2nd Hour
Poster: Machine Learning Unleashed 2
Power Pitch Poster
Acquisition, Reconstruction & Analysis
Wednesday, 15 May 2019
Power Pitch Theater A - Exhibition Hall
09:15 - 10:15
|
|
Plasma # |
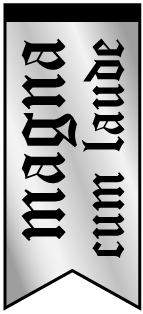 |
0667.
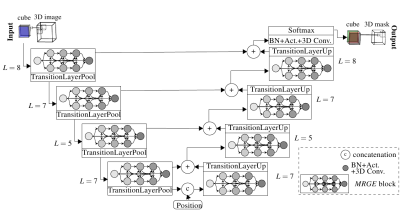 |
1 |
Automated whole-body adipose tissue segmentation in T1-weighted fast spin echo imaging in a cohort of subjects at increased risk for type 2 diabetes

Thomas Kuestner, Martin Schwartz, Yipin Zhu, Petros Martirosian, Bin Yang, Sergios Gatidis, Jürgen Machann, Fritz Schick
Quantification and localization of adipose tissues in whole-body T1-weighted MR images is of high interest to examine metabolic conditions. For correct identification and phenotyping of subjects at increased risk for metabolic diseases, reliable automatic segmentation of adipose tissue into subcutaneous adipose tissue and visceral adipose tissue is required. Full manual tissue delineation is a time-and cost-intensive task which is not advisable especially in cohort studies. We propose a 3D convolutional neural network to perform automated adipose tissue segmentation from T1-weighted whole-body fast spin echo images in a fast and robust way with reliable separation of visceral and subcutaneous fat masses.
|
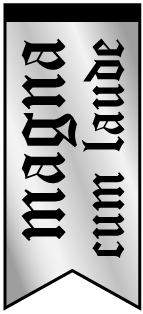 |
0668.
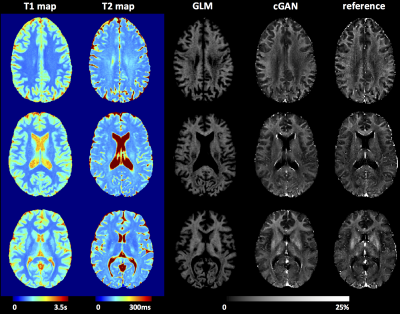 |
2 |
Deriving Brain Myelin Water Fraction Maps from Relaxometry: a Data-Driven Approach

Gian Franco Piredda, Tom Hilbert, Jonas Richiardi, Erick J. Canales-Rodríguez, Marco Pizzolato, Reto Meuli, Jean-Philippe Thiran, Tobias Kober
Currently, one of the gold-standard methods to obtain brain myelin water fraction (MWF) maps is the multi-echo spin-echo sequence. To overcome some of its limitations (e.g. long acquisition times), a data-driven approach for deriving MWF maps is proposed here. A general linear model (GLM) and a conditional generative adversarial network (cGAN) were trained to learn the reference MWF from T1 and T2 maps acquired in a healthy cohort. While GLM-derived maps exhibited MWF overestimation, especially in WM tissue, the cGAN yielded images in agreement with the reference. The proposed methods were preliminarily tested in patients and revealed myelin degradation in expected areas.
|
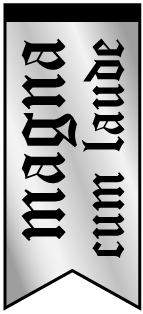 |
0669.
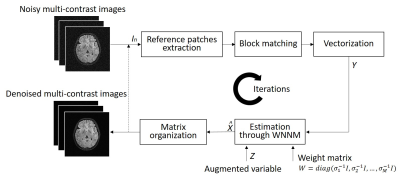 |
3 |
Simultaneous Denoising of Multi-contrast MR Images Using a Novel Weighted Nuclear Norm Minimization Approach

Yujiao Zhao, Yilong Liu, Henry Ka-Fung Mak, Ed X. Wu
A typical clinical MRI scanning session produces image sets with same geometries but different contrasts. These multi-contrast images often share strong structural similarities or correlations despite their contrast differences. Most existing MRI denoising methods deal with single-contrast images independently, and fail to explore and utilize such correlations across contrasts. In this study, we present a simultaneous denoising method for multi-contrast images based on low rank multi-contrast patch matrix completion. This denoising method exploits the structural similarities across contrasts, and outperforms the traditional method. Further, it does not compromise the image fidelity in absence of any structural similarities across contrasts.
|
|
0670
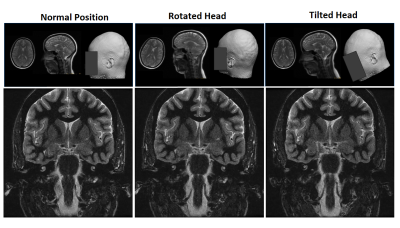 |
4 |
A generalized deep learning framework for multi-landmark intelligent slice placement using standard tri-planar 2D localizers Video Permission Withheld
Dattesh Shanbhag, Chitresh Bhushan, Andre Maximo, Arathi Sreekumari, Dan Rettmann, Dawei Gui, Anja Kammeier, Uday Patil, Rakesh Mullick, Teck Beng Desmond Yeo, Thomas Foo
We demonstrate a deep learning-based MRI scan workflow for intelligent slice placement (ISP) for multiple brain landmarks (MSP, AC-PC, entire visual pathway, pituitary, IAC, hippocampus, TOF-Angiography) based on standard 2D tri-planar localizer images. Unlike prior approaches to automatic plane prescription, this method uses deep learning to determine all necessary planes without the need for explicit delineation of landmark structures and provides visual feedback to the user. For all the landmarks, we demonstrate that the proposed method can achieve landmark slice placement with mean distance error < 1 mm (N = 505) on localizer images itself and is comparable or better to slice placement obtained using higher resolution images. Results indicate excellent feasibility of the method for clinical usage.
|
|
0671.
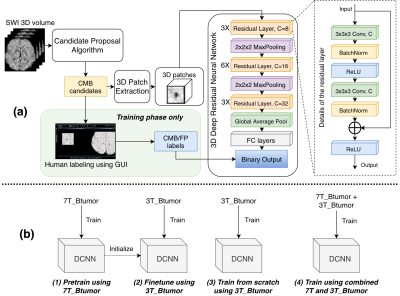 |
5 |
Generalization and Analysis of a Deep Convolutional Neural Networks (DCNN)-Based Cerebral Microbleed Detection Algorithm

Yicheng Chen, Melanie Morrison, Sivakami Avadiappan, Angela Jakary, Helen Kim, Janine Lupo
We tested and analyzed a deep learning based CMB detection algorithm previously developed using 7T SWI images we found that this method can be well generalized to different scan environment, parameters and pathologies.
|
|
0672.
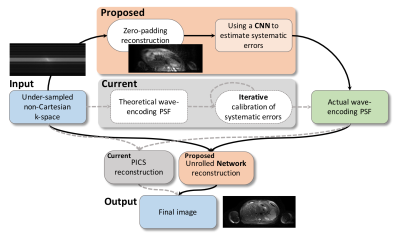 |
6 |
Learning Systematic Imperfections and Image Reconstruction with Deep Neural Networks for Wave-Encoded Single-Shot Fast Spin Echo

Feiyu Chen, Joseph Cheng, Valentina Taviani, John Pauly, Shreyas Vasanawala
Wave-encoded single-shot fast spin echo imaging (SSFSE) achieves good structural delineation in less than a second while its calibration and reconstruction usually take more than a minute to finish. This study proposes a method to accelerate the calibration and reconstruction for wave-encoded SSFSE with a deep-learning-based approach. This method first learns the systematic imperfections with a deep neural network, and then reconstructs the image with another unrolled convolutional neural network. The proposed approach achieves 2.8-fold speedup compared with conventional approaches. Further, it can also reduce the ghosting and aliasing artifacts generated in conventional calibration and reconstruction approaches.
|
|
0673.
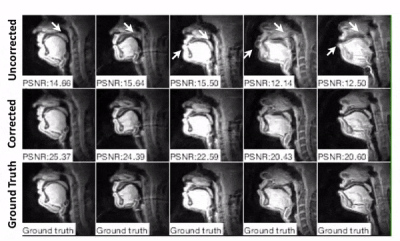 |
7 |
Calibrationless Deblurring of Spiral RT-MRI of Speech Production Using Convolutional Neural Networks

Yongwan Lim, Yannick Bliesener, Shrikanth Narayanan, Krishna Nayak
Spiral acquisitions are preferred in speech real-time MRI because of their high efficiency, making it possible to capture vocal tract dynamics during natural speech production. A fundamental limitation is signal loss and/or blurring due to off-resonance, which degrades image quality most significantly at air-tissue boundaries. Here, we present a machine learning method that corrects for off-resonance artifact in spiral images of upper airway without acquiring a field map. Residual neural networks are trained on images simulated with known spiral trajectories and readout duration. The off-resonance blurring is effectively resolved at the articulator boundaries for long readout (~8ms) images at 1.5T.
|
|
0674.
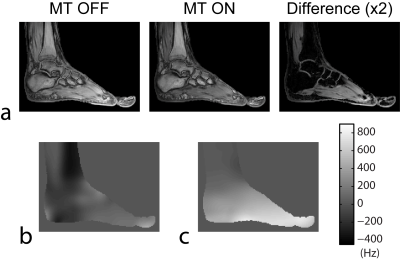 |
8 |
Robust Region-Growing Fat-Water Imaging Using MT-Based B0 Field Priors

Alexey Samsonov, Julia Velikina, Fang Liu
Region-growing (RG) is one of most computationally efficient fat-water separation methods, which exploits B0 smoothness assumption for separation with improved accuracy. However, practical robustness of RG method is majorly limited by low accuracy of initial seeded B0 values, which are problematic to select due to competing off-resonance from fat. Recently, it was demonstrated that insensitivity of fat to magnetization transfer (MT) preparation can be utilized to produce fat-insensitive B0 field priors. Here, we present a modified RG method that exploits this phenomenon to solve problems of seeding and stability of the original method and to attain robust F/W separation.
|
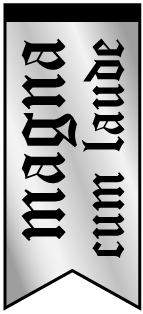 |
0675.
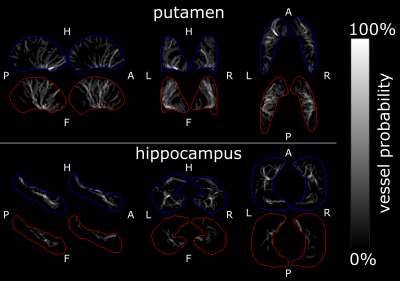 |
9 |
High resolution imaging of the arterial and venous vasculature in deep gray matter

Hendrik Mattern, Julio Acosta-Cabronero, Oliver Speck
Recent studies investigated the human vasculature by building vascular atlases and performing vessel morphometry. Even though these studies showed great potential, the used resolution might not be sufficiently high to study changes the microvasculature as there occur in i.e. Alzheimer’s. Therefore, an approach to assess the arterial and venous microvasculature in deep gray matter regions is presented. Based on high resolution ToF angiography and QSM acquired at 7T vascular densities and vessel probability maps were computed.
|
|
0676.
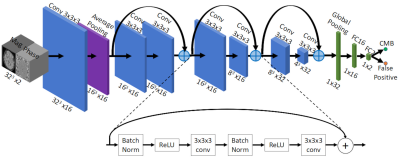 |
10 |
Automatic Detection of Cerebral Microbleeds using Susceptibility Weighted Imaging and Deep Learning

Saifeng Liu, David Utriainen, Chao Chai, Yongsheng Chen, Lin Wang, E. Mark Haacke
Detecting cerebral microbleeds can be time-consuming and prone to errors. Susceptibility weighted imaging (SWI) offers exquisite sensitivity to blood products. Furthermore, the use of SWI phase data makes it possible to differentiate diamagnetic calcifications from paramagnetic microbleeds. In this paper, we present a machine learning model based on residual neural networks, using SWI magnitude and phase data. The model was tested on 41 cases and compared with human raters with different levels of experience. A sensitivity of 93%, a positive predictive value of 80%, and 1.5 false positives per subject were achieved, outperforming both human raters and previously reported methods.
|
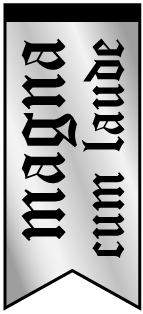 |
0677.
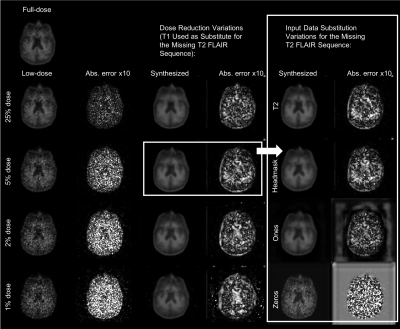 |
11 |
How to Generalize a Deep Learning Model to New Data Lacking Appropriate MR Inputs? An Exploration using Ultra-low-dose Amyloid PET/MRI

Kevin Chen, Matti Schürer, Jiahong Ouyang, Enhao Gong, Solveig Tiepolt, Osama Sabri, Greg Zaharchuk, Henryk Barthel
When generalizing a deep learning model to data acquired from different sites, non-harmonized protocols might result in missing or different data for use as model inputs. For an ultra-low-dose amyloid PET/MRI network we trained previously and wish to apply to other data, protocol differences resulted in missing MRI data and different PET image qualities. In this project we showed that structurally similar contrasts for substitution is a viable alternative in the case of missing input data and that noise reduction was observed when applying the network on any of the low-dose PET images.
|
|
0678.
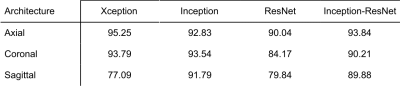 |
12 |
Visualizing and understanding deep learning features for MRI motion artifact detection

Irene Fantini, Letícia Rittner, Clarissa Yasuda, Roberto Lotufo
The high performance reported by Deep Convolutional Neural Networks (CNNs) on image classification, detection and segmentation are contributing to its usage increase, including the CNNs applied to Medical Image. To automatically detect motion artifacts on MRI we fine-tuned four different CNNs. Visualizing the features extracted by the CNN is crucial to understand the reported result from each architecture. Using a gradient-based visualization method, we noticed that all architectures have salient points on Cerebrospinal Fluid (CSF) and background. Furthermore, the architectures that reported better results also extract information from white-matter, confirming that this anatomical structure has essential information regarding the task.
|
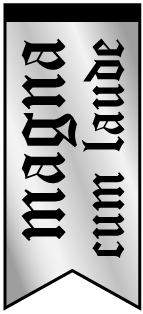 |
0679.
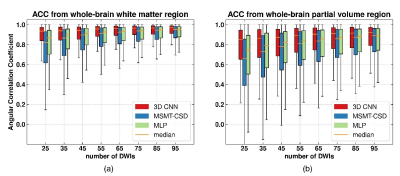 |
13 |
Accelerating Acquisition for the Reconstruction of Fiber Orientation Distribution Function Using Convolutional Neural Network

Ting Gong, Hongjian He, Zhichao Lin, Zhiwei Li, Feng Yu, Jianhui Zhong
Fiber orientation distribution function (fODF) is one of the key components for establishing brain connectivity maps. However, its reliable reconstruction usually requires a large number of diffusion weighted image (DWI) volumes leading to long acquisition time. Our previous study has shown the potential of multi-layer perceptron in recovering fODF directly from a small number of DWIs. In this study, we proposed a 3-dimentional convolution neural network to take the spatial correlation into consideration, allowing robust fODF reconstruction with up to eleven-fold reduction of number of DWIs. This method offers a new approach for fast fODF reconstruction which could facilitate its clinical applications.
|
|
0680.
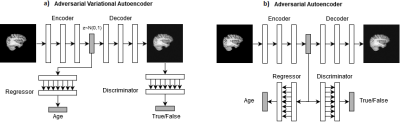 |
14 |
Variational vs Adversarial Autoencoders for Visualization and Interpretation of Deep Learning Features of Brain Aging

Luis Souto Maior Neto, Mariana Bento, David Gobbi, Richard Frayne
Deep learning models are state-of-the-art for numerous medical imaging prediction tasks. Exact understanding of learned prediction features is hard, slowing down their clinical application. New methods for interpreting such models are needed to enable clinical translation. Autoencoders are models that allow visualization of learned features, however they can lack detail in their visualizations and thus, cannot provide guidance on features that hinders their use. We propose a method for understanding relevant learned features by visualizing them in detailed images. We show that a model trained to predict age based on brain MR data learns known features of the aging brain.
|
|