ORGANIZERS: Jung-Ah Choi, Riccardo Lattanzi, Kimberly Amrami, Jan Fritz, Miika Nieminen, Hiroshi Yoshioka
Monday Parallel 4 Live Q&A |
Monday, 10 August 2020, 14:30 - 15:15 UTC |
Moderators: Richard Kijowski & Valentina Pedoia |
Skill Level: Intermediate to Advanced
Session Number: C-Tu-02
Overview
This session is intended to give an overview of machine learning in acquisition and reconstruction of musculoskeletal (MSK) MR images. The speakers will also give updates on how machine learning is used in image processing and interpretation of MSK MR images.
Target Audience
Clinicians and researchers interested in the application of machine learning in MSK imaging.
Educational Objectives
As a result of attending this course, participants should be able to:
- Explain how machine learning can be applied in acquisition and reconstruction of MR images in MSK imaging;
- Explain how machine learning can be applied in image processing and interpretation of MR images in MSK imaging; and
- Describe algorithms for machine learning to be used in acquisition, reconstruction, processing, and interpretation of MSK MR images.
|
|
Machine Learning for MSK Image Acquisition & Reconstruction
Fang Liu
Watch the Video
This talk will provide an overview of the latest deep learning techniques that have been applied to image reconstruction of musculoskeletal MRI. The topics focus on rapid imaging for both static MRI and quantitative MRI, such as T2 and T1rho mapping. The talk will conclude by highlighting potential challenges in deep learning-based reconstruction that warrant further investigation.
|
|
|
Machine Learning for MSK Image Processing & Interpretation
Cem Deniz
Watch the Video
This educational lecture will provide an overview of the machine learning approaches applied in MR image processing and interpretation for musculoskeletal disorders.
|
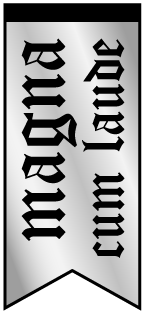 |
0250.
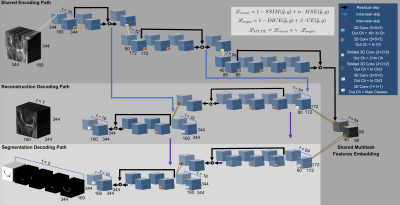 |
Task-Based UltraFast MRI: Simultaneous Image Reconstruction and Tissue Segmentation
Francesco Caliva1, Adam Noworolski2, Andrew Leynes1,3, Claudia Iriondo1,3, Sharmila Majumdar1, Peder Larson1, and Valentina Pedoia1
1Department of Radiology and Biomedical Imaging, University of California, San Francisco, San Francisco, CA, United States, 2EECS, University of California, Berkeley, Berkeley, CA, United States, 3Department of Bioengineering, University of California, Berkeley, Berkeley, CA, United States
Watch the Video
We propose a novel task based deep learning framework for simultaneous MRI reconstruction and segmentation. On a dataset of retrospectively undersampled knee-DESS volumes we demonstrate that irrespective of ultra-high acceleration factors (i.e. 48×) a multitask 3D encoder-decoder is capable of reconstructing with high fidelity the knee MRI, accurately segment cartilaginous and meniscal tissues and reliably provide cartilage thickness. Our multitask solution outperforms two other methods: a compressed sensing reconstruction step, followed by a deep learning-based tissue segmentation. The other method comprises a cascade of two convolutional neural networks that sequentially perform image reconstruction and segmentation.
|
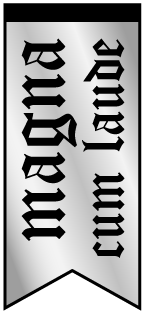 |
0251.
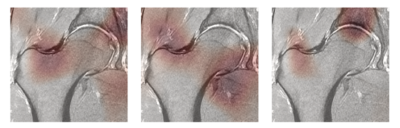 |
Computer Aided Detection AI Reduces Inter-Reader Variability in Grading Hip Abnormalities from MRI
Radhika Tibrewala1, Eugene Ozhinsky1, Rutwik Shah1, Io Flament1, Kay Crossley2, Ramya Srinivasan1, Thomas M Link1, Valentina Pedoia1, and Sharmila Majumdar1
1Radiology and Biomedical Imaging, University of California San Francisco, San Francisco, CA, United States, 2La Trobe Sport and Exercise Medicine Research Centre, Melbourne, Australia
Watch the Video
MRI based hip degeneration grading is difficult, time-intensive and prone to inter-reader variability, aggravated by the lack of a standard hip grading scale. Recent research using deep learning based clinical classification tasks has shown efficiency in knee degenerative changes. In this study, we aim to develop a deep learning based hip degenerative changes classification model (for cartilage lesions, bone marrow edemas and cysts) and evaluate its performance. In addition to that, we develop an AI-assist tool based on model predictions to test on two radiologists to see if the inter-reader agreement increases by using the AI-assist.
|
|
0252.
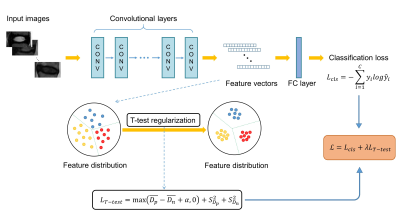 |
Automated Grading of Lumbar Disc Degeneration Using T-test Regularized Network
Shui Liu1, Fei Gao2, Xiaodong Zhang1, Jue Zhang2,3, Xiaoying Wang1,3, and Jing Fang2,3
1Department of Radiology, Peking University First Hospital, Beijing, China, 2College of Engineering, Peking University, Beijing, China, 3Academy for Advanced Interdisciplinary Studies, Peking University, Beijing, China
To enrich the representation capability of the CNN model and achieve more accurate lumbar disc degeneration grading, inspired by student T-test in statistics, we propose a T-test regularization strategy focusing on pushing away different categories from each other in feature space.
|
|
0253.
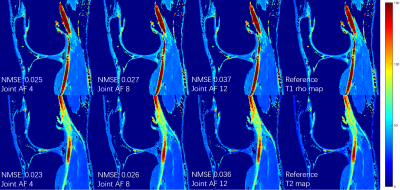 |
Deep Learning MR Relaxometry with Joint Spatial-Temporal Under-sampling
Hongyu Li1, Mingrui Yang2, Jeehun Kim2, Ruiying Liu1, Chaoyi Zhang1, Peizhou Huang1, Sunil Kumar Gaire1, Dong Liang3, Xiaojuan Li2, and Leslie Ying1
1Department of Biomedical Engineering, Department of Electrical Engineering, The State University of New York at Buffalo, Buffalo, NY, United States, 2Program of Advanced Musculoskeletal Imaging (PAMI), Cleveland Clinic, Cleveland, OH, United States, 3Paul C. Lauterbur Research Center for Biomedical Imaging, Medical AI research center, SIAT, CAS, Shenzhen, China
Watch the Video
This abstract presents a deep learning method to generate MR parameter maps from very few subsampled echo images. The method uses deep convolutional neural networks to learn the nonlinear relationship between the subsampled T1rho/T2-weighted images and the T1rho/T2 maps, bypassing the conventional exponential decay models. Experimental results show that the proposed method is able to generate T1rho/T2 maps from only 2 subsampled echo images with quantitative values comparable to those of the T1rho/T2 maps generated from fully-sampled 8 echo images using the conventional exponential decay curve fitting.
|
|