2nd Hour
Poster: fMRI Analysis: Task & Resting State
Power Pitch Poster
fMRI
Wednesday, 15 May 2019
Power Pitch Theater B - Exhibition Hall
16:45 - 17:45
|
|
Plasma # |
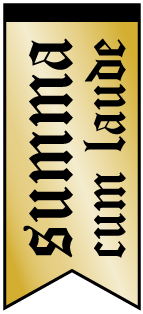 |
0906.
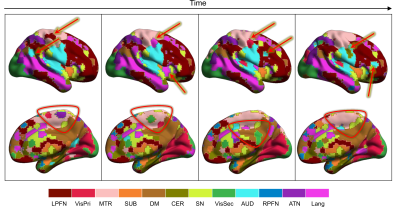 |
16 |
Capturing brain spatial topography reconfiguration using ultra-high-order independent component analysis (ICA)

Armin Iraji, Zening Fu, Thomas DeRamus, Shile Qi, Harshvardhan Gazula, Vince Calhoun
The brain reorganizes its activity interactively at different temporal and spatial scales. These reconfigurations include variations within a region’s function, and changes in the spatial topography of functional organizations. Here, we present a novel approach utilizing the concept of functional hierarchy to capture between- and within-subject spatiotemporal variations. The approach uses ultra-high-order independent component analysis (ICA) (1000 components) to estimate functional units overcoming the limitation of current parcellation approaches and captures brain spatiotemporal reconfiguration at a finer spatial level. Our regularized cost function optimizes the selection of the best subsets of changes at each time-window and protects against spurious fluctuations.
|
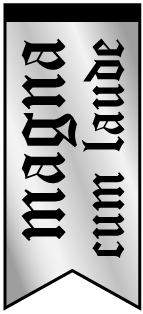 |
0907.
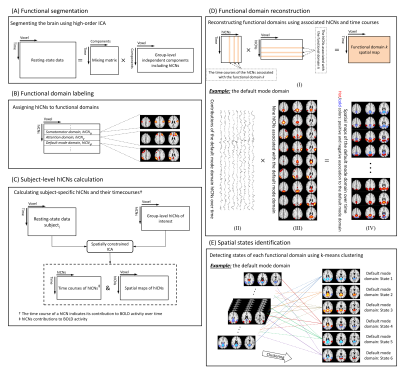 |
17 |
Characterizing Hierarchical Spatial Variations of the Brain: A Novel Analysis Framework to Study Dynamic Brain Function

Armin Iraji, Zening Fu, Eswar Damaraju, Thomas DeRamus, Noah Lewis, Juan Bustillo, Rhoshel Lenroot, Ayse Belger, Judy Ford, Sarah McEwen, Daniel Mathalon, Bryon Mueller, Godfrey Pearlson, Steven Potkin, Adrian Preda, Jessica Turner, Jatin Vaidya, Theo van Erp, Vince Calhoun
A novel, data-driven analysis framework was proposed to characterize the spatial variation of brain function. This framework utilizes the concept of a functional hierarchy and encapsulates the spatiotemporal variations of brain functional domains from its associated high-order intrinsic connectivity networks. The results show spatial fluidity behavior of functional domains, including a broad spectrum of changes in regional associations from strong coupling to complete decoupling. Interestingly, such spatial variations drive the interactions between functional domains, showing concurrent evaluation in time. Our results further highlight that the captured spatial dynamics can serve as potential biomarkers for patients with schizophrenia.
|
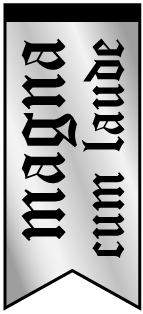 |
0908.
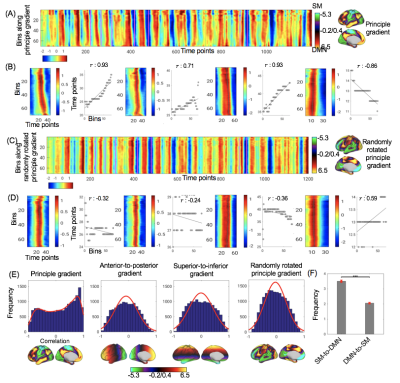 |
18 |
Principal gradient of resting-state functional connectivity represents a principal propagating direction of spontaneous brain activity

Yameng Gu, Xiao Liu
Decomposing functional connectome to low-dimensional spaces revealed a principal gradient cross brain hierarchy from the primary sensorimotor areas to the default mode network. However, the physiological meaning underlying this principal gradient remains unclear. Here, we showed significant propagating activities of resting-state fMRI along this gradient direction. We further used simulation to demonstrate that the propagating activity can result in a gradient in its propagating direction with the use of the low-dimensional embedding method. Overall, the findings suggest that the principal connectivity gradient actually represents the major propagating direction of spontaneous brain activity, which is likely across brain hierarchies.
|
|
0909
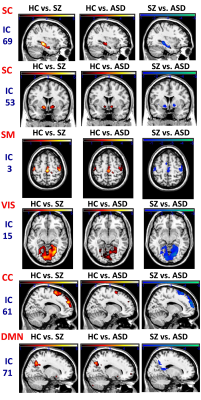 |
19 |
A unified ICA framework for identifying neuro-markers in functional connectivity among multiple different brain disorders Video Permission Withheld
Yuhui Du, Zening Fu, Dongdong Lin, Mustafa Salman, Md Rahaman, Anees Abrol, Jing Sui, Shuang Gao, Elizabeth Osuch, Vince Calhoun
Functional network from ICA using fMRI data has been applied to identify biomarkers of brain disorders. However, the networks from ICA might be slightly different, making the comparison of results across different studies/diseases difficult. We propose a data-driven framework to estimate functional network maps and their inter-connectivity for linking neuromarkers among different disorders and studies. Our method is capable of computing functional networks which are optimized for independence based on each coming individual-subject data, and remaining their correspondence across different subjects by using unbiased templates. The results show this approach is an effective method for studying and classifying multiple-disorders.
|
|
0910.
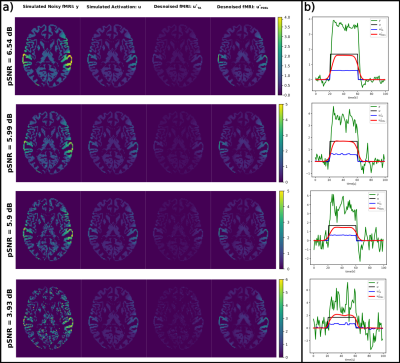 |
20 |
Novel 4-D Algorithm for Functional MRI Image Regularization using Partial Differential Equations

Isa Costantini, Samuel Deslauriers-Gauthier, Rachid Deriche
State-of-the-art techniques for denoising functional MRI (fMRI) images consider the problems of spatial and temporal regularization as decoupled tasks. In this work we propose a partial differential equations (PDEs) -based algorithm that acts directly on the 4-D fMRI image. Our approach is based on the idea that large image variations should be preserved as they occur during brain activation, but small variations should be smoothed to remove noise. Starting from this principle, by means of PDEs we were able to smooth the fMRI image with an anisotropic regularization, thus recovering the location of the brain activations in space and their timing and duration.
|
|
0911.
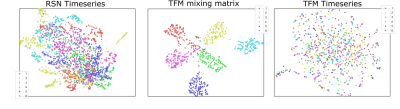 |
21 |
Subject specific functional connectivity fingerprints made possible with temporal ICA

Daniel Gomez, Alberto Llera Arenas, Zahra Fazal, David Norris
In the current contribution we use an ultra-fast fMRI sequence (TR=0.158s) and temporal ICA to generate subject specific fingerprints of unprecedented specificity. These "fingerprints" are generated from the mappings between common RSNs and time-series of temporal ICs. We also show that these time-series are virtually free from physiological contamination.
|
|
0912.
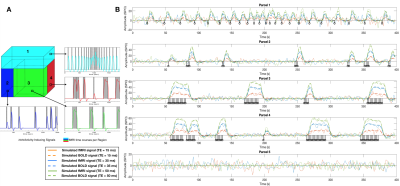 |
22 |
Deconvolution of multi-echo functional MRI data with Multivariate Multi-Echo Sparse Paradigm Free Mapping

Eneko Uruñuela-Tremiño, Stefano Moia, Javier Gonzalez-Castillo, César Caballero-Gaudes
This work introduces a novel multivariate deconvolution method to blindly estimate neuronal-related -signal changes in multi-echo fMRI data without prior knowledge of their timings. In contrast to current voxel-based approaches, this algorithm simultaneously deconvolves all brain’s voxel time series and uses structured spatio-temporal regularization to improve the quality of the estimates. Besides, it employs stability selection procedures to overcome the selection of regularization parameters of the deconvolution problem. We evaluate its performance in multiple realistic simulations, showing its potential to blindly detect BOLD events in paradigms when no prior information can be obtained.
|
|
0913.
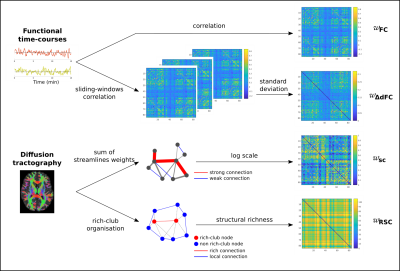 |
23 |
Dynamic functional connectivity and its relation to structural rich-club organisation

Marion Sourty, Andrew Zalesky, Fernando Calamante
The relationship between static functional connectivity (FC) and structural connectivity (SC) has been long-recognised. More recently, functional connectivity has increasingly focused on the investigation of temporal variations of the BOLD fMRI signal; however, the role of SC on the observed FC fluctuations is not trivial or well understood. We show here that the structural rich-club organisation of the brain better describes the FC fluctuations than simply considering SC strength.
|
|
0914.
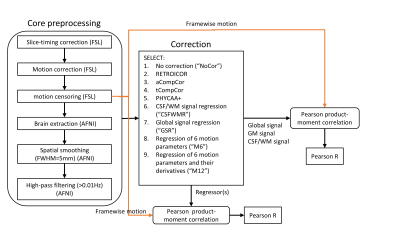 |
24 |
Effect of physiological correction on residual motion in resting-state fMRI

M. Aras Kayvanrad, J. Jean Chen, Stephen Strother
There remain significant residual motion effects in the resting-state fMRI signal after motion correction, motion censoring, and motion regression. Due to the residual motion in the BOLD signal, use of data-driven nuisance regressors for physiological noise correction can potentially be effective in removing the residual motion. The aim of the current study is to investigate the effect of different regressors on residual motion. Our data show that the residual motion is removed by aCompCor and GSR. We recommend the output of the preprocessing pipelines be correlated against framewise motion to ensure any residual motion is removed prior to subsequent analysis.
|
|
0915.
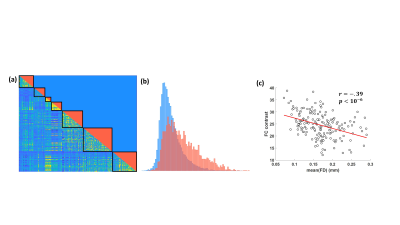 |
25 |
The Effect of Model-Based and Data-Driven Physiological Noise Correction Techniques on the Degree of Clustering in Resting-State fMRI Functional Connectivity

Michalis Kassinopoulos, Georgios Mitsis
One of the most essential steps in the analysis pipeline of fMRI studies is the correction for fluctuations due to physiological processes and head motion. This is particularly relevant for resting-state fMRI functional connectivity (FC) studies, where the SNR is lower and physiological fluctuations may introduce common variance in the signals from different areas of the brain, inflating FC. Several physiological noise correction techniques have been developed over the years. Nevertheless, an optimal preprocessing pipeline for FC has not yet been established. In this study, we examined more than 400 different pipelines using both model-based and data-driven techniques and have found that tissue-based regressors significantly improve the identifiability of well-known resting-state networks.
|
|
0916.
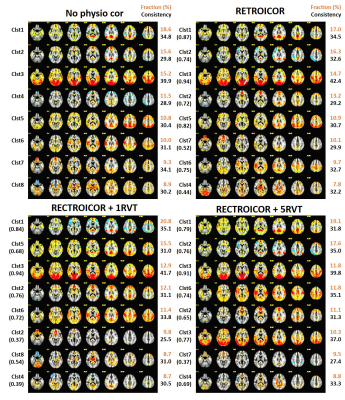 |
26 |
Physiologic noise correction effects on seed based spontaneous co-activation pattern analysis

Wanyong Shin, Xiao Liu, Mark Lowe
The Spontaneous co-activation (CAP) approach has shown the potential to investigate the dynamic change in resting state fMRI. However, dynamic rs-fMRI would be expected to be sensitive to physiologic fluctuation since dynamic changes of CAP pattern could be affected by the spatiotempolly correlated physiologic pulsation in the brain. We investigate the physiologic noise effects on seed-based CAP analysis in this study, and we find that the physiologic noise corrected data generates not only a different fraction and consistency of CAP patterns, but also different CAP patterns.
|
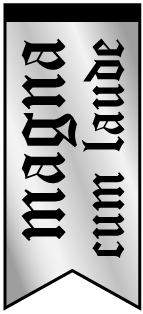 |
0917.
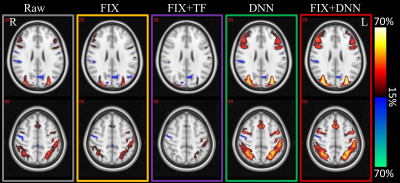 |
27 |
An automated deep neural network for denoising task-based fMRI data

Zhengshi Yang, Xiaowei Zhuang, Karthik Sreenivasan, Virendra Mishra, Dietmar Cordes
Deep neural networks (DNN) recently have gained increasing interest in neuroimaging research for different applications. However, it remains to be an open question whether and how artificial neural networks can be used for denoising neuroimaging data. In this study, we have designed a DNN network for denoising task-based fMRI data. The result showed that DNN can efficiently reduce physiological fluctuation and achieve more homogeneous fMRI activation maps.
|
|
0918.
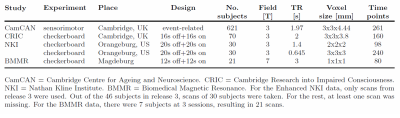 |
28 |
Task-fMRI: pre-whitening and hemodynamic response function modeling substantially impair specificity and sensitivity

Wiktor Olszowy, John Aston, Richard Henson, Catarina Rua, Guy Williams
Given the recent controversies in some neuroimaging statistical methods, we investigated pre-whitening and hemodynamic response function (HRF) modeling techniques available in AFNI, FSL and SPM and used in task-fMRI studies. We found that pre-whitening often leaves much positive autocorrelated noise in the data, which leads primarily to false positives. Also, we observed that for an event-related design dataset, the use of the simple canonical HRF led to a strong sensitivity deterioration. Currently, both specificity and sensitivity could be increased in task-fMRI studies with some less popular, but widely available statistical methods.
|
|
0919
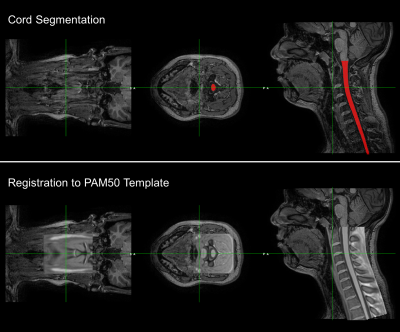 |
29 |
Towards a Standard Pipeline for the Analysis of Human Spinal Cord fMRI Data Series Video Permission Withheld
Mauro DiNuzzo, Julien Cohen-Adad, Fabio Mangini, Laura Maugeri, Marta Moraschi, Daniele Mascali, Federico Giove, Michela Fratini
Spinal cord functional magnetic resonance imaging (scfMRI) is still insufficiently exploited in clinical settings due to several challenging problems related to image acquisition and analysis. In the present study, we implemented and optimized a scfMRI data analysis pipeline built around the Spinal Cord Toolbox (SCT). We acquired axial and sagittal functional images at 3T from the spinal cord of forty-six healthy subjects performing an isometric motor task. Using our SCT-based pipeline, we substantially improved motion correction and image registration. Furthermore, we found task-induced activations with a high level of statistical significance.
|
|
0920.
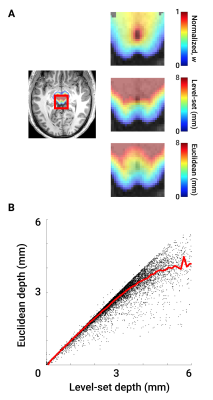 |
30 |
Computationally efficient depth-mapping scheme for human colliculi

Paulina Truong, Jung Hwan Kim, Ricky Savjani, David Ress
Determining depth in human colliculi is prone to errors due to its rapidly varying curvature. Thus, Euclidean definitions of depth fall short in describing the topography of deep collicular tissue. We developed a method of surface-based depth mapping that calculates a depth coordinate using an algebraic level-set method. We then generated kernels based on this level-set depth that sample functional data in a nonlinear trajectory with increasing depth. Using this method, we re-analyzed data on polar-angle representation of saccadic eye movements and were able to produce smoother laminar profiles that confirmed distinct depth-dependence of saccade-evoked activity.
|
|