Digital Poster Session
Neuro: Neuroimaging and AI
Back to Program-at-a-Glance
|
Digital Posters Neuro
1862 -1875 Neuroimaging and AI - Neuroimaging & AI: Applications from Stroke to MS
1876 -1888 Neuroimaging and AI - Neuroimaging & AI: Poster Session 3
1889 -1902 Neuroimaging and AI - Neuroimaging & AI: Poster Session 4
1903 -1915 Neuroimaging and AI - Neuroimaging & AI: Tumour & Hemorrhage
|
|
|
1862.
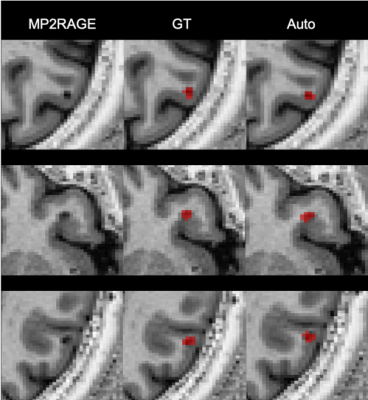 |
Automatic detection of Multiple Sclerosis cortical lesions based on 3D-FLAIR and MP2RAGE sequences
Francesco La Rosa1,2, Ahmed Abdulkadir3, Mário João Fartaria1,2,4, Reza Rahmanzadeh5,6, Riccardo Galbusera5,6, Jean-Philippe Thiran1,2, Cristina Granziera5,6, and Meritxell Bach Cuadra1,2
1LTS5, École Polytechnique Fédérale de Lausanne, Lausanne, Switzerland, 2Radiology Department, Center for Biomedical Imaging, Lausanne University Hospital and University of Lausanne, Lausanne, Switzerland, 3Universitäre Psychiatrische Dienste and University of Bern, Bern, Switzerland, 4Siemens Healthcare AG Switzerland, Lausanne, Switzerland, 5Neurologic Clinic and Policlinic, Departments of Medicine, Clinical Research and Biomedical Engineering, University Hospital Basel and University of Basel, Basel, Switzerland, 6Translational Imaging in Neurology (ThINk) Basel, Department of Medicine and Biomedical Engineering, University Hospital Basel and University of Basel, Basel, Switzerland
View the Poster
Multiple Sclerosis cortical lesions are not readily visible in conventional MRI, but they are clinically highly relevant and have been recently included in the MS diagnostic criteria. However, advanced MRI sequences such as the MP2RAGE are needed in order to identify them visually. In this work, we propose an automatic method based on a convolutional neural network to automatically detect cortical lesions. In a cohort of 84 patients with FLAIR and MP2RAGE acquisitions our framework achieves a 77% cortical lesion detection rate with a 26% lesion-wise false positive rate.
|
|
1863.
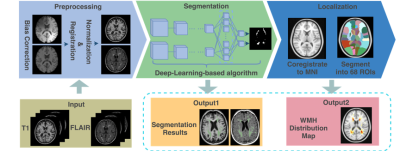 |
Deep-Learning based Segmentation and Localization of White Matter Hyperintensities on Magnetic Resonance Images
Wenhao Jiang1,2, Jian Zhang1, Peng Cao3, Jing Gu1, and Silun Wang1
1YiWei Medical Technology Co.,Ltd, Shenzhen, China, 2School of Computer Science and Technology, Harbin Institute of Technology, Harbin, China, 3Department of Diagnostic Radiology, The University of Hong Kong, Hong Kong, Hong Kong
View the Poster
In this work, deep-learning-based methods were utilized to segment white matter hyperintensities (WMH) on T2-weighted MRI from 213 patients diagnosed with ischemia and lacune. Vulnerability maps of each disease were generated regarding the prevalence of WMH registered to the standard MNI template. The WMH were allocated into 68 regions of interest using a Hammers atlas. Correlation among the region-specific WMH was analyzed for a lesion-symptom study.
|
|
1864.
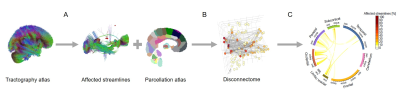 |
Brain graph representation of structural disconnectivity estimated with an atlas-based approach in multiple sclerosis
Veronica Ravano1,2,3, Michaela Andelova4, Mazen Fouad A-Wali Mahdi1, Reto Meuli2, Tomas Uher4, Jan Krasensky5, Manuela Vaneckova5, Dana Horakova4, Tobias Kober1,2,6, and Jonas Richiardi1,2
1Advanced Clinical Imaging Technology, Siemens Healthcare, Lausanne, Switzerland, 2Department of Radiology, Lausanne University Hospital and University of Lausanne, Lausanne, Switzerland, 3Medical Imaging Processing, École Polytechnique Fédérale de Lausanne (EPFL), Lausanne, Switzerland, 4Department of Neurology and Center of Clinical Neuroscience First Faculty of Medicine, Charles University and General University Hospital in Prague, Prague, Czech Republic, 5MR unit, Department of Radiology First Facutly of Medicine, Charles University and General University Hospital in Prague, Prague, Czech Republic, 6LTS5, Ecole Polytechnique Fédérale de Lausanne (EPFL), Lausanne, Switzerland
Watch the Video
In multiple sclerosis, the correlation between clinical scores and classical radiological metrics is poor (“clinico-radiological paradox”). To improve the prediction of future disease course, we suggest to study structural brain disconnectivity resulting from white matter lesions. We proposed an atlas-based approach to quantify structural disconnectomes without diffusion imaging, as it is typically not part of clinical routine MR protocols for multiple sclerosis. The disconnectome was modelled as a graph where brain regions are vertices and affected connections edges. Our method provides a new representation of brain disconnectivity that enables to stratify multiple sclerosis patients in two groups with different prognosis.
|
|
1865.
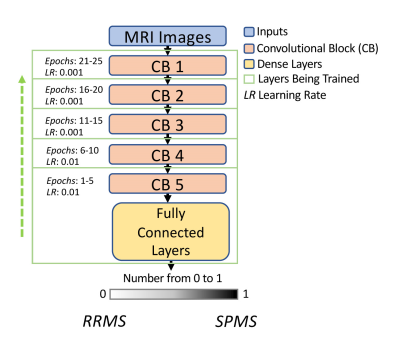 |
Transfer learning with progressive training as a novel approach for classifying clinical forms of multiple sclerosis based on clinical MRI
Daniel McClement1, Jinseo Lee2, Glen Pridham2, Olayinka Oladosu2, Zahra Hosseinpour2, and Yunyan Zhang2
1University of British Columbia, Vancouver, BC, Canada, 2University of Calgary, Calgary, AB, Canada
View the Poster
Transfer learning and greedy layer-wise training are two potential approaches to advance the performance of deep learning, particularly in fields with limited sample size including medical imaging. Taking the advantage of both, we have implemented a novel strategy that allows progressive training with transfer learning using the VGG19 network. Based on clinical MRI of 19 patients only, our approach achieved 88% accuracy in classifying relapsing remitting from secondary progressive multiple sclerosis (MS), 6% greater than training with the traditional approach. This innovative method may help provide new insight into the pathogenesis and progression mechanisms in MS.
|
|
1866.
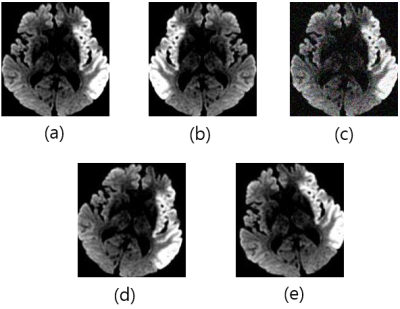 |
Automatic Assessment of DWI-ASPECTS for Assessment of Acute Ischemic Stroke using Recurrent Residual Convolutional Network
Luu-Ngoc Do1, Byung-Hyun Beak2, Seul-Kee Kim3, Hyung-Jeong Yang4, Woong Yoon5, and Ilwoo Park5
1Department of Radiology, Chonnam National University, Gwangju, Korea, Republic of, 2Department of Radiology, Chonnam National University Hospital, Gwangju, Korea, Republic of, 3Department of Radiology, Chonnam National University Hwasun Hospital, Gwangju, Korea, Republic of, 4Department of Electronics and Computer Engineering, Chonnam National University, Gwangju, Korea, Republic of, 5Department of Radiology, Chonnam National University Medical School and Hospital, Gwangju, Korea, Republic of
Poster Permission Withheld
The purpose of this study was to demonstrate the feasibility of using deep learning algorithms for automatic classification of DWI-ASPECTS from patients with acute ischemic stroke. DWI data from 319 patients with acute anterior circulation stroke were used to train and validate recurrent residual convolutional neural network models for binary task of classifying low- vs high- DWI-ASPECTS. Our model produced the accuracy of 84.9 ± 1.5% and the AUC of 0.925 ± 0.009, suggesting that this algorithm may provide an important ancillary tool for clinicians in a time-sensitive assessment of DWI-ASPECTS from acute ischemic stroke patients.
|
|
1867.
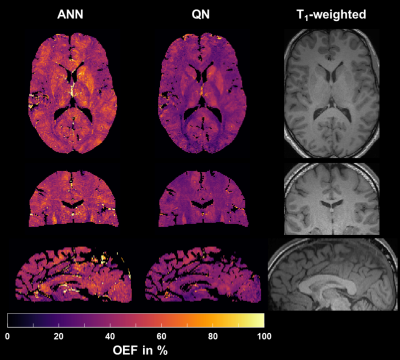 |
Using an Artificial Neural Network for Fast Mapping of the Oxygen Extraction Fraction with Combined QSM and qBOLD
Simon Hubertus1, Sebastian Thomas1, Junghun Cho2, Shun Zhang3,4, Yi Wang2,3, and Lothar R. Schad1
1Computer Assisted Clinical Medicine, Heidelberg University, Mannheim, Germany, 2Department of Biomedical Engineering, Cornell University, Ithaca, NY, United States, 3Department of Radiology, Weill Cornell Medical College, New York, NY, United States, 4Department of Radiology, Tongji Hospital, Wuhan, China
Poster Permission Withheld
MRI-based mapping of the oxygen extraction fraction (OEF) is a valuable addition to diagnosis and treatment planning of various diseases; yet, it often lacks robustness and suffers from elaborate, time-consuming reconstructions. We trained an artificial neural network (ANN) on simulated QSM values and qBOLD data, tested it in 7 healthy volunteers and compared it to a standard quasi-Newton approach. The ANN reduced the intersubject variability of OEF by regularizing the reconstruction. Moreover, it lowered the reconstruction time from approximately one hour to one second and removed the necessity of accurate parameter initialization through an additional acquisition.
|
|
1868.
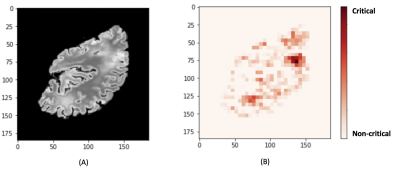 |
An end-to-end MR-based classification of arteriolar sclerosis using 3D convolutional neural networks
Nazanin Makkinejad1, Ashish A. Tamhane2, Carles Javierre Petit1, Arnold M. Evia2, David A. Bennett2, Julie A. Schneider2, and Konstantinos Arfanakis1,2
1Biomedical Engineering, Illinois Institute of Technology, Chicago, IL, United States, 2Rush Alzheimer's Disease Center, Rush University Medical Center, Chicago, IL, United States
View the Poster
Arteriolar sclerosis is common in the brains of older adults and has been shown to be associated with cognitive decline and dementia. Definitive diagnosis of arteriolar sclerosis is only possible at autopsy. The purpose of this work was to develop an end-to-end deep learning model to predict the presence of severe arteriolar sclerosis from MR images without the need to extract hand-engineered features. The model was developed by combining ex-vivo MRI and pathology data in a large community-based cohort of older adults.
|
|
1869.
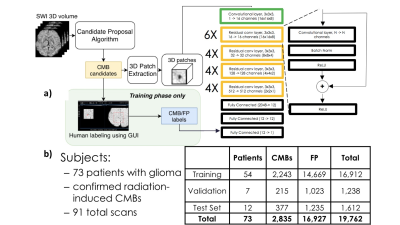 |
Fast prediction of whole-brain cerebral microbleed masks from 7T SWI imaging with a deep residual 3D UNet
James R Golden1, Yicheng Chen1, Melanie A Morrison1, Kate Nelson1, and Janine M Lupo1
1Radiology, UCSF, San Francisco, CA, United States
Watch the Video
Cerebral microbleeds (CMBs) are known risk factors of stroke and hemorrhage that can be a marker of cognitive impairment. Although CMBs are easily visualized with susceptibility weighted imaging, they are burdensome to localize and quantify manually. Image processing algorithms based on the radial symmetry transform have previously been used to identify candidate CMBs, and convolutional neural networks have been effective at distinguishing real CMBs from mimics with high sensitivity and specificity. A deep neural network was trained to carry out this entire pipeline and to predict CMB voxel masks using a dataset of radiation therapy-induded CMBs from patients with gliomas.
|
|
1870.
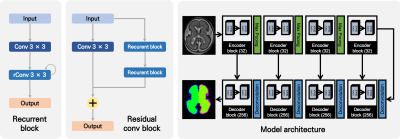 |
Fetal Cortical Plate Segmentation using 2D Recurrent Residual U-Net with Plane Aggregation
Jinwoo Hong1,2, HyukJin Yun1, Gilsoon Park2, Jong-Min Lee2, and Kiho Im1
1Division of Newborn Medicine, Boston Children's Hospital, BOSTON, MA, United States, 2Hanyang University, Seoul, Korea, Republic of
View the Poster
Quantitative measurements about sulcal patterns are a basic approach to characterize the possibility of neurological disorders. In this study, we used recurrent residual U-Net. Also, to improve the accuracy of segmentation, the models of each plain in the MR image were produced separately and the results combined. As a result, the cortical plate segmentation showed an average dice coefficient of 0.901 ± 0.030, and segmentation for the internal region of the cortical plate showed 0.978 ± 0.010. The proposed method is expected to be useful as a quantitative developmental measurement of the fetus.
|
|
1871.
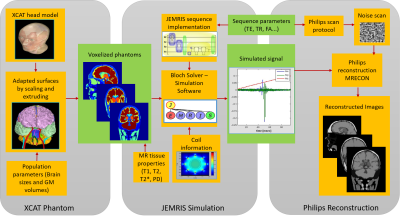 |
Realistic MRI simulation pipeline for anatomically variable normal young, aging and diseased brain
Aymen Ayaz1, Jouke Smink2, Tom Geraedts3, Cristian Lorenz4, Juergen Weese4, and Marcel Breeuwer1,2
1Biomedical Engineering, Eindhoven University of Technology, Eindhoven, Netherlands, 2MR R&D – Clinical Science, Philips Healthcare, Best, Netherlands, 3MR R&D – Collaboration Office, Philips Healthcare, Best, Netherlands, 4Philips Research Laboratories, Hamburg, Germany
View the Poster
A pipeline based on the XCAT phantom, the JEMRIS software for simulating the MR signal and a commercial reconstruction pipeline has been set-up for simulating realistic brain MRI images. Using this pipeline, an anatomically variable brain MRI population is simulated across age and gender. Anatomical variation is generated by means of changing individual brain sizes, and cortical gray matter volumes to mimic aging brain. MS lesions are simulated to mimic diseased brain as well. Significant contrast is generated across detailed brain structures. The commercial reconstruction pipeline increased the realism of simulated data.
|
|
1872.
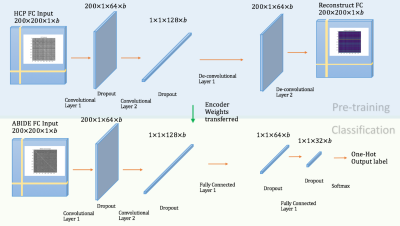 |
A robust transfer learning method to improve early diagnosis of autism spectrum disorder classification
Bonian Lu1 and Gopikrishna Deshpande1
1AU MRI Research Center, Electrical and Computer Engineering, Auburn University, Auburn, AL, United States
View the Poster
Overfitting, the main issue that constrains the validity and generalizability of machine-learning in neuroimaging-based diagnostic-classification, is in part due to small sample-sizes in relation to what is required for generalization. Even with data aggregation (such as in ABIDE), the relatively smaller sample-sizes are a result of the fact that it is difficult/expensive to acquire data from clinical-populations. With healthy-controls, we have comparatively larger samples available. Therefore, we propose to address overfitting by using larger healthy-samples (HCP) to learn the neural signature of healthy-controls, with the aim of transferring that learning into the context of discriminating Autism from healthy-controls.
|
|
1873.
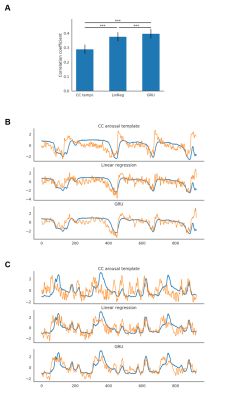 |
Predicting the brain state index, pupil dynamics, with rs-fMRI signal-trained models
Filip Sobczak1,2, Patricia Pais-Roldán1,3, Xiaoning Zhao1, and Xin Yu1,4
1Translational Neuroimaging and Neural Control Group, High Field Magnetic Resonance Department, Max Planck Institute for Biological Cybernetics, Tuebingen, Germany, 2Graduate Training Centre of Neuroscience, International Max Planck Research School, University of Tuebingen, Tuebingen, Germany, 3Forschungszentrum Jülich, Jülich, Germany, 4Athinoula A. Martinos Center for Biomedical Imaging, Massachusetts General Hospital and Harvard Medical School, Charlestown, MA, United States
View the Poster
Lately, we have acquired the resting state fMRI (rs-fMRI) signal with pupillometry from anesthetized rats to investigate specific resting-state network correlations with brain state-specific pupil dynamics. Here we used the acquired data to estimate the instantaneous arousal index based on the rs-fMRI signal. We evaluated predicting pupil dynamics using three methods: linear regression (LR), gated recurrent unit (GRU) neural networks and a previously proposed correlation-based (CC) approach. LR and GRU provided much better predictions than CC method. Also, using weighted PCA components, we can identify specific regions of the brain related to pupil dynamics as the brain state index.
|
|
1874.
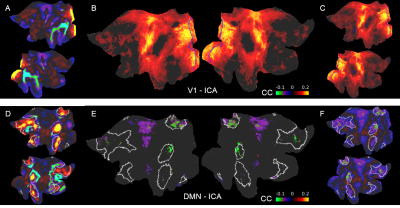 |
Decoupling the default mode network and global state oscillation by neural network-based prediction of the fMRI signal fluctuation
Filip Sobczak1,2, Yi He1,3, Terrence J. Sejnowski4,5, and Xin Yu1,6
1Translational Neuroimaging and Neural Control Group, High Field Magnetic Resonance Department, Max Planck Institute for Biological Cybernetics, Tuebingen, Germany, 2Graduate Training Centre of Neuroscience, International Max Planck Research School, University of Tuebingen, Tuebingen, Germany, 3Danish Research Centre for Magnetic Resonance, Hvidovre, Denmark, 4Howard Hughes Medical Institute, Computational Neurobiology Laboratory, Salk Institute for Biological Studies, La Jolla, CA, United States, 5Division of Biological Sciences, University of California, San Diego, CA, United States, 6Athinoula A. Martinos Center for Biomedical Imaging, Massachusetts General Hospital and Harvard Medical School, Charlestown, MA, United States
View the Poster
Previously we developed an echo-state network (ESN) to predict the future temporal evolution of the rs-fMRI slow oscillatory feature from both rodent and human brains. In particular, rs-fMRI signals from individual blood vessels that were strongly correlated with neural calcium oscillations were used to train an ESN to predict brain state-specific rs-fMRI signal fluctuations. Here, the ESN-based predictive model was applied to classify rs-fMRI datasets from the Human Connectome Project (HCP). The ESN enables to decouple the brain state-dependent global rs-fMRI signal fluctuation from the intrinsic activity of the default-mode network.
|
|
1875.
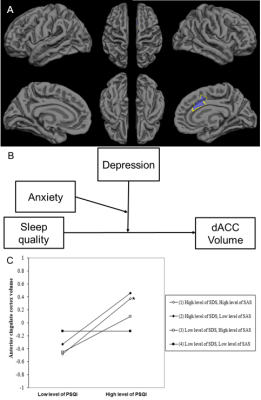 |
The moderation effects of depression and anxiety on sleep-linked cortical morphology in general population
Yanlin Wang1, Ping Jiang1, Shi Tang1, Lu Lu1, Xuan Bu1, Hailong Li1, Yingxue Gao1, Lianqing Zhang1, Lingxiao Cao1, Jing Liu1, Xinyue Hu1, Xinyu Hu1, Qiyong Gong1, and Xiaoqi Huang1
1Huaxi MR Research Center (HMRRC), Functional and molecular imaging Key Laboratory of Sichuan Province, Department of Radiology, West China Hospital, Sichuan University, Chengdu 610041, China, Chengdu, China
View the Poster
It remains unclear whether cortical changes in poor sleep quality are modulated by emotional distress in the general population. An Elastic-Net Regularized Generalized Linear Models based on bootstrapping was implemented to acquire candidate regions for sleep quality. The moderation effects of emotional distress on the relationship between sleep quality and cortical morphometry were acquired by a hierarchical regression. The low level of emotional distress could offset positive correlation between sleep quality and dorsal anterior cingulate cortex (dACC) volume. The result indicates dACC volume enlargement might be a compensatory response to poor sleep quality, or a marker of resilience against recurrent emotional distress.
|
|
Back to Top |
|
1876.
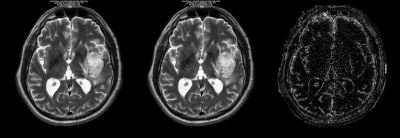 |
Deep learning based image reconstruction for improved multiparameter mapping and synthetic MRI
Ken-Pin Hwang1, Xinzeng Wang2, Marc Lebel2, Peter Johansson3, Catharina Petersen3, Marcel Warntjes3, Ersin Bayram2, Suchandriam Banerjee2, Jingfei Ma1, and Jason M Johnson4
1Department of Imaging Physics, The University of Texas M.D. Anderson Cancer Center, Houston, TX, United States, 2MR Applications and Workflow, GE Healthcare, Waukesha, WI, United States, 3SyntheticMR, Linkoping, Sweden, 4Department of Radiology, The University of Texas M.D. Anderson Cancer Center, Houston, TX, United States
View the Poster
Images from a multiparameter mapping sequence were reconstructed with a novel deep learning based reconstruction (DL Recon) method trained to remove noise and enhance edges. Mean T1, T2, and PD values as measured in a system phantom differed by less than 0.6% between the DL and conventional reconstructions, while noise was lower in all measurements on DL Recon images. In vivo synthetic images also exhibited reduced noise and increased definition of structures. We find that the SNR and resolution benefits of DL Recon applied to raw MR data extend to improve the fitted relaxation maps and subsequent synthetic images.
|
|
1877.
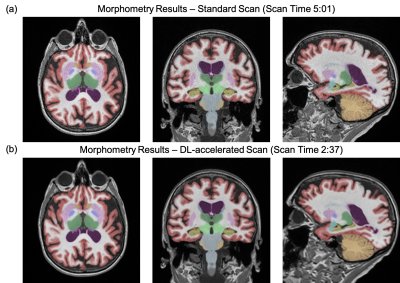 |
Deep Learning Enables Accurate Quantitative Volumetric Brain MRI with 2x Faster Scan Times
Long Wang1, Suzie Bash2, Sara Dupont1, Sebastian Magda3, Chris Airriess3, Enhao Gong1, Greg Zaharchuk1,4, Ajit Shankaranarayanan1, and Tao Zhang1
1Subtle Medical Inc, Menlo Park, CA, United States, 2RadNet, Encino, CA, United States, 3CorTechs Labs Inc, San Diego, CA, United States, 4Stanford University, Stanford, CA, United States
Poster Permission Withheld
3D T1-weighted MRI is valuable for providing high resolution structural information and is commonly used in brain MRI exams despite long scan times. The recent development of deep learning (DL) has shown great potential for scan time reduction. However, the generalizability of DL methods is of concern for actual clinical deployment. In this study, we apply FDA-cleared DL software to accelerate 3D T1-weighted MRI scans by two folds and evaluate the quantification accuracy using FDA-cleared image analysis software. This study provides insight into the generalizability and accuracy of DL in clinical settings.
|
|
1878.
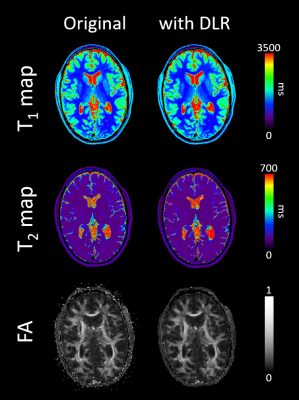 |
Quantitative imaging enhancement with deep learning based denoising: a validation study
Valentin H. Prevost1, Bei Zhang2, Clemence Bal3, and Wolter de Graaf2
1Canon Medical Systems Corporation, Tochigi, Japan, 2Canon Medical Systems Europe, Zoetermeer, Netherlands, 3Bordeaux University, Bordeaux, France
Watch the Video
In MRI, signal noise ratio is the key point, determining the image quality and its medical relevance. Different ways exist to significantly increase it and then to access to high resolution imaging. Preliminary works introduced deep learning based denoising on several contexts and conclude to a significant signal noise ratio on qualitative images. However, it has not been tested yet on quantitative imaging sequences, questioning its feasibility and potential in this context. In this study, we investigated the DLR impact on calculated T1 and T2 relaxation times and diffusion imaging in healthy human brain areas.
|
|
1879.
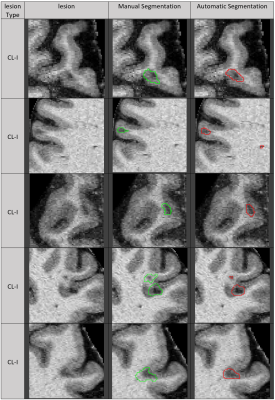 |
Automatic deep learning segmentation of grey and white matter lesions in 7T MRI data
Frederik Luca Sandig1,2, Julian Emmerich3,4, Edris El-Sanosy1,2, Mark Ladd1,2,3, Heinz-Peter Schlemmer1, and Sina Straub3
1Division of Radiology, German Cancer Research Center (DKFZ), Heidelberg, Germany, 2Faculty of Medicine, Heidelberg University, Heidelberg, Germany, 3Division of Medical Physics in Radiology, German Cancer Research Center (DKFZ), Heidelberg, Germany, 4Faculty of Physics and Astronomy, Heidelberg University, Heidelberg, Germany
Watch the Video
Multiple sclerosis is a chronic inflammatory disease characterized by demyelination. Magnetic resonance imaging (MRI) is an important method for diagnosis and prognosis predictions. The ongoing study presented here shows the use of deep learning algorithms for white and grey matter lesion segmentation in 7T MRI images. Results show high accuracy for patients with high lesion load. Furthermore, it is demonstrated that it is possible to train a neural net to find small cortical lesions, which can be used as a potential biomarker.
|
|
1880.
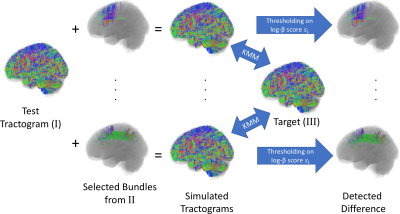 |
Representation Learning of Fiber Tracts and Direct Comparison of Tract Distributions
Kaikai Shen1,2,3, Lee Reid1, Samantha Burnham1, and Jurgen Fripp1
1Australian eHealth Research Centre, CSIRO, Herston, Australia, 2Department of Biomedical Sciences, Macquarie University, Sydney, Australia, 3Rapiscan Systems, Sydney, Australia
View the Poster
The distribution of fiber population in the whole brain can be inferred from the samples generated by tractography on diffusion MRI. In this paper, we modeled the distribution of fiber population globally based on representation learning method. Using deep neural networks, we performed dimension reduction on the fiber tracts, and modeled the fiber population by a probability distribution over a latent space in lower dimension. This method enabled us to identify tracts distributed with different densities when compared with another tractogram, and can thus be used to identify structural difference or to detect spurious tracts caused by probabilistic tractography.
|
|
1881.
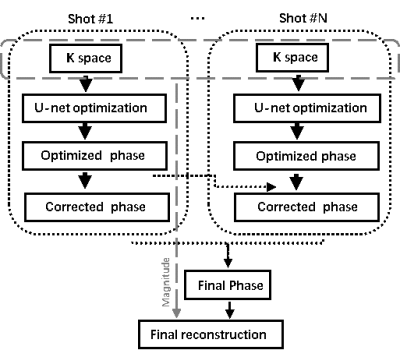 |
Incorporating Deep Learning into Multi-shot EPI DWI Reconstruction
Hui Zhang1, ZiYing Feng2, Fei Dai1, WeiBo Chen3, YiShi Wang4, ChengYan Wang2, and He Wang1,2
1Institute of Science and Technology for Brain-Inspired Intelligence, Fudan University, Shanghai, China, 2Human Phenome Institute, Fudan University, Shanghai, China, 3Philips Healthcare, Shanghai, China, 4Philips Heathcare, Beijing, China
View the Poster
This work tried to optimize MUSE for high resolution multi-shot EPI DWI reconstruction by using Convolutional neural network (CNN). By using multi-scale U-net learning neural network, final reconstructed images showed less artifacts due to the improved phase estimation. Besides, CNN can improve the computational efficiency for the image reconstruction process.
|
|
1882.
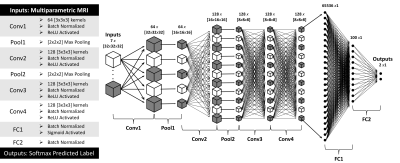 |
A Machine Learning Based Method to Distinguish between Tumor and Non-Tumor within FLAIR Non-Enhancing Regions of Brain Lesions
Robert Wujek1,2, Melissa Prah3, Mona Al-Gizawiy3, and Kathleen Schmainda3
1Graduate School, Medical College of Wisconsin, Milwaukee, WI, United States, 2Graduate School, Marquette University, Milwaukee, WI, United States, 3Biophysics, Medical College of Wisconsin, Milwaukee, WI, United States
View the Poster
There is a clinical need for a biomarker that can distinguish between infiltrative tumor and non-tumor within FLAIR non-enhancing brain lesions. In this study, we used multi-parametric MRI and FLAIR non-enhancing biopsy ground truths to train a 3D convolutional neural network to address this need.
|
|
1883.
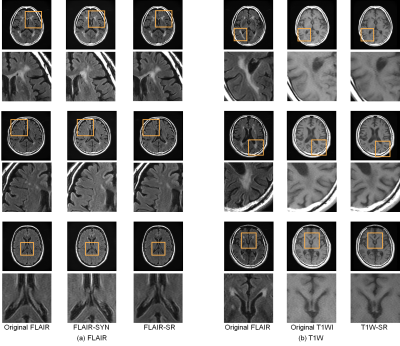 |
Initial clinical evaluation of deep-learning-based image synthesis and superresolution using a clinical dataset of patients with brain lesions
Ai Nakao1, Daiki Tamada2, Tomohiro Takamura2, Shintaro Ichikawa2, Utaroh Motosugi2, and Yasuhiko Terada1
1Institute of Applied Physics, University of Tsukuba, Tsukuba, Japan, 2Department of Radiology, University of Yamanashi, Chuo, Japan
Watch the Video
Shortening scan time has been a long-standing goal in MRI, and image synthesis and superresolution using deep learning (DL) are promising tools for achieving this goal. However, most of studies use datasets with healthy volunteers for network training, and the clinical evaluation has not yet been fully performed. Here we trained networks using a large, clinical dataset of patients with brain lesions, and evaluated the generated images in terms of the diagnostic image quality and performance. Our results showed that FLAIR superresolution outperformed FLAIR image synthesis. Our results could also provide useful guidelines for evaluating diagnostic performance of DL-based networks.
|
|
1884.
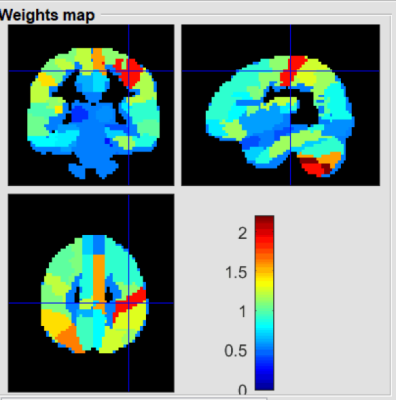 |
Regional gray matter volume predicts HIV-positive patients with HAND using a machine learning approach
Danhui Fu1, Guanqiao Jin1, Lidong Liu1, Wenjuan Deng1, Wei Zhang1, Qianlin Ding1, Weiyin Vivian Liu2, Long Qian2, and Danke Su1
1GuangXi Medical University Cancer Hospital, Nanning, China, 2MR Research, GE Healthcare, Beijin, China
View the Poster
Conventional neuropsychometric tests could not early detect HIV-associated neurocognitive disorder (HAND) on HIV-patients. More objective indicators with neuroimaging techniques have offered to sub-classify patients with specific symptoms. Significant differences of regional gray matter volumes (rGMV) betweenHIV-positive group and age-matched healthy group was found in our preliminary study. Ten major rGMV well distinguished HIV-positive patients with HAND from those without HAND. Our study showed rGMV might be an indicator to explain for neurocognitive impairments.
|
|
1885.
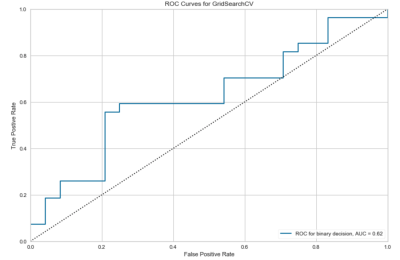 |
A Support Vector Machine Prediction Model for HIV-Status in Adults Using Magnetic Resonance Angiography and Arterial Spin Labeling of the Brain
Kyle Murray1, Igor B Titoff2, Henry Wang3, Jianhui Zhong1,3, and Giovanni Schifitto2,3
1Physics and Astronomy, University of Rochester, Webster, NY, United States, 2Neurology, University of Rochester, Rochester, NY, United States, 3Imaging Sciences, University of Rochester, Rochester, NY, United States
View the Poster
HIV-infection is known to be related to vascular diseases, which can be explored via cerebral imaging techniques such as magnetic resonance angiography (MRA) and arterial spin labeling (ASL). In this abstract, we use quantitative features extracted from demographic information and vascular imaging data, only, to predict HIV-status in adults using a support vector machine (SVM). This is the first SVM to reasonably predict HIV-status in an aging HIV-population on combination antiretroviral therapy, which may have future biological implications in HIV research.
|
|
1886.
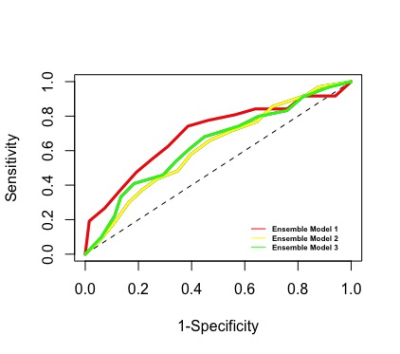 |
Functional and structural connectivity predict MS patients’ impairment level using an ensemble model applied with a machine learning method
Ceren Tozlu1, Keith Jamison1, Susan Gauthier1,2,3, and Amy Kuceyeski1,4
1Department of Radiology, Weill Cornell Medicine, New York City, NY, United States, 2Judith Jaffe Multiple Sclerosis Center, Weill Cornell Medicine, New York City, NY, United States, 3Department of Neurology, Weill Cornell Medicine, New York City, NY, United States, 4Brain and Mind Research Institute, Weill Cornell Medicine, Ithaca, NY, United States
View the Poster
No study to date has performed a rigorous analysis of the relative contributions of multi-modal imaging data including the brain’s functional (FC) and structural connectivity (SC) in the task of classifying high and low adapting MS patients for a deeper understanding of the connectome-level mechanism contributing to variability in MS-related impairment. We built a machine learning based ensemble model that can accurately classify MS patients as high and low adapters (AUC> 0.626). We observed that SC and FC networks can be used to identify the most discriminative regions and to accurately classify MS patients regarding their impairment level.
|
|
1887.
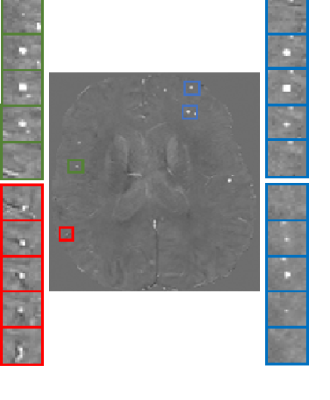 |
Synthetic CMB generation for Training classifiers on QSM images
Saba Momeni1,2, Amir Fazlollahi1, Pierrick Bourgeat1, Paul Yate3, Nawaf Yassi4, Patricia Desmond5, Yongsheng Gao2, Alan Wee Chung Liew6, and Olivier Salvado7
1CSIRO Health and Biosecurity, Brisbane, Australia, 2Griffith University, Brisbane, Australia, 3Department of Nuclear Medicine and Centre for PET, Brisbane, Australia, 4University of Melbourne, Parkville, Australia, Melbourne, Australia, 5Austin Health Heidelberg, Australia, Melbourne, Australia, 6Griffith University, Gold coast, Australia, 7Data61, Brisbane, Australia
View the Poster
The lack of clinical dataset with enough examples of rare lesions challenges supervised machine learning methods. Here we propose to generate synthetic lesions for training a classifier to identify microbleeds from MRI QSM. We show that the performance of the classifier is improved compare to standard data augmentation using actual data alone. Our synthetic dataset can have unlimited size allowing to perform validation experiment, while keeping the actual data for testing. Moreover, many aspects of the data can be investigated, which would not be possible when using actual lesions: lesions can be synthetize on any location, size, shape, and intensity.
|
|
1888.
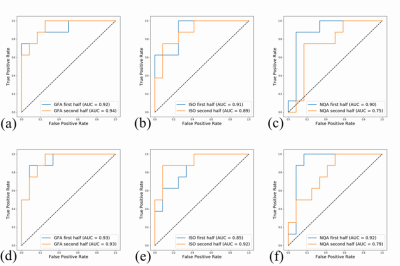 |
Predicting suicidal ideation from depressive patients using autoencoder machine-learning model with brain generalized q-sampling imaging
Jun-Cheng Weng1,2,3, Tung-Yeh Lin1, Man Teng Cheok1, Yuan-Hsiung Tsai4, Yi-Peng Eve Chang5, and Vincent Chin-Hung Chen3,6
1Department of Medical Imaging and Radiological Sciences, Chang Gung University, Taoyuan, Taiwan, 2Medical Imaging Research Center, Institute for Radiological Research, Chang Gung University and Chang Gung Memorial Hospital at Linkou, Taoyuan, Taiwan, 3Department of Psychiatry, Chang Gung Memorial Hospital, Chiayi, Taiwan, 4Department of Diagnostic Radiology, Chang Gung Memorial Hospital, Chiayi, Taiwan, 5Department of Counseling and Clinical Psychology, Columbia University, New York City, NY, United States, 6School of Medicine, Chang Gung University, Taoyuan, Taiwan
View the Poster
It is estimated that at least one million people die by suicide every year, showing the importance of suicide prevention and detection. An autoencoder and machine learning model was employed to predict people with suicidal ideation based on their brain structural imaging. Our results showed that the best pattern of structure across multiple brain locations can classify suicidal ideates from NS and HC with a prediction accuracy of 85%, a specificity of 100% and a sensitivity of 75%. The algorithms developed here might provide an objective tool to help identify suicidal ideation risk among depressed patients alongside clinical assessment.
|
|
Back to Top |
|
1889.
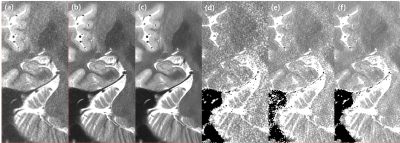 |
Feasibility of deep learned reconstruction for dual-echo fast spin echo based T2 mapping of the hippocampus and hippocampal subfield
Ho-Joon Lee1, Yeonah Kang1, Marc Lebel2, Min Soo Park3, and Joonsung Lee3
1Department of Radiology, Haeundae Paik Hospital, Busan, Republic of Korea, 2MR Collaboration and Development, GE Healthcare, Calagary, AB, Canada, 3GE Healthcare Korea, Seoul, Republic of Korea
Video Permission Withheld
With the increased scientific interest in hippocampal subfields, a clinically applicable T2 mapping method is demanding. With a dual echo fast spin echo based approach, we were able to acquire high resolution T2 maps <5 minutes scan time. With recently introduced deep learned reconstruction, improved image quality of T2 weighted image, and T2 map is observed. Differences in measurements regarding T2 values and volumes of hippocampal subfields will be presented.
|
|
1890.
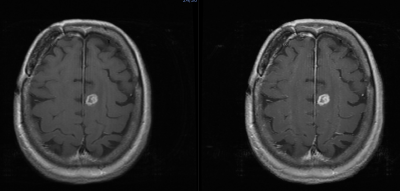 |
The efficacy of half dose gadolinium for contrast enhanced MRI of the brain using artificial intelligence algorithms and machine learning.
Matthew Joseph Kuhn1, Julia Patriarche2, Douglas Patriarche2, Annabel Kuhn3, and Andrew Scott Kuhn4
1Radiology, University of Illinois College of Medicine, Peoria, IL, United States, 2A.I. Analysis, Inc., Seattle, WA, United States, 3Medical College of Wisconsin, Milwuakee, WI, United States, 4Radiology, Yale University, New Haven, CT, United States
View the Poster
The use of half dose gadolinium coupled with artificial intelligence algorithms is effective in visualizing primary and metastatic disease to the brain and reduces gadolinium retention concerns. There is potential cost savings from the use of lower dose gadolinium.
|
|
1891.
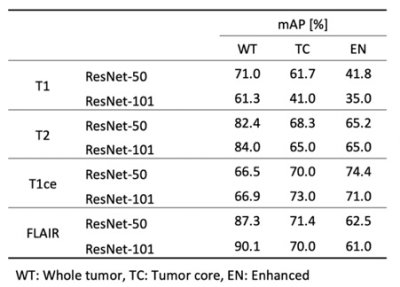 |
3D Mask R-CNN model comparison for brain tumor segmentation in individual modality dataset
Huijin Song1, Eunji Kim2, Hyunsil Cha2, Moon Jung Hwang3, Yongmin Chang2,4, and Chul-Ho Sohn5
1Biomedical Research Institute, Seoul National University Hospital, Seoul, Korea, Republic of, 2Medical & Biological Engineering, Kyungpook National University, Daegu, Korea, Republic of, 3GE Health Korea, Seoul, Korea, Republic of, 4Radiology, College of Medicine, Kyungpook National University, Daegu, Korea, Republic of, 5Radiology, Seoul National University Hospital, Seoul, Korea, Republic of
View the Poster
Deep learning-based brain tumor segmentation requires multi-modality dataset for a precision accuracy. However, multi-modality data acquisition has a limitation due to several reasons. In this study, we propose that a 3D Mask R-CNN network model could provide a reliable accuracy in individual modality dataset for brain tumor segmentation.
|
|
1892.
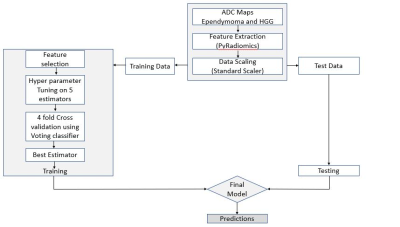 |
Differentiation of adult supratentorial ependymomas from high grade gliomas using textural features based on diffusion weighted imaging.
Abhilasha Indoria1, Madhura Ingalhalikar2, and Jitender Saini1
1Neuroimaging and Interventional Radiology, NIMHANS, Bengaluru, India, 2Symbiosis Centre for Medical Image Analysis (SCMIA), Symbiosis International University, Pune, India
High grade extraventricular supratentorial ependymoma’s in adults are uncommon neoplasms with imaging features that can mimic cortical tumors if small and high-grade gliomas (HGG) if large. No previous work has tried to discriminate ependymoma from high grade gliomas using MRI. Our work evaluates preoperative diffusion weighted imaging for discrimination of ependymomas from grade III and grade IV gliomas using textural analysis. Results demonstrate significant differences in the histogram and first order textural features derived from diffusion weighted imaging in cases of ependymomas and high-grade gliomas.
|
|
1893.
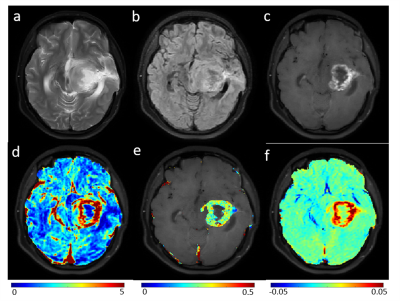 |
On Differentiation of Pilocytic Astrocytoma from High-Grade-Glioma Tumor using Machine Learning Based upon Quantitative T1 Perfusion MRI
Anup Singh1,2, Neha Vats1, Virendra Kumar Yadav1, Anirban Sengupta3, Rakesh Kumar Gupta4, Sumeet Agarwal5, Mamta Gupta6, Rana Patir7, Sunita Ahlawat6, and Jitender Saini8
1Centre for Biomedical Engineering, Indian Institute of Technology Delhi, New Delhi, India, 2Biomedical Engineering, AIIMS, New Delhi, India, 3Vanderbilt University Medical Center, Nashville, TN, United States, 4Department of Radiology, Fortis Memorial Research Institute, Gurugram, India, 5Electrical Engineering, Indian Institute of Technology Delhi, New Delhi, India, 6Fortis Memorial Research Institute, Gurugram, India, 7Neurosurgery, Fortis Memorial Research Institute, Gurugram, India, 8Department of Neuroimaging and Interventional Radiology (NIIR), NIMHANS Bangalore, Bangalore, India
View the Poster
Imaging based diagnosis of Pilocytic Astrocytoma (PA) is quite important for better prognosis. PA can easily be misdiagnosed since its location, growth pattern, and contrast enhancement often mimic a more aggressive high-grade glioma(HGG) tumor. In the current study, quantitative analysis of T1-Perfusion(DCE) MRI data was performed followed by extraction of various features from tumor region and development of an optimized support-vector-machine(SVM) classifier for automatic differentiation of PA vs HGG. The proposed machine learning based approach which uses features derived from quantitative T1 perfusion MRI and tumor volume fraction can enable accurate diagnosis of PA and HGG tumors.
|
|
1894.
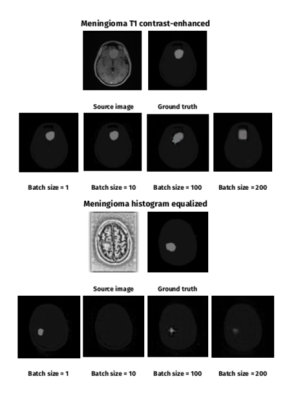 |
Automated Segmentation of Brain Meningioma MRIs with Generative Adversarial Networks
Agata Sularz1, Fulvio Zaccagna1, Dimitri A Kessler1, Fraser Tonnard1, Sonia Benitez1, Thomas Santarius2, Fiona J Gilbert1, Tomasz Matys1, and Joshua D Kaggie1
1Department of Radiology, University of Cambridge, Cambridge, United Kingdom, 2Department of Neurosurgery, University of Cambridge, Cambridge, United Kingdom
View the Poster
We describe a deep learning method for fully-automated brain meningioma MRI segmentation. A conditional generative adversarial network (cGAN) was trained on T1 contrast-enhanced (T1ce) MRI of 37 patients. We explored the effect of batch size, transfer learning and histogram equalization on segmentation accuracy. The highest results for T1ce images were achieved for meningioma dataset of batch size = 1 (DSC = 0.347). Histogram equalization improved segmentation accuracy for batch size = 1 (DSC = 0.364) and batch size = 200. Transfer learning on a publicly available glioma dataset did not improve segmentation results.
|
|
1895.
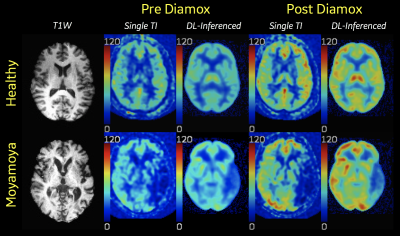 |
Deep Learning synthesis of PET-like CBF maps from arterial spin labeling MRI: Rapid Clinical Deployment & Evaluation
David D Shin1, David Yen-Ting Chen2, Audrey P Fan2, Moss Y Zhao2, Jia Guo3, Suchandrima Banerjee1, and Greg Zaharchuk2
1GE Healthcare, Menlo Park, CA, United States, 2Stanford University, Stanford, CA, United States, 3University of California, Riverside, CA, United States
Watch the Video
Deep learning ASL Pipeline is presented that automatically retrieves relevant MR images from multiple clinical scanners, performs preprocessing/inferencing and returns the derived synthesized PET-like CBF maps back to the scanners for rapid clinical evaluation and prototyping.
|
|
1896.
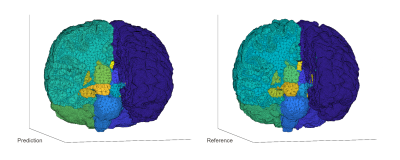 |
Rapid brain segmentation in T1w MRI with fully convolutional networks: development and comparison of different network constructions
Jeremiah W Sanders1, Jason M Johnson2, Jong Bum Son1, Zijian Zhou1, Henry Szu-Meng Chen1, Joshua Yung1, Jason Szu-Meng Stafford1, Melissa Chen2, Maria Gule-Monroe2, Ho-Ling Liu1, Mark D Pagel3, and Jingfei Ma1
1Imaging Physics, University of Texas MD Anderson Cancer Center, Houston, TX, United States, 2Neuroradiology, University of Texas MD Anderson Cancer Center, Houston, TX, United States, 3Cancer Systems Imaging, University of Texas MD Anderson Cancer Center, Houston, TX, United States
Poster Permission Withheld
Neurocognitive function is often associated with structural differences in the brain for patients with neurofibromatosis type-1 (NF1), and studies have shown that NF1 is associated with larger subcortical volumes and thicker cortices of certain brain structures. Routine monitoring of NF1 patients would be possible with tools that enable rapid whole-brain segmentation in standard of care T1w MRI. Modern machine learning techniques, including fully convolutional networks (FCNs), have demonstrated the ability to rapidly perform segmentation tasks across a range of applications. In this work, we investigate the performance of different FCNs for rapid whole-brain segmentation in pediatric T1w brain MRI.
|
|
1897.
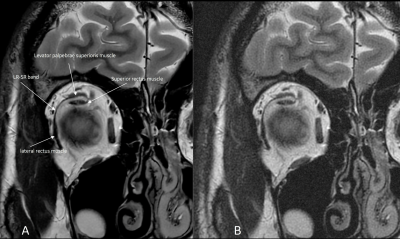 |
Deep-Learning Reconstruction Method for Improved High-Resolution Imaging of the Lateral Rectus-Superior Rectus Band in a Clinical Setting
Patrick Quarterman1, Gul Moonis2, and Marc Lebel3
1GE Healthcare, New York, NY, United States, 2Radiology, Columbia University, New York, NY, United States, 3GE Healthcare, Calgary, AB, Canada
The purpose of this study was to determine the effectiveness of using deep learning reconstruction (DL Recon) for improved signal-to-noise (SNR) allowing for higher resolution imaging of the lateral rectus-superior rectus band of the orbit without substantial increase in scan/exam time. Evaluation of this new reconstruction technique was performed on a group of 5 volunteers with results indicating that higher resolution protocols can produce images with increased SNR and removal of noise leading to higher confidence in identifying the lateral rectus-superior rectus band.
|
|
1898.
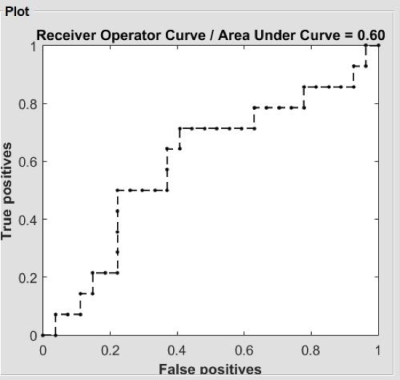 |
Evidence of altered degree centrality on patients with HIV: a machine learning approach
Danhui Fu1, Guanqiao Jin1, Lidong Liu1, Wenjuan Deng1, Sen Hong1, Qianlin Ding1, Long Qian2, Weiyin Vivian Liu2, and Danke Su1
1GuangXi Medical University Cancer Hospital, Nanning, China, 2MR Research, GE Healthcare, Beijin, China
View the Poster
Early detection of cognitive impairments in HIV carriers is essential. Neuroimaging studies has shown brain structure and function of HIV-positive patients are associated with cognition malfunction. However, the conclusions reported from previous studies are mainly based on the univariate analysis, such as t-test. In recent days, increasing attention have focused on the multivariate pattern analysis. Hence, in current study, we demonstrated the aberrant degree centrality (DC) using a machine learning approach. Our results suggested that DC might be an indicator to early detect neurocognitive impairments.
|
|
1899.
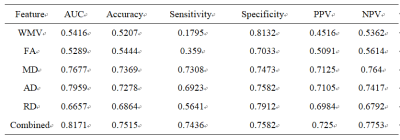 |
A Machine Learning Approach for investigation of white matter abnormalities in Parkinson’s disease
xiao hu1, chaoyong xiao2, xiangrong zhang2, sidong liu3, zaixu cui4, weiguo liu5, and long qian6
1radiology, nanjing brain hospital, nanjing, China, 2nanjing brain hospital, nanjing, China, 3Clinical Medicine, Macquarie University, Sydney, Australia, 4Psychiatry, University of Pennsylvania, Philadelphia, PA, United States, 5neurology, nanjing brain hospital, nanjing, China, 6Biomedical Engineering, Peking University, beijing, China
Parkinson's disease (PD) is a common neurodegenerative disorder characterized by disabling motor and non-motor symptoms.1 The abnormalities of white-matter (WM) tracts/regions have been demonstrated in PD. However, previous studies have largely dependent on univariate analysis, such as t-test, which may result in Type-1 error. Further, it remains unclear whether the disruption of WM tracts/regions provided worthwhile information to identify PD from HC. Hence, in current study, a machine learning approach was applied to investigate the white matter profiles of PD.
|
|
1900.
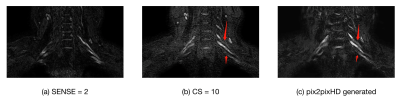 |
Application of GAN in optimizing compressed sensed MR imaging of brachxial plexus
Yan Fu1, Tianjing Zhang2, Haixia Li3, Yichen Tong3, Xiangchuang Kong4, and Dingxi Liu4
1EPFL, Lausanne, Switzerland, 2Philips Healthcare, Guangzhou, China, 3Sun Yat-Sen University, Guangzhou, China, 4Radiology, Union Hospital,Tongji Medical College, Huazhong University of Science and Technology, Wuhan, China
View the Poster
In routine MRI clinical application of compressed sensing, the image quality is often not useful for diagnosis when its CS(Compressed SENSE) acceleration factor is beyond a certain level(e.g. 8 or 10). It is desirable to further accelerate MR sequences in multiple applications such as brachial plexus nerve, coronary artery, and so on. It is possible to use generative adversarial network(GAN) models to further optimize the imaging workflow by improving the image quality of data acquired with high CS factors.
|
|
1901.
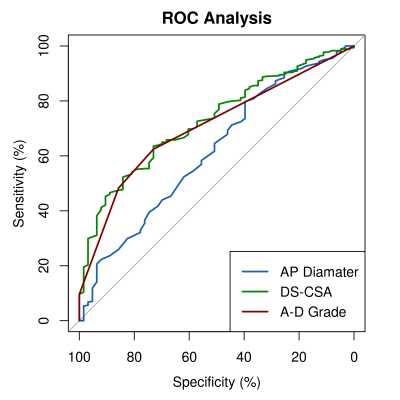 |
Can MRI of the lumbar spine identify neurogenic claudication patients reliably? - Comparison of single variable and machine learning approaches.
Richard Michael Gagen1, Carmen Dragos2, and Charles Hutchinson1
1Populations, Evidence and Technology, Warwick Medical School, University of Warwick, Coventry, United Kingdom, 2Imaging, University Hospital Covenry and Warwickshire, Coventry, United Kingdom
Watch the Video
A case-referant type study assessing the performance of MRI lumbar spine based measurements of the spinal canal in classifying participants as either having neurogenic claudication or non-specific low-back pain. Three measurements of the central lumbar canal are assessed: the dural-sac cross-sectional area, anteroposterior canal diameter and A-D grading system proposed by Schizas et al. The performance of these measurements is compared to a machine learning model incorporating a range of other MRI measurements and demographic information.
|
|
1902.
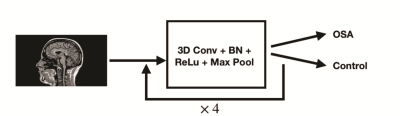 |
Deep Learning for Classifying Patients with Obstructive Sleep Apnea from Healthy Controls using High-resolution T1-weighted Images
Bo Pang1, Bhaswati Roy2, Milena Lai2, Luke Ehlert2, Ravi S. Aysola 3, Daniel W. Kang3, Ariana Anderson1,4, and Rajesh Kumar2,5,6,7
1Statistics, University of California at Los Angeles, Los Angeles, CA, United States, 2Anesthesiology, University of California at Los Angeles, Los Angeles, CA, United States, 3Medicine, University of California at Los Angeles, Los Angeles, CA, United States, 4Psychiatry and Biobehavioral Sciences, University of California at Los Angeles, Los Angeles, CA, United States, 5Radiology, University of California at Los Angeles, Los Angeles, CA, United States, 6Bioengineering, University of California at Los Angeles, Los Angeles, CA, United States, 7Brain Research Institute, University of California at Los Angeles, Los Angeles, CA, United States
View the Poster
Deep learning has demonstrated impressive performance in a wide range of complex and high-dimensional imaging data, including medical image classification and segmentation. One major challenge of harnessing the power of neural networks in image analysis is the small sample size. The present work utilizes deep learning models to classify high-resolution T1-weighted images of obstructive sleep apnea patients (OSA) from healthy controls. Using 193 participants and with adopted model regularization and exponential moving averaging of model weights, we showed 65% testing accuracy and 80% sensitivity. The findings demonstrate the potential for applying neural network models in assisting image-based OSA diagnoses.
|
|
Back to Top |
|
1903.
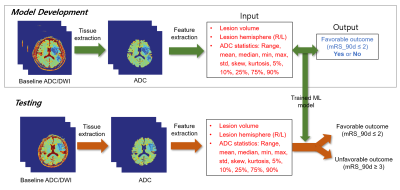 |
Automatic feature extraction and machine learning prediction of stroke functional outcome based on histogram information of baseline ADC
Yoon-Chul Kim1, In-Young Baek2, Ji-Eun Lee2, Ha Na Song2, and Woo-Keun Seo2
1Clinical Research Institute, Samsung Medical Center, Sungkyunkwan Univ. Sch. of Med., Seoul, Republic of Korea, 2Department of Neurology, Samsung Medical Center, Sungkyunkwan Univ. School of Medicine, Seoul, Republic of Korea
View the Poster
This study demonstrates an automatic method that predicts favorable/unfavorable clinical outcome based on pre-treatment DWI data and machine learning (ML) in acute ischemic stroke. We present the use of ADC histogram information in the brain tissue as features for the ML prediction. In the histogram analysis, the 5 or 10 percentile value of the ADC distribution was indicative of clinical outcome regardless of success/failure of recanalization. The ROC analysis in unseen test subjects resulted in an area under the curve (AUC) of 0.79 with the proposed feature extraction, which was greater than 0.71 with the DWI lesion volume only.
|
|
1904.
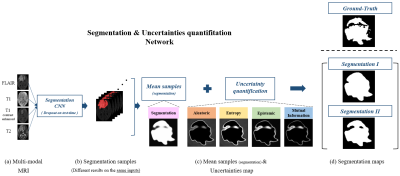 |
Improving Segmentation Method with the Combination between Deep Learning and Uncertainties in Brain Tumor
Joohyun Lee1, Jongho Lee1, and Haejin Kim2
1Department of Electrical and Computer Engineering, Seoul National University, Seoul, Republic of Korea, 2Department of Basic Science and Technology, Hongik University, Sejong, Republic of Korea
View the Poster
Although segmentation using deep learning performs well, it often works poorly on small lesions or boundaries of lesion. This can occur the serious issues when applying in the medical images and the more reliable method is essential. In this study, we developed a deep learning process based on the uncertainty measurements that improves brain tumor segmentation. For selectively maximizing either precision or recall, two types of segmentation methods were presented.
|
|
1905.
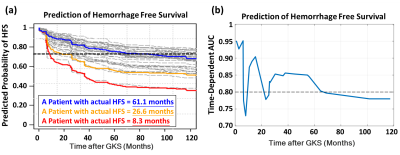 |
Prediction of Hemorrhage Free Survival after Gamma Knife Radiosurgery Based on Preradiosurgical MR Radiomics in Cavernous Malformation
Chia-Feng Lu1, Cheng-Chia Lee2,3,4, Hsiu-Mei Wu3,5, Huai-Che Yang2,3, Man-Chin Chen1, Chung-Jung Lin3,5, Wan-Yuo Guo3,5, and Wen-Yuh Chung2,3
1Department of Biomedical Imaging and Radiological Sciences, National Yang-Ming University, Taipei, Taiwan, 2Department of Neurosurgery, Neurological Institute, Taipei Veteran General Hospital, Taipei, Taiwan, 3School of Medicine, National Yang-Ming University, Taipei, Taiwan, 4Brain Research Center, National Yang-Ming University, Taipei, Taiwan, 5Department of Radiology, Taipei Veteran General Hospital, Taipei, Taiwan
View the Poster
Even though the gamma knife radiosurgery (GKRS) shows promising evidence in treating cavernous malformation (CM), there are still a part of patients will have a recurrent hemorrhage after radiosurgery. We proposed a prediction model based on preradiosurgical MR radiomics to estimate the personalized hemorrhage free survival after GKRS. The satisfactory results of the proposed model can benefit the healthcare in patients with CM by providing a reliable prognosis before treatment.
|
|
1906.
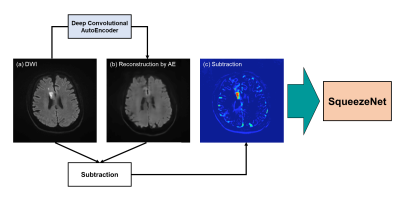 |
Detection of cerebral infarction and estimation of vascular territory via deep convolutional autoencode
Yuya Saito1,2, Akihiko Wada2, Shimpei Kato2, Koji Kamagata2, Masaaki Hori3, and Shigeki Aoki2
1Department of Radiological Sciences, Graduate School of Human Health Sciences, Tokyo Metropolitan University, Tokyo, Japan, 2Department of Radiology, Graduate School of Medicine, Juntendo University, Tokyo, Japan, 3Department of Radiology, Toho University Omori Medical Center, Tokyo, Japan
View the Poster
In the treatment of acute infarction, the detection of abnormal high signals in diffusion weighted images contributes to early diagnosis and treatment of infarction. In this study, we developed a deep learning neural network model via autoencoder (AE) to diagnosis brain infarction and predict vascular territory automatically from a DWI image. 1582 brain images including normal and abnormal brain which had infarctions were used as a training and test dataset. As a result, our model detected brain infarction and estimated vascular territory with high accuracy. It can be an effective indicator for diagnosing correctly infarction and predicting treatment effect.
|
|
1907.
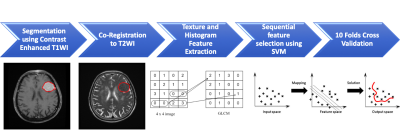 |
Radiomics and Machine Learning for Prediction of Recurrence in Meningiomas
Ching-Chung Ko1,2, Yang Zhang3, Kai-Ting Chang3, Jeon-Hor Chen3,4, and Min-Ying Lydia Su3
1Department of Medical Imaging, Chi Mei Medical Center, Tainan, Taiwan, 2Department of Pharmacy, Chia Nan University of Pharmacy and Science, Tainan, Taiwan, 3Department of Radiological Sciences, University of California, Irvine, CA, United States, 4E-Da Hospital/I-Shou University, Kaohsiung, Taiwan
Poster Permission Withheld
A subset of benign meningiomas may show early progression/recurrence (P/R) after surgery. In clinical practice, one of the main challenges in the treatment of meningiomas is to determine factors that correlate with P/R. This study investigated the role of radiomics and machine learning for the prediction of P/R in meningiomas. 128 patients diagnosed with WHO grade I meningioma were studied. Total 214 descriptors were extracted from the various MR sequences. The prediction accuracy of P/R was 74% and the AUC of the prediction model was 0.80.
|
|
1908.
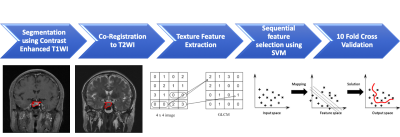 |
Radiomics Approach for Prediction of Recurrence in Pituitary Macroadenomas
Ching-Chung Ko1,2, Kai-Ting Chang3, Yang Zhang3, Jeon-Hor Chen3,4, and Min-Ying Lydia Su3
1Department of Medical Imaging, Chi Mei Medical Center, Tainan, Taiwan, 2Department of Pharmacy, Chia Nan University of Pharmacy and Science, Tainan, Taiwan, 3Department of Radiological Sciences, University of California, Irvine, CA, United States, 4E-Da Hospital/I-Shou University, Kaohsiung, Taiwan
Poster Permission Withheld
A subset of nonfunctioning pituitary macroadenomas (NFMAs) show early progression/recurrence (P/R) after surgery. In clinical practice, one of the main challenges in the treatment of NFMAs is to determine factors that correlate with P/R. This study investigated the role of radiomics for the prediction of P/R in NFMAs. 50 patients diagnosed with benign NFMAs were studied. Totally 214 descriptors were extracted from the various MR sequences. The prediction accuracy of P/R was 82% and the AUC of the prediction model was 0.78.
|
|
1909.
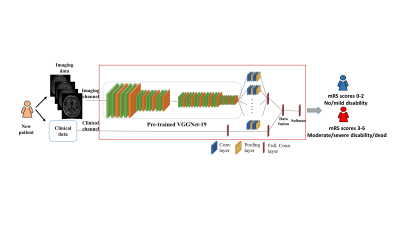 |
A Deep Transfer Learning Model to Predict Patient Outcome in ICH using the Fusion of Clinical and Fluid-Attenuated Inversion Recovery Imaging Data
Jinghua Wang1, Ming Chen2,3, Lili He2,4, Hailong Li2, Vivek Khandwala1, David Wang1, Brady Williamson1, Daniel Woo5, and Achala Vagal1
1Radiology, University of Cincinnati, Cincinnati, OH, United States, 2The Perinatal Institute and Section of Neonatology, Perinatal and Pulmonary Biology, Cincinnati Children’s Hospital Medical Center, Cincinnati, OH, United States, 3Electrical Engineering and Computer Science, University of Cincinnati, Cincinnati, OH, United States, 4Pediatrics, University of Cincinnati, Cincinnati, OH, United States, 5Neurology and Rehabilitation Medicine, University of Cincinnati, Cincinnati, OH, United States
View the Poster
Timely and reliable prognostic tools for intracerebral hemorrhage (ICH) have great potential to guide physician decision making. They are potentially useful for targeting patients for interventions and optimizing rehabilitation strategies. The objective of this study is to investigate if a deep transfer learning model can capture individual variability to predict clinical outcome for ICH patients at 3 months using the integration of clinical and T2-weighted fluid-attenuated inversion recovery (FLAIR) imaging data. Our model was able to correctly identify patients likely to have unfavorable outcomes with an AUC of 0.87 (95% confidence interval: 0.86, 0.89).
|
|
1910.
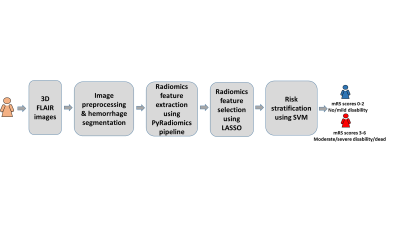 |
A machine learning model using T2-weighted FLAIR radiomics features to predict patient outcome in ICH
Jinghua Wang1, Ming Chen2,3, Lili He2,4, Hailong Li2, Vivek Khandwala1, David Wang1, Brady Williamson1, Daniel Woo5, and Achala Vagal1
1Radiology, University of Cincinnati, Cincinnati, OH, United States, 2The Perinatal Institute and Section of Neonatology, Perinatal and Pulmonary Biology, Cincinnati Children’s Hospital Medical Center, Cincinnati, OH, United States, 3Electrical Engineering and Computer Science, Cincinnati Children’s Hospital Medical Center, Cincinnati, OH, United States, 4Pediatrics, University of Cincinnati, Cincinnati, OH, United States, 5Neurology and Rehabilitation Medicine, University of Cincinnati, Cincinnati, OH, United States
View the Poster
Intracerebral hemorrhage (ICH) accounts for 10% - 30% of all strokes and is associated with high short-term mortality (≤50% at 3 month). There is a critical unmet need for an effective prognostic tool using imaging markers to identify patients at risk for poor outcome and thereby better facilitating treatments at individual level as well as tailoring personalized interventions and optimizing rehabilitation strategies. In this work, we developed a machine learning method using radiomics features derived from T2-weighted FLAIR images to predict recovery outcome in patients with ICH at 3 months with a accuracy of 80.8% (95% confidence interval: 78.9%, 82.8%).
|
|
1911.
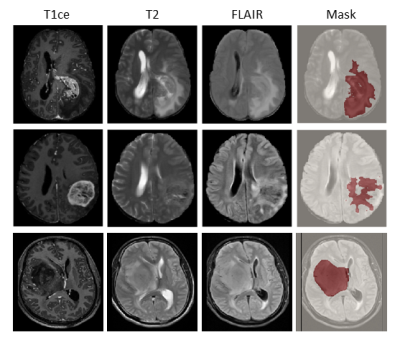 |
A radiomics signature for supratentorial extra-ventricular ependymomas on multimodal MRI
Sumeet Shinde1, Tanay Chougule1, Jitender Saini2, and Madhura Ingalhalikar1
1Symbiosis Center for Medical Image Analysis, Symbiosis International University, Pune, India, 2Department of Radiology, National Institute of Mental Health and Neurosciences, Bengaluru, India
View the Poster
High grade supratentorial ependymomas in adults are rare neoplasms with imaging features that can often be confused for high grade gliomas. However, the pathogenesis of ependymoma’s differs significantly and it therefore crucial to determine pre-operative non-invasive markers for treatment planning and optimization. Our work creates a multi-variate 3-way classification framework based on multimodal MRI radiomics to discriminate supra-tentorial ependymomas, grade III and IV gliomas. Results demonstrate high accuracy with specific textures that evolve as the top discriminative features and can be pursued for clinical applicability.
|
|
1912.
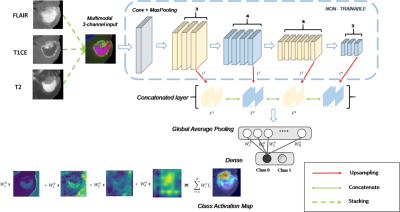 |
Validating multimodal MRI based stratification of IDH genotype using radiomics and CNNs
Madhura Ingalhalikar1, Tanay Chougule1, Sumeet Shinde1, Vani Santosh2, and Jitender Saini3
1Symbiosis Center for Medical Image Analysis, Symbiosis International University, Pune, India, 2Department of Neuropathology, National Institute of Mental Health and Neurosciences, Bangalore, India, 3Department of Radiology, National Institute of Mental Health and Neurosciences, Bangalore, India
View the Poster
Radiomics based multi-variate models and state-of-art convolutional neural networks (CNNs) have demonstrated their usefulness for predicting IDH genotype in gliomas from MRI images. However, adaptability and clinical explanability of these models on unseen multi-center datasets has not been investigated. Our work trains radiomics and CNN based classifiers on a large dataset (TCIA) and tests multiple local datasets. Results demonstrate higher adaptability of radiomics than standard CNNs, except for transfer learned CNNs. Better interpretability was obtained from feature ranking (in case of radiomics) and high resolution class activation maps (in case of CNNs).
|
|
1913.
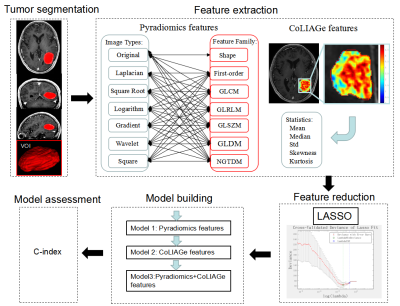 |
Combined Co-occurrence of Local Anisotropic Gradient Orientations (CoLlAGe) and Pyradiomics features to predict the prognosis of gliomas.
Xiaoxue Liu1, Baoming Li2, Jianrui Li1, Li Yu2, Jun Xu2, Guangming Lu1, and Zhiqiang Zhang1
1Department of Medical Imaging, Jinling Hospital, Nanjing University School of Medicine, Nanjing, China, 2Jiangsu Key Laboratory of Big Data Analysis Technique and CICAEET, Nanjing University of Information Science and Technology, Nanjing, China
View the Poster
We tried to establish a model based on features extracted from Pyradiomic framework and Co-occurrence of Local Anisotropic Gradient Orientations (CoLlAGe) in MRI to predict the prognosis of gliomas. Fifty-five pathologically confirmed glioma patients were retrospectively collected. All patients underwent 3DTI enhancement, standardized treatment and follow-up for overall survival. 1781 Pyradiomic features and 260 CoLlAGe features were collected. Three Cox proportional hazards models were fitted with Pyradiomics features, CoLlAGe features and Pyradiomics+CoLlAGe features. The C-index in these models were 0.835, 0.820 and 0.844, respectively. The model with Pyradiomics+CoLlAGe features demonstrated improved survival predictive performance than the single model.
|
|
1914.
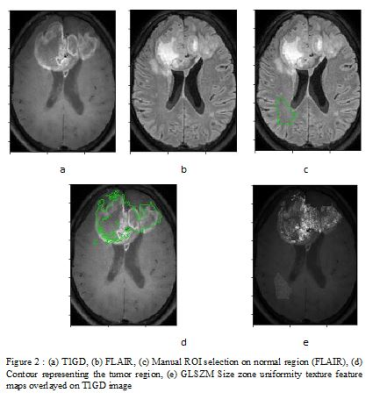 |
Identifying texture features that may serve as bio-markers of various subgroups of glioblastoma segmented using T1-perfusion MRI
Suhail Pathan Parvaze1, Mamta Gupta2, Anup Singh3, Rana Patir4, Sunita Ahlawat2, Madhura Ingalhalikar5, Neha Vats3, and Rakesh Kumar Gupta2
1Philips Innovation Campus, Bangalore, India, 2Fortis Memorial Research Institute, Gurgaon, India, 3Indian Institute of Technology, New Delhi, India, 4Department of Neurosurgery, Fortis Memorial Research Institute, Gurgaon, India, 5Symbiosis Center for Medical Image analysis, Symbiosis International University, Pune, India
View the Poster
This work aimed at identifying radiomic signatures that discriminate glioblastoma sub-regions ( enhancing tumor, non-enhancing, necrosis and edema) using DCE T1 perfusion based post contrast MRI. Results demonstrated texture features that delineate the four regions as well as separate the tumoral region from normative white matter. These radiomic signatures can be further investigated to gain deeper understanding of tumor progression and recurrence.
|
|
1915.
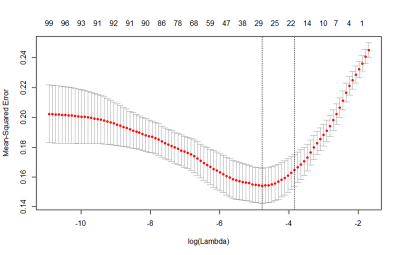 |
Radiomics biomarker analysis for differentiating glioblastoma and brain solitary metastasis from lung cancer using T2-weighted imaging
Zhe Liu1, Wenxin Xue1, Xiaotong Liu2, Ting Liang1, Chao Jin1, Xiaocheng Wei3, Buyue Qian2, and Jian Yang1
1The first affiliated Hospital of Xi’an Jiaotong University, Xi’an, China, 2Xi’an Jiaotong University, Xi’an, China, 3MR Research China, GE Healthcare,, Xi'an, China
Glioblastoma and brain solitary metastasis from lung cancer have similar peritumoral edema on T2-weighted imaging (T2WI). However, indistinguishable signs between these two tumors embarrass the radiologists and lead to high misdiagnosis rate. To address such issue, radiomics biomarkers were analyzed to detail the tumors’ histologic and morphologic characteristics. Results indicated that radiomics biomarkers including histogram of oriented gradient, shape and grey level co-occurrence matrix, which charaterize the lesion’s shape and signal showed good performance in differentiating these two tumors. Furthermore, using those radiomics biomarkers, a gradient-boosting machine learning model was established and showed good performance (Area under the curve=0.88).
|
|