2nd Hour
Poster: Machine Learning Unleashed 1
Power Pitch Poster
Acquisition, Reconstruction & Analysis
Tuesday, 14 May 2019
Power Pitch Theater B - Exhibition Hall
14:30 - 15:30
|
|
Plasma # |
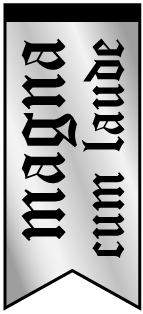 |
0465.
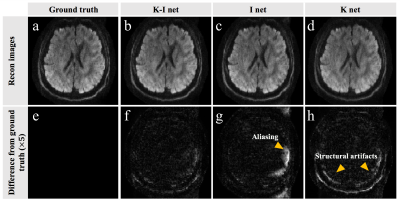 |
16 |
Reconstruction of multi-shot diffusion-weighted MRI using unrolled network with U-nets as priors

Yuxin Hu, Xinwei Shi, Qiyuan Tian, Hengkai Guo, Minda Deng, Miao Yu, Catherine Moran, Grant Yang, Jennifer McNab, Bruce Daniel, Brian Hargreaves
In this work, we demonstrated the feasibility of using deep neural networks for rapid multi-shot DWI reconstruction. An unrolled network with six U-nets, which operated in frequency and image domains alternatively, was shown to have the capability to remove aliasing artifacts from shot-to-shot phase variations, and achieved about 60-fold speed up and around 1% amplitude difference compared with conventional iterative reconstruction methods.
|
|
0466.
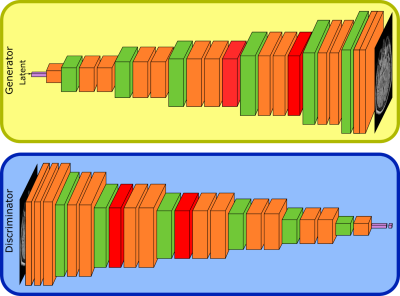 |
17 |
Generation of globally consistent non-confidential MRI data using deep generative adversarial networks

Karim Armanious, Mario Döbler, Bin Yang, Sergios Gatidis
The lack of easily accessible open-source medical datasets is one of the biggest limiting factors to advance the development of deep models for any medical task. In this work, we utilize progressive growing of generative adversarial networks to generate high-resolution, realistic, non-confidential MRI data. Additionally, self-attention is used to model long-range dependencies to improve global consistency of the generated data. For a feasibility study, our models are trained on MRI data of the head region and are evaluated qualitatively and quantitatively. The results illustrate the capability of the proposed model to generate MRI data.
|
|
0467.
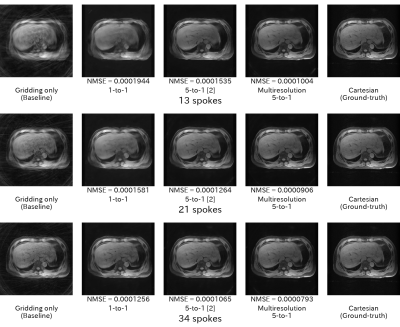 |
18 |
Fast Acquisition and Low-delay Reconstruction of Stack-of-stars Trajectory Using Temporal Multiresolution Images and a Convolutional Neural Network

Hidenori Takeshima, Hideaki Kutsuna
For fast data acquisition and low-delay reconstruction in applications using stack-of-stars trajectory, the authors propose a new reconstruction method using a CNN with temporal multiresolution inputs. Conventionally, stack-of-stars images reconstructed from a few spokes contain streaking artifacts. By utilizing view sharing technique for suppressing the artifacts, reconstructed images are often blurred. For low-delay reconstruction, it is not straightforward to use well-studied methods based on compressed sensing with temporal priors. The proposed method aims to adjust spatio-temporal resolution to a suitable one. Experimental results show that the proposed method could reconstruct highly under-sampled radial dynamic images with reduced artifacts.
|
|
0468.
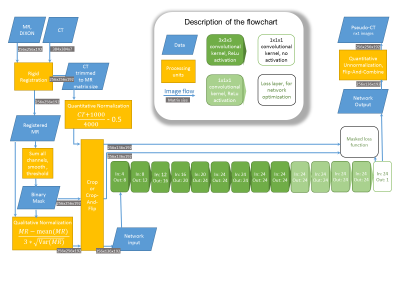 |
19 |
Integrating Imaging Prior Knowledge in Deep Convolutional Network - A Novel Approach of Cranial Pseudo-CT Generation

Max W.K. Law, Gladys Lo, Yihang Zhou, Jing Yuan, Oilei Wong, S.K. Yu
A deep convolutional network for cranial pseudo-CT generation was developed with the consideration of prior knowledge involved in radiotherapetic imaging workflow. This prior knowledge has been scarcely studied along with deep learning. It could greatly reduce the complexity of image data handled by the network. Examined on 14 sets of DIXON-MR and CT images, the proposed model achieved low generalization gap and offered accurate results regardless of the amount of training data. It achieved an average of 89.77±29.32HU mean-absolute-difference in two-fold cross-validation. It is experimentally shown that the proposed method is well-suited for generating clinical pseudo-CT for radiotherapeutic applications.
|
|
0469.
 |
20 |
Exploring Complex-Valued Neural Networks with Trainable Activation Functions for Magnetic Resonance Imaging

Guillaume Daval-Frerot, Xiao Chen, Simon Arberet, Boris Mailhé, Peter Speier, Mathias Nittka, Heiko Meyer, Mariappan Nadar
MR signals by nature are complex valued. However, most of the current deep neural networks for MR are derived from applications dealing with real-valued images. Recent studies proposed an adaptation of neural networks to the complex domain to learn a better representation of the signal. In this study, multiple CVNN with trainable complex-valued activation functions are proposed and validated on MR fingerprinting regression problem. 2D activation functions with trainable parameters have been demonstrated here to suit the CVNN well and provide significant improvement over the non-trainable versions.
|
|
0470.
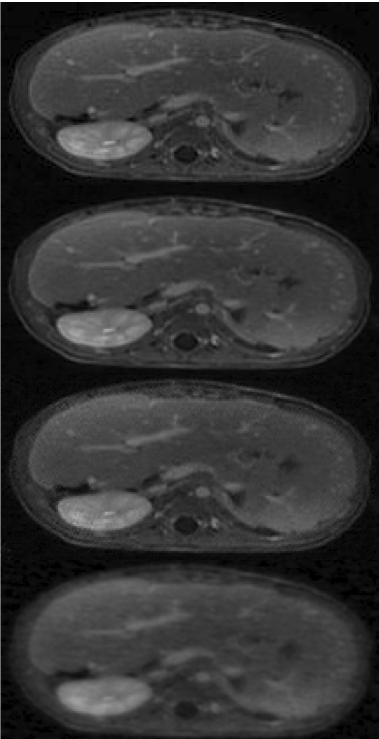 |
21 |
Learning Perceptual Neural Proximals for Robust MRI Recovery

Morteza Mardani, David Donoho, John Pauly, Shreyas Vasanawala
This abstract proposes a framework for robust reconstruction of MR images from highly undersampled measurements. Inspired by proximal gradient descent (PGD) iterations a recurrent neural network is trained to learn a high diagnostic proximal using Wasserstein GANs. For a Knee dataset, the proximal modeled with only two residual blocks shared across 5 iterations not only trains fast but also reveals fine diagnostic details with limited training data. All in all, this study suggests that sharing the proximal weights across iterations regularizes the reconstruction and improves the generalization.
|
|
0471.
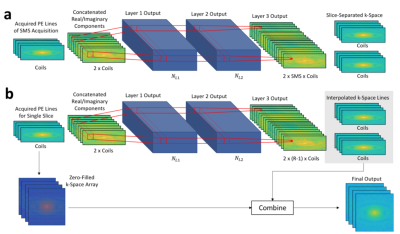 |
22 |
Application of a k-Space Interpolating Artificial Neural Network to In-Plane Accelerated Simultaneous Multislice Imaging for Motion Monitoring

Nikolai Mickevicius, Eric Paulson, Andrew Nencka
A scan-specific deep learning approach to Cartesian k-space interpolation was extended to in-plane accelerated simultaneous multislice imaging. This method yields images more accurate and less noisy reconstructions than conventional SMS parallel imaging algorithms, particularly as acceleration factors approach the number of receive coils. Furthermore, the models trained in one particular motion state are applicable to test data from a different motion state. This suggests that this method will be useful for cine imaging.
|
|
0472.
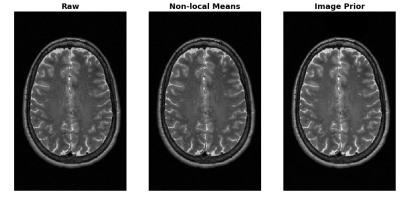 |
23 |
Deep learning without ground truth: an unsupervised method for MR image denoising and super-resolution

Xue Feng, Craig Meyer
Deep learning has shown great success in MR image segmentation, enhancement and reconstruction. However, most methods, if not all, rely on a pair of the input image and the ground-truth image to train the network for a given task. In practice, it is often hard to get the corresponding ground-truth MR images due to limitations in data acquisition. In this study, we aim to use the convolutional neural network (CNN) structure itself as a constraint without using ground-truth images in an optimization task and to evaluate its performance in MR image denoising and super-resolution applications.
|
|
0473.
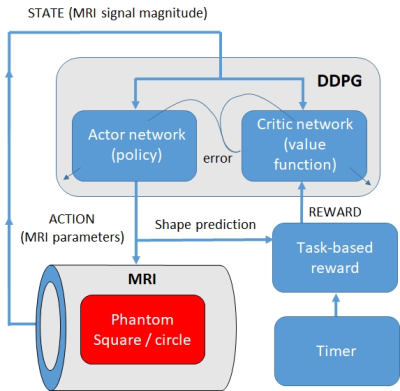 |
24 |
Using deep reinforcement learning to actively, adaptively and autonomously control a simulated MRI scanner

Simon Walker-Samuel
In deep reinforcement learning (DRL), software agents based on deep neural networks are used to explore environments in order to maximise a reward (e.g. score in a video game). Here, DRL was used to control a virtual MRI scanner and actively interpret acquired data. An environment was constructed in which correctly determining the shape of a phantom was rewarded with a high score, and penalised by increasing acquisition time. Following training, the algorithm had learnt to acquire sparse images, assigning TE, TR and flip angles that enabled it to act as an edge detector and deduce shape with 99.8% accuracy.
|
|
0474.
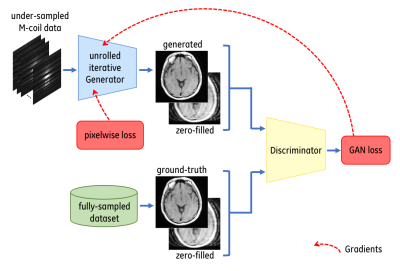 |
25 |
Leveraging conditional GANs with adaptive loss balancing for MRI sparse reconstruction.

Itzik Malkiel, Sangtae ahn, Valentina Taviani, Anne Menini, Zachary Slavens, Christopher Hardy
We propose a Conditional Wasserstein Generative Adversarial Network (cWGAN), trained with a novel Adaptive Loss Balancing (ALB) technique that stabilizes the training and minimizes the presence of artifacts, while maintaining a high-quality reconstruction with more natural appearance (compared to non-GAN techniques). Multi-channel 2D brain data with fourfold undersampling were used as inputs, and the corresponding fully-sampled reconstructed images as references for training. The algorithm produced higher-quality images than state-of-the-art deep learning-based models in terms of perceptual quality and realistic appearance.
|
|
0475.
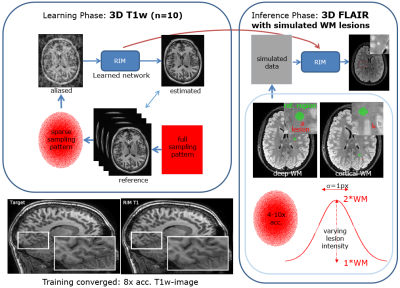 |
26 |
Reconstructing Lesions not seen during training using a Recurrent Inference Machine

Bob Hoek, Kai Lønning, Hanneke Hulst, Frans Vos, Matthan Caan
Deep learning can accelerate MRI beyond what is currently possible. Broad clinical application requires generalizability to multiple contrasts, acceleration levels and pathologies. Here we explore how a Recurrent Inference Machine trained on healthy volunteer T1-weighted brain images performs in such a situation, by reconstructing FLAIR images with white matter lesions, in simulation and prospectively undersampled patient data. Lesion contrast is maintained up to 6x acceleration and higher than in compressed sensing (CS) reconstruction, and all lesions are retained compared to CS.
|
|
0476.
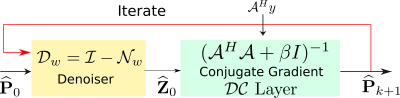 |
27 |
Multi-Shot Diffusion MRI using Deep MUSSELS

Hemant K. Aggarwal, Merry P. Mani, Mathews Jacob
This work proposes a deep-learning based computationally efficient method to reduce phase errors in multi-shot diffusion-weighted images. Recently, we introduced a navigator-free method to reduce these errors using structured low-rank matrix completion based approach termed as MUSSELS. This approach produces state-of-the-art results but is computationally expensive and requires a matrix lifting to estimate filter bank for each direction and slice, independently. We propose a generic deep-learning based approach to estimate phase errors for an unseen dataset by pre-learning the denoiser from exemplary data. The resulting model-based deep learning architecture reduces the computation time by a factor of around 450 with comparable reconstruction quality.
|
|
0477.
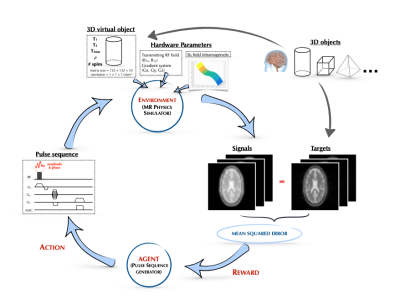 |
28 |
AUTOmated pulse SEQuence generation (AUTOSEQ) for MR spatial encoding in unknown inhomogeneous B0 fields

Bo Zhu, Jeremiah Liu, Neha Koonjoo, Bruce Rosen, Matthew Rosen
The equations of motion that govern nuclear magnetic resonance lead to an incredible variety of MRI contrast mechanisms and spatial encoding schemes to be accessed via the application of cleverly constructed sequences of applied magnetic fields. However, the full potential of the Bloch equations has been difficult to exploit due to their non-intuitive, nonlinear dynamics which can devolve into chaotic behaviors and otherwise have intractable, non-analytical solutions1. Our previous work4 introduced a model-free reinforcement learning approach to pulse sequence generation, with an AI agent that explores an unknown MR imaging environment with pulse sequence “actions,” and constructs a model through corresponding RF receive-signal “rewards.” In this work, we demonstrate the same AI agent learning to generate optimal RF waveforms to perform slice selection in unknown inhomogeneous B0 settings.
|
|
0478.
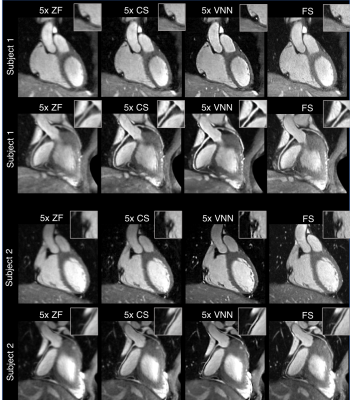 |
29 |
A Variational Neural Network for Accelerating Free-breathing Whole-Heart Coronary MR Angiography

Niccolo Fuin, Aurelien Bustin, Thomas Kuestner, René Botnar, Claudia Prieto
3D whole-heart coronary MR angiography (CMRA) has shown great potential to visualize the coronary arteries. However, scan times remain lengthy as a large amount of data needs to be acquired to obtain high-resolution images. Several undersampled compressed-sensing (CS) reconstruction approaches have been applied to accelerate CMRA. However, CS-based techniques suffer from residual aliasing artifacts, high-dependency on regularization parameters and long reconstruction times. We propose a Variational Neural Network for fast reconstruction of undersampled motion-compensated 3D cardiac MRI, which combined with 100% respiratory efficiency, enables the acquisition of high-quality isotropic CMRA images in ~2-4 minutes, and their reconstruction in ~20 seconds.
|
|
0479.
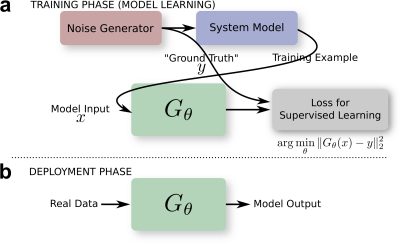 |
30 |
NoiseFlow: Deep Learning using Noise-Driven Training

Joseph Cheng, David Zeng, John Pauly, Shreyas Vasanawala, Bob Hu
Deep learning provides a powerful data-driven solution to a wide range of imaging tasks, from data acquisition to image interpretation. To train these deep and highly nonlinear models, a well labeled and very large dataset is typically required. However, data with accurate labels are difficult, sometimes impossible, and expensive to collect. Without enough data, the learned model will be highly biased and unable to generalize. In the worst case, the application of the deep model may result in misdiagnosis and improper patient management. Thus, we propose NoiseFlow, a solution to reduce the dependency of deep learning solutions on real data through noise-driven training.
|
|