MRS/MRSI Analysis
Oral
Spectroscopy & Non-Proton MR
Tuesday, 14 May 2019
Room 512A-H |
13:30 - 15:30 |
Moderators: Jamie Near, Lijing Xin |
13:30
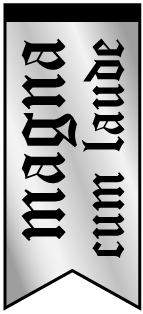 |
0510.
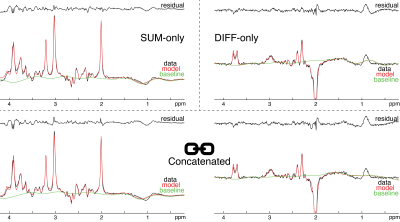 |
Simultaneous linear-combination modeling of MEGA-PRESS sum and difference spectra without soft constraints

Georg Oeltzschner, Gašper Zupan, Richard Edden
J-difference edited magnetic resonance spectroscopy is widely used to estimate levels of low-concentration metabolites with overlapping signals. Quantification is commonly performed on the difference spectra only, either using single-resonance fitting or linear-combination modeling based on simulated basis functions. Here, simultaneous linear-combination modeling of GABA-edited MEGA-PRESS sum and difference spectra is demonstrated. Simultaneous modeling incorporates all available spectral information, and does not require the definition of soft constraints on the low-concentration metabolite estimates. Across a large dataset, this new approach gave lower coefficients of variation for estimates of GABA, glutamate, and glutamine than modeling of the difference and sum spectra only.
|
13:42
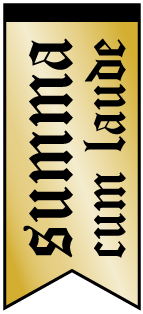 |
0511.
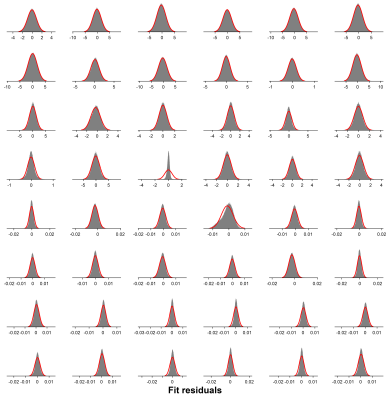 |
Robust Correction of Frequency and Phase Errors in Edited MRS Data

Mark Mikkelsen, Jamie Near, Muhammad Saleh, Stewart Mostofsky, Nicolaas Puts, Richard Edden
MRS data are subject to shot-to-shot frequency and phase errors that often arise from B0 field drift and participant motion. These result in misalignment of individual subspectra, leading to signal loss and—in the case of J-difference editing—subtraction artifacts. Here, we present a frequency-and-phase correction algorithm, built upon the time-domain-based spectral registration method, that is robust against alignment errors resulting from large B0 field drift, substantial head motion and strong lipid contamination. The method has the same strengths as standard spectral registration but outperforms it in challenging cases and is applicable to multiplexed edited MRS data.
|
13:54
|
0512.
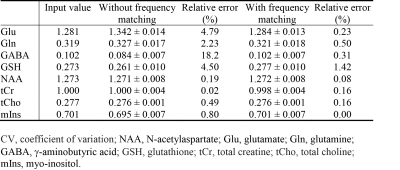 |
Effects of Carrier Frequency Mismatch on Frequency-Selective Spectral Editing

Li An, Maria Araneta, Christopher Johnson, Jun Shen
This study investigated the effects of carrier frequency mismatch on spectral editing and its correction by frequency matching of basis functions. Full density matrix computations and Monte Carlo simulations based on MRS data collected from five healthy volunteers at 7 T were used to analyze the effects of carrier frequency mismatch on spectral editing. There were significant errors in metabolite quantification without frequency matching of basis functions when carrier frequency mismatch was generally considered negligible. By matching basis functions with the history of frequency deviation, errors in glutamate, glutamine, g-aminobutyric acid, and glutathione concentrations were significantly reduced.
|
14:06
|
0513.
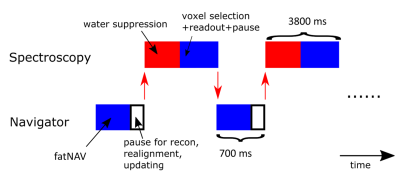 |
Prospective motion correction in single voxel spectroscopy at 7T using fat navigators and higher order shimming

Mads Andersen, Anouk Marsman, Anna Lind, Esben Petersen, Vincent Boer
Navigator based motion correction is shown for single voxel spectroscopy at 7T, utilizing fat-selective navigators and static higher order shims, but with linear shims that are switched between 2 sets for the navigator and spectroscopy sub-sequences.
|
14:18
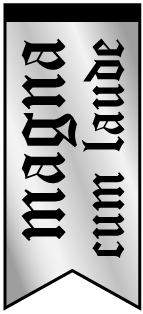 |
0514.
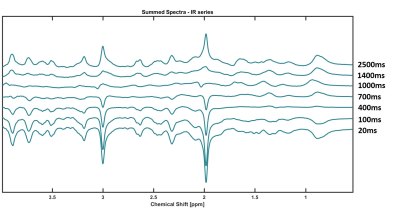 |
Longitudinal Relaxation Times of Metabolites in vivo at 9.4 T

Andrew Wright, Saipavitra Murali-Manohar, Tamas Borbath, Anke Henning
Longitudinal relaxation times for 11 human brain metabolites are reported for GM and WM rich voxels at 9.4T. These values are reported to potentiate the ability to perform absolute quantification at 9.4T in humans with reference to water. A bi-exponential model was used to fit the signal curve from using an inversion recovery metabolite cycling STEAM sequence. Results are further extrapolated to report the T1-relaxation from a theoretically pure WM and GM voxel by means of a linear assumption of the relaxation time and tissue contribution of a voxel.
|
14:30
|
0515.
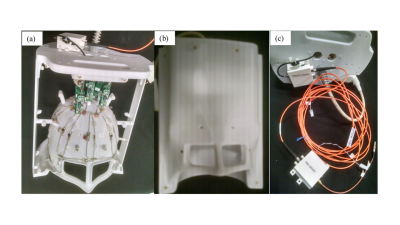 |
Towards absolute quantification of brain metabolites using Electronic REference To access In vivo Concentrations (ERETIC) for MR spectroscopic imaging (MRSI)

Bijaya Thapa, Azma Mareyam, Jason Stockmann, Boris Keil, Philipp Hoecht, Zhe Wang, Yulin Chang, Stefan Carp, Xianqi Li, Bernhard Strasser, Lawrence Wald, Ovidiu Andronesi
Absolute quantification of metabolite concentration from MRSI data requires a reference signal of known concentration. An external synthetic electronic reference signal method (Electronic REference To access In vivo Concentrations – ERETIC) has shown great promise for absolute quantification and calibration. However, ERETIC based absolute quantification is challenging for MR spectroscopic imaging (MRSI) data and here we set on investigating strategies and performance of ERETIC in combination with MRSI and multi-channel receive arrays.
|
14:42
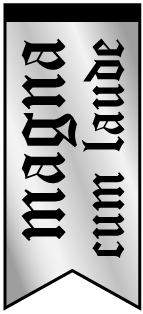 |
0516.
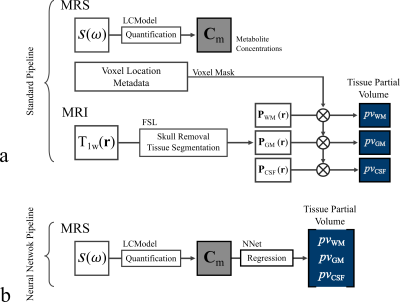 |
Estimation of Brain Tissue Composition and Voxel Location in MR Spectroscopy Using Neural Networks

Eduardo Coello, Molly Charney, Tyler Starr, Huijun Liao, Marcia Louis, Alexander Lin
This work presents a machine learning method to estimate the tissue partial volumes of gray matter (GM), white matter (WM) and cerebrospinal fluid (CSF), in a given MR spectroscopy voxel, providing an alternative to the standard time-intensive MRI segmentation pipeline. The tissue composition was determined from quantified metabolic concentrations using a neural networks regression model. Moreover, a classification model was trained to determine the brain region corresponding to a measured spectrum from both metabolic components and tissue volumes.
|
14:54
|
0517.
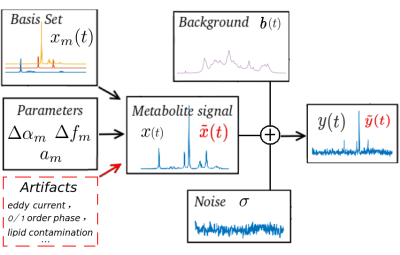 |
MR SPECTROSCOPY ARTIFACT REMOVAL WITH U-NET CONVOLUTIONAL NEURAL NETWORK

Nima Hatami, Hélène Ratiney, Michaël Sdika
In in vivo MR spectroscopy, a variety of artifacts may affect spectral quality and are not easy to detect and remove by non-experts. A U-NET architecture is proposed to remove artifacts from MRS spectra with deep learning. The principle is demonstrated on synthetic simulated data mimicking in vivo conditions.
|
15:06
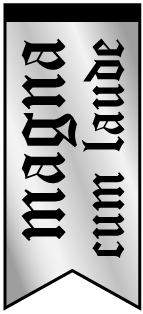 |
0518.
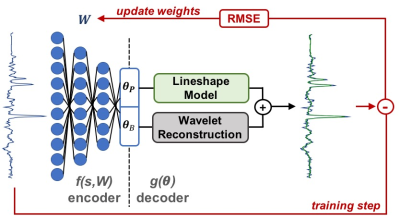 |
Accelerated Spectral Fitting Using Convolutional Neural Networks

Saumya Gurbani, Sulaiman Sheriff, Andrew Maudsley, Lee Cooper, Hyunsuk Shim
3D whole-brain spectroscopic MRI can measure quantitative metabolite concentrations without any contrast agents and is useful in identifying occult glioblastoma beyond that seen on standard MRI. However, a key hurdle in its widespread adoption is spectral fitting, which can take up to an hour for scan consisting of ~10,000 voxels. In this work, we develop a deep learning architecture for rapid spectral fitting within the context of an a priori spectral model. We demonstrate that this architecture can perform whole-brain spectral fitting in <30 seconds, pushing spectroscopic MRI towards on-board scanner processing to fit in the rapid clinical workflow.
|
15:18
|
0519.
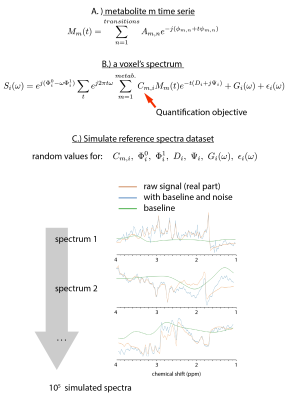 |
High-Resolution Magnetic Resonance Spectroscopic Imaging quantification by Convolutional Neural Network

Sebastien Courvoisier, Antoine Klauser, Peter Lichard, Michel Kocher, François Lazeyras
High-resolution magnetic resonance spectroscopic imaging quantification at 3T is affected by a poor signal to noise ratio as well as signal contamination from macromolecules and field inhomogeneities. For the metabolite identification problem, the convolutional neural network (CNN) algorithm seems to be a very adapted tool. We demonstrate here the performance our CNN on metabolite concentration estimation compared to the well-used LCModel. Achieving better accuracy on simulated datasets, we obtained also comparable results as LCModel on concentration maps on in-vivo data but with 103 times less computing time.
|
|