Diffusion MRI: Signal Representation & Modelling
Oral
Diffusion
Tuesday, 14 May 2019
Room 710B |
13:30 - 15:30 |
Moderators: Corey Baron, Rafael Neto Henriques |
13:30
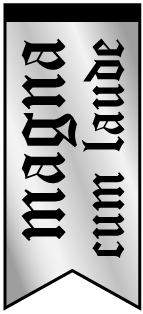 |
0550.
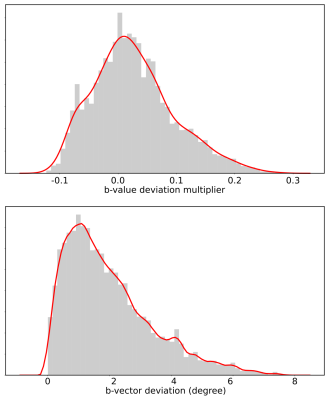 |
Gradient Non-Linearity Correction for Spherical Mean Diffusion Imaging

Michael Paquette, Cornelius Eichner, Alfred Anwander
Gradient non-linearities are a significant source of errors in MRI systems with strong gradients. In the case of diffusion imaging, they induce spatial deviation of the b-vectors. The spherical mean methods in diffusion relies on the acquisition of spherical b-shell. To recover accurate spherical mean values, it is necessary to undistort the diffusion signal. Therefore, we evaluated three correction methods for gradient non-linearities using the Connectom gradient system as a showcase. We show how a simple heuristic can reduce the spherical mean errors by 20 folds.
|
13:42
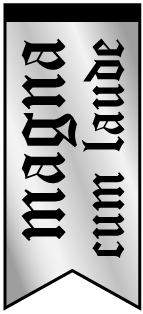 |
0551.
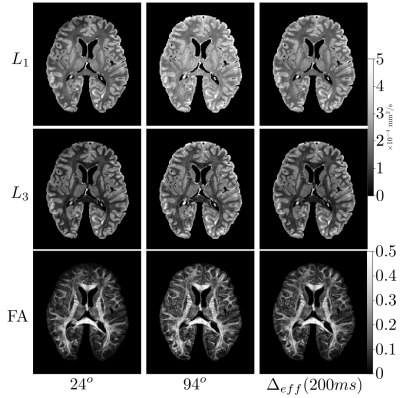 |
Disentangling diffusion-weighted SSFP: ADC estimates in terms of an effective diffusion time

Benjamin Tendler, Saad Jbabdi, Sean Foxley, Menuka Pallebage-Gamarallage, Moises Hernandez-Fernandes, Martin Turner, Olaf Ansorge, Karla Miller
Diffusion-weighted SSFP (dwSSFP) is a high-SNR-efficiency diffusion imaging method. Unlike conventional diffusion measurements, the dwSSFP signal reflects a range of diffusion times because the signal is recycled over multiple excitations. This complicates interpretation and leads to an ill-defined b-value. We present a framework to describe dwSSFP-derived ADC estimates in terms of an effective diffusion time. To achieve this, we require dwSSFP measurements at two flip-angles. Experimental results are presented in a whole, postmortem brain at 7T. This enables us to simultaneously addresses flip-angle inhomogeneity at 7T and provide ADC estimates that are more comparable to conventional diffusion MRI.
|
13:54
|
0552.
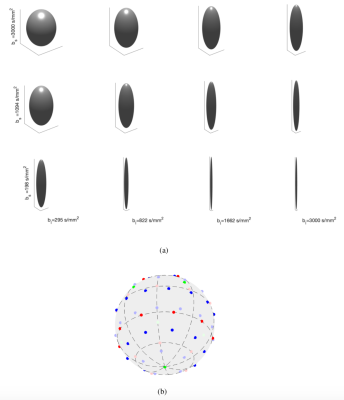 |
Multi-Dimensional Diffusion MRI Sampling Scheme: B-tensor Design and Accurate Signal Reconstruction

Alice Bates, Alessandro Daducci, Emmanuel Caruyer
b-tensor encoding enables the separation of isotropic and anisotropic tensors. However, little consideration has been given as to how to design a b-tensor encoding sampling scheme. In this work, we propose the first 4D basis for representing the diffusion signal acquired with b-tensor encoding. We study the properties of the diffusion signal in this basis to give recommendations for optimally sampling the space of axisymmetric b-tensors. We show, using simulations, that the proposed sampling scheme enables accurate reconstruction of the diffusion signal by expansion in this basis using a clinically feasible number of samples.
|
14:06
|
0553.
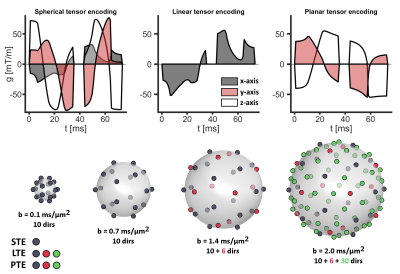 |
Technical validation of b-tensor encoding shows the need for standardized acquisition protocols

Patrik Brynolfsson, Markus Nilsson, Lars Olsson, Carl-Fredrik Westin, Filip Szczepankiewicz
Tensor-valued diffusion encoding can be used to separate effects of microscopic anisotropy, orientation dispersion, and isotropic kurtosis. The aim of this work was to determine the impact of encoding tensor shapes and sizes (b-values) on the estimated model parameters using in-vivo data and simulated signals from several microdiffusion environments. We found that some signal sampling protocol had a relevant impact on the estimated diffusion parameters and may negatively impact the parameter reproducibility. This demonstrates the need for a standardization of sampling schemes to facilitate study comparisons, data pooling, and meta analysis.
|
14:18
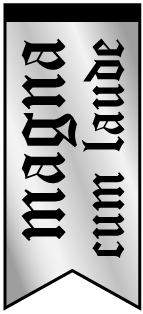 |
0554.
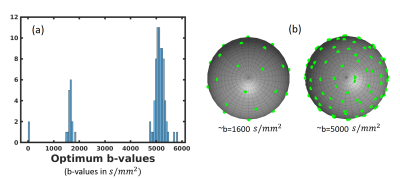 |
Learning-Based Jointly-Optimal Design of the Diffusion Encoding Scheme and Orientation Estimation Method for Diffusion MRI

Divya Varadarajan, Justin Haldar
Diffusion MRI is powerful but limited by long scan times. When optimizing diffusion MRI, most previous methods have either optimized the encoding scheme (i.e., q-space samples) or have optimized the parameter estimation method. In this work, we propose and evaluate a novel approach that jointly optimizes both the encoding scheme and the estimation scheme. This is enabled by combining linear estimation theory with machine learning techniques. Our results show the strong potential of our new approach. Perhaps surprisingly and in contrast to conventional wisdom, we observe that a two-shell sampling scheme appears to be preferred for orientation estimation.
|
14:30
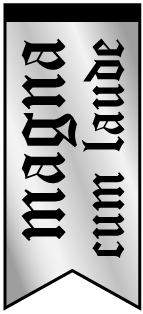 |
0555.
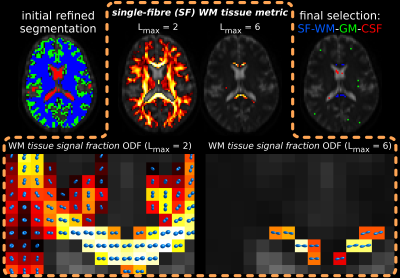 |
Improved white matter response function estimation for 3-tissue constrained spherical deconvolution

Thijs Dhollander, Remika Mito, David Raffelt, Alan Connelly
Multi-shell multi-tissue constrained spherical deconvolution (MSMT-CSD) and single-shell 3-tissue CSD (SS3T-CSD) decompose the diffusion MRI signal in a white matter (WM) fibre orientation distribution (FOD) and grey matter (GM) and cerebrospinal fluid (CSF) compartments. An unsupervised method was recently proposed to estimate the required WM/GM/CSF response functions. In this work, we improved WM response function estimation by leveraging WM properties across b-values, resulting in better 3-tissue CSD fit to most data. Slightly beyond the scope of this work, we also make an interesting observation in developing Human Connectome Project data.
|
14:42
|
0556.
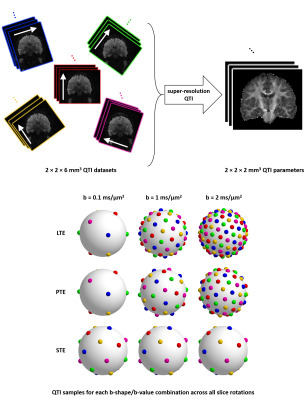 |
Improved precision and accuracy in q-space trajectory imaging by model-based super-resolution reconstruction

Ben Jeurissen, Carl-Fredrik Westin, Jan Sijbers, Filip Szczepankiewicz
Q-space trajectory imaging (QTI) facilitates tensor-valued diffusion encoding with variable shapes and provides more specific parameters than those available from conventional stick-shaped encoding. However, it generally requires longer echo times than conventional encoding, impacting the spatial resolution, scan time or signal-to-noise ratio. In this work, we propose a super-resolution acquisition and reconstruction approach for QTI that allows high-resolution parameter maps to be estimated from multiple low-resolution images. Using simulations and real data, we show that this does not only improve QTI’s precision, it also significantly improves its accuracy, as it avoids deleterious signal bias caused by the noise floor.
|
14:54
|
0557.
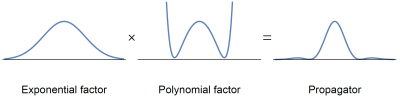 |
Non-negative mean apparent propagators using sum-of-squares optimization: MAP+

Tom Dela Haije, Evren Özarslan, Aasa Feragen
Mean apparent propagator (MAP) MRI provides an efficient representation of the three-dimensional q-space signal that is used to characterize the diffusional characteristics of the tissue. The standard implementation of MAP-MRI imposes soft constraints, leaving the possibility for negative values of the propagator; we observed that such physically unjustifiable reconstructions are widespread throughout the brain. Here, we introduce a new framework based on sum-of-squares optimization that guarantees the non-negativity of the reconstructed propagator, thus yielding improved estimates of the propagator as well as the scalar measures derived from it.
|
15:06
|
0558.
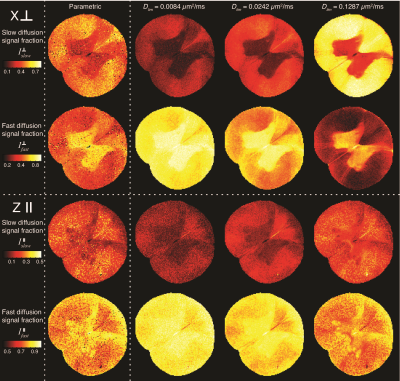 |
Water mobility spectral imaging of the spinal cord: parametrization of model-free Laplace MRI

Dan Benjamini, Peter Basser
Diffusion magnetic resonance imaging of biological systems most often results in non-monoexponential signal, due to their complexity and heterogeneity. One approach to interpreting the data without imposing microstructural models is to fit it to a multiexponential function, and to display the coefficients as a distribution of the diffusivities. Here we suggest parameterizing the measured water mobility spectra using a bimodal lognormal function. This approach allows for a compact representation of the spectrum, while also resolving overlapping spectral peaks. We apply the method on a spinal cord sample and use it to generate robust intensity images of slow and fast-diffusion components.
|
15:18
|
0559.
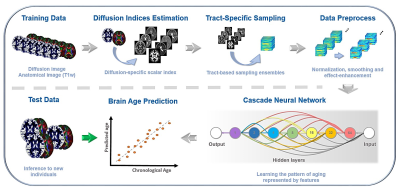 |
Generalize diffusion-MRI-based brain age predictive model using transfer learning

Chang-Le Chen, Yung-Chin Hsu, Wen-Yih Tseng
Heterogeneity of diffusion MRI data limits the diffusion-MRI-based machine learning model to be generalized to the data acquired at other sites. To generalize the brain age model based on diffusion-MRI-derived features, we used transfer learning techniques to transfer the pre-trained model from the source domain to the target domain with a few tuning data. We found that 75 tuning data with transfer learning framework achieved the acceptable performance, and 150 tuning data achieved the performance comparable to the maximum samples in the target domain. This study provides a practical solution to solve the limitation of diffusion-MRI-based model using transfer learning.
|
|