated spinal cord segmentation methods (SCT-PropSeg, SCT-DeepSeg, ITK-SNAP, NeuroQLab, Xinapse JIM) in terms of their reproducibility, robustness, and the influence of lesions on the segmentations. MUCCA from all scanners was highly reproducible within-scanner, but not between-scanner or between-methods. The presence of lesions in the upper cervical cord did not affect the accuracy of MUCCA measurements in any of the methods tested.
|
|
4826.
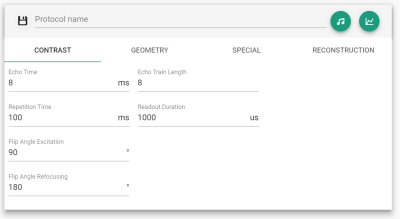 |
108 |
Configuring, Viewing, Exploring and Exporting Reproducible, Vendor-Independent MRI Pulse Sequences

Cristoffer Cordes, Simon Konstandin, Daniel Mensing, Saulius Archipovas, Robin Wilke, Matthias Günther
This work introduces a web technology-based tool that can load device vendor-independent sequence descriptions of a previously described format to then provide interactive tools for configuring protocol parameters, viewing pulse sequence diagrams and details, and exporting raw pulse shapes.
|
|
4827.
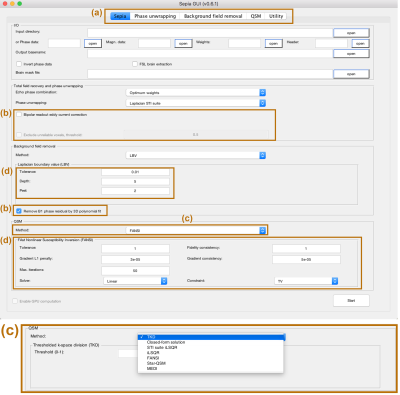 |
109 |
SEPIA – SuscEptibility mapping PIpeline tool for phAse images

Kwok-Shing Chan, José Marques
With the ever-increasing number of quantitative susceptibility mapping (QSM) methods and research applications, it becomes difficult for application-driven researchers to choose a (best) QSM method or pipeline for their study. Here, we present a susceptibility mapping pipeline tool for phase images (SEPIA) which includes a user interface for non-experienced users and the possibility of generating code that can be used for scripting large studies. SEPIA incorporates various QSM toolboxes available in Matlab as well as a wide range of methods to process MR phase data, including signal phase unwrapping, background field removal and field-to-source inversion.
|
|
4828.
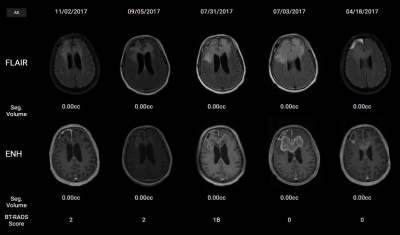 |
110 |
A Cloud Platform for Longitudinal Follow-up for Patients with Glioblastoma

Saumya Gurbani, Karthik Ramesh, Hyunsuk Shim, Brent Weinberg
Patients diagnosed with glioblastoma are typically treated with a combination of stereotactic surgical resection followed by chemoradiation. Follow-up of these patients post-treatment involves regular imaging to identify disease recurrence and plan adjuvant therapies. In this work, we present a cloud app that will facilitate radiologists and the treating physician team in quantitatively tracking post-treatment disease course using semi-automated segmentation of tumor and a structured scoring system to standardize monitoring of disease progression.
|
|
4829.
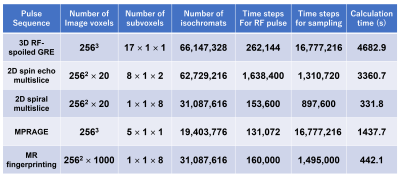 |
111 |
Bloch image simulations of brain pulse sequences using a GPU-installed gaming PC

Katsumi Kose, Ryoichi Kose
Bloch image simulations for typical brain pulse sequences were performed using a GPU-installed gaming laptop PC and a numerical brain phantom. Artifact-free brain MR images were obtained by the Bloch image simulation using optimized numbers of subvoxels. Because the simulation times were the same order as the imaging time for the experiments, we concluded that the Bloch image simulator installed in an inexpensive gaming PC can be a powerful research tool for many MRI engineers and scientists.
|
|
4830.
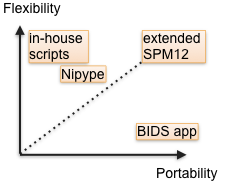 |
112 |
New neuroimaging technologies in SPM: BIDS, docker, boutique, and quality control

Tanguy Duval, Vincent Lubrano
Today, sharing pipelines across the community is still a complex issue. New technologies and standards, however, change our methods for better collaboration. Here we propose to integrate them seamlessly into the stable and popular SPM pipeline manager. The graphical user interface gives enough flexibility for understanding, modifying, creating and sharing the standard pipelines that are not available today. These pipelines can finally be run with a simple BIDS-app command on any computer.
|
|
4831.
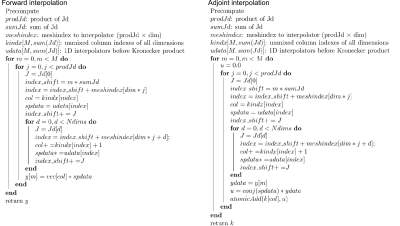 |
113 |
Application of memory reduced NUFFT to multi-dimensional non-Cartesian MRI

Jyh-Miin Lin, Grzegorz Kowalik , Javier Montalt Tordera, Benoit Sarthou, Philippe Ciuciu, Jennifer Steeden, Vivek Muthurangu
A precomputed interpolation matrix on a GPU has been commonly used for fast iterative NUFFT MRI reconstructions, but the size of a 3D interpolation matrix may exceed the memory available on a single GPU. We propose a memory reduced interpolation method that would reduce the size of a multidimensional non-Cartesian interpolation matrix on a GPU. The memory reduced NUFFT reduces the matrix size by more than 90%, while allowing a performance of 38% - 106% of the precomputed version. We also apply the memory reduced NUFFT to a large scale 3D generalized basis pursuit denoising algorithm (GBPDNA) reconstruction.
|
|
4832.
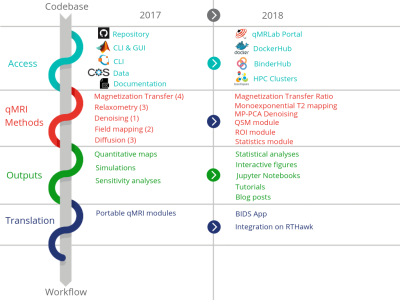 |
114 |
The qMRLab workflow: From acquisition to publication

Agah Karakuzu, Mathieu Boudreau, Tanguy Duval, Ilana Leppert , Tommy Boshkovski, Julien Cohen-Adad, Nikola Stikov
qMRLab is open-source software that provides a wide selection of quantitative MRI (qMRI) methods for data fitting, simulation and protocol optimization. Currently, most qMRI methods are developed in-house and are difficult to port across sites. Our vision for qMRLab is to build standardized workflows for these methods, beginning at the scanner console and extending all the way to journal publication. We developed a web portal (https://qmrlab.org) that includes interactive tutorials and Jupyter Notebooks running on BinderHub, tailored for qMRI methods. The last piece of this workflow puzzle is the integration of qMRLab on MR systems, by deploying it as a plugin on a custom MRI application development platform (e.g. RTHawk).
|
|
4833.
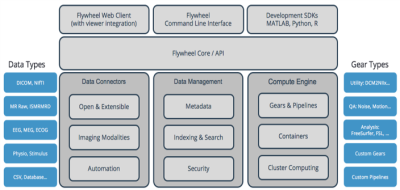 |
115 |
MR Research in the cloud - preliminary results at Columbia University

Can Akgun, John Vaughan
MR researchers are challenged with managing large data sets, scaling complex computational analyses, and supporting cross-functional collaboration. To tackle these challenges, Columbia University has fully integrated their data management and processing in the cloud to take advantage of high-performance yet low-cost storage, scalable on-demand compute resources, and secure regulatory-compliant infrastructure for sharing of data and algorithms. The result is a platform that has enabled more efficient workflows, greater productivity, and multi-site collaboration.
|
|
4834.
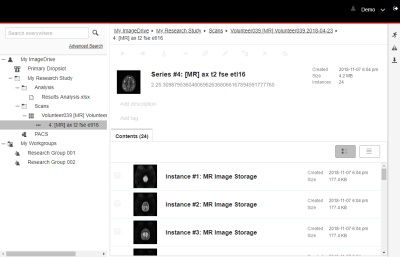 |
116 |
A Web-Based Data Management System as a Collaborative Imaging Research Platform

Norman Young, Jonathan Resnick, Steven Wranovsky, Thanh Huynh, Jasper Yeh, Stephen Leung, Stewart Bright, Jay Liu
A web-based data management system specifically aimed at imaging researchers is presented as a possible solution to the challenges of systematic data management and processing in a research environment. The system was employed during the development of a head-only MRI for post-processing quality assurance. Extending the use of the system to facilitate training of machine learning algorithms is proposed.
|
|
4835.
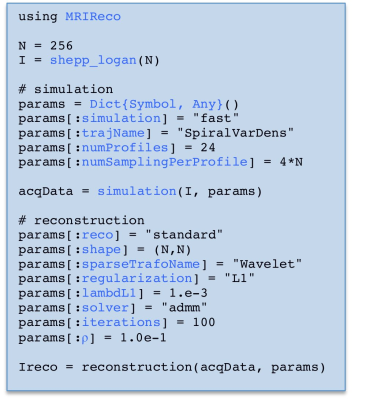 |
117 |
MRIReco.jl: An Extensible Open-Source Image Reconstruction Framework written in Julia

Tobias Knopp, Mirco Grosser
Image reconstruction plays a major role in the recent years of development in magnetic resonance imaging (MRI) and has been one of the main drivers for reductions in scan time. Within this work we introduce a new software package MRIReco.jl that is very flexible to use and allows for rapid development of new reconstruction algorithms. The package uses the programming language Julia, which is very suitable for implementing reconstruction algorithms on a high abstraction level while still allowing for the generation of runtime-optimized machine code.
|
|
4836.
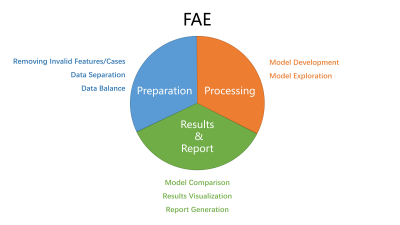 |
118 |
FeAture Explorer (FAE): a Tool of Radiomics Feature Analysis and Exploration

Yang Song, Jing Zhang, Yu-Dong Zhang, Xu Yan, Yida Wang, Minxiong Zhou, Bingwen Hu, Guang Yang
Radiomics studies often requires researchers spend large amount of time trying out various combinations of different data preprocessing strategies, feature selection algorithms, classifiers, and associated hyper-parameters to find the best model. We developed a tool with graphics user interface named FeAture Explorer (FAE) to automate this tedious process. With FAE, to find the best model, researchers only need to specify the choices for each step in radiomics pipeline and let FAE do the rest. Results, such as clinical statistics of each model, can be reviewed and visualized. We used the PROSTATEx dataset to illustrate the function of FAE.
|
|
4837.
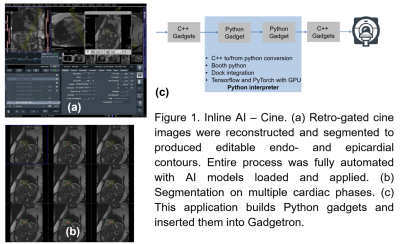 |
119 |
Gadgetron Inline AI: Effective Model inference on MR scanner

Hui Xue, Rhodri Davies, David Hansen, Ethan Tseng, Marianna Fontana, James Moon, Peter Kellman
We extended Gadgetron, a widely used open-source framework, to support AI inference on clinical MR scanners. Specially designed software modules (InlineAI) was added to Gadgetron, allowing to load and apply AI neural network models on incoming MR data for compelte "in-line" fashion. That is, without any user interaction, results will be sent back to scanner and available immediately after data acquisition. Two AI based applications were developed as demenstration: Inline AI cine segmenation and perfusion flow mapping and analysis.
|
|
4838.
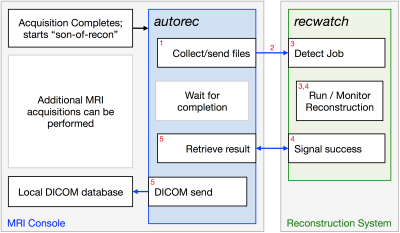 |
120 |
Automated Reconstruction Processing

Eric Borisch, Roger Grimm, Stephen Riederer
A new pair of open-source tools designed to make it easier for researchers to perform automated (no operator intervention) processing of acquired data is described. The first tool handles collection of the required input files on the MR system, submission to an external reconstruction server, and retrieval and import of resulting DICOM images to the system. The second tool manages the reconstruction system, handling prioritization, launching, and monitoring of the reconstruction process.
|
|
4839.
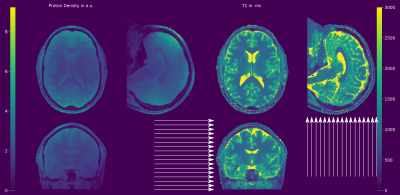 |
121 |
3D Model-Based Parameter Quantification on Resource Constrained Hardware using Double-Buffering

Oliver Maier, Matthias Schloegl, Kristian Bredies, Rudolf Stollberger
Reconstructing 3D parameter maps of huge volumes entirely on the GPU is highly desirable due to the offered computation speed-up. However, GPU memory restrictions limit the coverable volume. To overcome this limitation, a double-buffering strategy in combination with model-based parameter quantification and 3D-TGV regularization is proposed. This combination warrants whole volume reconstruction while maintaining the speed advantages of GPU-based computation. In contrast to sequential transfers, double-buffering splits the volume into blocks and overlaps memory transfer and kernel execution concurrently, hiding memory latency. The proposed method is able to reconstruct arbitrary large volumes within 5.3 min/slice, even on a single GPU.
|
|
4840.
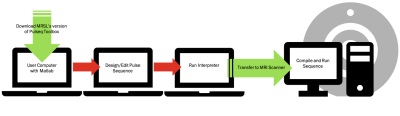 |
122 |
Development of Interpreter Module for Generating Varian VNMRJ Compatible Pulse Sequences using Pulseq Open-Source Toolbox

Courtney Bauer, Steven Wright
Introduction of a Pulseq interpreter module to enable use of the Pulseq toolbox with Varian-based legacy systems. Targeted as an aid for both educational and research applications, the interpreter module’s development centered around flexibility and ease of use. Preliminary evaluation of the interpreter module has presented promising results when compared to the existing Varian standard sequences. The interpreter module is still in refinement, with plans to introduce new features such as variable names for amplitudes and comparison methods to identify user defined shapes that are already present in the system library.
|
|
4841.
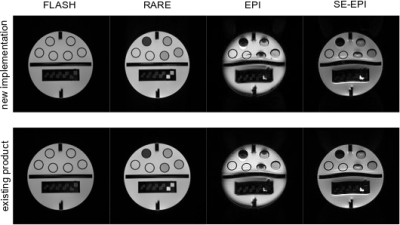 |
123 |
Dynamic platform-independent MRI vs. manufacturer’s implementations

Simon Konstandin, Cristoffer Cordes, Matthias Günther
MR sequence development is usually performed within vendor-specific frameworks, which do not allow for an easy sequence transfer to other manufacturers’ scanners. A platform-independent rapid prototyping environment for MR sequences was presented to allow both, a sequence transfer without code compilation and the generation of dynamic sequences at the scanner. This framework was used to implement a set of standard sequences and modules, which can easily be exchanged or implemented into different sequences. The aim of this work is to show that this approach of vendor-independent sequence development produces same image results as the sequences provided by the manufacturer.
|
|
4842.
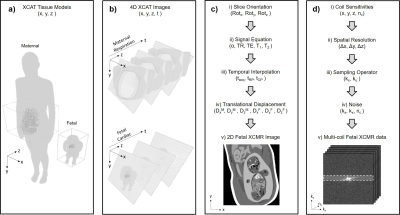 |
124 |
A Realistic Numerical Simulation for Fetal Cardiac MRI

Christopher Roy, Davide Marini, William Segars, Mike Seed, Christopher Macgowan
Validating new techniques for fetal CMR is challenging due to random fetal movement that precludes repeat measurements. Consequently, fetal CMR development has been largely performed using physical phantoms or postnatal volunteers. In this work, we present an open-source simulation designed to aide in the development and validation of new approaches for fetal CMR. Our phantom: Fetal XCMR, builds on established methods for simulating MR acquisitions but is tailored toward the dynamic physiology of the fetal heart and body. We present comparisons between the Fetal XCMR phantom and data acquired in utero, resulting in image quality, anatomy, tissue signals and contrast.
|
|
4843.
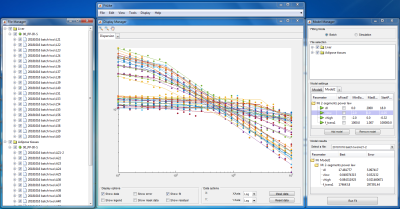 |
125 |
FitLike, a software for the analysis of T1 dispersion for Fast Field-Cycling experimentation

Manuel Petit, Hana Lahrech, Lionel Broche
Since early 2000 commercial solutions are available to study the dispersion of T1 with the magnetic field strength. This has generated a growing interest in T1 relaxometry study of sample material and a large amount of data to analyse. Yet data analysis for T1 relaxometry is almost entirely done with homemade software, which makes access to the technology difficult and limits the exchanges between research groups. Here we propose a new tool for the analysis of T1 dispersion profiles, software called FitLike that runs with Matlab.
|
|
Top |
Machine Learning for Image Enhancement, Quality Assessment & Synthetic Image Generation
Digital Poster
Acquisition, Reconstruction & Analysis
Thursday, 16 May 2019
Exhibition Hall |
13:45 - 14:45 |
|
|
Computer # |
|
4844.
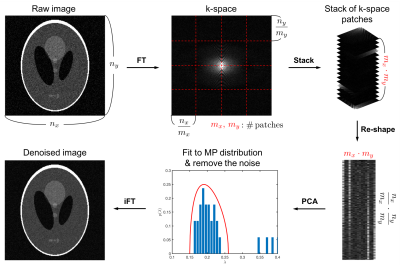 |
126 |
Single image denoising and noise map estimation using random matrix theory

Hong Hsi Lee, Els Fieremans, Dmitry Novikov
Conventional denoising and noise level estimation typically require data redundancy from multiple measurements or prior assumptions, such as smooth image prior or similarity between image patches. Here, we propose a single image denoising algorithm with noise map estimation by identifying the noise-only principle components based on universal properties of random covariance matrices, with the data redundancy created by segmenting data in the Fourier or wavelet domains. The proposed method is applicable to medical and other imaging modalities with spatially-varying noise, and is particularly beneficial to quantitative MRI acquisitions with a limited number of scans.
|
|
4845.
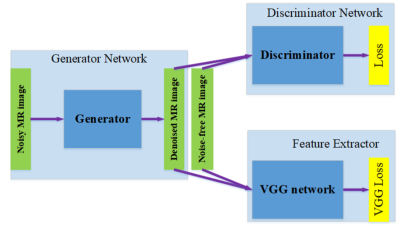 |
127 |
3D MRI Denoising with Wasserstein Generative Adversarial Network

Maosong Ran, Jinrong Hu, Yang Chen, Hu Chen, Huaiqiang Sun, Jiliu Zhou, Yi Zhang
MR image is easily affected by noise during the high-speed and high-resolution acquisition procedure. To effectively remove the noise and fully explore the potential of latest technique -- deep learning, in this abstract, we propose a novel MRI denoising method based on generative adversarial network. Specifically, to explore the structure similarity among neighboring slices, 3-D configuration are utilized as the basic processing unit. Residual autoencoder, combined with deconvolution operations are introduced into the generator network. The experimental results show that the proposed method achieves superior performance relative to several state-of-art methods in both noise suppression and structure preservation.
|
|
4846.
 |
128 |
Automated slice-to-volume registration between histology and whole-brain post-mortem MRI

Istvan Huszar, Menuka Pallebage-Gamarallage, Benjamin Tendler, Sean Foxley, Mattias Heinrich, Martin Turner, Olaf Ansorge, Karla Miller, Mark Jenkinson
Validating MRI data against histological ground truth is essential in the process of devising disease-specific imaging biomarkers that are sensitive to early microstructural changes in neurodegeneration. Current MRI–histology registration techniques are too labour- or resource-intensive to be used in large-scale studies. We introduce an automated pipeline for registering sparsely sampled, small (25x30mm) 2D stained histological images with 3D post-mortem MRI of the whole human brain. Our tests indicate sub-voxel (<0.5 mm) precision using simulated data, and <1 mm precision with real data. Implemented in a new, flexible image registration framework (TIRL), the pipeline is adaptable to various research needs.
|
|
4847.
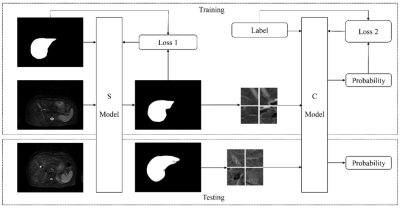 |
129 |
A Two-Step Automated Liver MR Images Quality Assessment based on Convolutional Neural Network

Yida Wang, Yang Song, Fang Wang, Zhe Han, Lei Shi, Guoliang Shao, Guang Yang
We proposed a two-step approach to evaluate automatically liver MR image quality. Firstly, we used a U-Net to segment the liver region. Then image patches were extracted from this region and another CNN was applied to estimate the quality of each image patch. The quality of the entire image was calculated based on the total percentage of 'bad' image patches in all patches. Receiver operating characteristic curve and confusion matrix were used to evaluate the performance of the proposed method. The performance of our method was comparable to human image readers.
|
|
4848.
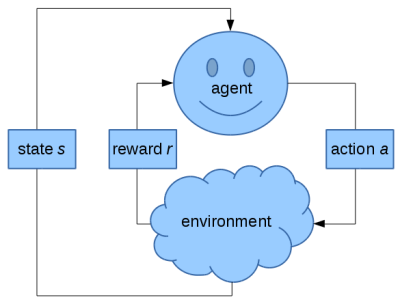 |
130 |
Reinforcement Learning for Automated Reference-free MR Image Quality Assessment

Annika Liebgott, Jianming Yi, Thomas Küstner, Konstantin Nikolaou, Bin Yang, Sergios Gatidis
Reinforcement learning is a method aiming to model a learner similar to human learning behavior. In this study, we investigate the possibility to utilize this technique to select an optimal feature set for automated reference-free MR image quality assessment. In our proposed setup, we use Q-learning and a random forest classifier to provide feedback to the learner. Moreover, we investigate a combination of multiple reinforcement learning models. Results show that our random-forest-based reinforcement learning setup can achieve higher accuracies than the previously used support vector machines or feature-based deep neural networks combined with traditional feature reduction like PCA.
|
|
4849
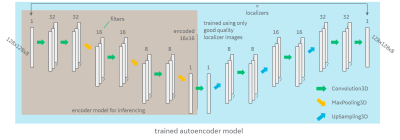 |
131 |
A deep autoencoder method for image quality assessment Video Permission Withheld
Andre Maximo, Chitresh Bhushan, Desmond Yeo, Thomas Foo
We demonstrate a classification approach for MRI image-quality based on deep auto-encoder that can be trained with samples coming from only one class (eg. only good image-quality). This approach is helpful in situations where class-imbalance is unavoidable (i.e. it is easy to obtain a large number of image samples from one class but very difficult to obtain similar number of samples from other class). Our approach shows excellent accuracy in binary classification with AUC of 0.975 in identifying MRI images of good & bad quality in clinical practice from several sites.
|
|
4850.
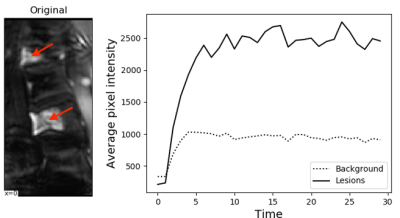 |
132 |
Automated Identification of Noise Signal in Spinal DCE-MRI using Independent Component Analysis and Unsupervised Machine Learning

Lucy Wang, Yi Wang, Murat Alp Oztek, Nina Mayr, Simon Lo, William Yuh, Mahmud Mossa-Basha
Dynamic Contrast-Enhanced (DCE) MR perfusion has shown early promise in evaluation of spinal metastatic disease and can improve prediction of treatment responses and post-treatment complications. However, spinal DCE-MRI exams frequently suffer from suboptimal image quality due to factors including cerebral spinal fluid (CSF) and vascular pulsation, respiration, bowel motion and patient bulk motion. Independent component analysis has been successfully used as a method to identify and remove motion artifacts from functional MR images. In this work, we combine ICA with an unsupervised machine learning method to automatically identify image components arising from contrast-enhancing tissues and those due to artifacts.
|
|
4851.
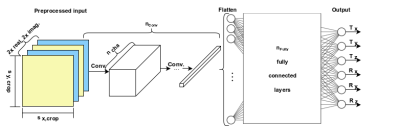 |
133 |
Deep Neural Networks for Motion Estimation in k-space: Applications and Design

Julian Hossbach, Daniel Splitthoff, Melissa Haskell, Stephen Cauley, Heiko Meyer, Josef Pfeuffer, Andreas Maier
While image-based motion estimation with Deep Learning has the advantage of an easier comprehension by a human observer, there are benefits to address the issue in k-space, as the distortion only affects echo trains locally; furthermore, Neural Networks can be designed to rely on the intrinsic k-space structure instead of image features. To our knowledge, these advantages have not been exploited so far. We show that fundamental Deep Neural Network techniques can be used for motion estimation in k-space, by examining different networks and hyperparameters on a simplified problem. We find suitable architectures for extracting 2D transformation parameters from under-sampled k-spaces for slice registration. This leads to a minimum residual of around 1.2 px/deg.
|
|
4852.
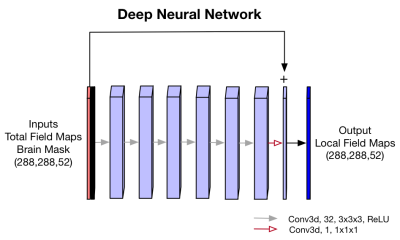 |
134 |
Deep Residual Neural Networks for QSM Background Removal

Juan Liu, Andrew Nencka, Kevin Koch
Quantitative Susceptibility Mapping (QSM) is a MR post-processing technique that estimates underlying tissue magnetic susceptibilities. In QSM processing pipelines, background field removal is of vital importance to obtain local tissue field estimates for precise susceptibility quantification. Existing background field removal methods such as SHARP, RESHARP, PDF, and LBV can effectively remove the background field. However, they struggled in clinical applications with large slice thickness and resulting non-isotropic resolutions. To address the limitations of these existing pre-processing methods in clinical QSM practice, a deep-learning-based method was proposed to approximate the underlying tissue field maps from total field maps. In-vivo datasets acquired using clinical SWI protocol demonstrated the improved performance of this approach, compared to conventional existing methods.
|
|
4853.
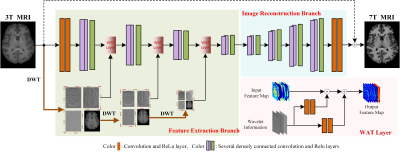 |
135 |
3T to 7T MRI Synthesis via Deep Learning in Spatial-Wavelet Domains

Liangqiong Qu, Shuai Wang , Yongqin Zhang, Pew-Thian Yap, Dinggang Shen
Ultra-high field 7T MRI scanners, while producing images with exceptional anatomical details, are cost prohibitive and hence highly inaccessible. In this abstract, we propose a novel deep learning network to synthesize 7T T1-weighted images from their 3T counterparts. Our network jointly considers both spatial and wavelet domains to facilitate learning for coarse to fine details.
|
|
4854.
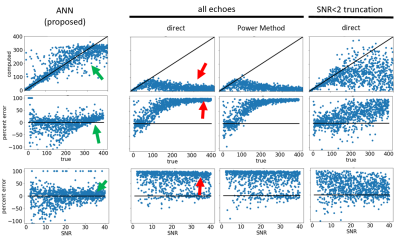 |
136 |
Overcoming the Rician Noise Bias of T2* Relaxometry with an Artificial Neural Network (ANN)

Ferdinand Schweser, Thomas Jochmann, Robert Zivadinov
Rician noise represents the major source of bias in parametric fitting techniques, such as the estimation of the T2* relaxation time. This bias is particularly strong when the signal-to-noise ratio is low or T2* values are short, such as in clinical cases of severe brain or liver iron overload. In this work, we trained a deep convolutional neural network to recognize Rician noise and compute unbiased relaxation parameters from multi-echo gradient echo data.
|
|
4855.
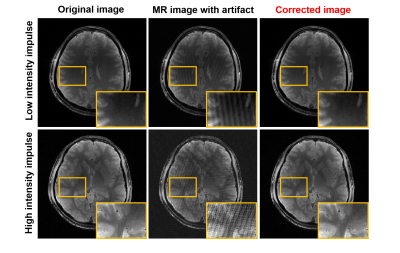 |
137 |
k-space deep learning for MR herringbone artifact correction

Juyoung Lee, Jong Chul Ye
Herringbone artifact is caused by power fluctuation of MR equipment or unstable shielding. Herringbone artifact image is difficult to analyze because it scatters on whole image region of single or multiple slices. There is a study for MR artifact correction which can be represented as sparse outliers on k-space. This method exploits the duality between the low-rankness of Hankel matrix in k-space and the sparsity in the image domain. However, this method has high computational complexity, and consumes much time. In this research, we suggest the new effective and fast MR artifact correction method using deep learning.
|
|
4856.
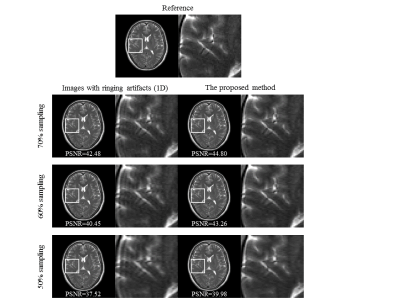 |
138 |
Gibbs-Ringing Artifact reduction in MR images with varying sampling levels Via a Single Convolutional Neural Network

Guohui Ruan, Qianqian Zhang, Biaoshui Liu, Wei Yang, Yingjie Mei, Ed X. Wu, Yanqiu Feng
Gibbs-ringing artifact is caused by the insufficient sampling of the high frequency data. And in clinical practice, the appearance of ringing artifact, i.e. the real sampling level, is not accurately obtained. To address this problem, a single convolutional neural network (CNN) has been trained for reducing Gibbs-ringing artifact in MR images under varying sampling levels. The experimental results demonstrate that Gibbs-ringing artifact can be effectively reduced by the proposed method without introducing noticeable blurring.
|
|
4857.
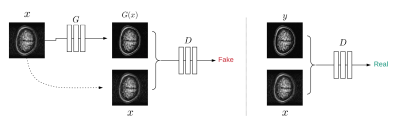 |
139 |
Motion Correction of Magnitude MR Images using Generative Adversarial Networks

Yuan Bian, Ye Wang, Stanley Reeves
Motion during MRI scan can reduce image quality due to the induced artifacts. We present a novel data-driven motion correction method for magnitude MR images using generative adversarial networks (GANs). GANs (Pix2pix model) is implemented to reduce motion artifacts and reconstruct motion-corrupted images through adversarial training between generator and discriminator to force motion-corrected image close to the reference image. The training set is made of image pairs, which consist of motionless reference images and corresponding motion-simulated images. The proposed method was validated by a simulated motion test set and a real motion (experimental) test set.
|
|
4858.
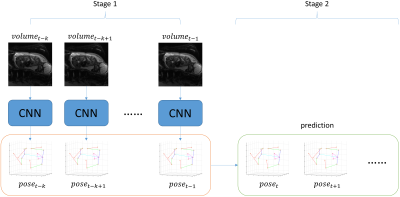 |
140 |
Fetal Motion Prediction from Volumetric MRI using Machine Learning

Junshen Xu, Molin Zhang, Larry Zhang, Ellen Grant, Polina Golland, Elfar Adalsteinsson
Prospective motion correction is a challenge in clinical fetal MR imaging as fetal motion is erratic and often substantial. To address this problem, we propose a two-stage machine learning pipeline to extract fetal poses from echo planar MRI volumes at previous time points to predict future pose. This pipeline can be used to learn kinematic models of fetal motion and serve as valuable auxiliary information for real-time, online slice prescription in fetal MRI.
|
|
4859.
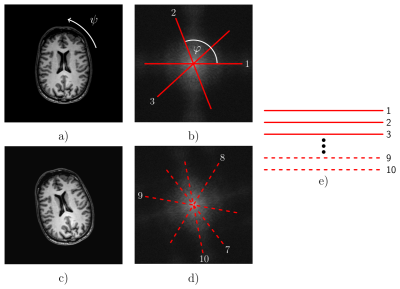 |
141 |
Head Movement Detection from Radial k-Space Lines using Convolutional Neural Networks – A Digital Phantom Study

Maximilian Wattenberg, Jannis Hagenah, Constantin Schareck, Floris Ernst, Martin Koch
Magnetic resonance imaging-guided linear particle accelerators use reconstructed images to adapt the radiation beam to the tumor location. Image-based approaches are relatively slow, causing healthy tissue to be irradiated upon subject movement. This study targets on the use of convolutional neural networks to estimate rigid patient movements directly from few acquired radial k-space lines. Thus, abrupt patient movements were simulated in image data of a head. Depending on the number of acquired spokes after movement, the network quantified this motion precisely. These first results suggest that neural network-based navigators can help accelerating beam guidance in radiotherapy.
|
|
4860.
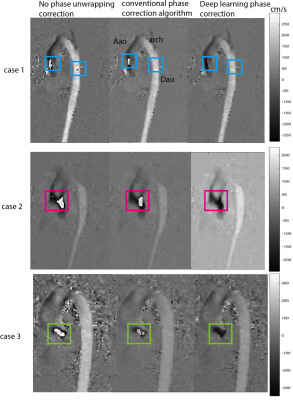 |
142 |
Deep Learning based Velocity Aliasing Correction for 4D Flow MRI

Haben Berhane, Hassan Haji-Valizadeh, Joshua Robinson, Michael Markl, Cynthia Rigsby
We developed a convolutional neural network to detect and correct velocity aliasing in 4D Flow datasets. Our network uses an Unet architecture and was trained, validated, and tested on 100, 10, and 100 datasets respectively. It was able to detect as many or more phase wrapped voxels compared to the conventional algorithm and performed better on highly aliased regions of the dataset.
|
|
4861.
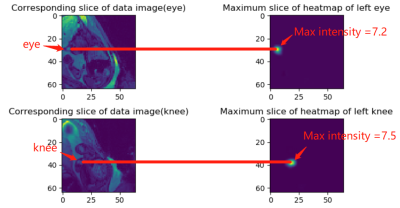 |
143 |
Fetal Pose Estimation via Deep Neural Network by Detection of Fetal Joints, Eyes, and Bladder

Molin Zhang, Junshen Xu, Esra Turk, Larry Zhang, P.Ellen Grant, Karen Ying, Polina Golland, Elfar Adalsteinsson
Neural networks and deep learning have achieved great success in human pose estimation through the identification of key human points in conventional photography and video. We propose fetal pose estimation in a time series of echo planar MRI volumes of the pregnant abdomen via deep learning algorithms for detection of key fetal landmarks, including joints, eyes, and bladder. Fetal pose estimation in an EPI time series yields novel means of quantifying fetal movements in health and disease, and enables learning of kinematic models that may enhance mitigation of fetal motion artifacts during MRI acquisition.
|
|
4862.
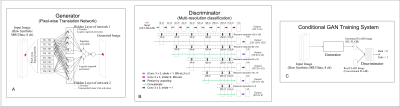 |
144 |
Improving the Quality of Synthetic FLAIR Images with Deep Learning Using a Conditional Generative Adversarial Network for Pixel-by-Pixel Image Translation

Akifumi Hagiwara, Yujiro Otsuka, Masaaki Hori, Yasuhiko Tachibana, Kazumasa Yokoyama, Shohei Fujita, Christina Andica, Koji Kamagata, Ryusuke Irie, Saori Koshino, Tomoko Maekawa, Lydia Chougar, Akihiko Wada, Mariko Takemura, Nobutaka Hattori, Shigeki Aoki
Synthetic FLAIR images are of lower quality than conventional FLAIR images. Here, we aimed to improve the synthetic FLAIR image quality using deep learning with pixel-by-pixel translation through conditional generative adversarial network training. Forty patients with MS were prospectively included and scanned to acquire synthetic MRI and conventional FLAIR images. Acquired data were divided into 30 training and 10 test datasets. Using deep learning, we improved the synthetic FLAIR image quality by generating FLAIR images that have contrast that is closer to that of conventional FLAIR images and fewer granular and swelling artifacts, while preserving the lesion contrast.
|
|
4863.
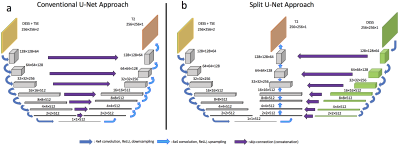 |
145 |
Synthesizing T2 Maps from Morphological OAI Scans Using Conditional GANs and a Split U-Net

Bragi Sveinsson, Bo Zhu, Akshay Chaudhari, Neha Koonjoo, Matthew Rosen
We explore the feasibility of artificially adding an exam to an MRI scan protocol by synthesizing the desired exam from the acquired images. To achieve this, we both use a normal U-Net as well as a modified U-Net structure, which takes advantage of prior information of which exams of the protocol are most relevant to the high-resolution and low-resolution components of the desired contrast. We demonstrate results based on synthesizing T2 relaxation time maps using imaging data obtained from the Osteoarthritis Initiative.
|
|
4864.
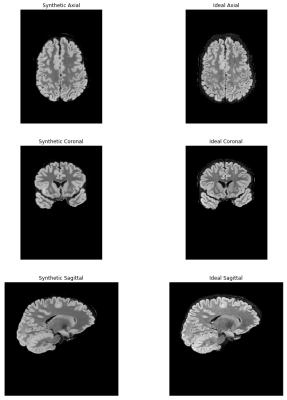 |
146 |
Build-a-FLAIR: The synthetic generation of T2-FLAIR contrast from T2-weighted and diffusion metric images through a deep neural network.

Andrew Nencka, Brad Swearingen, Kevin Koch, Michael McCrea
A deep neural network is presented to synthetically generate T2FLAIR weighted images from other standard neuroimaging acquisitions. Network performance improved with input images that share components with similar physical sources of contrast as the T2FLAIR contrast, while performance was degraded when disparate sources of contrast, like fractional anisotropy, were included. This suggests that a level of feature engineering is appropriate when building deep neural networks to perform style transforms with respect to MRI contrast, with input features containing shared physical sources of contrast with the desired output contrast. In the optimally trained network, pathology present in the acquired T2FLAIR images and not present in the training dataset was correctly reconstructed.
|
|
4865.
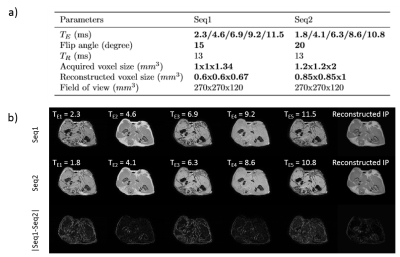 |
147 |
The impact of variable MRI acquisition parameters on deep learning-based synthetic CT generation

Mateusz Florkow, Frank Zijlstra, Koen Willemsen, René Castelein, Peter Seevinck, Marijn van Stralen
Deep learning-based synthetic CT generation models are generally trained and evaluated on MR images obtained with a single set of acquisition parameters. In this study, we investigated the robustness of such models to clinically plausible changes in acquisition parameters by training and evaluating models on MR images acquired and reconstructed from gradient echo sequences at different echotimes (TE), resolution and flip angles. We investigated the sensitivity to TEs by training models on randomly interspersed multi-echo gradient echo MR images acquired at different TEs. Multi-echo trained models achieved better generalization performance to varying acquisition parameters without excessively compromising results on dedicated data.
|
|
4866.
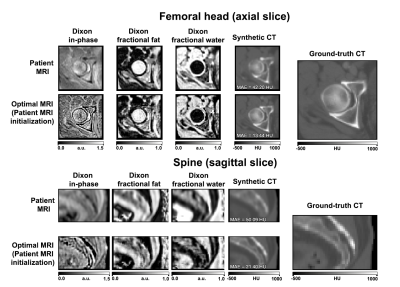 |
148 |
Visualizing the “ideal” input MRI for synthetic CT generation with a trained deep convolutional neural network: Can we improve the inputs for deep learning models?

Andrew Leynes, Peder Larson
Deep learning has found wide application in medical image reconstruction, transformation, and analysis tasks. Unlike typical machine learning workflows, MRI researchers are able to change the characteristics of images that are used as inputs to deep learning models. We proposed an algorithm that allows us to visualize the “ideal” input images that would provide the least error for a trained deep neural network. We apply this visualization technique on a deep convolutional neural network that converts Dixon MRI to synthetic CT images. We briefly characterize the optimization behavior and qualitatively analyze the features of the “ideal” input image.
|
|
4867.
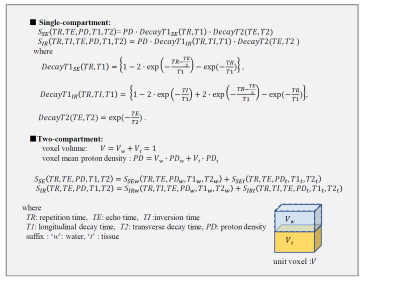 |
149 |
Synthetic MRI with water suppression technique to reduce CSF partial-volume artifacts

Tokunori Kimura, Yuki Takai, Hiroshi Kusahara, Hitoshi Kanazawa, Ryo Shiroishi
We proposed a new synthetic-MRI technique combined with water suppression to reduce CSF partial volume effects (PVE) artifacts problematic in a conventional synthetic-MRI. Our water suppression was simply achieved by subtracting additionally acquired long-TE SE image of water signal dominant. After the quantitative parameter maps of original and with water suppression were generated, water-suppressed synthetic-SE and -FLAIR images were calculated using those suitable combinations. We demonstrated that CSF PVE artifacts were dramatically reduced in our proposed synthetic-FLAIR, and furthermore that, by the two-compartment model simulation and volunteer MR brain study, our synthetic-SE provided better gray-white matter contrasts compared to our synthetic-FLAIR.
|
|
4868.
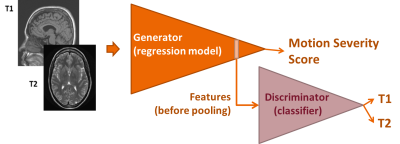 |
150 |
Towards Contrast-Independent Automated Motion Detection Using 2D Adversarial DenseNets

Silvia Arroyo-Camejo, Benjamin Odry, Xiao Chen, Kambiz Nael, Luoluo Liu, David Grodzki, Mariappan Nadar
Patient motion is a challenging and common source of artifacts in MRI. Two recent studies investigating motion detection with convolutional neural networks showed promising results, but did not generalize to varying MRI contrasts. We present a unified, domain adapted deep learning routine to provide automated image motion assessment in MR brain scans with T1 and T2 contrast. We aim to limit the influence of varying image contrasts, scanner models, and scan parameters in the motion detection routine by using adversarial training.
|
|
Top |
Machine Learning for Prediction & Image Analysis
Digital Poster
Acquisition, Reconstruction & Analysis
Thursday, 16 May 2019
Exhibition Hall |
13:45 - 14:45 |
|
|
Computer # |
|
4869.
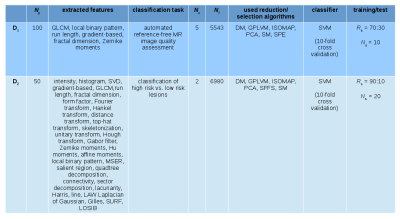 |
151 |
Feature Reduction and Selection: a Study on their Importance in the Context of Radiomics

Annika Liebgott, Janik Steyer-Ege, Tobias Hepp, Thomas Küstner, Konstantin Nikolaou, Bin Yang, Sergios Gatidis
Using large amounts of image features in the context of Radiomics to perform complex image analysis tasks yields promising results for clinical applications. While it is easy to extract a large amount of features from medical images, it is complex to select the right features for a specific scientific problem. This study aims to show, how important it is to pay attention to choosing the right technique to select the most suitable features by means of feature reduction or selection on the example of two Radiomics-related MR image classification tasks.
|
|
4870.
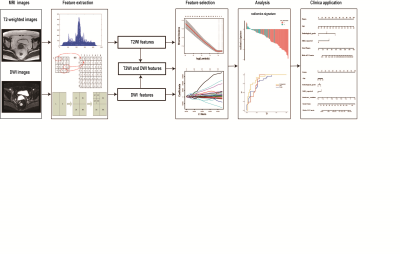 |
152 |
Preoperative prediction of pelvic lymph nodes metastasis in early-stage cervical cancer using radiomics model based on T2-weighted and diffusion-weighted MRI

Tao Wang, Tingting Gao, Liyu Huang, Ming Zhang
1. A radiomics nomogram for preoperatively predicting of PLN metastasis in patients with ECC was developed and validated. 2. The model displayed good performance (C-index=0.893 in primary cohort and C-index=0.922 in validation cohort).
3. The radiomics nomogram showed a significant improvement over the clinical nomogram in predicting PLN metastasis.
4. The radiomics signature derived from the combined T2WI and DWI has the best performance.
|
|
4871.
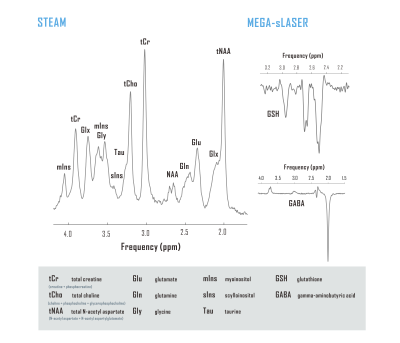 |
153 |
Diagnosis of Multiple Sclerosis Subtype through Machine Learning Analysis of Frontal Cortex Metabolite Profiles

Abhinav Kurada, Kelley Swanberg, Hetty Prinsen, Christoph Juchem
The onset and progression of multiple sclerosis (MS) is accompanied by changes in brain biochemistry. Magnetic resonance spectroscopy (MRS) is a powerful tool for investigating these changes in vivo. Machine learning analysis of MRS-derived biochemical profiles may reveal metabolic patterns inherent in certain MS subtypes to inform their diagnosis. By employing a feature set of only metabolite concentrations derived from brain MRS data acquired at 7 Tesla, we achieved an 80% validation set accuracy for differentiating MS patients from healthy controls and a 70% validation set accuracy for differentiating relapsing-remitting and progressive MS patients.
|
|
4872.
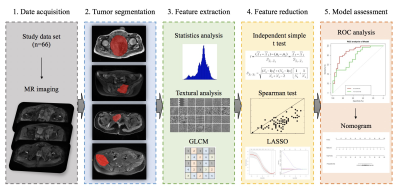 |
154 |
Differentiation of Osteosarcoma and Ewing Sarcoma Using Radiomic AnalysisBased on T2 and CET1 MRI

Yi Dai, Nan Hong, Guanxun Cheng
In this study, we assessed the ability of our newly established radiomic model based on using multiparametric MR data to help differentiate OS from EWS of the pelvis. We evaluated 16 features that were extracted and selected by using the LASSO method. Our radiomics model yielded favorable results and constituted a new technique for the discrimination of OS and EWS. The AUC was high for both T2-FS and CET1. High specificity was achieved when using data both from T2-FS and CET1 (82.9% and 100%, respectively) and the sensitivity was also high from T2-FS (74.2%). In brief, we believe that the methodology developed in this work may serve as a reliable additional tool for differentiation OS from EWS.
|
|
4873.
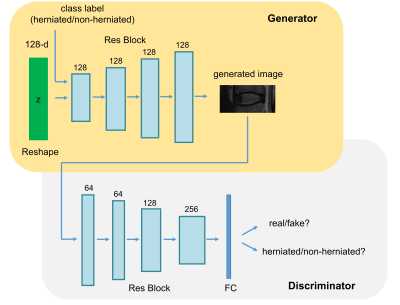 |
155 |
Generated data can boost the recognition performance for Intervertebral disc herniation Presentation Not Submitted
Fei Gao, Shui Liu, Xiaodong Zhang, Jue Zhang, Xiaoying Wang
Although deep convolutional neural network has shown encouraging performance regarding lesion classification, it is limited due to the high requirement of data labeling. In this study, we attempted to improve the recognition performance under limited labeled data using generated data for lumbar intervertebral disc herniation classification.
|
|
4874.
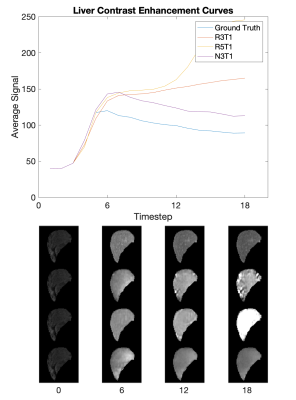 |
156 |
Deep Predictive Modeling of Dynamic Contrast-Enhanced MRI Data

Jiacheng Jason He, Christopher Sandino, Shreyas Vasanawala, Joseph Cheng
This work demonstrates the use of recurrent generative spatiotemporal autoencoders to predict up to fifteen future frames of abdominal DCE-MRI video data, starting with only three ground truth input frames for context. The objective is to predict what healthy patient video data and organ-specific contrast curves look like, to expedite anomaly detection and enable pulse sequence optimization. The model in this study shows promise; it was able to learn contrast changes without losing structural resolution during training time, and lays the foundation for future work.
|
|
4875.
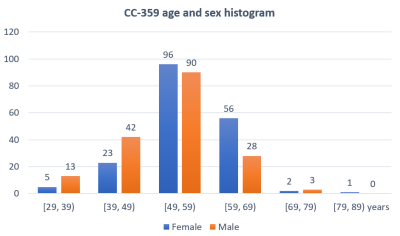 |
157 |
Automatic detection of age- and sex-related differences in human brain morphology

Renzo Phellan, Lívia Rodrigues, Gustavo Retuci Pinheiro, Andrés Quiroga Soto, Igor Duarte Rodrigues, Leticia Rittner, Ricardo Ferrari, Matthew Brown, Nils Forkert, Roberto Medeiros, Mariana Bento
Research on neurological and mental disorders has shown the diagnostic potential of volumetric brain analysis, also evidencing differences of human brain structures regarding sex and aging in normal subjects. This study aims at identifying the most important volumetric sex- and age-related differences of brain structures using machine learning approaches. It was found that the most important brain structures were different for age- and sex-related differences, which should be taking into account when diagnosing neurological and mental disorders based on morphological features.
|
|
4876.
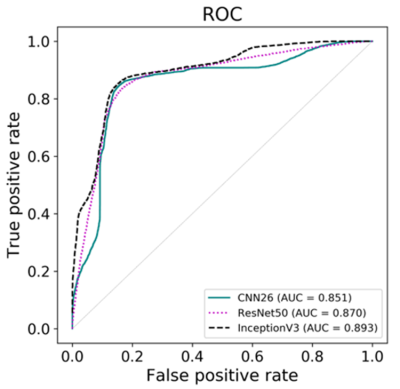 |
158 |
Survival prediction from DCE-MRI kinetic parameters in patients with osteosarcoma using deep learning

Junyu Guo, Wilburn Reddick
DCE-MRI may be a prognostic biomarker for some tumors including osteosarcoma. The purpose of this study was to assess whether a DCE-MRI kinetic parameter map of osteosarcoma can provide prognostic indicators for clinical results using three deep convolution neural networks (DCNN). In this study, we found that DCNNs can provide biomarkers for overall survivals with accuracy over 0.8; three DCNNs have the comparable performance in prediction of clinical results; and the predictions using DCNN with tumor mask were significantly better than those without using tumor mask.
|
|
4877.
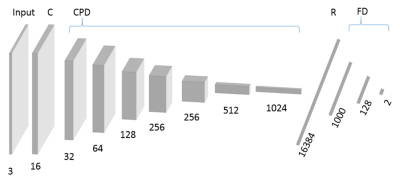 |
159 |
Deep neural network processing of original DCE-MRI data for survival prediction

Junyu Guo, Wilburn Reddick
DCE-MRI is a valuable tools in many clinical applications, but data analysis is complex. The purpose of this study was to assess whether the original DCE images without complex modeling can be used to predict the clinical results of osteosarcoma using deep convolution neural network (DCNN). We also assess whether the prediction from original images were different from those using the kinetic parameters. We found that DCNN can predict overall survivals with an accuracy of about 0.8 using a set of 2D DCE tumor images, which is not significantly different from results based on kinetic parameter maps.
|
|
4878.
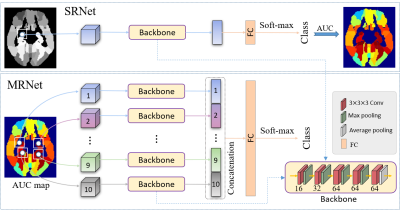 |
160 |
Characterizing MRI Biomarkers for Conversion Prediction of Preclinical Mild Cognitive Impairment

Yongsheng Pan, Mingxia Liu*, Chunfeng Lian, Ling Yue, Shifu Xiao, Yong Xia*, Dinggang Shen*
Identifying subjects at the stage of preclinical mild cognitive impairment (pre-MCI) is fundamental for early intervention of pathologic cognitive decline. This study aims to investigate the progression from cognitive normal (CN) and subjective cognitive decline (SCD) to MCI, by characterizing imaging biomarkers in brain MRI data via a deep-learning framework. This deep-learning framework is designed to first evaluate the discriminative capability of regions-of-interest (ROIs) in brain MR images, and then to predict the progression of CN/SCD subjects to MCI within 36 months. The results suggest that brain structure changes at the pre-MCI stage can be objectively detected in MR images by our method.
|
|
4879.
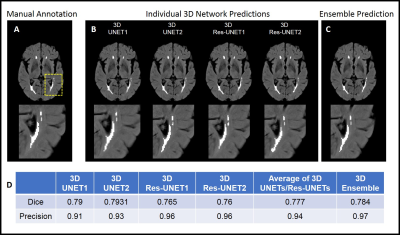 |
161 |
Detection of White Matter Hyperintensities using Ensemble 3D Deep Learning Networks

Lavanya Umapathy, Gloria Guzman, Jose Rosado-Toro, Gokhan Kuyumcu, Maria Altbach, Blair Winegar, Craig Weinkauf, Ali Bilgin
White matter hyperintensities (WMH), hyperintense on T2-weighted FLAIR images are prominent features of demyelination and axonal degeneration in cerebral white matter. The time-consuming nature of manual segmentation necessitates the need for faster and reliable automated segmentation algorithms. In this work, we propose three deep learning architectures for WMH detection on 3D FLAIR images: a modified UNET3D, Res-UNET3D and their ensemble combination. Two UNET3D and two Res-UNET3D were trained with random initialization using 3D patches sampled from within the brain. The posterior probabilities for WMH from individual networks were averaged to obtain a revised posterior probability for the ensemble. Performance of the individual networks as well as that of the ensemble was assessed using dice and precision scores. It was observed that the ensemble of 3D networks yields improved dice and precision scores in comparison to an average of individual networks, thereby reducing the effect of choice of network or parameters. Furthermore, the average dice scores for the ensemble approached the inter-observer variability of human observers.
|
|
4880.
 |
162 |
3D Convolutional Networks to predict Total Knee Replacement using Structural MRI

Tianyu Wang, Kevin Leung, Kyunghyun Cho, Gregory Chang, Cem Deniz
Osteoarthritis (OA) is a chronic degenerative disorder of joints and is the most common reason leading to total knee joint replacement (TKR). In this work, we developed an automated OA-relevant imaging biomarker identification system based on MR images and deep learning (DL) methods to predict knee OA progression. Our results indicate that the combination of multiple MR images with different contrast and resolution provides the best model to predict TKR with AUC 0.88±0.01.
|
|
4881.
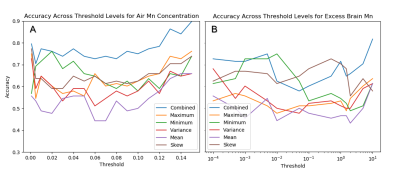 |
163 |
Whole-Brain R1 mapping predicts occupational Mn air exposure: a support vector machine approach

David Edmondson, Sébastien Hélie, Ulrike Dydak
Manganese (Mn) is a neurotoxin that can lead to symptoms similar to Parkinson’s disease. Welders exposed to welding fume can accumulate quantities of Mn in their brain eliciting T1 contrast effects. Mn exposure estimates are useful for determining a welder’s risk for symptoms, but quantifying Mn in the brain would be more beneficial. While R1 (1/T1) is proportional to local Mn accumulation, the relationship is likely non-linear, complicating interpretation of R1. Therefore, we propose a support vector machine model using whole-brain R1 maps to predict classes as determined by group, Mn air exposure, and excess brain Mn accumulation.
|
|
4882.
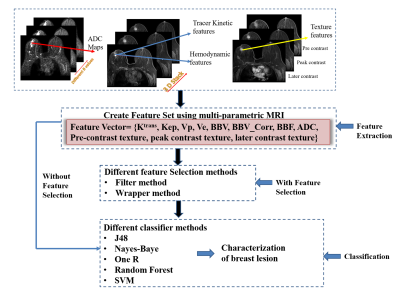 |
164 |
To evaluate the role of machine learning for characterization of breast lesion using multi-parametric MRI.

Snekha Thakran, Dinil Sasi S, Rupsa Bhattacharjee, Ayan Debnath, Rakesh Gupta, Anup Singh
The role of machine learning in medical imaging is increasing day by day. It can help in combining a variety of complementary information obtained using multi-parametric MRI(mpMRI). The objective of this study was to differentiate benign vs. malignant breast tumor using machine learning with optimized feature set obtained from mpMRI data. The study included mpMRI data of 49 patients with breast cancer. Quantitative mpMRI parameters as well as texture features were used as feature set in machine learning. The combination of the wrapper method with SVM resulted in high sensitivity (100%) and specificity (93.75%) in the binary classification of benign and malignant.
|
|
4883.
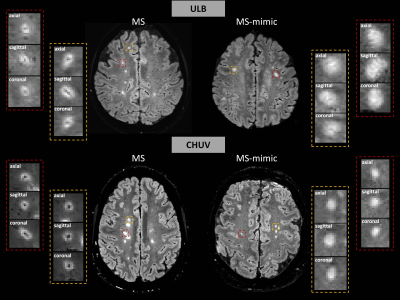 |
165 |
Differential diagnosis of multiple sclerosis based on the central vein sign assessment using deep learning: a multicentre study.

Mário João Fartaria, Jonas Richiardi, Pietro Maggi, Pascal Sati, Daniel S. Reich, Cristina Granziera, Meritxell Bach Cuadra, Tobias Kober
Prospective multicentre studies are needed to establish the clinical value of the central vein sign for diagnosis of multiple sclerosis. This type of studies requires manual segmentation and classification of lesions with and without the central vein sign, which are time-consuming tasks. In this work, we evaluate the performance of an in-house deep-learning-based prototype algorithm for automated assessment of the central vein sign using data from two different healthcare units.
|
|
4884.
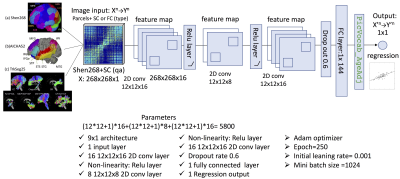 |
166 |
Structural- and Functional-Connectivity Convolution Neural Networks (SCFCnn) for Integrated Brain-Behavior Prediction in the HCP dataset

Ying-Chia Lin, Steven Baete, Xiuyuan Wang, Fernando Boada
In this work, we investigate an efficient structural (SC)- and functional (FC)-connectivity convolution neural network (SCFCnn) architecture applied on both FC and SC to detect the links between individual non-imaging language traits and invivo MRI measurements in a subset of the Human Connectome Project (HCP) s900 dataset. The identified structure-function relationships can be used to infer neurocognitive measures from neuroimaging. Our new architecture outperforms popular deep learning neural networks, confirming the importance of convolutional neural networks applied to brain connectivity for better predictive performance in neurocognitive measurements.
|
|
4885.
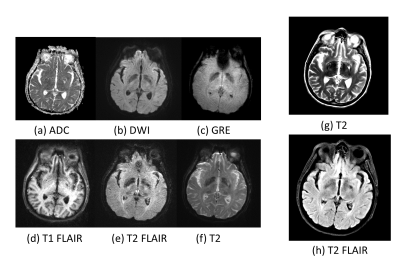 |
167 |
Enhance One-minute EPIMix Brain MRI Exams with Unsupervised Cycle-Consistent Generative Adversarial Network Presentation Not Submitted
Jiang Liu, Enhao Gong, Stefan Skare, Greg Zaharchuk
Recently, a new one-minute multi-contrast echo-planar imaging (EPI) based sequence (EPIMix) is proposed for brain magnetic resonance imaging (MRI). Despite the ultra-fast acquisition speed, EPIMix images suffer from lower signal-to-noise ratio (SNR) and resolution than standard scans. In this study, we tested whether an unsupervised deep learning framework could improve the image quality of EPIMix exams. We evaluated the proposed network on T2 and T2 FLAIR images and achieved promising qualitative results. The results suggest that deep learning could enable high image quality for ultra-fast EPIMix exams, which could have great clinical utility especially for patients with acute diseases.
|
|
4886.
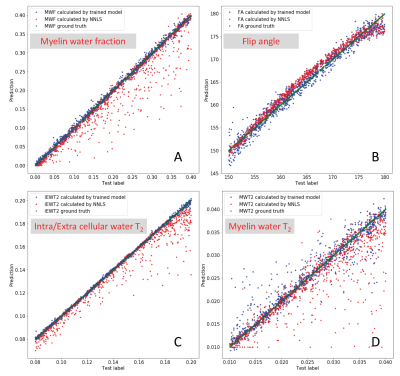 |
168 |
Analyzing multi-exponential T2 decay data using a neural network

Hanwen Liu, Roger Tam, John Kramer, Cornelia Laule
The water molecules within a single voxel may exist in different microenvironments so that the T2 relaxation is considered as a multi-exponential decay. A few quantitative imaging techniques such as myelin water imaging attempt to extract the short T2 component as a marker specific to myelin. However, decomposition of multi-exponential T2 decay data is an ill-posing problem. Commonly used non-negative least squares fitting method is slow, complex and unstable, even with strong regularization and B1 correction. We used synthetic data to train a single neural network for a better and faster analysis of the multi-exponential T2 decay data.
|
|
4887.
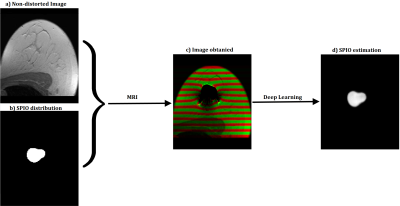 |
169 |
DeepSPIO: A SPIO particles quantification method using Deep Learning

Gabriel della Maggiora, Carlos Castillo-Passi, Qiu Wenqi, Masaki Sekino, Carlos Milovic, Pablo Irarrazaval
In this study we propose a method to quantify the distribution of Super Paramagnetic Iron Oxide (SPIO) particles with MRI. This task is particularly challenging due to the extreme distortion that these particles produce in the image. Our method is based on a supervised feed-forward deep learning model. The estimation of total quantity of SPIO was in the order of 9% error. This is potentially useful for detecting breast cancer metastasis by identifying residual particles in the breast and eventually other organs.
|
|
4888.
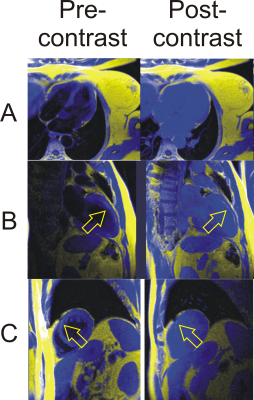 |
170 |
Will a Convolutional Neural Network Trained for Non-contrast Water-Fat Separation Generalize to Post-Contrast Acquisitions?

James Goldfarb, Jie Cao
A deep learning CNN trained using precontrast images generalizes to post-contrast images, providing equivalent image quality with fewer swap artifacts. For wide-spreadadoption of deep learning methods, it is important that they have the capability to generalize beyond training data for flexible usage. This work provides important evidence that magnetic resonance deep learning water-fat separation can be used in a variety of settings.
|
|
4889.
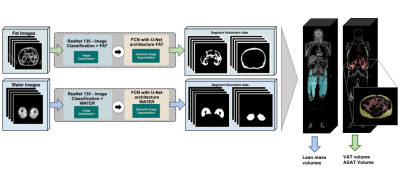 |
171 |
Fully Automated 3D Body Composition Using Fully Convolutional Neural Networks and DIXON Imaging

Alex Graff, Dmitry Tkach, Jian Wu, Hyun-Kyung Chung, Natalie Schenker-Ahmed, David Karow, Christine Leon Swisher
Here we show the first fully automated method for body composition profiling with MRI DIXON imaging. The fully automated body composition method developed can be used for radiation-free MRI risk stratification without any manual processing steps making it more accessible clinically. This would be most likely used for risk prediction and risk stratification for diseases such as type II diabetes, cardiovascular disease, and obesity.
|
|
4890.
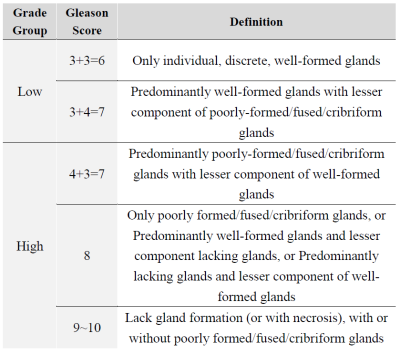 |
172 |
Proving b1000 DWI has performance advantage to classify low and high risk Gleason groups by using Neural Network classifier Presentation Not Submitted
Hongtao Zhang, Bo Wang, Zeyu Hu, Zhenjie Wu, Jiamu Xiao, Gang Wang, Shulong Wang, Huiyi Ye
The Gleason grading of histological samples is recommended for the assessment of prostate cancer risk. Assessing Gleason grade correctly can improve patient prognosis and implement early diagnosis. The aim of this work was to prove that b1000 DWI has the best effect on Gleason high-risk and low-risk grading in T2WI and DWIs with b=1000,b=2000, and b=3000. We use NN (Neural Network) with Ensemble Method on each sequence. The AUC of b1000 DWI was 0.8734, which is significantly higher than those observed for other DWIs.
|
|
4891.
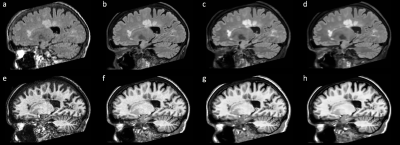 |
173 |
Harmonization of Longitudinal MRI Scans in the Presence of Scanner Changes

Blake Dewey, Can Zhao, Aaron Carass, Jiwon Oh, Peter Calabresi, Peter van Zijl, Jerry Prince
Longitudinal studies are frequently hampered by changes to scanning protocols, forcing research centers to forgo recommended upgrades to scanning equipment, software, and scan protocol design to allow for consistent scanning. Using a harmonization method that utilizes deep learning and a small (n=12) overlap cohort to learn specific differences between structural MR images before and after a significant scanning change and examined longitudinal data acquired annually over 10 years to determine if bias induced by the scanner change is still present after harmonization. We assessed these results using quantitative metrics for contrast and probed volumetric results using automated segmentation algorithms.
|
|
4892
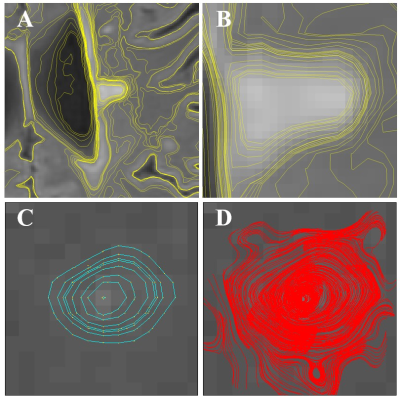 |
174 |
Deep Learning with a Novel Surface Feature for Fully Automatic Quantification of Lesion Hyperintensities in Multiple Sclerosis Video Permission Withheld
Peter Adany, In-Young Choi, Scott Belliston, Jong Chul Ye, Sharon Lynch, Phil Lee
Manual lesion segmentation presents major labor and limitations for quantitative MS lesion analysis, and recent improvements in deep learning promise more consistent, fully automatic lesion segmentation. However, convolutional neural networks still rely on learned thresholding of the arbitrary boundaries of diffuse hyperintensities. Therefore, we aimed to develop a new DL framework pairing a CNN and a custom surface feature that could detect hyperintense isocontour in 3 dimensions very sensitively. Our goal is to achieve detection of MS lesions and quantification of lesion hyperintensity volume with a new DL algorithm that combines traditional imaging and a specially designed surface feature.
|
|
4893.
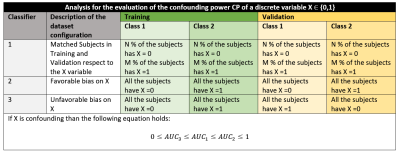 |
175 |
Tools for assessing and mitigating confounding effects in Machine-Learning based studies of MRI data

Elisa Ferrari, Giovanna Spera, Letizia Palumbo, Alessandra Retico
Using Machine Learning (ML) techniques on neuroanatomical data obtained with magnetic resonance imaging (MRI) is becoming increasingly popular in the study of Psychiatric Disorders (PD). However, this kind of analyses can be affected by overfitting and thus be sensitive to biases in the dataset, producing hardly reproducible results. It is therefore important to identify and correct possible bias sources in the sample. We present two tools aimed at addressing this matter: a methodology to assess the confounding power of a variable in a specific classification task, and a cost function to use during classifier training on highly biased data.
|
|
Back to Program-at-a-Glance | Back to Top |
The International Society for Magnetic Resonance in Medicine is accredited by the Accreditation Council for Continuing Medical Education to provide continuing medical education for physicians. |