Machine Learning for Image Reconstruction
Oral
Acquisition, Reconstruction & Analysis
Tuesday, 14 May 2019
Room 710B |
15:45 - 17:45 |
Moderators: Kerstin Hammernik, Shanshan Wang |
15:45
|
0657.
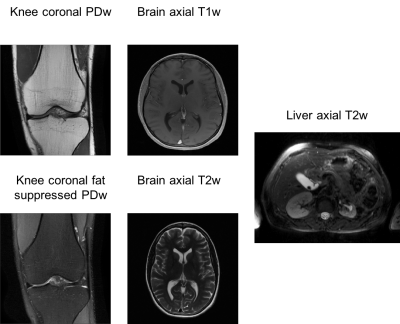 |
fastMRI: a publicly available raw k-space dataset for accelerated MRI reconstruction using machine learning

Florian Knoll, Matthew Muckley, Jure Zbontar, Anuroop Sriram, Aaron Defazio, Michal Drozdzal , Krzysztof Geras, Mary Bruno, Marc Parente, Nafissa Yakubova, Mike Rabbat, Adriana Romero Soriano, Pascal Vincent, Erich Owens, Joe Katsnelson, Hersh Chandarana, Yvonne Lui, Daniel Sodickson, Larry Zitnick, Michael Recht
Despite the substantial increase in research activity in machine learning for MR image reconstruction, no large scale raw k-space data set is publicly available. This makes it challenging to reproduce and validate comparisons of different approaches, and it restricts access to work on this problem to researchers associated with large academic medical centers. This abstract introduces the first large-scale database of MRI data for reconstruction. The database currently includes about 7500 raw MRI k-space data sets from a range of MRI systems and clinical patient populations, with corresponding images derived from the rawdata using reference image reconstruction algorithms. Approximately 30000 additional clinical image datasets not directly associated with the rawdata are also included, and we plan to add to the database over time.
|
15:57
|
0658.
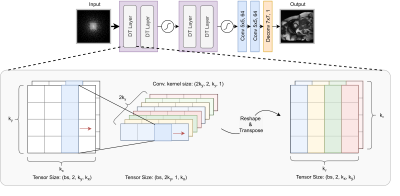 |
dAUTOMAP: Decomposing AUTOMAP to Achieve Scalability and Enhance Performance

Jo Schlemper, Ilkay Oksuz, James Clough, Jinming Duan, Andrew King, Julia Schnabel, Joseph Hajnal, Daniel Rueckert
AUTOMAP is a promising generalized reconstruction approach, however, it is not scalable and hence the practicality is limited. We present a novel way for decomposing the domain transformation, which makes the model scale linearly with the input size. We show the proposed method, termed dAUTOMAP, outperforms AUTOMAP with significantly fewer parameters.
|
16:09
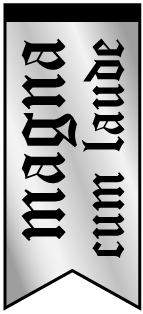 |
0659.
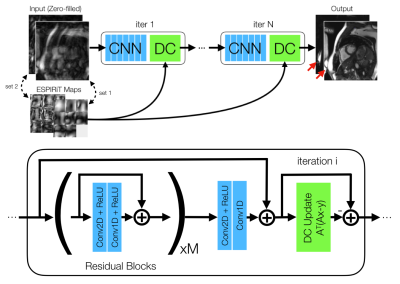 |
DL-ESPIRiT: Improving robustness to SENSE model errors in deep learning-based reconstruction

Christopher Sandino, Peng Lai, Shreyas Vasanawala, Joseph Cheng
Parallel imaging concepts, such as sensitivity encoding (SENSE), have been incorporated into DL reconstruction frameworks by augmenting the acquisition model with knowledge of the coil sensitivities. However, SENSE-based methods rely on accurate estimation of sensitivity maps; otherwise, residual aliasing may arise due to model errors such as in reduced field-of-view imaging. Here we propose DL-ESPIRiT, an ESPIRiT-based neural network architecture with improved robustness to model errors. We show that DL-ESPIRiT can reconstruct 10X accelerated 2D cardiac CINE data with higher fidelity and allow for more accurate automatic assessment of cardiovascular function than l1-ESPIRiT.
|
16:21
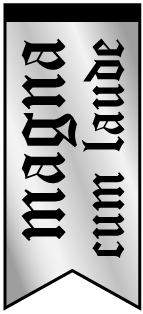 |
0660.
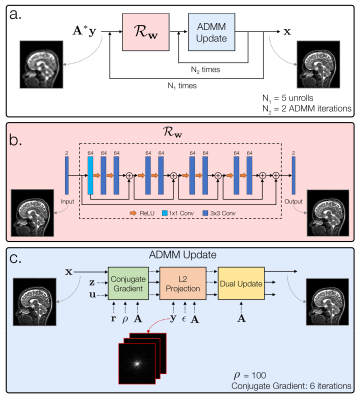 |
Unsupervised Deep Basis Pursuit: Learning Reconstruction without Ground-Truth Data

Jonathan Tamir, Stella Yu, Michael Lustig
Basis pursuit is a compressed sensing optimization in which the l1-norm is minimized subject to model error constraints. Here we use a deep neural network prior instead of l1-regularization. Using known noise statistics, we jointly learn the prior and reconstruct images without access to ground-truth data. During training, we use alternating minimization across an unrolled iterative network and jointly solve for the neural network weights and training set image reconstructions. At inference, we fix the weights and pass the measurements through the network. We compare reconstruction performance between unsupervised and supervised (i.e. with ground-truth) methods. We hypothesize this technique could be used to learn reconstruction when ground-truth data are unavailable, such as in high-resolution dynamic MRI.
|
16:33
|
0661
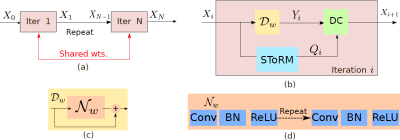 |
Dynamic MRI using Model-based deep learning and SToRM priors: MoDL-SToRM Video Permission Withheld
Sampurna Biswas, Hemant Aggarwal, Mathews Jacob
We introduce a novel framework to combine deep-learned priors along with complementary image regularization penalties to reconstruct free breathing & ungated cardiac MRI data from highly undersampled multi-channel measurements. Image recovery is formulated as an optimization problem, where the cost function is the sum of data consistency term, convolutional neural network (CNN) denoising prior, and SmooThness regularization on manifolds (SToRM) prior that exploits the manifold structure of images in the dataset. Our results show the benefit in combining deep learned CNN priors with complementary image regularization penalties.
|
16:45
|
0662.
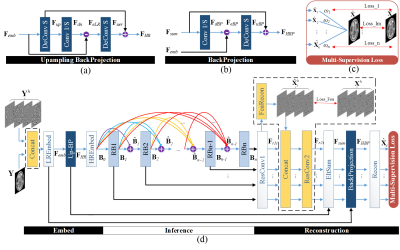 |
Undersampled MR Image Reconstruction Using an Enhanced Recursive Residual Network

Lijun Bao, Fuze Ye
We propose an enhanced recursive residual network (ERRN) that improves the basic recursive residual network with both a high-frequency feature guidance and dense connections. The feature guidance is designed to predict the underlying anatomy based on image a priori learning from the label data, playing a complementary role to the residual learning. The ERRN is adapted to include super resolution MRI and compressed sensing MRI, while an application-specific error-correction unit is added into the framework, i.e. back projection for SR-MRI and data consistency for CS-MRI due to their different sampling schemes.
|
16:57
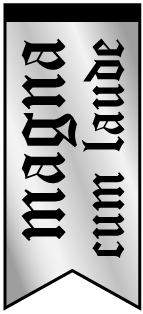 |
0663.
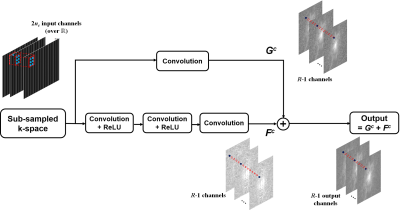 |
Accelerated MRI Using Residual RAKI: Scan-specific Learning of Reconstruction Artifacts

Chi Zhang, Steen Moeller, Sebastian Weingärtner, Kâmil Ugurbil, Mehmet Akçakaya
Recently, there has been an interest in machine learning reconstruction techniques for accelerated MRI, where the focus has been on training regularizers on large databases. Another line of work, called Robust Artificial-neural-networks for k-space Interpolation (RAKI) explored the use of CNNs, trained on subject-specific ACS data for improving parallel imaging. In this work, we propose a ResNet architecture, called Residual RAKI (rRAKI) for training a subject-specific CNN that simultaneously approximates a linear convolutional operator and a nonlinear component that compensates for noise amplification artifacts that arise from coil geometry. Brain data shows improved noise resilience at high acceleration rates.
|
17:09
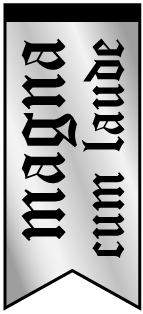 |
0664.
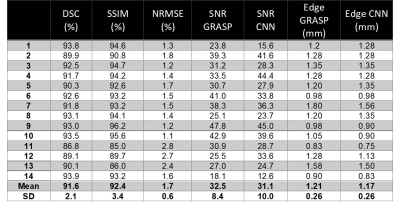 |
Rapid Reconstruction of Accelerated, Free-Breathing, Thoracic, Non-Contrast Magnetic Resonance Angiography using Convolutional Neural Network

Hassan Haji-valizadeh, Daming Shen, Florian Schiffers, Oliver Schiffers, Daniel Kim
In this study we developed a convolutional neural network (CNN) for reconstructing 3D non-contrast magnetic resonance angiography (NC-MRA) images. We trained our proposed CNN using 4,800 zero-filled images and the corresponding GRASP reconstructed images from 10 patients as input and output, respectively. For validation, we used 6,720 zero-filled images from 14 patients as input to our trained CNN. Comparison between CNN and GRASP reconstructions showed excellent agreement using quantitative metrics and quantified aortic diameters . The mean reconstruction time, excluding the pre- and post-processing steps, for CNN (74 s) was 99% shorter than GRASP (12,703 s).
|
17:21
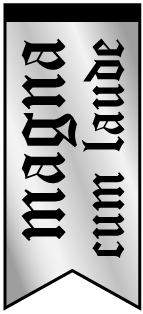 |
0665.
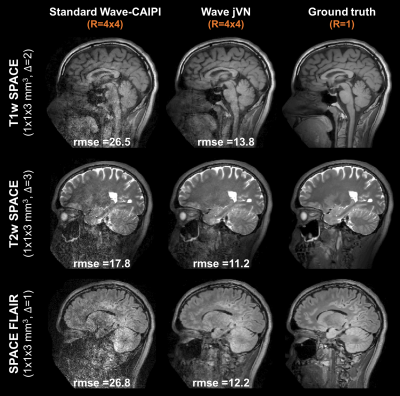 |
Joint multi-contrast Variational Network reconstruction (jVN) with application to Wave-CAIPI acquisition for rapid imaging

Daniel Polak, Stephen Cauley, Berkin Bilgic, Esther Raithel, Peter Bachert, Elfar Adalsteinsson, Kawin Setsompop
We introduce a joint Variational Network (jVN) to reconstruct multi-contrast data jointly from accelerated MRI acquisitions. By taking advantage of the shared structural information among different clinical contrasts, jVN better preserved small anatomical features when compared to standard single-contrast VN. Combining jVN with the efficient Wave-CAIPI acquisition scheme enabled rapid 3D volumetric scans at R=16x acceleration. This approach was evaluated at 3T using in-vivo data from three clinical contrasts, resulting in up to a 54% reduction in RMSE when compared to standard Wave-CAIPI reconstructions. The jVN reconstructions preserved both high spatial resolution and good image quality.
|
17:33
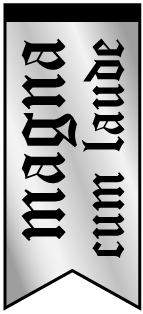 |
0666.
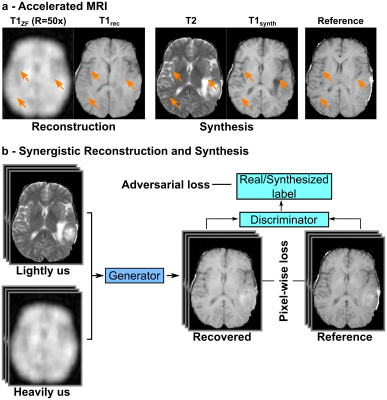 |
Joint recovery of variably accelerated multi-contrast MRI acquisitions via generative adversarial networks

Salman Ul Hassan Dar, Mahmut Yurt, Mohammad Shahdloo, Muhammed Emrullah Ildiz, Tolga Çukur
Two frameworks to recover missing data in accelerated MRI are reconstruction of undersampled acquisitions and synthesis of missing acquisitions. In reconstruction, performance diminishes towards higher acceleration factors and in synthesis, lack of evidence regarding the target contrast can lead to artefactual sensitivity or insensitivity to image features. To address these issues, we propose an approach that synergistically performs reconstruction and synthesis of multi-contrast MRI using generative adversarial networks. Demonstrations on brain MRI datasets from healthy subjects and patients indicate that the proposed method preserves intermediate spatial frequency details and prevents artefactual feature synthesis or feature loss as compared to previous state-of-the-art methods.
|
|