Artificial Intelligence in Body MRI
Oral
Body: Breast, Chest, Abdomen, Pelvis
Wednesday, 15 May 2019
Room 520A-F |
13:30 - 15:30 |
Moderators: Mirabela Rusu |
13:30
|
0878.
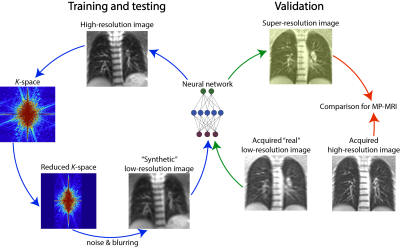 |
Super-resolution convolutional neural networks applied to functional lung MRI at 1.5T

Orso Pusterla, Franesco Santini, Rahel Heule, Damien Nguyen, Robin Sandkühler, Simon Andermatt, Pilippe C. Cattin, Corin Willers, Sylvia Nyilas, Philipp Latzin, Oliver Bieri, Grzegorz Bauman
High-resolution images are needed in many MR applications to enhance the diagnostic information at early stages of the disease. Often, the achievable resolution is limited by acquisition time constraints, in particular in moving organs such as the lung, where rapid imaging is a necessity. The low proton density in the lung parenchyma further constrains the resolution as sufficiently high signal-to-noise ratio (SNR) requires large voxel size. In this work, the concept of super-resolution is investigated to increase the spatial resolution and potentially shorten the acquisition time for functional assessment in the lung without SNR penalty.
|
13:42
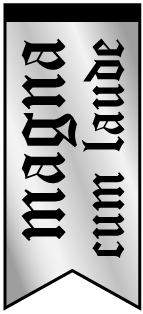 |
0874.
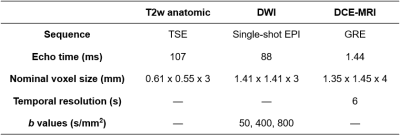 |
Investigatory usage of a framework for automated cancer annotation of pathology slides of radical prostatectomy specimens: effect on performance of a predictive model for mpMRI detection of prostate cancer.

Ethan Leng, Jin Jin, Jonathan Henriksen, Joseph Koopmeiners, Stephen Schmechel, Gregory Metzger
The development of CAD systems for prostate cancer detection requires large amounts of training data with correlated pathologic ground truth. The gold standard is manual annotation of cancer by pathologists, which is tedious and difficult to obtain. Here, we retrospectively applied a previously-described digital-pathology framework for automating cancer annotation. We trained a Bayesian predictive model on the original ground truth (from manual annotation) and on the new ground truth, and compared the performances. The results suggest the ground truths are very similar and largely equivalent, which provides support for prospective usage of our approach for automatic annotation of prostate cancer.
|
13:54
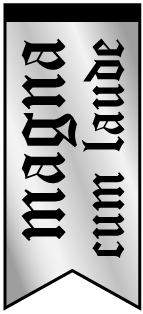 |
0875.
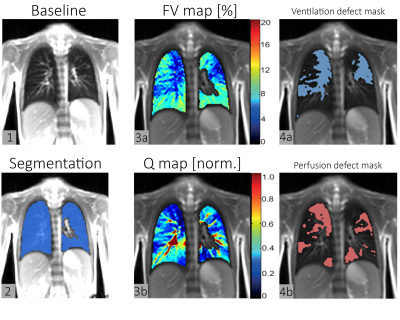 |
On the prospects of deep learning for automated lung segmentation for functional lung MRI

Corin Willers, Grzegorz Bauman, Simon Andermatt, Sylvia Nyilas, Francesco Santini, Simon Pezold, Philippe C. Cattin, Philipp Latzin, Oliver Bieri, Orso Pusterla
Deep learning algorithms have shown promise for precise organ segmentation. In this work, we investigate the prospects of deep learning for automated lung segmentation to assess impaired ventilation and perfusion measures using functional lung MRI.
|
14:06
|
0877.
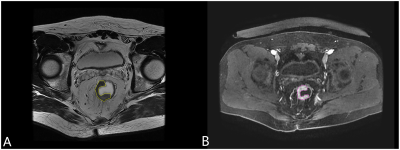 |
Machine Learning-based Analysis of Rectal Cancer MRI Radiomics for Prediction of Metachronous Liver Metastasis Did Not Present
Meng Liang, Zhengting Cai, Chencui Huang, Xinming Zhao
Early detecting patients at high risk of metachronous liver metastasis (MLM) in rectal cancer would provide the opportunity for improving prognosis and survival. In this study, we attempted to construct a non-invasive and convenient model based on rectal cancer T2WI and venous phase (VP) MR radiomics to predict MLM using support vector machine (SVM) and logistic regression (LR) algorithms. The results showed that the Modeloptimal using the LR algorithm had high potential for MLM prediction than other models. And except for ModelVP, the LR algorithm was not superior to the SVM algorithm for model construction.
|
14:18
|
0879.
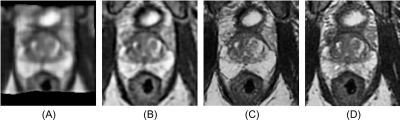 |
3D Super-resolution Prostate MRI using Generative Adversarial Networks and unpaired data

Yucheng Liu, Yulin Liu, Daniel Litwiller, Rami Vanguri, Michael Liu, Richard Ha, Hiram Shaish, Sachin Jambawalikar
We developed a novel method to generate 3D isotropic super-resolution prostate MR images using a class of machine learning algorithms known as Generative Adversarial Networks (GANs). We use GANs to generate super-resolution images with 3D SVR image slices as inputs. Super-resolution is enforced as the discriminator network is trained to distinguish the output image from in-plane T2 FSE images, resulting in the generation of super-resolution images. We use unpaired GANs since slices of 3D SVR do not usually have corresponding super-resolution images. The result is a generated continuous 3D volume with super-resolution throughout all three planes in isotropic voxel size.
|
14:30
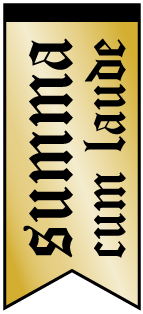 |
0872.
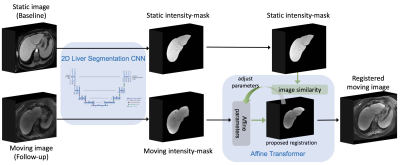 |
Fully automated registration of cross-sectional liver images using fully convolutional and affine transformation networks

Kyle Hasenstab, Guilherme Cunha, Kang Wang, Brian Hurt, Alexandra Schlein, Timoteo Delgado, Ryan Brunsing, Armin Schwartzman, Kathryn Fowler, Albert Hsiao, Claude Sirlin
Proper spatial alignment of anatomical landmarks during and between liver imaging exams is challenging due to the dynamic morphology of the liver. Liver-focused registration algorithms have been developed but are typically semiautomatic. We propose a fully-automated pipeline for affine-based registration of inter- and intra-exam liver images and assess performance on clinical liver MRI exams at 1.5T and 3T. The proposed pipeline achieved comparable or superior accuracy and scalability to that reported for previously proposed algorithms. Retrospective image review by an expert abdominal radiologist confirmed subjective improvement in anatomic registration and lesion co-localization. Proof of concept of multimodal scalability was demonstrated.
|
14:42
|
0880.
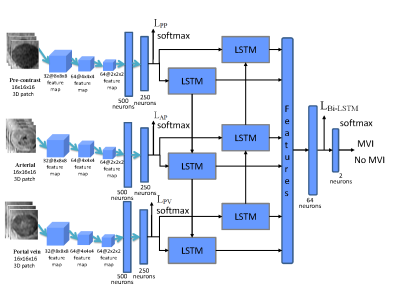 |
3D Convolutional Neural Network with Contrast-enhanced MR for Microvascular invasion prediction of hepatocellular carcinoma

Wu Zhou, Yaoqin Xie, Guangyi Wang
Microvascular invasion (MVI) of Hepatocellular carcinoma (HCC) is a crucial histopathologic prognostic factor leading to recurrence after liver transplantation or hepatectomy, and preoperative prediction of MVI is significant in clinical practice. In this work, we propose a deep learning framework based on 3D Convolutional Neural Network (CNN) to extract discriminative information from HCCs in Contrast-enhanced MR images for MVI prediction. Experimental results demonstrated that the proposed deep learning framework could make full use of the spatial and temporal information of HCCs from Contrast-enhanced MR for MVI prediction, outperforming the radiomics based approach and the method of 3D deep feature concatenation.
|
14:54
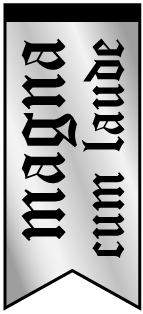 |
0873.
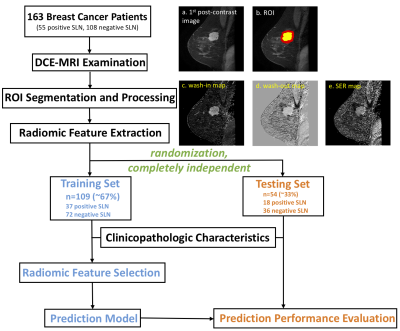 |
Optimizing peritumoral features on radiomic analysis of breast cancer

Jie Ding, Karl Spuhler, Mario Serrano Sosa, Chunling Liu, Chuan Huang
Recently, we developed a radiomic pipeline to non-invasively predict sentinel lymph node (SLN) metastasis in breast cancer using image features extracted from the primary tumor on the DCE-MRI. In this study, we further investigated the usefulness of the peritumoral features in the radiomic analysis and evaluated the effect of the thickness of the peritumoral regions to optimize the prediction performance. The result shows that the peritumoral features can indeed improve the prediction performance and using 4mm as the thickness of the peritumoral regions achieved the optimal prediction result in an independent validation set.
|
15:06
|
0876.
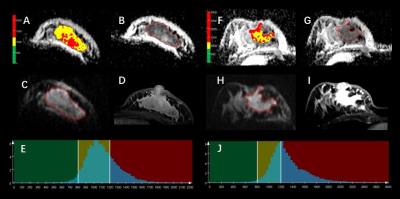 |
Differentiation Idiopathic Granulomatous Mastitis and Breast Carcinoma: Value of Whole-Lesion Histogram and Texture Analysis Using Quantitative ADC Map Did Not Present
Qiufeng Zhao, Tianwen Xie, Caixia Fu, Qiong Li, Mingqin Yan, Shijie Zhang, Fei Duan, Huimei Chao, Robert Grimm, Song Wang
Recently, DWI has been increasingly used in distinguishing benign inflammation lesions and breast cancers. A total of 15 patients with invasive ductal carcinoma (IDC) and 10 patients with idiopathic granulomatous mastitis (IGM) were retrospectively evaluated. We extracted the whole-lesion histogram and textural features from the ADC map. Univariate and multivariate logistic regression analysis was performed. The area under the curve (AUC) at the best cut-off point was assessed. Using the three significant features (difference entropy, difference variance and entropy of ADC), we obtained an AUC of 0.953 (95% CI: 0.787, 0.998) for the differentiation between IGM group from tumor group.
|
|