Digital Poster Session
Acquisition, Reconstruction & Analysis: ML: Post Processing, Analysis, & Applications
Back to Program-at-a-Glance
|
Digital Posters Acquisition, Reconstruction & Analysis
3508 -3522 ML: Post Processing, Analysis, & Applications - Machine Learning: Image Segmentation
3523 -3536 ML: Post Processing, Analysis, & Applications - Machine Learning: Disease, Diagnosis, Pathology & Treatment
3537 -3552 ML: Post Processing, Analysis, & Applications - Machine Learning: Super-Resolution, Synthesis & Adversarial Learning
3553 -3568 ML: Post Processing, Analysis, & Applications - Machine Learning: Segmentation, Localization & Registration
3569 -3584 ML: Post Processing, Analysis, & Applications - Machine Learning: General Applications
|
|
|
3508.
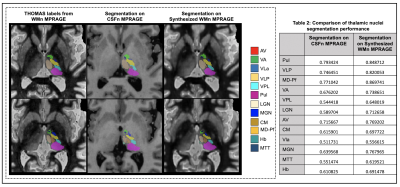 |
A Cascaded 3D CNN Approach for Thalamic Nuclei Segmentation
Lavanya Umapathy1, Mahesh Bharath Keerthivasan2,3, Natalie M Zahr4, and Manojkumar Saranathan1,2,5
1Department of Electrical and Computer Engineering, University of Arizona, Tucson, AZ, United States, 2Department of Medical Imaging, University of Arizona, Tucson, AZ, United States, 3Siemens Healthcare USA, Tucson, AZ, United States, 4Department of Psychiatry & Behavioral Sciences, Stanford University, Menlo Park, CA, United States, 5Department of Biomedical Engineering, University of Arizona, Tucson, AZ, United States
View the Poster
We propose the use of conventional MPRAGE images to synthesize WMn-MPRAGE images for thalamic nuclei segmentation. We compare thalamic nuclei segmentation performance on the synthesized WMn images to those directly on CSFn-MPRAGE. We also validate the clinical utility of our method by analyzing differences in thalamic nuclei volumes between patients with alcohol use disorder (AUD) and age-matched healthy controls using conventional MPRAGE data.
|
|
3509.
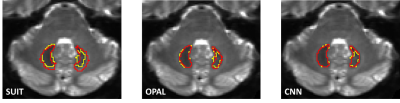 |
Automatic segmentation of dentate nuclei for microstructure assessment: application to temporal lobe epilepsy patients
Marta Gaviraghi1, Giovanni Savini2, Gloria Castellazzi1,3, Nicolò Rolandi4, Simone Sacco5,6, Egidio D’Angelo4,7, Fulvia Palesi4, Paolo Vitali2, and Claudia A.M. Gandini Wheeler-Kingshott2,4,8
1Department of Electrical, Computer and Biomedical Engineering, University of Pavia, Pavia, Italy, 2Neuroradiology Unit, Brain MRI 3T Research Center, IRCCS Mondino Foundation, Pavia, Italy, 33Queen Square MS Centre, Department of Neuroinflammation, UCL Queen Square Institute of Neurology, Faculty of Brain Sciences, University College London, London, United Kingdom, 4Department of Brain and Behavioral Sciences, University of Pavia, Pavia, Italy, 5UCSF Weill Institute for Neurosciences, Department of Neurology, University of California, San Francisco, CA, United States, 6Department of Clinical Surgical Diagnostic and Pediatric Sciences, University of Pavia, Pavia, Italy, 7Brain Connectivity Center (BCC), IRCCS Mondino Foundation, Pavia, Italy, 8Brain MRI 3T Research Center, IRCCS Mondino Foundation, Pavia, Italy
View the Poster
Dentate nuclei (DN) segmentation is necessary for assessing whether DN are affected by pathologies through quantitative analysis of parameter maps, e.g. calculated from diffusion weighted imaging (DWI). This study developed a fully automated segmentation method using non-DWI (b0) images. A Convolution Neural Network was optimised on heathy subjects’ data with high spatial resolution and was used to segment the DN of Temporal Lobe Epilepsy (TLE) patients, using standard DWI. Statistical comparison of microstructural metrics from DWI analysis, as well as volumes of each DN, revealed altered and lateralised changes in TLE patients compared to healthy controls.
|
|
3510.
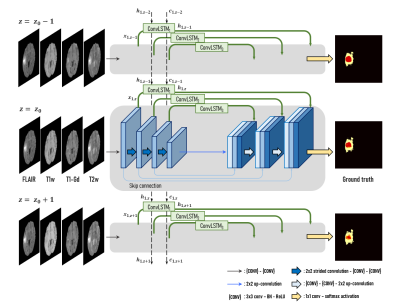 |
A deep neural network with convolutional LSTM for brain tumor segmentation in multi-contrast volumetric MRI
Namho Jeong1, Byungjai Kim1, Jongyeon Lee1, and Hyunwook Park1
1Korea Advanced Institute of Science and Technology, Daejeon, Republic of Korea
View the Poster
A medical image segmentation method is a key step in contouring of designs for radiotherapy planning and has been widely studied. In this work, we propose a method using inter-slice contexts to distinguish small objects such as tumor tissues in 3D volumetric MR images by adding recurrent neural network layers to existing 2D convolutional neural networks. It is necessary to apply a convolutional long-short term memory (ConvLSTM) since 3D volumetric data can be considered as a sequence of 2D slices. We verified through the analysis that the correlation between neighboring segmentation maps and the overall segmentation performance was improved.
|
|
3511.
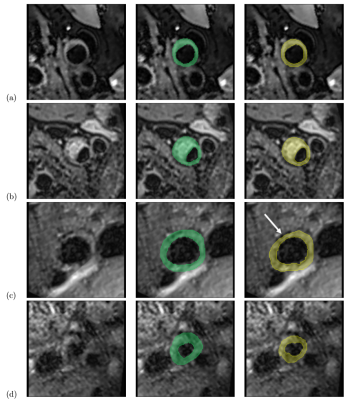 |
CNN-based segmentation of vessel lumen and wall in carotid arteries from T1-weighted MRI
Lilli Kaufhold1,2, Axel J. Krafft3, Christoph Strecker4, Markus Huellebrand1,2, Ute Ludwig3, Jürgen Hennig3, Andreas Harloff4, and Anja Hennemuth1,2
1Charité - Universitätsmedizin Berlin, Berlin, Germany, 2Fraunhofer MEVIS, Bremen, Germany, 3Department of Radiology, Medical Center - University of Freiburg, Faculty of Medicine, Freiburg, Germany, 4Department of Neurology, Medical Center – University of Freiburg, Faculty of Medicine, Freiburg, Germany
View the Poster
Internal carotid artery stenosis is a major source of ischemic stroke. Multi-contrast MRI can be used for assessing wall characteristics and plaque progression. The quantification of vessel wall morphology requires an accurate segmentation of the vessel wall. To reduce inter- and intra-observer variability, we aim to provide a fully automatic segmentation method. Our approach for segmenting the lumen and vessel wall of the extracranial carotid arteries in T1-weighted 3D MR images is based on a 2D convolutional neural network. Average dice coefficients were 0.947/0.859 for the lumen/vessel wall and the median Hausdorff-distance was below the voxel-size of 0.6mm for both.
|
|
3512.
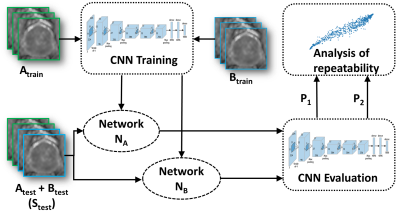 |
Test-retest repeatability of convolutional neural networks in detecting prostate cancer regions on diffusion weighted imaging in 112 patients
Amogh Hiremath1, Rakesh Shiradkar1, Harri Merisaari1,2, Prateek Prasanna1, Otta Ettala3, Pekka Taimen4, Hannu J Aronen5, Peter J Boström3, Ivan Jambor2,6, and Anant Madabhushi1
1Department of Biomedical Engineering, Case Western Reserve University, Cleveland, OH, United States, 2Department of Diagnostic Radiology, University of Turku and Turku University Hospital, Turku, Finland, 3Department of Urology, University of Turku and Turku University hospital, Turku, Finland, 4Institute of Biomedicine, University of Turku and Department of Pathology, Turku University Hospital, Turku, Finland, 5Medical Imaging Centre of Southwest Finland, Turku University Hospital, Turku, Finland, 6Department of Radiology, Icahn School of Medicine at Mount Sinai, New York, NY, United States
View the Poster
We evaluated the short-term repeatability of convolutional neural networks (CNNs) in detecting prostate cancer (PCa) using DWI collected from patients who underwent same day test-retest MRI scans. DWI was post-processed using monoexponential fit (ADCm). Two models with similar architecture were trained on test-retest scans and short-term repeatability of network predictions in terms of intra-class correlation coefficient (ICC(3,1)) was evaluated. Although the observed ICC(3,1) was high for CNN when optimized for classification performance, our results suggest that network optimization with respect to classification performance might not yield the best repeatability. Higher repeatability was observed at lower learning rates.
|
|
3513.
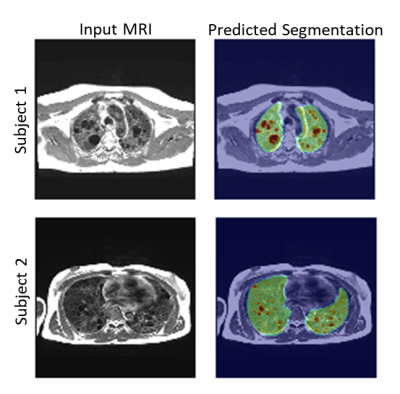 |
Automated Quantification of Lung Cysts at 0.55T MRI with Image Synthesis from CT using Deep Learning
Ipshita Bhattacharya1, Marcus Y Chen1, Joel Moss1, Adrienne Campbell-Washburn1, and Hui Xue1
1National Institutes of Health, Bethesda, MD, United States
View the Poster
We propose a novel machine learning approach for segmentation of lung cystic structures using MRI. Following our recent development on improved structural lung imaging at low-field MRI we use a combination of generative adverserial networks and modified UNet for segmentation of cyst and lung tissues. This provides a non-ionizing radiation free alternative for patients with Lymphangioleiomyomatosis who are evaluated using CT imaging. We employ cross-modality image synthesis and segmentation approaches which work synergistically to take advantage of available CT data. In this work we demonstrate the potential of MRI for quantitative analysis of cystic lung .
|
|
3514.
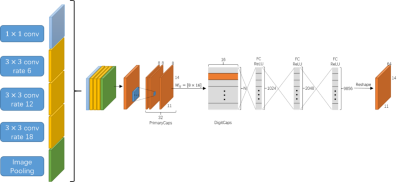 |
Multi-scale Entity Encoder-decoder Network Learning for Stroke Lesion Segmentation
Hao Yang1, Kehan Qi1, Xin Yu2, Hairong Zheng1, and Shanshan Wang1
1Paul C. Lauterbur Research Center for Biomedical Imaging, Shenzhen Inst. of Advanced Technology, Shenzhen, China, 2Case Western Reserve University, Cleveland, OH, United States
View the Poster
The encoder-decoder structure have demonstrated encouraging progress in biomedical image segmentation. Nevertheless, there are still many challenges related to the segmentation of stroke lesions, including dealing with diverse lesion locations, variations in lesion scales, and fuzzy lesion boundaries. In order to address these challenges, this paper proposes a deep neural network architecture denoted as the Multi-Scale Deep Fusion Network (MSDF-Net) with Atrous Spatial Pyramid Pooling (ASPP) for the feature extraction at different scales, and the inclusion of capsules to deal with complicated relative entities. Experimental results shows that the proposed model achieved a higher evaluating score compared to 5 models.
|
|
3515.
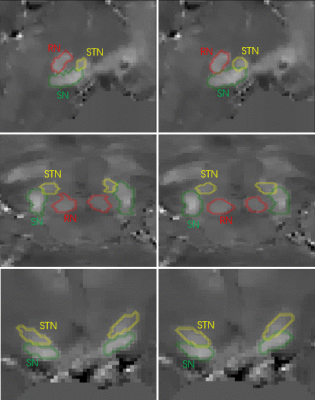 |
Automated segmentation of midbrain structures using convolutional neural network
Weiwei Zhao1, Fangfang Zhou1, Yida Wang1, Yang Song1, Gaiying Li1, Xu Yan2, Yi Wang3, Guang Yang1, and Jianqi Li1
1Shanghai Key Laboratory of Magnetic Resonance, School of Physics and Electronic Science, East China Normal University, Shanghai, China, 2MR Collaboration NE Asia, Siemens Healthcare, Shanghai, China, Shanghai, China, 3Department of Radiology, Weill Medical College of Cornell University, New York, NY, United States
View the Poster
Accurate and automated segmentation of substantia nigra (SN), the subthalamic nucleus (STN), and the red nucleus (RN) in quantitative susceptibility mapping (QSM) images has great significance in many neuroimaging studies. In the present study, we present a novel segmentation method by using convolution neural networks (CNN) to produce automated segmentations of the SN, STN, and RN. The model was validated on manual segmentations from 21 healthy subjects. Average Dice scores were 0.82±0.02 for the SN, 0.70±0.07 for the STN and 0.85±0.04 for the RN.
|
|
3516.
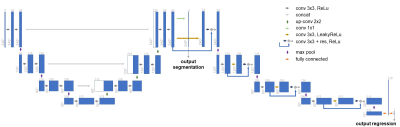 |
Multi-Task Learning: Segmentation as an auxiliary task for Survival Prediction of cancer using Deep Learning
José Maria da Silva Moreira1,2, João da Silva Santinha1,2, Thomas Varsavsky3, Carole Sudre3, Jorge Cardoso3, Mário Figueiredo2, and Nickolas Papanikolaou1
1Computational Clinical Imaging Group, Champalimaud Center for the Unknown, Lisbon, Portugal, 2Instituto de Telecomunicações, Instituto Superior Técnico, Lisbon, Portugal, 3Biomedical Engineering and Imaging Sciences, King's College London, London, United Kingdom
Watch the Video
This work presents a new method for multi-task learning that aims to increase the performance of the regression task, using the support of the segmentation task. While requiring further validation to guarantee the increase in performance, the preliminary data of this study suggests that using a ”helper” function might increase performance on the main task. In our study, a better performance of the survival prediction model was observed on the validation set when using the multi-task network, compared to a simpler single-task process.
|
|
3517.
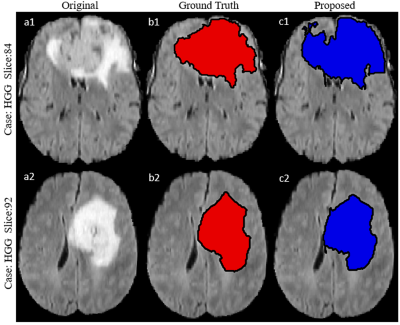 |
Segmenting Brain Tumor Lesion from 3D FLAIR MR Images using Support Vector Machine approach
Virendra Kumar Yadav1, Neha Vats1, Manish Awasthi1, Dinil Sasi1, Mamta Gupta2, Rakesh Kumar Gupta2, Sumeet Agarwal3, and Anup Singh1,4
1Center for Biomedical Engineering, Indian Institute of Technology, Delhi, India, 2Fortis Memorial Research Institute, Gurugram, India, 3Electrical Engineering, Indian Institute of Technology, Delhi, India, 4Biomedical Engineering, AIIMS, New Delhi, India
View the Poster
Segmentation of brain tumor lesion is important for diagnosis and treatment planning. Tumor tissue and edema usually appears hyperintense on fluid-attenuated-inversion-recovery (FLAIR) MR images. FLAIR images are widely used for brain tumor localization and segmentation purpose. In this study, a Support-Vector-Machine (SVM) model was developed for segmentation of FLAIR hyper-intense region semi-automatically using BraTS 2018 dataset. The proposed approach require a minimal user involvement in selecting one region around tumor in the central slice. It was observed that proposed SVM approach segmentation results shows better dice coefficient in comparison to what reported in literature.
|
|
3518.
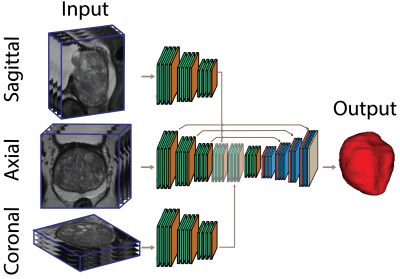 |
Anisotropic Deep Learning Multi-planar Automatic Prostate Segmentation
Tabea Riepe1, Matin Hosseinzadeh1, Patrick Brand1, and Henkjan Huisman1
1Diagnostic Image Analysis Group, Radboudumc, RadboudUMC, Nijmegen, Netherlands
View the Poster
Optimized acquisition of prostate MRI for detection of clinically significant prostate cancer requires automatic prostate segmentation. State of the art automatic prostate segmentation is performed with convolution neural networks (CNNs). Exention of our previously developed anisotropic single plane CNN to handle multi-planar input is expected to decrease segmentation problems caused by the low inter-plane resolution of t2-weighted images. Data preprocessing includes volume alignment, intensity clipping and normalization. Comparing the performance to a similar axial network, the multi-stream model shows a visually relevant improvement in prostate segmentation.
|
|
3519.
 |
Simulated CMR images can improve the performance and generalization capability of deep learning-based segmentation algorithms
Yasmina Al Khalil1, Sina Amirrajab1, Cristian Lorenz2, Jürgen Weese2, and Marcel Breeuwer1,3
1Biomedical Engineering Department, Eindhoven University of Technology, Eindhoven, Netherlands, 2Philips Research Laboratories, Hamburg, Germany, 3Philips Healthcare, MR R&D - Clinical Science, Best, Netherlands
View the Poster
The generalization capability of deep learning-based segmentation algorithms across different sites and vendors, as well as MRI data with high variance in contrast, is limited. This affects the usability of such automated segmentation algorithms in clinical settings. The lack of freely accessible medical datasets additionally limits the development of stable models. In this work, we explore the benefits of adding a simulated dataset, containing realistic contrast variance, into the training procedure of the neural network for one of the most clinically important segmentation tasks, the CMR ventricular cavity segmentation.
|
|
3520.
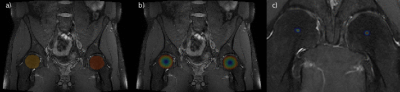 |
Region of Interest Localization in Large 3D Medical Volumes by Deep Voting
Marc Fischer1,2, Tobias Hepp3, Ulrich Plabst2, Bin Yang2, Mike Notohamiprodjo3, and Fritz Schick1
1Section on Experimental Radiology, Department of Radiology, University Hospital Tübingen, Tübingen, Germany, 2Institute of Signal Processing and System Theory, University of Stuttgart, Stuttgart, Germany, 3Diagnostic and Interventional Radiology, University Hospital Tübingen, Tübingen, Germany
View the Poster
Identifying Regions of Interest (ROI) such as anatomical landmarks, bounding boxes around organs, certain Field of Views or the selection of a particular body region is of increasing relevance for fully automated analysis pipelines of large cohort imaging data. In this work, a 3D Deep Voting approach based on recent advancements in the field of Deep Learning is proposed, which is able to locate ROIs including single points like anatomical landmarks as well as planes to identify region separators within 3D MRI and CT datasets.
|
|
3521.
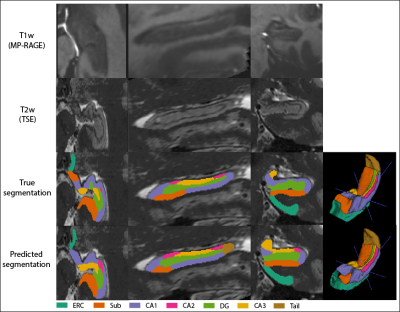 |
Multi-Contrast Hippocampal Subfield Segmentation for Ultra-High Field 7T MRI Data using Deep Learning
Daniel Ramsing Lund1,2, Mette Tøttrup Gade1,2, Tina Jensen1,2, Thomas B Shaw2, Maciej Plocharski1, Lasse Riis Østergaard1, Steffen Bollmann2, and Markus Barth2,3
1Department of Health Science and Technology, Aalborg University, Aalborg, Denmark, 2Centre for Advanced Imaging, University of Queensland, Brisbane, Australia, 3School of Information Technology and Electrical Engineering, University of Queensland, Brisbane, Australia
View the Poster
Ultra-high field 7T MRI and the utilization of multiple MRI contrasts potentially enable a superior hippocampal subfield segmentation. A residual-dense fully convolutional neural network based on U-net, including a dilated-convolutional-block was implemented for hippocampal subfield segmentation. Two data sets were combined for training and mean DSC of 0.7723 was obtained. DSC was higher for larger subfields, which were undersegmented, while smaller subfields were oversegmented. Results were comparable to the atlas-based method ASHS, while providing a substantially faster processing time.
|
|
3522.
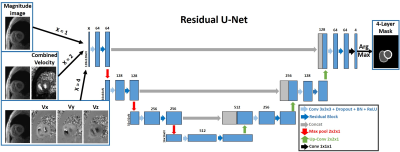 |
Automated Segmentation for Myocardial Tissue Phase Mapping Images using Deep Learning
Daming Shen1,2, Ashitha Pathrose2, Justin J Baraboo1,2, Daniel Z Gordon2, Michael J Cuttica3, James C Carr1,2,3, Michael Markl1,2, and Daniel Kim1,2
1Biomedical Engineering, Northwestern University, Evanston, IL, United States, 2Radiology, Northwestern University Feinberg School of Medicine, Chicago, IL, United States, 3Medicine, Northwestern University Feinberg School of Medicine, Chicago, IL, United States
View the Poster
Tissue phase mapping (TPM) provides regional biventricular myocardial velocities, while the slow manual segmentation process limits it use in clinic. The purpose of this study was to develop a fully automated segmentation method for TPM images with deep learning and explore the optimal method to use the magnitude and phase information.
|
|
Back to Top |
|
3523.
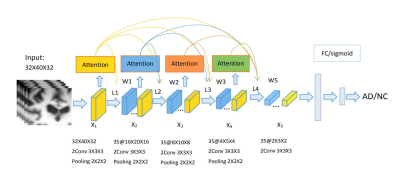 |
A 3D attention model based Recurrent Neural Network for Alzheimer’s Disease Diagnosis
Jie Zhang1,2, Xiaojing Long1, Xin Feng2, and Dong Liang1
1Shenzhen Institutes of Advanced Technology, Chinese Academy of Sciences, shenzhen, China, 2Chongqing University of Technology, ChongQing, China
The early diagnosis of AD is important for patient care and disease management. However, early diagnosis of AD is still challenging. In this work, we proposed a 3D attention model based densely connected Convolution Neural Network to learn the multilevel features of MR brain images for AD classification and prediction. The proposed network was constructed with the emphasis on the interior resource utilization and introduced the attention mechanism into the classification of AD for the first time. Our results showed that the proposed model is effective for AD classification.
|
|
3524.
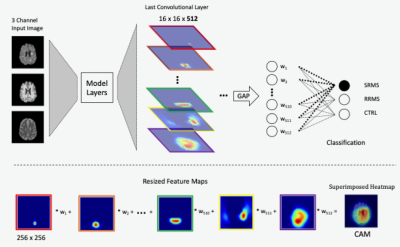 |
Class activation mapping methods for interpreting deep learning models in the classification of MRI with subtypes of multiple sclerosis
Jinseo Lee1, Daniel McClement2, Glen Pridham1, Olayinka Oladosu1, and Yunyan Zhang1
1University of Calgary, Calgary, AB, Canada, 2University of British Columbia, Vancouver, BC, Canada
As deep learning technologies continue to advance, the availability of reliable methods to accurately interpret these models is critical. Based on a trained deep learning model (VGG19) for image classification, we have shown that methods using class activation mapping (CAM) and Grad-CAM have the potential to detect the most critical MRI feature patterns associated with relapsing remitting and secondary progressive multiple sclerosis, and healthy controls, and that these patterns seem to differentiate the two continuing subtypes of MS. This can help further understand the mechanisms of disease development and discover new biomarkers for clinical use.
|
|
3525.
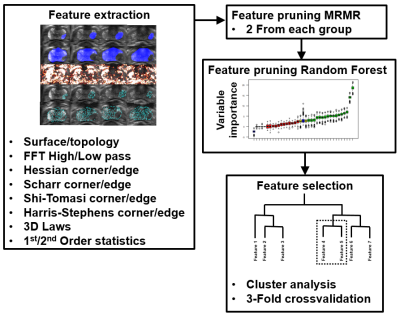 |
Prediction of prostate cancer aggressiveness using open-source machine learning tools for 5-minute prostate MRI: PRODIF CAD 1.0
Harri Merisaari1,2,3, Pekka Taimen4, Otto Ettala5, Marko Pesola1, Jani Saunavaara6, Anant Madabhushi3, Peter J Boström5, Hannu Aronen1,6, and Ivan Jambor1,7
1Department of Diagnostic Radiology, University of Turku, Turku, Finland, 2Department of Future Technologies, University of Turku, Turku, Finland, 3Department of Biomedical Engineering, Case Western Reserve University, Cleveland, OH, United States, 4Department of Pathology, Institute of Biomedicine, Turku, Finland, 5Department of Urology, Turku University Hospital, Turku, Finland, 6Medical Imaging Centre of Southwest Finland, Turku University Hospital, Turku, Finland, 7Department of Radiology, Icahn School of Medicine at Mount Sinai, New York, NY, United States
View the Poster
Acquisition time of a routine prostate MRI can be up to 20-25 minutes leading to significant financial burden on healthcare systems as the number of prostate MRI continue to increase. We developed, validated and tested an open-source radiomics/texture tools for 5-minute biparametric prostate MRI (T2-weighed imaging and DWI obtained using 4 b-values (0, 900, 1100, 2000 s/mm2)) using whole mount prostatectomy sections of 157 men with prostate cancer, PCa, (244 PCa lesions). Best features were corner detectors with AUC (clinically insignificant vs insignificant prostate cancer) in the range of 0.82-0.89. Code and data are available at: https://github.com/haanme/ProstateFeatures and http://mrc.utu.fi/data .
|
|
3526.
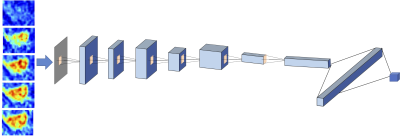 |
Differentiation of Breast Cancer Molecular Subtypes on DCE-MRI by Using Convolutional Neural Network with Transfer Learning
Yang Zhang1, Yezhi Lin1,2, Siwa Chan3, Jeon-Hor Chen1,4, Jiejie Zhou2, Daniel Chow1, Peter Chang1, Meihao Wang2, and Min-Ying Su1
1Department of Radiological Science, University of California, Irvine, CA, United States, 2Department of Radiology, First Affiliate Hospital of Wenzhou Medical University, Wenzhou, China, 3Department of Medical Imaging, Taichung Tzu-Chi Hospital, Taichung, Taiwan, 4Department of Radiology, E-Da Hospital and I-Shou University, Kaohsiung, Taiwan
View the Poster
A total of 244 patients were analyzed, 99 in Training, 83 in Testing-1 and 62 in Testing-2. Patients were classified into 3 molecular subtypes: TN, HER2+ and (HR+/HER2-). Deep learning using CNN and Convolutional Long Short Term Memory (CLSTM) were implemented. The mean accuracy in Training dataset evaluated using 10-fold cross-validation was higher using CLSTM (0.91) than CNN (0.79). When the developed model was applied to testing datasets, the accuracy was very low, 0.4-0.5. When transfer learning was applied to re-tune the model using one testing dataset, it could greatly improve accuracy in the other dataset from 0.4-0.5 to 0.8-0.9.
|
|
3527.
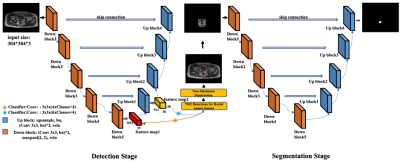 |
A two-stage deep learning method for the identification of rectal cancer lesions in MR images
Jiaxin Li1, Cheng Li2, Xiran Jiang1, Zhenkun Peng2, Chaohe Zhang3, Qiegen Liu4, and Shanshan Wang2
1China Medical University, Shenyang, China, 2Paul C. Lauterbur Research Center for Biomedical Imaging, Shenzhen Institutes of Advanced Technology, Chinese Academy of Sciences, Shenzhen, China, 3Cancer Hospital of China Medical University, Shenyang, China, 4Department of Electronic Information Engineering, Nanchang University, Nanchang, China
View the Poster
End-to-end deep learning methods, such as the well-known U-Net, have achieved great successes in biomedical image segmentation tasks. These models are often fed with the full field of view images which may contain irrelevant organs or tissues influencing the segmentation performance. In this study, targeting at the accurate segmentation of rectal cancer lesions in T1-weighted MR images, we propose a two-stage deep learning method that is composed of a detection stage and a segmentation stage. Experimental results show that under the guidance of the detected bounding boxes, better segmentation performance is achieved.
|
|
3528.
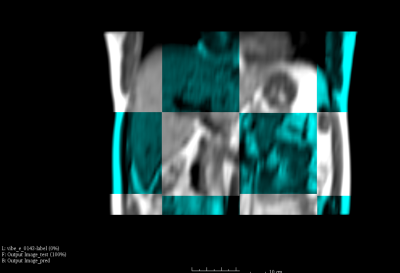 |
A Deep-Learning Based 3D Liver Motion Prediction for MR-guided-Radiotherapy
Yihang Zhou1, Jing Yuan1, Oi Lei Wong1, Kin Yin Cheung1, and Siu Ki Yu1
1Medical Physics & Research Department, Hong Kong Sanatorium & Hospital, Hong Kong, China
Respiratory induced organ motion reduces radiation delivery accuracy of radiotherapy in thorax and abdomen. MR-guided-radiotherapy (MRgRT) is capable of continuous MRI acquisition during treatment. However, the latency due to MRI acquisition and reconstruction, the detection of tumor position change, and the interaction with multileaf collimator (MLC) have been identified as the major challenges for real-time MRgRT. In this study, we proposed a deep-learning based 3D motion prediction technique to predict liver motion from volumetric dynamic MR images. Our algorithm showed promising results (< 0.3 cm prediction error on average) , suggesting its possibility of real-time motion tracking in the future MRgRT.
|
|
3529.
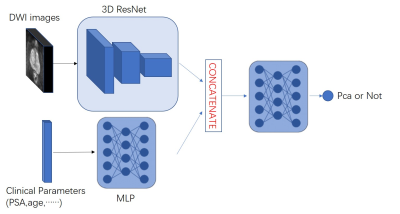 |
Combined clinical parameters and Magnetic Resonance Images for prostate cancer detection
Yi Zhu1, Rong Wei1, Ge Gao2, Jue Zhang1, and Xiaoying Wang2
1Peking University, Beijing, China, 2Peking University First Hospital, Beijing, China
View the Poster
In the wake of population aging, prostate cancer has become one of the most important diseases in elderly men. The low specificity in only image-based diagnosis may lead to unnecessary biopsies. Therefore, clinicians need to consider other variables to make diagnosis, such as age, PSA, and prostate volume. In this study we developed a novel 3D CNN model which combined clinical parameters and MR images for differentiating benign and malignant prostate lesions. The area under the receiver operating characteristics (ROC) of our proposed model (0.84) is significantly higher than that of traditional prediction model (0.71, P < 0.001).
|
|
3530.
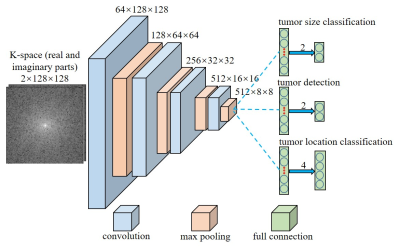 |
Direct Pathology Detection and Characterization from MR K-Space Data Using Deep Learning
Linfang Xiao1,2, Yilong Liu1,2, Peiheng Zeng1,2, Mengye Lyu1,2, Xiaodong Ma1,2, Alex T. Leong1,2, and Ed X. Wu1,2
1Laboratory of Biomedical Imaging and Signal Processing, The University of Hong Kong, Hong Kong, China, 2Department of Electrical and Electronic Engineering, The University of Hong Kong, Hong Kong, China
Watch the Video
Present MRI diagnosis comprises two steps: (i) reconstruction of multi-slice 2D or 3D images from k-space data; and (ii) pathology identification from images. In this study, we propose a strategy of direct pathology detection and characterization from MR k-space data through deep learning. This concept bypasses the traditional MR image reconstruction prior to pathology diagnosis, and presents an alternative MR diagnostic paradigm that may lead to potentially more powerful new tools for automatic and effective pathology screening, detection and characterization. Our simulation results demonstrated that this image-free strategy could detect brain tumors and their sizes/locations with high sensitivity and specificity.
|
|
3531.
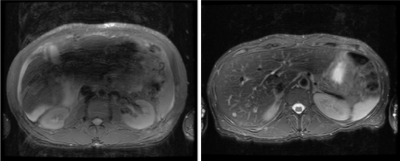 |
Analysis of Deep Learning models for Diagnostic Image Quality Assessment in Magnetic Resonance Imaging.
Jeffrey Ma1, Ukash Nakarmi1, Cedric Yue Sik Kin1, Joseph Y. Cheng1, Christopher Sandino2, Ali B Syed1, Peter Wei1, John M. Pauly2, and Shreyas S Vasanawala1
1Department of Radiology, Stanford University, Stanford, CA, United States, 2Department of Electrical Engineering, Stanford University, Stanford, CA, United States
View the Poster
In this abstract we investigate deep learning frameworks for medical image quality assessment and automatic classification of diagnostic and non-diagnostic quality images.
|
|
3532.
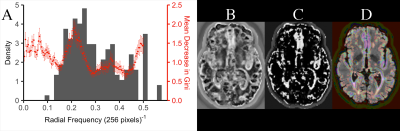 |
Utility of Stockwell Transform variants for local feature extraction from MR images: evidence from multiple sclerosis lesions
Glen Pridham1, Olayinka Oladosu2, and Yunyan Zhang1
1Department of Radiology, Department of Clinical Neurosciences, Hotchkiss Brain Institute, University of Calgary, Calgary, AB, Canada, 2Department of Neurosciences, Hotchkiss Brain Institute, University of Calgary, Calgary, AB, Canada
Watch the Video
The Stockwell Transform (ST) is an advanced local spectral feature estimator, that is prohibitively large for use in machine learning applications for typical MR images. We compared two memory-efficient variants: the Polar ST (PST) and the Discrete Orthogonal ST (DOST) as feature extraction steps in competing random forest classifiers, built to classify white matter regions-of-interest as: lesion or normal-appearing. The DOST failed to out-perform guessing, whereas the PST: out-performed guessing, and improved the accuracy of an intensity-based random forest, achieving 88.8% accuracy. We conclude that the PST can complement MR intensity, whereas the DOST may not.
|
|
3533.
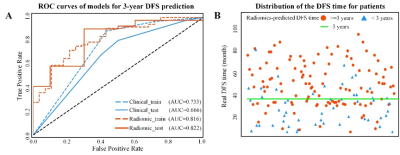 |
Association of MRI-derived radiomic biomarker with disease-free survival in patients with early-stage cervical cancer
Jin Fang1, Bin Zhang1, Shuo Wang2, and Shuixing Zhang1
1The First Affiliated Hospital of Jinan University, Guangzhou, China, 2Key Laboratory of Molecular Imaging, Institute of Automation, Chinese Academy of Sciences, Beijing, China
Radiomics is a promising methodology that automatically extracts high-dimensional features from imaging data for supplementary evaluation of prognosis. Herein, we developed radiomic signature based on pretreatment MRI, which can be used as a biomarker for risk stratification for disease-free survival (DFS) in patients with early-stage cervical cancer. This study provides a non-invasive and cost-effective preoperative predictive tool to identify the early stage cervical cancer patients with high possibility of recurrence or metastasis; and they may help to determine whether more intensive observation and aggressive treatment regimens should be administered, aim at assisting clinical treatment and healthcare decisions.
|
|
3534.
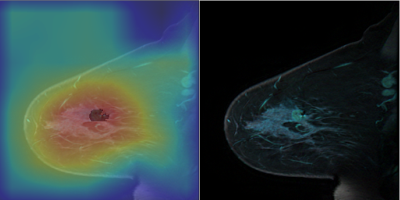 |
Weakly Supervised Exclusion of Non-Tumoral Enhancement in Low Volume Dataset for Breast Tumor Segmentation
Michael Liu1,2, Richard Ha1, Yu-Cheng Liu1, Tim Duong2, Terry Button2, Pawas Shukla1, and Sachin Jambawalikar1
1Radiology, Columbia University, New York, NY, United States, 2Stony Brook University, Stony Brook, NY, United States
Watch the Video
Quantitative measures of breast functional tumor volume are important response predictors of breast cancer undergoing chemotherapy. Automated segmentation networks have difficulty excluding non tumoral enhancing structures from their segmentations. Using a small small DCE-MRI dataset with coarse slice level labels to weakly supervised segmentation was able to exclude large portions of non tumor structures. Without manual pixel wise segmentation, our Class activation map based region proposer excluded 67% of non-tumoral voxels in a sagittal slice from downstream segmentation networks while maintaining 94% sensitivity.
|
|
3535.
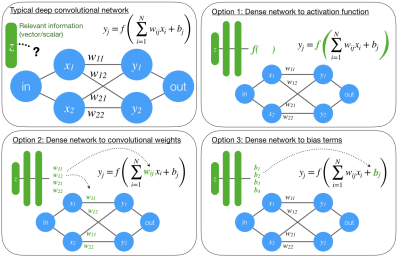 |
Informed deep convolutional neural networks
R. Marc Lebel1 and Daniel Litwiller2
1Applications and Workflow, GE Healthcare, Calgary, AB, Canada, 2Applications and Workflow, GE Healthcare, New York, NY, United States
View the Poster
Convolutional neural networks are an emerging tool in medical imaging. Conventional CNNs accept an image as input and return a task-specific output (e.g., a filtered image, a disease probability). Conventional CNNs struggle to generalize or perform poorly when image data alone is insufficient to solve the problem. We propose three ways to incorporate relevant scan information into a CNN. The value of this method is demonstrated on rSOS image denoising, a previously unstable problem.
|
|
3536.
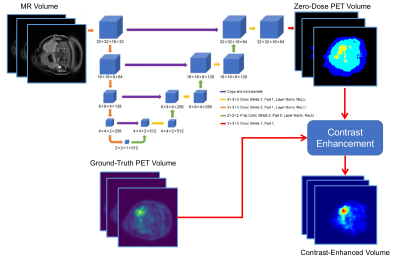 |
Deep PET-Prior: MR-derived Zero-Dose PET Prior for Differential Contrast Enhancement of PET
Abhejit Rajagopal1, Andrew P. Leynes1, Thomas Hope1, and Peder E.Z. Larson1
1Department of Radiology and Biomedical Imaging, University of California, San Francisco, San Francisco, CA, United States
Watch the Video
We introduce a deep neural network scheme for predicting FDG-PET activity from a T1-weighted MR volume. This is useful for creating realistic anatomy-conforming synthetic PET data for prototyping of PET reconstruction algorithms, e.g. from abundant MR-only exam data. While deep networks can learn the average or nominal uptake patterns, in most cases, MR is ultimately incapable of fully predicting PET activity due to fundamental differences in the sensing modalities. We show, however, that these MR-derived “zero-dose” images can aid in differential contrast enhancement and visualization of PET by localizing and highlighting activity uniquely detected by the PET radiotracers.
|
|
Back to Top |
|
3537.
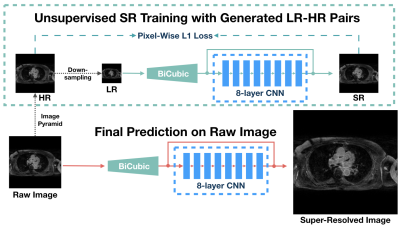 |
USR-Net: A Simple Unsupervised Single-Image Super-Resolution Method for Late Gadolinium Enhancement CMR
Jin Zhu1, Guang Yang2,3, Tom Wong2,3, Raad Mohiaddin2,3, David Firmin2,3, Jennifer Keegan2,3, and Pietro Lio1
1Department of Computer Science and Technology, University of Cambridge, Cambridge, United Kingdom, 2Cardiovascular Research Centre, Royal Brompton Hospital, London, United Kingdom, 3National Heart and Lung Institute, Imperial College London, London, United Kingdom
Watch the Video
Three-dimensional late gadolinium enhanced (LGE) CMR plays an important role in scar tissue detection in patients with atrial fibrillation. Although high spatial resolution and contiguous coverage lead to a better visualization of the thin-walled left atrium and scar tissues, markedly prolonged scanning time is required for spatial resolution improvement. In this paper, we propose a convolutional neural network based unsupervised super-resolution method, namely USR-Net, to increase the apparent spatial resolution of 3D LGE data without increasing the scanning time. Our USR-Net can achieve robust and comparable performance with state-of-the-art supervised methods which require a large amount of additional training images.
|
|
3538.
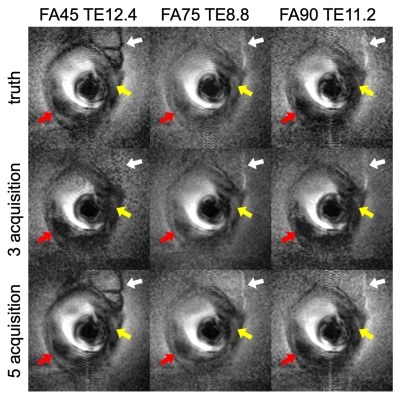 |
Empirically-Trained Image Contrast Synthesis for Intravascular MRI Endoscopy
Xiaoyang Liu1,2 and Paul Bottomley1,2
1Department of Electrical and Computer Engineering, Johns Hopkins University, Baltimore, MD, United States, 2MR Research Division, Department of Radiology, Johns Hopkins University, Baltimore, MD, United States
View the Poster
Parameterized image synthesis is desirable for region-specific image contrast and protocol optimization. While this could arguably be performed with quantitative mapping and Bloch equation simulations, it is not well-suited for real-time cine MRI or MRI endoscopy in a real-time interventional application with views changing from frame-to-frame. Here we propose to use empirical data to calibrate signal behavior patterns from a specific MRI endoscopic device and pulse sequences to fit new acquisitions from which extra images and ultimately guide automated local contrast optimization.
|
|
3539.
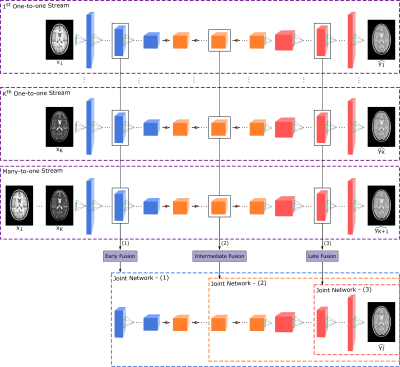 |
A Multi-Stream GAN Approach for Multi-Contrast MRI Synthesis
Mahmut Yurt1,2, Salman Ul Hassan Dar1,2, Aykut Erdem3, Erkut Erdem3, and Tolga Çukur1,2,4
1Department of Electrical and Electronics Engineering, Bilkent University, Ankara, Turkey, 2National Magnetic Resonance Research Center, Bilkent University, Ankara, Turkey, 3Department of Computer Engineering, Hacettepe University, Ankara, Turkey, 4Neuroscience Program, Sabuncu Brain Research Center, Bilkent University, Ankara, Turkey
Watch the Video
For synthesis of a single target contrast within a multi-contrast MRI protocol, current approaches perform either one-to-one or many-to-one mapping. One-to-one methods take as input a single source contrast and learn representations sensitive to unique features of the given source. Meanwhile, many-to-one methods take as input multiple source contrasts and learn joint representations sensitive to shared features across sources. For enhanced synthesis, we propose a novel multi-stream generative adversarial network model that adaptively integrates information across the sources via multiple one-to-one streams and a many-to-one stream. Demonstrations on neuroimaging datasets indicate superior performance of the proposed method against state-of-the-art methods.
|
|
3540.
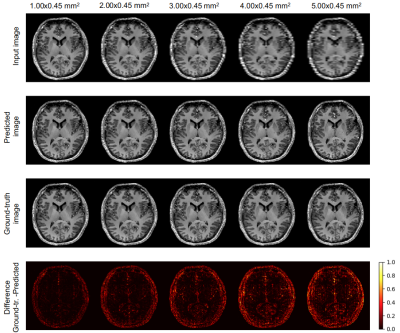 |
Super-Resolution with Conditional-GAN for MR Brain Images
Alessandro Sciarra1,2, Max Dünnwald1,3, Hendrik Mattern2, Oliver Speck2,4,5,6, and Steffen Oeltze-Jafra1,4
1MedDigit, Department of Neurology, Medical Faculty, Otto von Guericke University, Magdeburg, Germany, 2BMMR, Biomedical Magnetic Resonance, Otto von Guericke University, Magdeburg, Germany, 3Faculty of Computer Science, Otto von Guericke University, Magdeburg, Germany, 4Center for Behavioral Brain Sciences (CBBS), Magdeburg, Germany, 5German Center for Neurodegenerative Disease, Magdeburg, Germany, 6Leibniz Institute for Neurobiology, Magdeburg, Germany
View the Poster
In clinical routine acquisitions, the resolution in the slice direction is often worse than the in-plane resolution. Super-resolution techniques can help to retrieve the lack of information. Employing a conditional generative adversarial network (c-GAN), known as pix2pix for T1-w brain images, we were able to reconstruct downsampled images till 10-fold. The neural network was compared with the traditional bivariate interpolation method, and the results show that pix2pix is a valid alternative.
|
|
3541.
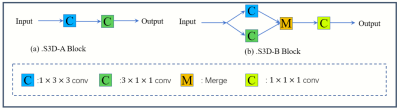 |
A Lightweight deep learning network for MR image super-resolution using separable 3D convolutional neural networks
Li Huang1, Xueming Zou1,2,3, and Tao Zhang1,2,3
1School of Life Science and Technology, University of Electronic Science and Technology of China, Chengdu, China, 2Key Laboratory for Neuroinformation, Ministry of Education, Chengdu, China, 3High Field Magnetic Resonance Brain Imaging Laboratory of Sichuan, Chengdu, China
The existing deep learning networks for MR super-resolution image reconstruction using standard 3D convolutional neural networks typically require a huge amount of parameters and thus excessive computational complexity. This has restricted the development of deeper neural networks for better performance. Here we propose a lightweight separable 3D convolution neural network for MR image super-resolution. Results show that our method can not only greatly reduce the amount of parameters and computational complexity but also improve the performance of image super-resolution.
|
|
3542.
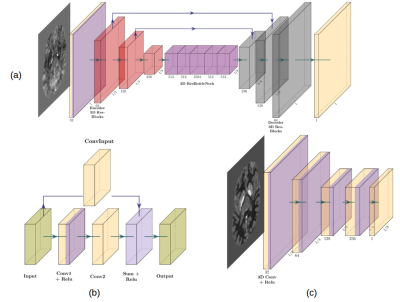 |
QSMResGAN - Dipole inversion for quantitative susceptibilitymapping using conditional Generative Adversarial Networks
Francesco Cognolato1,2, Steffen Bollmann1,3, and Markus Barth1,3
1The University of Queensland, Brisbane, Australia, 2Technical University of Munich, Munich, Germany, 3ARC Training Centre for Innovation in Biomedical Imaging Technology, The University of Queensland, Brisbane, Australia
View the Poster
In our abstract we present QSMResGAN, a conditional Generative Adversarial Network (cGAN) with a novel architecture for the generator (ResUNet), trained only with simulated data of different shapes to solve the dipole inversion problem for quantitative susceptibility mapping (QSM). The network has been compared with other state-of-the-art QSM methods on the QSM challenge 2.0 and on in vivo data.
|
|
3543.
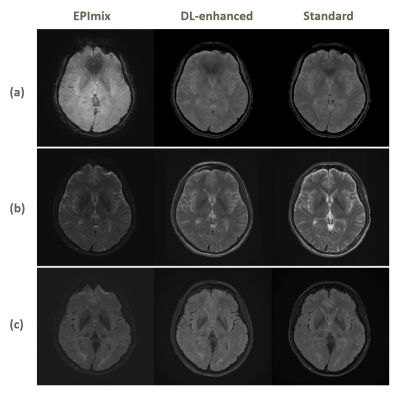 |
Enhanced One-minute EPImix Brain MRI Scans with distortion correction Based on supervised GAN model
Haining Wei1, Jiang Liu2, Enhao Gong3, Stefan Skare4, and Greg Zaharchuk5
1Tsinghua University, Beijing, China, 2The Johns Hopkins University, Baltimore, MD, United States, 3Subtle Medical Inc., Menlo Park, CA, United States, 4Karolinska Institutet, Stockholm, Sweden, 5Stanford University, Stanford, CA, United States
View the Poster
EPImix is a one-minute full brain magnetic resonance exam using a multicontrast echo-planar imaging (EPI) sequence, which can generate six contrasts at a time. However, the low resolution and signal-to-noise ratio impeded its application. And EPI distortion makes it harder to improve the quality of imaging. In this study, we applied topup for distortion correction and propose a supervised deep learning model to enhance the EPImix images. We test the GRE, T2, T2FLAIR images on network and analyze the peak signal to noise ratio and structural similarity results. The results suggest that the proposed method can effectively enhance EPImix images.
|
|
3544.
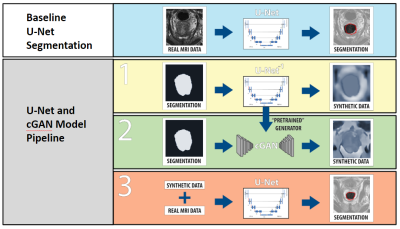 |
Data Augmentation with Conditional Generative Adversarial Networks for Improved Medical Image Segmentation
Gregory Kuling1, Matt Hemsley 1,2, Geoff Klein1, Philip Boyer3, and Marzyeh Ghassemi4
1Medical Biophysics, University of Toronto, Toronto, ON, Canada, 2Physical Sciences Platform, Sunnybrook Research Institute, Toronto, ON, Canada, 3Institute of Biomaterials and Biomedical Engineering, University of Toronto, Toronto, ON, Canada, 4Computer Science and Medicine, University of Toronto, Toronto, ON, Canada
Watch the Video
Performance of machine learning models for medical image segmentation is often hindered by a lack of labeled training data. We present a method for data augmentation wherein additional training examples are synthesized using a conditional generative adversarial network (cGAN) conditioned on a ground truth segmentation mask. The mask is later used as a label during the segmentation task. Using a dataset of N=48 T2-weighted MR volumes of the prostate, our results demonstrate the mean DSC score of a U-Net prostate segmentation model increased from 0.74 to 0.76 when synthetic training images are included with real data.
|
|
3545.
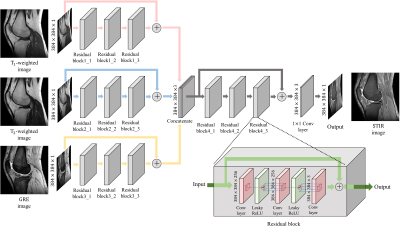 |
Multi-Contrast-Specific Objective Functions for MR Image Deep Learning - Losses for Pixelwise Error, Misregistration, and Local Variance
Hanbyol Jang1, Sewon Kim1, Jinseong Jang1, Young Han Lee2, and Dosik Hwang*1
1School of Electrical and Electronic Engineering, Yonsei University, Seoul, Republic of Korea, 2Yonsei University College of Medicine, Seoul, Republic of Korea
View the Poster
The goal of this study is to make new contrast image from multiple contrast Magnetic Resonance Image (MRI) using deep learning with loss function specialized for multiple image processing. Our contrast-conversion deep neural network (CC-DNN) is an end-to-end architecture that trains the model to create one image (STIR image) from three images (T1-weighted, T2-weighted, and GRE images). And we propose a new loss function to take into account intensity differences, misregistration, and local intensity variations.
|
|
3546.
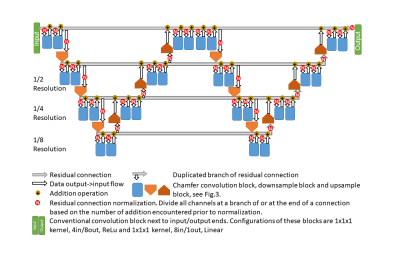 |
A Self-Regularized and Over-Determined Deep Network for Cranial Pseudo-CT Generation
Max W.K. Law1, Oilei Wong1, Jing Yuen1, and S.K. Yu1
1Hong Kong Sanatorium & Hospital, Hong Kong, Hong Kong
View the Poster
This study presented a hyperparameter-free deep network modal for cranial pseudo-CT generation. The model was potentially universal to various scanning machines without the need of network hyperparameter adjustment and could handle testing images from MR- and CT-simulators different from the training data. It is beneficial to perform clinical trial in institutions where multiple MR- and CT-machines are in operations, without supervision by deep learning experts. The proposed model was examined using training and testing datasets acquired from two sets of MR- and CT-simulators, showing promising accuracy, <79 mean-absolute-error and <170 root-mean-squared-error.
|
|
3547.
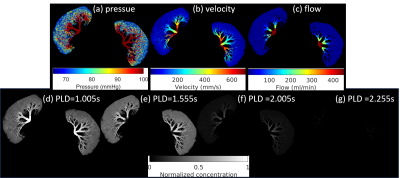 |
Deep Learning-based Perfusion Parameter Mapping (DL-PPM) with Simulated Microvascular Network Data
Liangdong Zhou1, Jinwei Zhang1,2, Qihao Zhang1,2, Pascal Spincemaille1, Thanh D Nguyen1, Yi Wang1,2, and Liangdong Zhou3
1Weill Medical School of Cornell University, New York, NY, United States, 2Cornell University, Ithaca, NY, United States, 3Radiology, Weill Medical School of Cornell University, New York, NY, United States
View the Poster
Perfusion parameters, including blood flow (BF), apparent blood velocity (V), blood volume (BV) and arterial transit time (ATT) are useful for the disgnosis of many dieases. Typically, perfusion quantification methods utilize the tracer concentration (ASL, DEC, DSC, etc.) as input and blood flow map as output. We proposed a deep learning-based perfusion parameters mapping (DL-PPM), which uses 4D time-revolved tracer concentration as input and perfusion parameters (BF, V, BV, ATT) as output. We tested the propose method using simulated data and in vivo data in kidney.
|
|
3548.
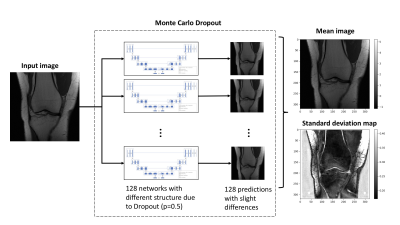 |
Visualizing intrinsic magnetic resonance imaging (MRI) dataset variations in image-space through Bayesian deep auto-encoding
Andrew P. Leynes1,2, Abhejit Rajagopal1, Valentina Pedoia1,2, and Peder E.Z. Larson1,2
1Radiology and Biomedical Imaging, University of California San Francisco, San Francisco, CA, United States, 2UC Berkeley - UC San Francisco Joint Graduate Program in Bioengineering, Berkeley and San Francisco, CA, United States
Watch the Video
We investigated the use of a Bayesian deep auto-encoder to visualize intrinsic variations within a dataset in image-space. The variations were visualized by calculating a voxel-wise standard deviation over the predictions of the Bayesian deep auto-encoder. The low mutual information that was measured between the MRI and the standard deviation maps suggests that new information is contained in the standard deviation maps. This may be useful in the training of deep learning models for anomaly detection.
|
|
3549.
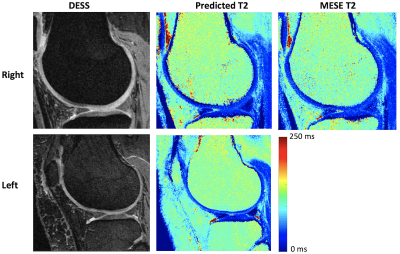 |
Predicting T2 Maps from Morphological OAI Images with an ROI-Focused GAN
Bragi Sveinsson1,2, Akshay Chaudhari3, Bo Zhu1,2, Neha Koonjoo1,2, and Matthew Rosen1,2
1Massachusetts General Hospital, Boston, MA, United States, 2Harvard Medical School, Boston, MA, United States, 3Stanford University, Stanford, CA, United States
Watch the Video
The osteoarthritis initiative (OAI) performed several morphological MRI scans on both knees of a large patient cohort, but only acquired T2 maps in the right knee of most patients. We train a conditional GAN to use the morphological scans acquired in both knees to predict the T2 map, using the acquired T2 map in the right knee as a training target. Post-training, we apply the network to predict T2 values in the left knee, without an acquired T2 map.
|
|
3550.
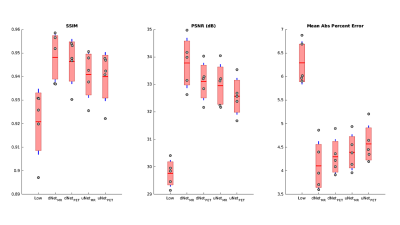 |
PET Image Denoising Using Structural MRI with a Dilated Convolutional Neural Network
Mario Serrano-Sosa1, Christine DeLorenzo1,2, and Chuan Huang1,2,3
1Biomedical Engineering, Stony Brook University, Stony Brook, NY, United States, 2Psychiatry, Stony Brook University, Stony Brook, NY, United States, 3Radiology, Stony Brook University, Stony Brook, NY, United States
View the Poster
We developed a new PET denoising model by utilizing a dilated CNN (dNet) architecture with PET/MRI inputs (dNetPET/MRI) and compared it to three other deep learning models with objective imaging metrics Structural Similarity index (SSIM), Peak signal-to-noise ratio (PSNR) and mean absolute percent error (MAPE). The dNetPET/MRI performed the best across all metrics and performed significantly better than uNetPET/MRI (pSSIM=0.0218, pPSNR=0.0034, pMAPE=0.0305). Also, dNetPET performed significantly better than uNetPET (p<0.001 for all metrics). Trend-level improvements were found across all objective metrics in networks using PET/MRI compared to PET only inputs within similar networks (dNetPET/MRI vs. dNetPET and
uNetPET/MRI vs. uNetPET).
|
|
3551.
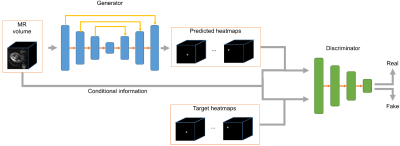 |
Fetal pose estimation from volumetric MRI using generative adversarial network
Junshen Xu1, Molin Zhang1, Esra Turk2, Polina Golland1,3, P. Ellen Grant2,4, and Elfar Adalsteinsson1,5
1Department of Electrical Engineering & Computer Science, MIT, Cambridge, MA, United States, 2Fetal-Neonatal Neuroimaging and Developmental Science Center, Boston Children’s Hospital, Boston, MA, United States, 3Computer Science and Artificial Intelligence Laboratory (CSAIL), MIT, Cambridge, MA, United States, 4Harvard Medical School, Boston, MA, United States, 5Institute for Medical Engineering and Science, MIT, Cambridge, MA, United States
Watch the Video
Estimating fetal pose from 3D MRI has a wide range of applications including fetal motion tracking and prospective motion correction. Fetal pose estimation is challenging since fetuses may have different orientation and body configuration in utero. In this work, we propose a method for fetal pose estimation from low-resolution 3D EPI MRI using generative adversarial network. Results show that the proposed method produces a more robust estimation of fetal pose and achieves higher accuracy compared with conventional convolution neural network.
|
|
3552.
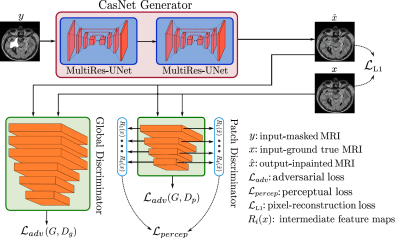 |
Adversarial Inpainting of Arbitrary shapes in Brain MRI
Karim Armanious1,2, Sherif Abdulatif1, Vijeth Kumar1, Tobias Hepp2, Bin Yang1, and Sergios Gatidis2
1University of Stuttgart, Stuttgart, Germany, 2University Hospital Tübingen, Tübingen, Germany
View the Poster
MRI suffers from incomplete information and localized deformations due to a manifold of factors. For example, metallic hip and knee replacements yield local deformities in the resultant scans. Other factors include, selective reconstruction of data and limited fields of views. In this work, we propose a new deep generative framework, referred to as IPA-MedGAN, for the inpainting of missing or complete information in brain MR. This framework aims to enhance the performance of further post-processing tasks, such as PET-MRI attenuation correction, segmentation or classification. Quantitative and qualitative comparisons were performed to illustrate the performance of the proposed framework.
|
|
Back to Top |
|
3553.
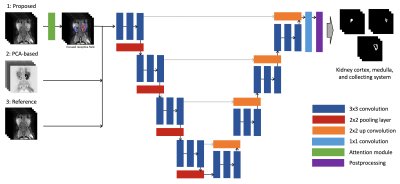 |
Attention-Based Deep Kidney Segmentation Framework for GFR Prediction
Edgar Rios Piedra1,2, Morteza Mardani1,2, and Shreyas Vasanawala1,2
1Department of Radiology, Stanford University, Stanford, CA, United States, 2Department of Electrical Engineering, Stanford University, Stanford, CA, United States
View the Poster
Automated segmentation of kidneys and their sub-components is a challenging problem, particularly in pediatric patients and in the presence of anatomical deformations or pathology. We present an improved segmentation framework using a multi-channel U-Net with added attention block that allows for the automated segmentation of the multi-phase DCE-MRI of kidneys as well as a functional evaluation of the glomerular filtration rate. Results achieve an average Dice similarity coefficient of 0.912, 0.853, and 0.917 for kidney cortex, medulla, and collector system, respectively.
|
|
3554.
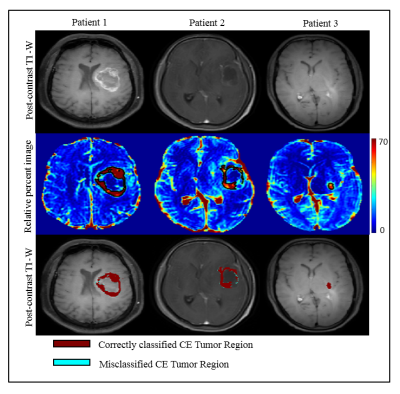 |
Segmentation of Contrast Enhancing Brain Tumor Region using a Machine Learning Framework based upon Pre and Post contrast MR Images
Neha Vats1, Virendra Kumar Yadav1, Manish Awasthi1, Dinil Sasi1, Mamta Gupta2, Rakesh Kumar Gupta2, and Anup Singh1,3
1Centre for Biomedical Engineering, Indian Institute of Technology, Delhi, New Delhi, India, 2Department of Radiology, Fortis Memorial Research Institute, Gurugram, India, 3Biomedical Engineering, AIIMS, New Delhi, India
Watch the Video
Segmentation of contrast enhancing tumor region from post-contrast T1-W MR images is sometime difficult due to low enhancement or presence of infarct tissue around or inside tumor, which exhibits similar intensity as contrast enhancement. Relative difference map obtained from pre-and post-contrast T1-weighted images can increase sensitivity to enhancement visualization as well as clearly differentiate infarct tissue from enhancing lesion. The objective of the current study was to evaluate accuracy of segmentation of contrast enhancing lesion using Support Vector Machine (SVM) classifier developed on relative difference map intensities. Optimized SVM classifier enabled accurate segmentation of contrast enhancing tumor lesion.
|
|
3555.
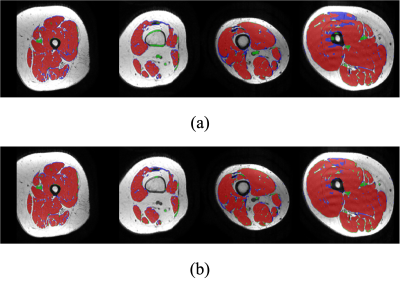 |
Attention-based Semantic Segmentation of Thigh Muscle with T1-weighted Magnetic Resonance Imaging
Zihao Tang1, Kain Kyle2, Michael H Barnett2,3, Ché Fornusek4, Weidong Cai1, and Chenyu Wang2,3
1School of Computer Science, University of Sydney, Sydney, Australia, 2Sydney Neuroimaging Analysis Centre, Sydney, Australia, 3Brain and Mind Centre, University of Sydney, Sydney, Australia, 4Discipline of Exercise and Sport Science, Faculty of Medicine and Health, University of Sydney, Sydney, Australia
View the Poster
Robust and accurate MRI-based thigh muscle segmentation is critical for the study of longitudinal muscle volume change. However, the performance of traditional approaches is limited by morphological variance and often fails to exclude intramuscular fat. We propose a novel end-to-end semantic segmentation framework to automatically generate muscle masks that exclude intramuscular fat using longitudinal T1-weighted MRI scans. The architecture of the proposed U-shaped network follows the encoder-decoder network design with integrated residual blocks and attention gates to enhance performance. The proposed approach achieves a performance comparable with human imaging experts.
|
|
3556.
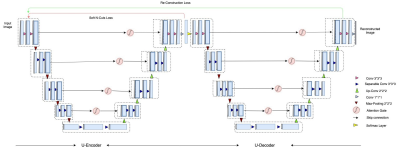 |
Unsupervised learning for Abdominal MRI Segmentation using 3D Attention W-Net
Dhanunjaya Mitta1, Soumick Chatterjee1,2,3, Oliver Speck2,4,5,6, and Andreas Nürnberger1,3
1Faculty of Computer Science, Otto von Guericke University, Magdeburg, Germany, 2Department of Biomedical Magnetic Resonance, Otto von Guericke University, Magdeburg, Germany, 3Data & Knowledge Engineering Group, Otto von Guericke University, Magdeburg, Germany, 4Center for Behavioral Sciences, Magdeburg, Germany, 5German Center for Neurodegenerative Disease, Magdeburg, Germany, 6Leibniz Insitute for Neurobiology, Magdeburg, Germany
Watch the Video
Image segmentation is a process of dividing an image into multiple coherent regions. Segmentation of biomedical images can assist diagnosis and decision making. Manual segmentation is time consuming and requires expert knowledge. One solution is to segment medical images by using deep neural networks, but traditional supervised approaches need a large amount of manually segmented training data. A possible solution for the above issues is unsupervised medical image segmentation using deep neural networks, which our work tries to solve with our proposed 3D Attention W-Net.
|
|
3557.
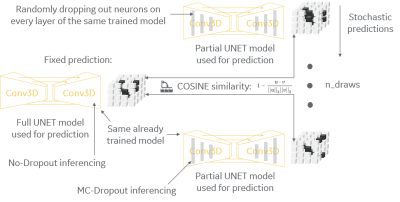 |
Model uncertainty for MRI segmentation
Andre Maximo1, Chitresh Bhushan2, Dattesh D. Shanbhag3, Radhika Madhavan2, Desmond Teck Beng yeo2, and Thomas Foo2
1GE Healthcare, Rio de Janeiro, Brazil, 2GE Research, Niskayuna, NY, United States, 3GE Healthcare, Bengaluru, India
Poster Permission Withheld
It is common practice to use dropout layers on U-net segmentation deep-learning models, and it is usually desirable to measure uncertainty of a deployed model while inferencing in clinical scenario. We present a method to convert a pre-trained model to a Bayesian model that can estimate uncertainty by posterior distribution of its trained weights. Our method uses both regular dropouts and converted Monte-Carlo dropouts to estimate uncertainty via cosine similarity of fixed and stochastic predictions. It can identify cases differing from training set by assigning high uncertainty and can be used to ask for human intervention with tough cases.
|
|
3558.
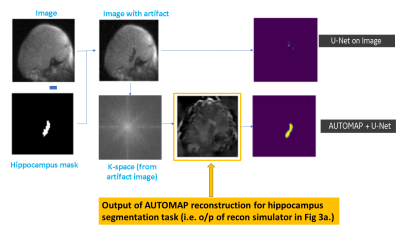 |
MRI raw k-space mapped directly to outcomes: A study of deep-learning based segmentation and classification tasks
Hariharan Ravishankar1, Chitresh Bhushan2, Arathi Sreekumari1, and Dattesh D Shanbhag1
1GE Healthcare, Bangalore, India, 2GE Global Research, Niskayuna, NY, United States
Most of the advancements with deep learning have come from mapping the reconstructed MRl images to outcomes (e.g. tumor segmentation, survival rate, pathology risk map). In this work, we present methods to arrive at critical medical imaging tasks like segmentation, classification directly from raw k-space data without image reconstruction. We specifically demonstrate that from k-space MRI data, we can perform hippocampus segmentation as well as detection of motion affected scans with similar performance to that obtained from imaging data. We also demonstrate that such an approach is more resilient to localized artifacts (e.g signal loss in hippocampus due to metal).
|
|
3559.
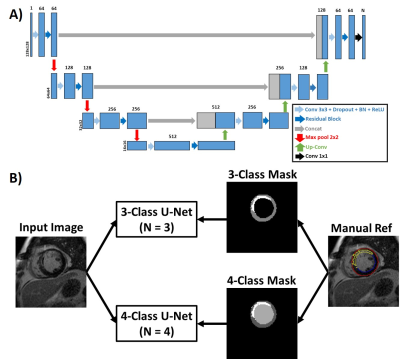 |
Automated Segmentation of Late Gadolinium Enhanced CMR with Deep Learning
Daming Shen1,2, Justin J Baraboo1,2, Brandon C Benefield3, Daniel C Lee2,3, Michael Markl1,2, and Daniel Kim1,2
1Biomedical Engineering, Northwestern University, Evanston, IL, United States, 2Radiology, Northwestern University Feinberg School of Medicine, Chicago, IL, United States, 3Feinberg Cardiovascular Research Institute, Northwestern University Feinberg School of Medicine, Chicago, IL, United States
View the Poster
Late gadolinium enhanced (LGE) CMR is the gold standard test for assessment of myocardial scarring. While quantifying scar volume is helpful to clinical decision making, its lengthy image segmentation time limits its use in practice. The purpose of this study is to enable fully automated LGE image segmentation using deep learning (DL) and explore a more efficient way of using annotation.
|
|
3560.
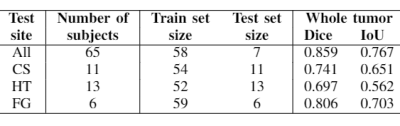 |
Addressing the need for less MRI sequence dependent DL-based segmentation methods: model generalization to multi-site and multi-scanner data
Yasmina Al Khalil1, Cristian Lorenz2, Jürgen Weese2, and Marcel Breeuwer1,3
1Biomedical Engineering Department, Eindhoven University of Technology, Eindhoven, Netherlands, 2Philips Research Laboratories, Hamburg, Germany, 3Philips Healthcare, MR R&D - Clinical Science, Best, Netherlands
View the Poster
The versatility of MRI acquisition parameters and sequences can have a substantial impact on the design and performance of medical image segmentation algorithms. Even though recent studies report excellent results of deep-learning (DL) based algorithms for tissue segmentation, their generalization capability and sequence dependence is rarely addressed, while being crucial for inclusion in clinical settings. This study attempts to demonstrate the lack of adaptation of such algorithms to unseen data from different sites and scanners. For this purpose, we use a 3D U-Net trained for brain tumor detection and test it site-wise to evaluate how well generalization can be achieved.
|
|
3561.
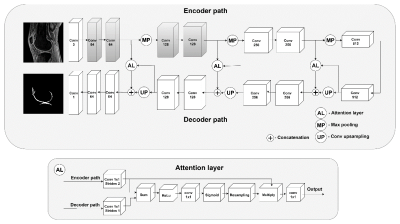 |
Whole Knee Cartilage Segmentation using Deep Convolutional Neural Networks for Quantitative 3D UTE Cones Magnetization Transfer Modeling
Yanping Xue1,2, Hyungseok Jang1, Zhenyu Cai1, Hoda Shirazian1, Mei Wu1, Michal Byra1, Yajun Ma1, Eric Y Chang1,3, and Jiang Du1
1University of California, San Diego, San Diego, CA, United States, 2Beijing Chao-Yang Hospital, Beijing, China, 3VA San Diego Healthcare System, San Diego, CA, United States
View the Poster
The existence of short T2 tissues and high ordered collagen fibers in cartilage render it “invisible” to conventional MR and sensitive to the magic angle effect. Segmentation is the first step to obtain parameters of cartilage, which is often performed manually (time-consuming and variable). Automatic segmentation and providing a biomarker that visualizes both short and long T2 tissues and insensitive to the magic angle effect is desideratum. U-Net is based on CNN to process images. The purpose of this study is to describe and evaluate the pipeline of fully-automatic segmentation of cartilage and extraction of MMF in 3D UTE-Cones-MT modeling.
|
|
3562.
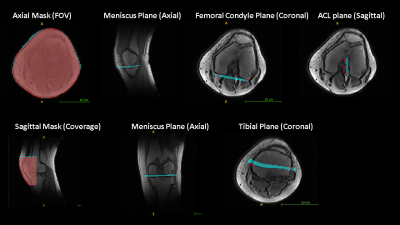 |
Intelligent Knee MRI slice placement by adapting a generalized deep learning framework
Chitresh Bhushan1, Dattesh D Shanbhag2, Andre de Alm Maximo3, Arathi Sreekumari2, Dawei Gui4, Uday Patil2, Brandon Pascual4, Rakesh Mullick2, Teck Beng Desmond Yeo1, and Thomas Foo1
1GE Global Research, Niskayuna, NY, United States, 2GE Healthcare, Bangalore, India, 3GE Healthcare, Rio de Janeiro, Brazil, 4GE Healthcare, Waukesha, WI, United States
Poster Permission Withheld
We demonstrate a deep learning-based workflow for intelligent slice placement (ISP) in MR knee imaging: meniscus plane, femoral condyle plane, tibial plane, sagittal plane and ACL plane, based on standard 2D tri-planar localizer images. We leveraged a previously described generalized architecture for ISP planning in brain, with only the training data and plane definitions adapted for knee. The mean absolute distance error between GT plane and predicted plane was < 0.5 mm for all planes except tibial plane (~ 1 mm). The results indicate the generalization of deep-learning ISP framework and its suitability for ISP in any anatomy of interest.
|
|
3563.
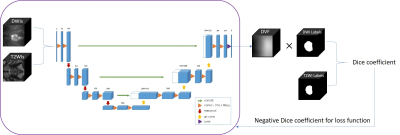 |
Fully automatic three dimensional prostate images registration: from T2-weighted-images to diffusion‐weighted images
Rong Wei1, Yi Zhu1, Xiaoying Wang2, Ge Gao2, and Jue Zhang1
1Peking University, Beijing, China, 2Peking University First Hospital, Beijing, China
View the Poster
In the wake of population aging, prostate cancer has become one of the most important diseases in western elderly men. T2-weighted-images (T2WIs) provides the best depiction of the prostate’s anatomy, and diffusion‐weighted images (DWIs) provides clues of cancer as prostate cancer appears as an area of high signal on DWIs. However, high b-value DWIs is affected by the susceptibility effects. In this paper,we describe and utilize a fully automatic 3D deep neutral network to correct misalignment between T2WIs and DWIs. The average increasement of Dice coefficient of before-and-after registration is 73% and 84%, which reflects the removal of susceptibility effects.
|
|
3564.
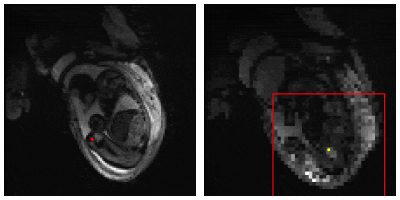 |
Landmark detection of fetal pose in volumetric MRI via deep reinforcement learning
Molin Zhang1, Junshen Xu1, Esra Turk2, Borjan Gagoski2,3, P. Ellen Grant2,3, Polina Golland1,4, and Elfar Adalsteinsson1,5
1Department of Electrical Engineering and Computer Science, Massachusetts Institute of Technology, Cambridge, MA, United States, 2Fetal-Neonatal Neuroimaging and Developmental Science Center, Boston Children’s Hospital, Boston, MA, United States, 3Harvard Medical School, Boston, MA, United States, 4Computer Science and Artificial Intelligence Laboratory (CSAIL), Massachusetts Institute of Technology, Cambridge, MA, United States, 5Institute for Medical Engineering and Science, Massachusetts Institute of Technology, Cambridge, MA, United States
Watch the Video
Fetal pose estimation could play an important role in fetal motion tracking or automatic fetal slice prescription by real-time adjustments of the prescribed imaging orientation based on fetal pose and motion patterns. In this abstract, we used a multiple image scale deep reinforcement learning method (DQN) to train an agent finding the target landmark of fetal pose by optimizing searching policy based on landmark features and its surroundings. Under an error tolerance of 15-mm, the detection accuracy reaches 58%.
|
|
3565.
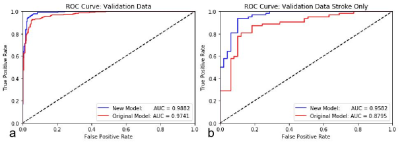 |
Retraining a Deep Learning Model to Detect Cerebral Microbleeds Using Single-Echo Stroke Data
Miller Fawaz1, Saifeng Liu1, David Utriainen1, Sean Sethi1, Zhen Wu1, and E. Mark Haacke1
1Magnetic Resonance Innovations, Inc., Bingham Farms, MI, United States
View the Poster
Automatic cerebral microbleed detection is attainable with our two step model for many disease states. We attributed previously shown lower performance in stroke data to different scenarios unique to stroke, including asymmetrically prominent cortical veins. We improved our existing pipeline for this detection by retraining the deep learning step of our model using stroke cases both in the acute and subacute stages. The results were improved performance in validation data in stroke cases as well as our previously tested data (multiple diseases). This makes our pipeline a viable and versatile real time automatic microbleed detection procedure.
|
|
3566.
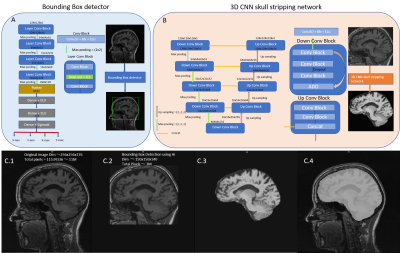 |
Retrofitting a Brain Segmentation Algorithm with Deep Learning Techniques: Validation and Experiments
Punith B Venkategowda1, Asha K Kumaraswamy1,2, Jonas Richiardi3,4,5, Sanjeev Krishnan Thampi1, Tobias Kober3,4,5, Bénédicte Maréchal3,4,5, and Ricardo A. Corredor-Jerez3,4,5
1Siemens Healthcare Pvt. Ltd., Bangalore, India, 2Vidyavardhaka College of Engineering, Mysuru, India, 3Advanced Clinical Imaging Technology, Siemens Healthcare AG, Lausanne, Switzerland, 4Department of Radiology, Centre Hospitalier Universitaire Vaudois (CHUV) and University of Lausanne (UNIL), Lausanne, Switzerland, 5Signal Processing Laboratory (LTS 5), École Polytechnique Fédérale de Lausanne (EPFL), Lausanne, Switzerland
Watch the Video
Deep learning techniques have proved their robustness in solving medical image analysis problems. This study proposes a conservative approach to benefit from the use of these methods to incrementally improve the performance of a well-established brain segmentation method. For this purpose, convolutional neural networks are trained to perform a reliable skull-stripping, based on weak labels of the original algorithm. The performance of the new pipeline is evaluated in a large cohort of dementia patients and healthy controls. The results present significant improvements in reproducibility and computation speed, while preserving accuracy and power of discrimination between groups.
|
|
3567.
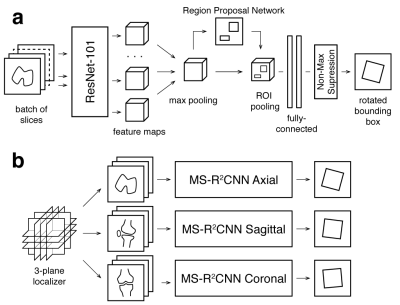 |
Oriented Object Detection Convolutional Neural Network for Automated Prescription of Oblique MRI Acquisitions
Eugene Ozhinsky1, Valentina Pedoia1, and Sharmila Majumdar1
1Radiology and Biomedical Imaging, University of California San Francisco, San Francisco, CA, United States
Watch the Video
High quality scan prescription that optimally covers the area of interest with scan planes aligned to relevant anatomical structures is crucial for error-free radiologic interpretation. The goal of this project was to develop a machine learning pipeline for oblique scan prescription that could be trained on localizer images and metadata from previously acquired MR exams. To achieve that, we have developed a novel multislice rotational region-based convolutional neural network (MS-R2CNN) architecture and evaluated it on dataset of knee MRI exams.
|
|
3568.
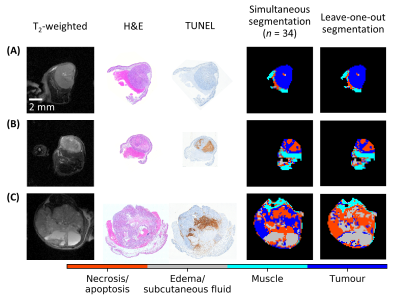 |
Segmenting Tumour Habitats Using Machine Learning and Saturation Transfer Imaging
Wilfred W Lam1, Wendy Oakden1, Elham Karami1,2,3, Margaret M Koletar1, Leedan Murray1, Stanley K Liu1,2,4, Ali Sadeghi-Naini1,2,3,4, and Greg J Stanisz1,2
1Sunnybrook Research Institute, Toronto, ON, Canada, 2University of Toronto, Toronto, ON, Canada, 3York University, Toronto, ON, Canada, 4Sunnybrook Health Sciences Centre, Toronto, ON, Canada
View the Poster
Saturation transfer-weighted images along with T1 and T2 maps at 7 T for 31 tumour xenografts in mice were used to automatically segment 1) tumour, 2) necrosis/apoptosis, 3) edema, and 4) muscle. Independent component analysis and Gaussian mixture modeling were used to segment these regions. Qualitatively excellent agreement was found between MRI and histopathology. An nine-image subset was identified that resulted in a 96% match in voxel labels compared to those found using the entire 24-image dataset. This subset had positive and negative predictive values of 96% and 97%, respectively, for tumour and 88% and 97%, respectively, for necrosis/apoptosis voxels.
|
|
Back to Top |
|
3569.
 |
No more localizers: deep learning based slice prescription directly on calibration scans
Andre de Alm Maximo1, Chitresh Bhushan2, Dawei Gui3, Uday Patil4, and Dattesh D Shanbhag4
1GE Healthcare, Rio de Janeiro, Brazil, 2GE Global Research, Niskayuna, NY, United States, 3GE Healthcare, Waukesha, WI, United States, 4GE Healthcare, Bangalore, India
Poster Permission Withheld
In this work, we demonstrate a novel automated MRI scan plane prescription workflow by making use of the pre-scan calibrations scans to generate prescription planes for knee MRI planning. Using large-FOV, low-resolution 3D calibration data, we find the meniscus plane with very-high accuracy (angle error = 0.76, distance error = 0.07 mm). The approach obviates the need to acquire any localizer images with potential benefits: (1) avoiding subsequent retakes for correct planning of plane prescription; (2) reducing total scan time; and (3) easing the MRI scanning experience for both patient and technologist by enabling single push scan.
|
|
3570.
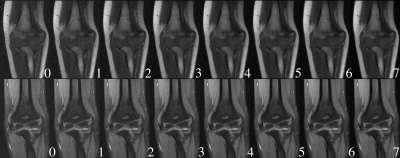 |
No-Reference Assessment of Perceptual Noise Level Defined by Human Calibration and Image Rulers
Ke Lei1, Shreyas S. Vasanawala2, and John M. Pauly1
1Electrical Engineering, Stanford University, Stanford, CA, United States, 2Radiology, Stanford University, Stanford, CA, United States
View the Poster
We propose accessing the MRI quality, perceptual noise level in particular, during a scan to stop it when the image is good enough. A convolutional neural network is trained to map an image to a perceptual score. The label score for training is a statistical estimation of error standard deviation calibrated with radiologist inputs. Image rulers for different scan types are used in the inference phase to determine a flexible classification threshold. Our proposed training and inference methods achieve a 89% classification accuracy. The same framework can be used to tune the regularization parameter for compressed-sensing reconstructions.
|
|
3571.
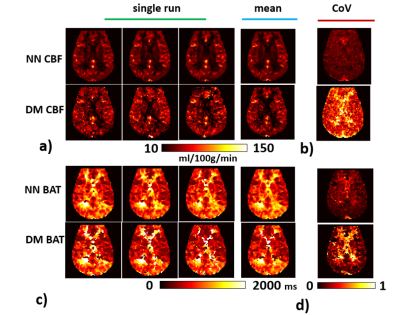 |
Whole-brain CBF and BAT mapping in 4 minutes using deep-learning-based, multi-band MR fingerprinting (MRF) ASL
Hongli Fan1,2, Pan Su1, Yang Li1, Peiying Liu1, Jay J. Pillai1,3, and Hanzhang Lu1,2,4
1The Russell H. Morgan Department of Radiology & Radiological Science, Johns Hopkins School of Medicine, Baltimore, MD, United States, 2Department of Biomedical Engineering, Johns Hopkins School of Medicine, Baltimore, MD, United States, 3Department of Neurosurgery, Johns Hopkins School of Medicine, Baltimore, MD, United States, 4F. M. Kirby Research Center for Functional Brain Imaging, Kennedy Krieger Institute, Baltimore, MD, United States
Watch the Video
Arterial-Spin-Labeling (ASL) MRI has not been used widely in clinical practice because of lower SNR and the lack of ability to resolve cerebral-blood-flow (CBF) from bolus-arrival-time (BAT) effects1. MR fingerprinting (MRF) ASL is a recently developed technique which has the potential to provide multiple parameters such as CBF, BAT, T1 and cerebral-blood-volume (CBV) in one single scan2-6. However, it still suffers from low SNR. The present work proposes a multi-band MRF-ASL in combination with deep learning, which can improve the reliability of MRF-ASL parametric maps up to 3-fold and provide whole-brain mapping of CBF and BAT in 4 minutes.
|
|
3572.
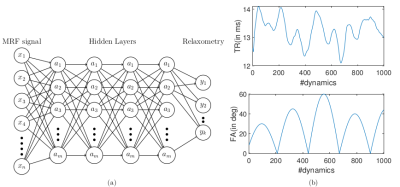 |
Deep Learning Magnetic Resonance Fingerprinting for in vivo Brain and Abdominal MRI
Peng Cao1, Di Cui1, Vince Vardhanabhuti1, and Edward S. Hui1
1Diagnostic Radiology, The University of Hong Kong, Hong Kong, China
View the Poster
We proposed a multi-layer perceptron deep learning method to achieve 100-fold acceleration for MRF quantification.
|
|
3573.
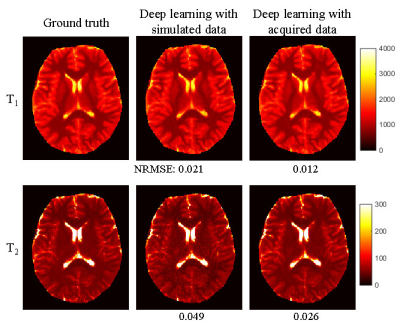 |
Dictionary-based convolutional neural network (CNN) for MR Fingerprinting with highly undersampled data
Yong Chen1,2, Zhenghan Fang3, and Weili Lin1,2
1Radiology, University of North Carolina at Chapel Hill, Chapel Hill, NC, United States, 2Biomedical Research Imaging Center, University of North Carolina at Chapel Hill, Chapel Hill, NC, United States, 3Biomedical Engineering, University of North Carolina at Chapel Hill, Chapel Hill, NC, United States
View the Poster
In this study, we proposed a framework to generate simulated training dataset to train a convolutional neural network, which can be applied to highly undersampled MR Fingerprinting images to extract quantitative tissue properties. This eliminates the necessity to acquire training dataset from multiple subjects and has the potential to enable wide applications of deep learning techniques in quantitative imaging using MR Fingerprinting.
|
|
3574.
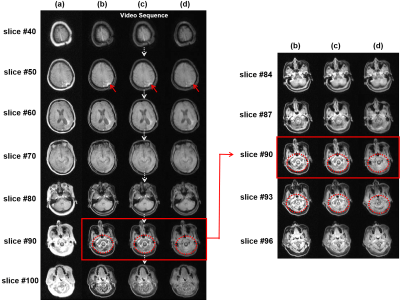 |
3D MRI Processing Using a Video Domain Transfer Deep Learning Neural Network
Jong Bum Son1, Mark D. Pagel2, and Jingfei Ma1
1Imaging Physics Department, The University of Texas MD Anderson Cancer Center, Houston, TX, United States, 2Cancer Systems Imaging Department, The University of Texas MD Anderson Cancer Center, Houston, TX, United States
Watch the Video
3D deep-learning neural networks can help ensure the slice-to-slice consistency. However, the performance of 3D networks may be degraded due to limited hardware. In this work, we developed a video domain transfer framework for 3D MRI processing to combine benefits of 2D and 3D networks with less graphical processing unit memory demands and slice-by-slice coherent outputs. Our approach consists of first translating “3D MRI images” to “a time-sequence of 2D multi-frame motion pictures,” then applying the video domain transfer to create temporally coherent multi-frame video outputs, and finally translating the output back to compose “spatially consistent volumetric MRI images.”
|
|
3575.
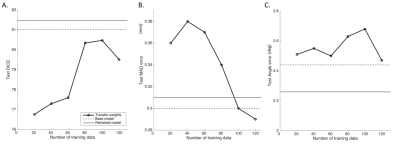 |
Transfer learning framework for knee plane prescription
Radhika Madhavan1, Andre Maximo2, Chitresh Bhushan1, Soumya Ghose1, Dattesh D Shanbhag3, Uday Patil3, Matthew Frick4, Kimberly K Amrami4, Desmond Teck Beng Yeo1, and Thomas K Foo1
1GE Global Research, Niskayuna, NY, United States, 2GE Healthcare, Rio de Janeiro, Brazil, 3GE Healthcare, Bangalore, India, 4Mayo Clinic, Rochester, MN, United States
Poster Permission Withheld
On model deployment, ideally deep learning models should be able learn continuously from new data, but data privacy concerns in medical imaging do not allow for ready sharing of training data. Retraining with incremental data generally leads to catastrophic forgetting. In this study, we evaluated the performance of a knee plane prescription model by retraining with incremental data from a new site. Increasing the number of incoming training data sets and transfer learning significantly improved test performance. We suggest that partial retraining and distributed learning frameworks may be more suitable for retraining of incremental data.
|
|
3576.
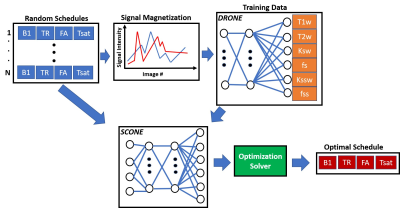 |
Deep Learning Global Schedule Optimization for Chemical Exchange Saturation Transfer MR Fingerprinting (CEST-MRF)
Or Perlman1, Christian T Farrar1, and Ouri Cohen2
1Radiology, Athinoula A. Martinos Center for Biomedical Imaging, Charlestown, MA, United States, 2Medical Physics, Memorial Sloan Kettering Cancer Center, New York, NY, United States
Watch the Video
Chemical exchange saturation transfer MR fingerprinting (CEST-MRF) enables quantification of multiple tissue parameters. Optimization of the acquisition schedule can improve tissue discrimination and reduce scan times but is highly challenging because of the large number of acquisition and tissue parameters. The goal of this work is to demonstrate a scalable deep learning based global optimization method that provides schedules with improved discrimination. The benefits of our approach are demonstrated in an in vivo mouse tumor model.
|
|
3577.
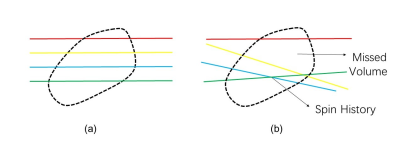 |
Fetal Brain Motion Estimation to Evaluate Motion-induced Artifacts in HASTE MRI
Yi Xiao1, Muheng Li1, Ruizhi Liao2,3, Tingyin Liu3, Junshen Xu2, Esra Turk4, Borjan Gagoski4,5, Karen Ying1, Polina Golland2,3, P.Ellen Grant4,5, and Elfar Adalsteinsson2,6
1Department of Engineering Physics, Tsinghua University, Beijing, China, 2Department of Electrical Engineering and Computer Science, Massachusetts Institute of Technology, Cambridge, MA, United States, 3Computer Science and Artificial Intelligence Laboratory (CSAIL), Massachusetts Institute of Technology, Cambridge, MA, United States, 4Fetal-Neonatal Neuroimaging and Developmental Science Center, Boston Children’s Hospital, Boston, MA, United States, 5Harvard Medical School, Boston, MA, United States, 6Institute for Medical Engineering and Science, Massachusetts Institute of Technology, Cambridge, MA, United States
View the Poster
Artifacts generated by severe and unpredictable fetal and maternal movements during MRI limit the success of imaging during pregnancy. Although modern clinical applications use single-shot imaging sequences, such as HASTE, to partially mitigate this problem, inter-slice motion artifacts are unavoidable and their impact on fetal imaging is not fully characterized. In order to analyze this problem, we exploit a large repository of volumetric EPI over long duration across many pregnant women to estimate the artifact load due to inter-slice motion on single-shot fetal brain MRI.
|
|
3578.
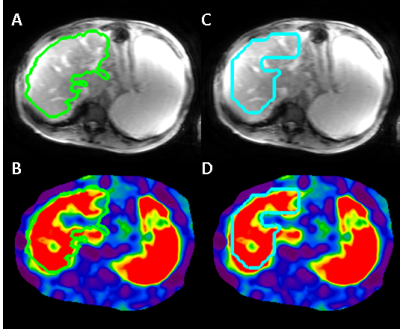 |
Magnetic Resonance Elastography Analysis using Convolutional Neural Networks
Bogdan Dzyubak1, Joel P Felmlee1, and Richard L. Ehman1
1Radiology, Mayo Clinic, Rochester, MN, United States
View the Poster
Magnetic Resonance Elastography (MRE) accurately predicts fibrosis by measuring liver stiffness. The subjectivity in human analysis poses the biggest challenge to stiffness measurement reproducibility, and also complicates the training of a neural network to automate the task. In this work, we present a CNN-based stiffness measurement tool, giving special attention to training and validation in context of reader subjectivity. Compared to an older automated tool used by our institution in a reader-verified workflow, the CNN reduces ROI failure rate by 50%, and has an excellent agreement in measured stiffness with reader-verified target ROIs.
|
|
3579.
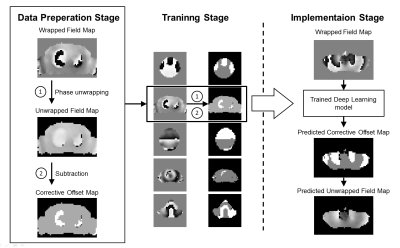 |
Rapid phase unwrapping with deep learning for shimming applications
Yuhang Shi1
1Corporate Research Institute, United Imaging Healthcare, Shanghai, China
View the Poster
The implementation of shimming relies on phase unwrapping techniques to measure the underlying field inhomogeneity. This work presents a simple and effective deep learning based phase unwrapping method to accelerate the field mapping stage for shimming applications. The method significantly reduces the compulational time and shows similar performance compared to the conventional path-following method for shimming applications.
|
|
3580.
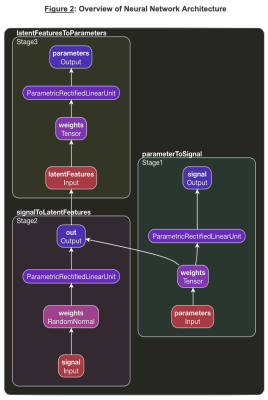 |
Deep Inversion Net: A Novel Neural Network Architecture for Rapid, and Accurate T2 Relaxometry Inversion
Jeremy Kim1, Thanh Nguyen2, Pascal Spincemaille2, and Yi Wang2
1Hunter College High School, New York, NY, United States, 2Weill Cornell Medical College, New York, NY, United States
Watch the Video
A novel deep neural network architecture, Deep Inversion Net, and a training scheme is proposed to accurately solve the multi-compartmental T2 relaxometry inverse problem for myelin water imaging in multiple sclerosis. Multiple neural networks communicate their outputs to regularize each other — thus better handling the ill-posed nature of this inverse problem. Results in simulated T2 relaxometry data and patients with demyelination show that Deep Inversion Net outperforms conventional optimization algorithms and other neural network architectures.
|
|
3581.
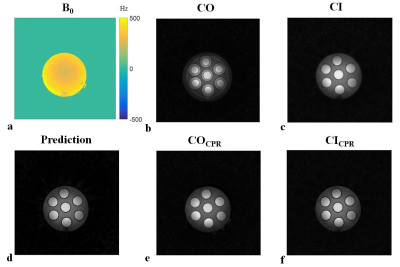 |
Learned Off-Resonance Correction for Simultaneous Radial 23Na and 1H Acquisitions at 7T
Kirsten Koolstra1, Olga Dergachyova2, Zidan Yu2,3, Andrew Webb1, and Martijn Cloos2,3
1C.J. Gorter Center for High Field MRI, Department of Radiology, Leiden University Medical Center, Leiden, Netherlands, 2Center for Advanced Imaging Innovation and Research, Department of Radiology, New York University School of Medicine, New York, NY, United States, 3Sackler Institute of Graduate Biomedical Sciences, NYU Langone Health, New York, NY, United States
View the Poster
Simultaneous proton (1H) and sodium (23Na) acquisition can provide important metabolic information. However, proton data may suffer from off-resonance artifacts due to the long dwell time required to obtain sufficient SNR for 23Na. In this work we use center outward and center inward image pairs to train a convolutional neural network that performs an off-resonance correction for the proton data without an additional measured field map.
|
|
3582.
 |
Learned Unrolled Optimization for Rapid Computation of Local RF field Enhancement Near Implants.
Peter Stijnman1,2, Cornelis van den Berg1, and Alexander Raaijmakers1,2
1Computational Imaging, UMC Utrecht, Utrecht, Netherlands, 2Biomedical Engineering, Eindhoven University of Technology, Eindhoven, Netherlands
View the Poster
The RF safety assessment of implants is a computationally demanding task. An acceleration method was presented in [1] where the local field enhancement was determined by sparse matrix inversion. In this work, we show how a model-based deep learning approach for unrolled optimization could significantly reduce the number of iterations required. The benefit of this approach is that traditional minimization is still possible afterwards, combining short computation times with high accuracy. We trained 5 iterations with 10.000 randomly generated implants. The hybrid approach finds a numerically equivalent solution in$$$\,\frac{1}{13}^{th}\,$$$of the traditional method. This approach would enable online RF safety assessment.
|
|
3583.
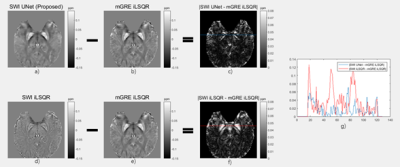 |
Reconstruction of Quantitative Susceptibility Maps from the Phase of Susceptibility-Weighted Images Using a Deep Neural Network
Jun Li1, Hongjian He1, Yi-Cheng Hsu2, and Jianhui Zhong1,3
1Center for Brain Imaging Science and Technology, Department of Biomedical Engineering, Key Laboratory for Biomedical Engineering of Ministry of Education, Zhejiang University, Hangzhou, China, 2MR Collaboration, Siemens Healthcare Ltd, Shanghai, China, 3Department of Imaging Sciences, University of Rochester, Rochester, NY, United States
View the Poster
There is a need to obtain quantitative measures of tissue susceptibility in the form of susceptibility-weighted imaging (SWI). In this study, we used a deep neural network to generate QSM maps from SWI high pass (HP)–filtered phase images. Using the QSM maps reconstructed from mGRE data by iLSQR (mGRE iLSQR) as the ground truth, the QSM maps generated from SWI HP-filtered phase images by UNet (SWI UNet) resulted in lower residuals and a better performance in quantitative metrics compared with the QSM maps reconstructed from SWI HP-filtered phase images by iLSQR (SWI iLSQR).
|
|
3584.
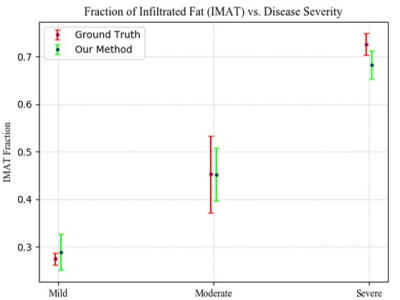 |
Quantification of Inter-Muscular Adipose Infiltration in Calf/Thigh MRI using Fully and Weakly Supervised Semantic Segmentation
Rula Amer1, Jannette Nassar1, David Bendahan2, Hayit Greenspan1, and Noam Ben-Eliezer1,3,4
1Department of Biomedical Engineering, Tel Aviv University, Tel Aviv, Israel, 2CNRS, CRMBM, Aix Marseille University, Marseille, France, 3Center for Advanced Imaging Innovation and Research, New York University, New York, NY, United States, NY, United States, 4Sagol School of Neuroscience, Tel Aviv University, Ramat-Aviv, Israel
Watch the Video
Quantification of subcutaneous fat infiltration in diseased muscle regions holds great prognostic value as it helps monitor the progression of muscular dystrophies. To estimate the infiltration, two stages are performed. The first isolates the region of muscle from the thigh/calf anatomy using U-net architecture for fully supervised segmentation. The second stage classifies muscle into diseased/healthy pixels using a weakly supervised segmentation method, incorporating a deep convolutional auto-encoder with triplet loss, and creates two clusters in the embedded feature space using k-means. The results showed a high Dice coefficient and a strong correlation between the fat infiltration and disease severity level.
|
|