QSM & ETM
Oral
Contrast Mechanisms
Monday, 13 May 2019
Room 710B |
16:00 - 18:00 |
Moderators: Xu Li, Sina Straub |
16:00
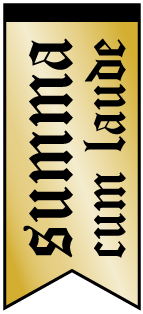 |
0317.
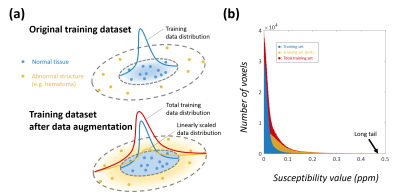 |
On the linearity of deep neural network trained QSM

Woojin Jung, Jaeyeon Yoon, Joon Yul Choi, Eung-Yeop Kim, Jongho Lee
In this work, the linearity property of a recently proposed neural network-based QSM is explored. The QSMnet, which was trained using healthy volunteers, was quantitatively evaluated for patients with hemorrhage whose susceptibility values were outside of the trained range. The results showed that the QSMnet underestimated the susceptibility in hemorrhage, breaking linearity between true susceptibility and QSMnet-generated susceptibility. To overcome this limitation, we developed a linear scaling method that generalized the network for a wider range of susceptibility. The new network successfully reconstructed the patient data with good linearity results.
|
16:12
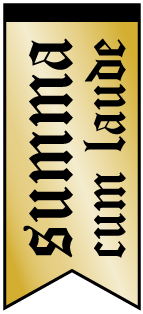 |
0318.
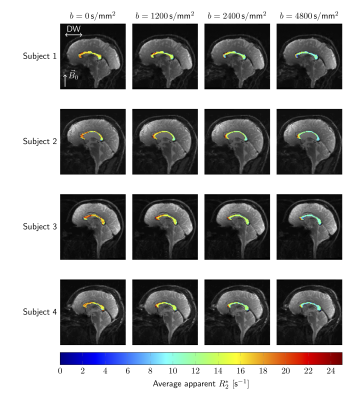 |
Separating intra- and extra-axonal susceptibility effects using a Diffusion-Filtered Asymmetric Spin Echo (D-FASE) sequence

Elena Kleban, Chantal Tax, Umesh Rudrapatna, Derek Jones, Richard Bowtell
The investigation of brain white matter is a key area of application of MRI, with much effort focused on using MR techniques to quantify tissue microstructure. Here, we bring together two complementary approaches that are commonly used to probe tissue microstructure (signal dephasing due to: (i) diffusion and (ii) microscopic susceptibility effects) using a novel Diffusion-Filtered Asymmetric Spin Echo (D-FASE) technique. Using D-FASE we can separately assess the evolution of the intra- and extra-axonal signals under the action of susceptibility effects, revealing differences in the behaviour in fibres that are parallel and perpendicular to B0.
|
16:24
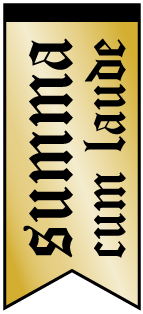 |
0319.
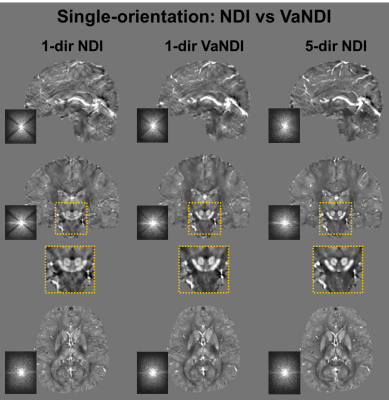 |
VaNDI: Variational Nonlinear Dipole Inversion enables QSM without free parameters

Daniel Polak, Itthi Chatnuntawech, Jaeyeon Yoon, Siddharth Iyer, Kawin Setsompop, Berkin Bilgic
We propose Nonlinear Dipole Inversion (NDI) for high-quality Quantitative Susceptibility Mapping (QSM) without additional regularization, while matching the RMSE of state-of-the-art regularized reconstruction techniques. In addition to avoiding over-smoothing these techniques often face, we also obviate the need for parameter selection. NDI is flexible enough to allow for reconstruction from an arbitrary number of head orientations, and outperforms COSMOS using as few as 2-direction data. This is made possible by a nonlinear forward-model that uses the magnitude as an effective prior, for which we derived a simple gradient descent update. We synergistically combine this physics-model with Variational Networks (VN) to leverage the power of deep learning in the VaNDI algorithm. VaNDI adopts this simple gradient descent rule and learns the network parameters during training, hence requires no additional parameter tuning.
|
16:36
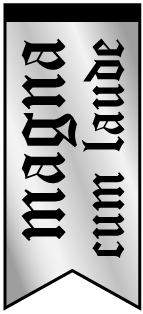 |
0320.
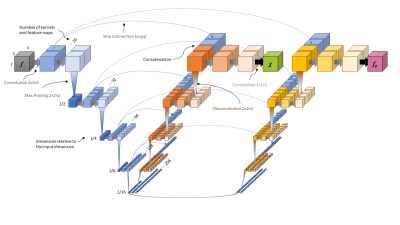 |
U2-Net for DEEPOLE QUASAR–A Physics-Informed Deep Convolutional Neural Network that Disentangles MRI Phase Contrast Mechanisms

Thomas Jochmann, Jens Haueisen, Robert Zivadinov, Ferdinand Schweser
Magnetic susceptibility is a physical property of tissues that changes with iron level and (de-)myelination. Mapping the susceptibility can help us improve our understanding of the brain and its diseases, such as multiple sclerosis and Alzheimer disease. Quantitative Susceptibility Mapping (QSM) derives the susceptibility using MRI phase data. QUASAR adds a more sophisticated physical model to QSM. Our novel U2-Net for DEEPOLE QUASAR uses deep learning to separate the magnetic field into two components from different contrast mechanisms, yields an improved susceptibility map, and shows where in the brain the tissue does not adhere to the basic QSM model, e.g. due to microstructural anisotropy.
|
16:48
|
0321.
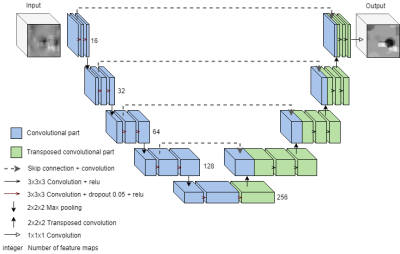 |
Deep Learning for solving ill-posed problems in Quantitative Susceptibility Mapping – What can possibly go wrong?

Pia Høy, Kristine Sørensen, Lasse Østergaard, Kieran O'Brien, Markus Barth, Steffen Bollmann
Quantitative susceptibility mapping (QSM) aims to solve an ill-posed field-to-source inversion to extract magnetic susceptibility of tissue. QSM algorithms based on deep convolutional neural networks have shown to produce artefact-free susceptibility maps. However, clinical scans often have a large variability, and it is unclear how a deep learning-based QSM algorithm is affected by discrepancies between the training data and clinical scans. Here we investigated the effects of different B0 orientations and noise levels of the tissue phase on the final quantitative susceptibility maps.
|
17:00
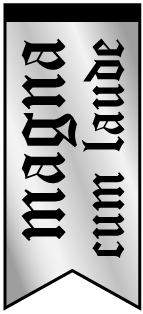 |
0322.
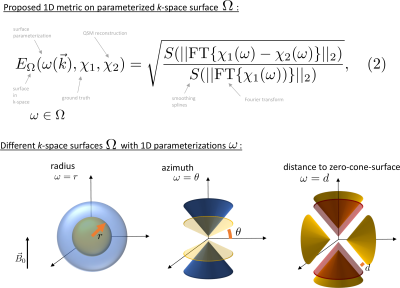 |
One-Dimensional k-Space Metrics on Cone Surfaces for Quantitative Susceptibility Mapping

Maximilian Diefenbach, Christof Böhm, Jakob Meineke, Chunlei Liu, Dimitrios Karampinos
An important question in QSM is how to compare different QSM reconstructions. We propose new metrics to compare susceptibility maps on cone surfaces in the Fourier domain following the intrinsic geometry of the dipole kernel. This physically motivated approach augments the idea of previously proposed error spectrum plots in MRI image reconstruction to specifically suit the QSM dipole inversion. The novel metrics complement previous metrics used in the 2016 QSM reconstruction challenge.
|
17:12
|
0323.
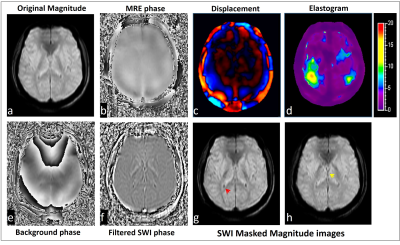 |
Free lunch may not exist, but free contrast does: Calculation of Susceptibility weighted Imaging contrast from data obtained for MR Elastography

Yogesh kannan Mariappan, Jaladhar Neelavalli, Nehul Makani
In typical phase contrast based MR imaging studies like MR Elastography, the phase of interest is extracted from the data and is used in downstream processing to calculate the relevant clinical parameters of interest. While the background phase is typically discarded, we explored the feasibility of obtaining other relevant contrast like SWI contrast from this background phase. This indeed provides useful independent information and we provide evidence on a phantom and in-vivo human experiments. For example, SWI contrast obtained from MRE data of the brain improves the visualization of diamagnetic choroid plexus and paramagnetic internal cerebral veins.
|
17:24
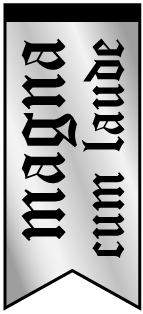 |
0324.
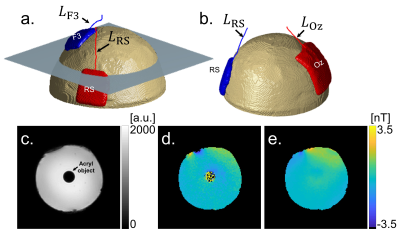 |
Artifact free direct conductivity reconstruction method using the Dual Loop and artificial neural network with one current injection in MREIT.

Saurav Zaman Khan Sajib, Munish Chauhan, Rosalind Sadleir
Most the image reconstruction algorithms in magnetic resonance electrical impedance tomography (MREIT) and diffusion tensor magnetic resonance electrical impedance tomography (DT-MREIT) require at least two independent current patterns to uniquely reconstruct conductivity distributions. However, in transcranial electrical current stimulation (tES) or deep brain stimulation (DBS) only one current injection data is available. We applied Kirchhoff’s voltage law (KVL) in a mimetic discretized network, additional current data obtained from a computational model, and a radial basis function artificial neural network (RBF-ANN) approach, to demonstrate that it is possible to reconstruct the conductivity images using a single experimental current administration.
|
17:36
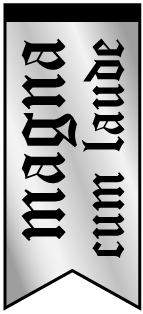 |
0325.
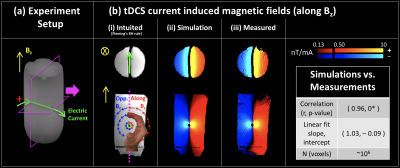 |
Concurrent mapping of electric currents and neurophysiological changes during Transcranial Direct Current Stimulation (tDCS)

Mayank Jog, Kay Jann, Lirong Yan, Danny Wang
Transcranial Direct Current Stimulation(tDCS) is a non-invasive neuromodulation technique that uses milliampere currents (applied at the scalp) to modulate cortical excitability. Although tDCS has been shown to improve clinical symptoms in depression, stroke rehabilitation and pain, the exact mechanism of tDCS is not yet fully understood. To help advance the study of tDCS mechanisms, we developed a novel technique that, for the first time, enables concurrent mapping of (i) the applied electric current and (ii) the neurophysiological changes induced in-vivo. In this work, we demonstrate the feasibility as well as reliability of this novel technique through phantom and in-vivo experiments.
|
17:48
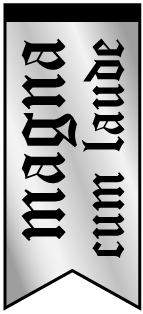 |
0326.
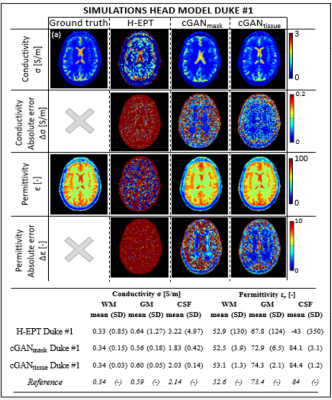 |
Deep Learning based MR Electrical Properties Tomography

Stefano Mandija, Ettore Meliadò, Niek Huttinga, Peter Luijten, Cornelis van den Berg
The inability of Magnetic Resonance Electrical Properties Tomography to accurately reconstruct tissue electrical properties severely limits its clinical use, e.g. as a biomarker in oncology. We demonstrate that the electrical properties reconstruction problem can be casted as a supervised deep learning task. Deep learning based electrical properties reconstructions for simulations and MR measurements (3 Tesla) on phantoms and human brains demonstrate great improvement in the quality of reconstructed electrical properties maps. This could be major step forward to turn electrical properties tomography into a reliable biomarker where pathological conditions can be revealed and characterized by abnormalities in tissue electrical properties.
|
|