2nd Hour
Poster: Artificial Intellience Neuro
Power Pitch Poster
Neuro
Monday, 13 May 2019
Power Pitch Theater B - Exhibition Hall
09:15 - 10:15
|
|
Plasma # |
|
0016.
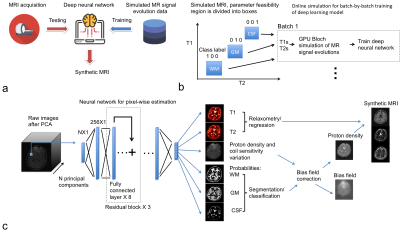 |
16 |
Synthetic MRI through a Deep Neural Network Based Relaxometry and Segmentation

Peng Cao, Jing liu, Shuyu Tang, Andrew Leynes, Peder Larson
This study demonstrated a method for 3D synthetic MRI through a deep neural network Based Relaxometry and Segmentation. Ranges of T1 and T2 values for gray matter, white matter and cerebrospinal fluid (CSF) were used as the prior knowledge. The proposed method can directly generate brain T1 and T2 maps in conjunction with segmentation based bias field correction and synthetic MRI.
|
|
0017.
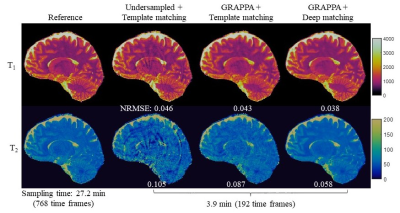 |
17 |
High-resolution 3D MR Fingerprinting using parallel imaging and deep learning

Yong Chen, Zhenghan Fang, Dinggang Shen, Weili Lin
In this study, a high-resolution 3D MR Fingerprinting technique, combining parallel imaging and deep learning, was developed for rapid and simultaneous quantification of T1 and T2relaxation times. Our preliminary results show that, with the integration of parallel imaging and deep learning techniques, whole-brain quantitative T1 and T2 mapping with 1-mm isotropic resolution can be achieved in ~6 min, which is feasible for routine clinical practice.
|
|
0018.
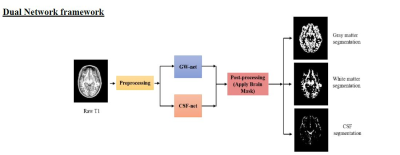 |
18 |
AUTOMATED BRAIN TISSUE SEGMENTATION USING DEEP LEARNING AND IMPERFECT LABELING

Chandan Ganesh Bangalore Yogananda, Benjamin Wagner, Gowtham Murugesan, Sahil Nalawade, Ananth Madhurantakam, Joseph Maldjian
This work presents a deep learning pipeline to perform brain tissue segmentation on T1w Magnetic Resonance images (MRI). Two separate 3D-Dense-Unets were designed: GW-net to segment the gray matter (GM) and white matter (WM) and CSF-net to segment the cerebrospinal fluid (CSF). The network was trained on T1w MRI from 785 datasets in the iTAKL study with their corresponding SPM12 segmentations as ground truth and tested on 50 held-out subjects from the iTAKL study, 50 subjects from the AADHS study and 131 subjects from the Human Connectome project (HCP). Our pipeline showed improved segmentations when tested on simulated data with known ground truth as compared to the existing neuroimaging packages including SPM12, FSL and CAT12.
|
|
0019.
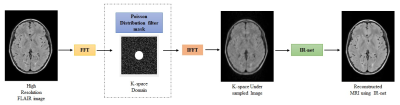 |
19 |
MR IMAGE RECONSTRUCTION FROM UNDERSAMPLED k-SPACE USING DEEP LEARNING

Chandan Ganesh Bangalore Yogananda, Sahil Nalawade, Gowtham Murugesan, Benjamin Wagner, Ananth Madhurantakam, Joseph Maldjian
This work presents a deep learning approach to reconstruct MR images from undersampled k-space on 3D-FLAIR MR images. IR-net, a patch based 3D-Dense U-net, was designed to achieve this. 600 [JM1] [CGBY2] 3D-FLAIR MR images were used for training and testing. Aliased images were created by undersampling the high resolution 3D-FLAIR images in k-space using a Poisson distribution filter. The network was trained on patches from 550 aliased k-space data with their corresponding high resolution 3D-FLAIR MR images as ground truth and 50 images were held out for testing. IR-net successfully reconstructed the aliased images with significant improvement in SSIM and PSNR. [JM1]Are these 600 image slices, or 600 3D image volumes? [CGBY2]600 3D images.
|
|
0020.
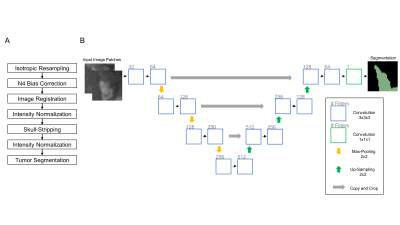 |
20 |
Fully-Automated Glioma Volumetric Segmentation and Treatment Response Assessment in MRI using Deep Learning

Ken Chang, Andrew Beers, Harrison Bai, James Brown, K Ly, Xuejun Li, Joeky Senders, Vasileios Kavouridis, Alessandro Boaro, Chang Su, Ena Agbodza, Wenya Bi, Otto Rapalino, Weihua Liao, Qin Shen, Hao Zhou, Bo Xiao, Yinyan Wang, Paul Zhang, Marco Pinho, Patrick Wen, Tracy Batchelor, Omar Arnaout, Bruce Rosen, Elizabeth Gerstner, Li Yang, Raymond Huang, Jayashree Kalpathy-Cramer
Longitudinal assessment of glioma burden is important for evaluating treatment response and tumor progression. Delineation of tumor regions is typically performed manually but is time-consuming and subject to inter-rater and intra-rater variability. Therefore, there has been interest in developing automated approaches to calculate 1) glioma volume and 2) the product of maximum diameters of contrast-enhancing tumor (the key measure used in the Response Assessment for Neuro-Oncology (RANO) criteria). We present a fully automated pipeline for brain extraction, tumor segmentation, and RANO measurement (AutoRANO). We show the utility of this pipeline on 713 MRI scans from 54 post-operative glioblastoma patients, demonstrating capacity for tumor burden measurement.
|
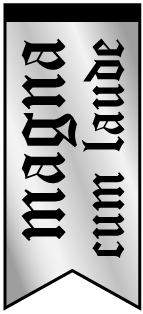 |
0021
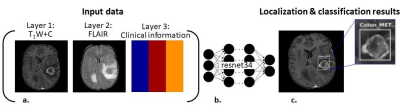 |
21 |
Automatic detection and classification of brain tumors using deep learning and based on conventional MRI and clinical information

Moran Artzi, Moshe Yerachmiel, Snir Shalom, Dafna Ben Bashat
Automatic detection and classification of brain tumors was performed using deep learning based on conventional MRI and clinical information. A total of 441 patients where included: 202 patients with high grade glioma and 239 patients with brain metastases. Classification was performed using resnet34 architecture. The input data for classification were FLAIR images, post contrast T1W images and patients’ clinical information. Classification results showed high accuracy=89%, specificity=91% and sensitivity=86%. For lesion localization the mean intersection over union (IoU) score was 0.64±0.17. Our results indicate the promising potential of a deep learning approach for automatic non-invasive diagnosis of patients with brain tumors.
|
|
0022.
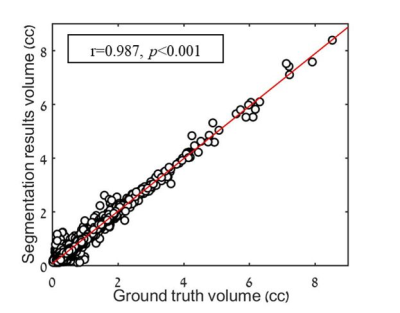 |
22 |
Automatic segmentation and follow-up of optic pathway gliomas using deep learning and based on conventional MRI

Moran Artzi, Sapir Gershov, Idan Bressler, Liat Ben-Sira, Shlomi Constantini, Tomer Gazit, Tali Halag-Milo, Dafna Ben Bashat
Optic pathway gliomas (OPG) are heterogeneous tumors with complex shape. The aim of this study was to implement a deep-learning approach for automatic segmentation and follow-up of patients with OPG based on conventional MRI. A total of 354 MRI scans from 53 patients where included. A neural-network with a U-net architecture was trained for segmentation of lesion area. The similarity coefficient score between segmentation results and ground truth was 0.812±0.159, with sensitivity=0.799±0.188, specificity=0.999±0.002 and correlation of r=0.987 (p<0.001) between lesion volumes. These results demonstrate the potential applicability of the proposed method for automatic radiological follow-up of patients with OPG.
|
|
0023.
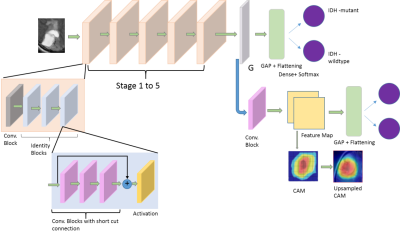 |
23 |
Predictive and discriminative localization of IDH genotype in high grade gliomas using deep convolutional neural nets

Adnan Ahmad, Srinjay Sarkar, Apurva Shah, Santosh Vani, Jitender Saini, Madhura Ingalhalikar
Radiomics and state-of-art convolutional neural networks (CNNs) have demonstrated their usefulness for predicting genotype in gliomas from brain MRI. However, these techniques rely on accurate tumor segmentation and do not facilitate insights into the critical discrimative features. To mitigate this, we employ a novel technique called CNNs with discriminative localization (DL-CNN) on a clinical T2 weighted MRI dataset of IDH1 mutant and wild-type tumor patients, which is not only free of tumor segmentation with high classification accuracy of 86.7% but also demonstrates that the tumoral area is discriminative in mutants while in IDH1 wildtype the peri-tumoral edema is also involved.
|
|
0024.
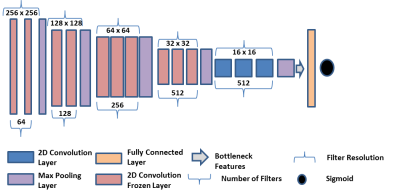 |
24 |
Deep Learning for Identification of Active Lesions in Multiple Sclerosis Without Administration of Gadolinium Based Contrast Agent

Ponnada Narayana, Ivan Coronado, Sushmita Datta, Sheeba Sujit, Fred Lublin, Jerry Wolinsky, Refaat Gabr
Gadolinium based contrast agents (GBCA) are routinely administered for identifying active lesions in multiple sclerosis (MS). Because of the safety concerns with GBCA, alternative methods are highly desirable to identify active lesions without GBCA. We used Deep Learning, specifically multi-layered VGG16 network, to identify active MS lesions on the pre-contrast images. The network was trained using a large number of annotated multi-modal magnetic resonance image volumes (792) acquired as a part of phase 3 clinical. The DL results look quite promising as judged by the accuracy, sensitivity, and specificity of 0.729, 0.861, 0.598, respectively.
|
|
0025.
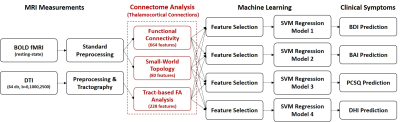 |
25 |
Thalamic Connectome Based Machine Learning for Predicting Individual Symptoms after Mild Traumatic Brain Injury

Chia-Feng Lu, Yu-Chieh Kao, Li-Chun Hsieh, Sho-Jen Cheng, Nai-Chi Chen, Cheng-Yu Chen
Mild traumatic brain injury (mTBI) can cause persistent post-concussion symptoms in 15-20% patients, however the presented type and severity of symptoms differ largely between patients. This study recruited 53 mTBI patients and 44 healthy controls to demonstrate the feasibility in using the imaging features of thalamic connectome combined with machine-learning regression models for the individualized prediction of clinical symptoms.
|
|
0026.
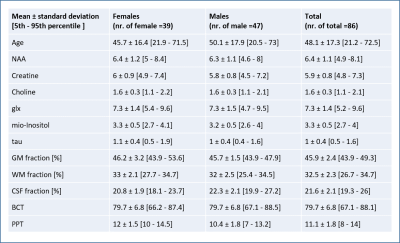 |
26 |
A Machine Learning approach to Predict Age-Related Motor Performance using MRI-MRS data

Akila Weerasekera, Adrian Ion-Margineanu, Oron Levin, Diana Sima, Sabine Van Huffel, Stephan Swinnen, Uwe Himmelreich
Aging is associated with gradual alterations in structural and neurochemical characteristics of the brain, which can be assessed in vivo by MRI and MRS modalities. The process of brain aging occurs in accord with a general decline in cognitive-motor performance and increases the risk of neurodegeneration. We used MRI-MRS data from 86 individuals as inputs for machine learning models5 to predict motor performance in healthy individuals. Our analysis shows that application of machine learning algorithms on combination of age, gender and MR data can accurately predict motor performance and has potential to be used as a biomarker for neuro related diseases.
|
|
0027.
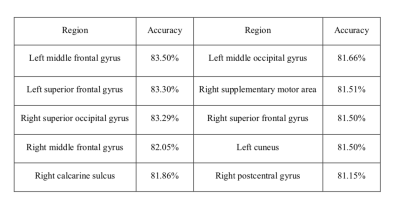 |
27 |
A deep learning framework with redundancy removal and its diagnostic performance of Parkinson's disease

Fan Huang, Mingyi Zhou, Shi-ming Wang, Jing Wu, Liaqat Ali, Yi-Hsin Weng, Yao-Liang Chen, Jiun-Jie Wang, Yipeng Liu
Computer-aided diagnosis using deep learning methods shows its potential in medical images classifications. This study aims to examine the diagnostic performance of diffusion tensor imaging using a 4-steps framework for deep learning to differentially diagnose patients with Parkinson's disease(PD) and normal controls(NC).
|
|
0028.
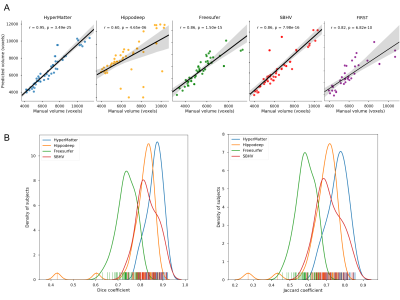 |
28 |
Hippocampal segmentation for brains with extensive atrophy using three-dimensional convolutional neural networks

Maged Goubran, Edward Ntiri, Hassan Akhavein, Melissa Holmes, Sean Nestor, Ramirez Joel, Sabrina Adamo, Fuqiang Gao, Christopher Scott, Anne Martel, Walter Swardfager, Mario Masellis, Rick Swartz, Bradley MacIntosh, Sandra Black
Obtaining hippocampal volumes through manual segmentation requires an expert and is time consuming. Automated segmentation techniques would benefit from user-friendly and publicly accessible to tools, and robust results in the face of brain diseases. To accomplish these objectives, we trained a 3D convolutional neural network to segment the hippocampus automatically. Our algorithm was more accurate and time efficient compared to 4 publicly available state-of-the-art methods when considering a wide range of patient groups. Thus, we present a new method for obtaining hippocampal volumes, an important biomarker in aging, disease, and dementia.
|
|
0029.
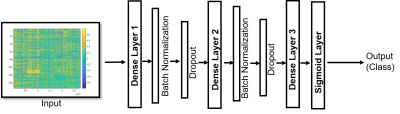 |
29 |
Functional MRI-based Deep Learning Classification between Temporal Lobe Epilepsy Patients and Healthy Controls

Maribel Torres-Velázquez, Gyujoon Hwang, Cole Cook, Bruce Hermann, Jeffery Binder, M. Elizabeth Meyerand, Alan McMillan
Common machine learning approaches to differentiate between Temporal Lobe Epilepsy (TLE) and healthy controls often include extensive preprocessing techniques that often entail feature extraction, resulting in a more time-intensive and variable approach. Utilizing data from both the Epilepsy Connectome Project (ECP) and Human Connectome Project (HCP), this study attempts to develop, train, and validate a deep learning classifier to automatically differentiate between TLE patients and healthy subjects using resting-state fMRI (rs-fMRI) and task fMRI (t-fMRI) data alone without advanced preprocessing steps or feature extraction.
|
|
0030.
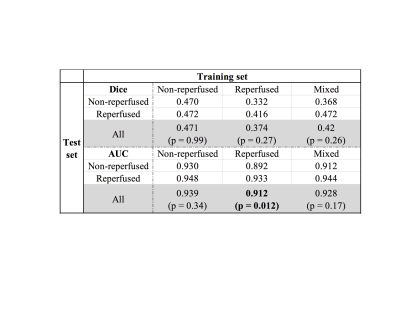 |
30 |
Using convolutional neural networks to predict infarct development in acute ischemic stroke patients: does reperfusion status matter?

Yuan Xie, Yannan Yu, Thoralf Thamm, Charles Huang, Enhao Gong, Soren Christensen, Maarten Lansberg, Greg Zaharchuk
Convolutional Neural Network has shown promising results in stroke treatment outcome predictions. Our study explores the hypothesis of whether training a CNN model with patients who have similar treatment outcomes can improve the model prediction of day 5 stroke lesion.
|
|