Wednesday Parallel 1 Live Q&A |
Wednesday, 12 August 2020, 14:30 - 15:15 UTC |
Moderators: Mehdi Hedjazi Moghari & Shanshan Wang |
Session Number: O-23
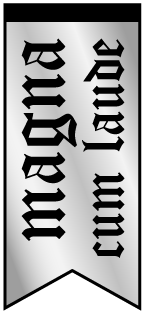 |
0774.
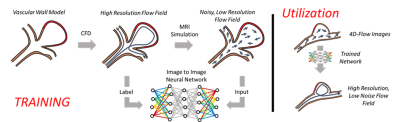 |
In-vivo application of a trained neural network using a fusion of computational fluid dynamic and 4D flow MRI data
David R Rutkowski1,2, Alejandro Roldán-Alzate1,2,3, and Kevin M Johnson1,4
1Radiology, University of Wisconsin, Madison, WI, United States, 2Mechanical Engineering, University of Wisconsin, Madison, WI, United States, 3Biomedical Engineering, University of Wisconsin, Madison, WI, United States, 4Medical Physics, University of Wisconsin, Madison, WI, United States
Watch the Video
Augmentation of 4D flow MRI data with computational fluid dynamics (CFD) -informed training networks may provide a method to produce highly accurate physiological flow fields. In this preliminary work, the potential utility of such a method was demonstrated by using high resolution patient-specific CFD data to train a neural network, and then using the trained network to enhance MRI-derived velocity fields.
|
|
0775.
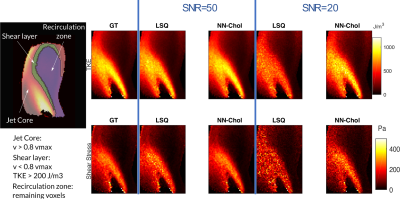 |
Improved In Vivo Estimation of the Reynolds Stress Tensor from 4D und 5D Flow MRI Using Cholesky Decomposition-Based Neural Networks
Valery Vishnevskiy1,2, Hannes Dillinger1,2, Jonas Walheim1,2, Lin Zhang1, and Sebastian Kozerke1,2
1ETH Zurich, Zurich, Switzerland, 2University of Zurich, Zurich, Switzerland
Watch the Video
A novel approach using Cholesky decomposition-based neural networks for the estimation of Reynolds stress tensors in 4D Flow MRI is presented. Evaluation is carried out for simulated MRI signals using particle tracking velocimetry data and tested on in-vivo data obtained in a healthy volunteer and a patient with bioprosthetic aortic valve. The proposed method allows to account for non-Gaussian acquisition noise and guarantees positive-definiteness of the estimated tensors, which yields 68% improvement in turbulent shear stress estimation compared to standard least squares estimation.
|
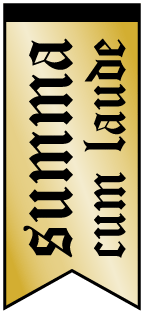 |
0776.
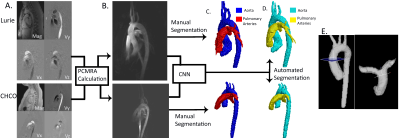 |
Fully Automated Multivendor and Multisite Artificial Intelligence-based 3D Segmentation of the Proximal Arteries from 4D flow MRI
Haben Berhane1, Michael Scott2, Takashi Fujiwara3, Lorna Browne3, Joshua Robinson1, Cynthia Rigsby1, Michael Markl2, and Alex Barker3
1Lurie Children's Hospital of Chicago, Chicago, IL, United States, 2Northwestern University, Chicago, IL, United States, 3University of Colorado, Anschutz Medical Campus, Aurora, CO, United States
Watch the Video
We trained and validated a multi-label convolutional neural network for the segmentation of the aorta and pulmonary arteries from 4D flow MRI for rapid flow analysis across multiple vendors and centers. Using 67 whole-heart 4D flow MRI scans, including 29 with cardiac pathologies, across two institutions and vendors, we trained and tested our CNN using 10-fold cross validation. For flow analysis, We calculated net flow, peak velocity, and Qp-Qs. Across all flow metrics, we found that automated segmentations showed moderate to strong agreement with the manual segmentations, while taking a fraction of the time.
|
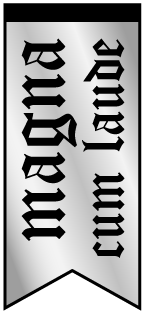 |
0777.
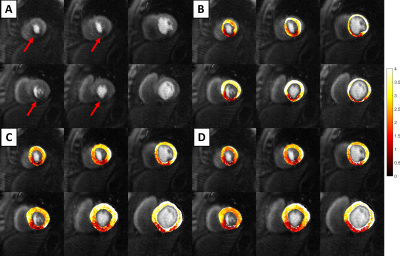 |
Automatic quantification of ultra-high resolution quantitative first-pass perfusion imaging using deep-learning based segmentation and MOCO
Matthew Van Houten1, Xue Feng1, Yang Yang2, Austin Robinson3, Craig Meyer1, and Michael Salerno1,3
1Department of Biomedical Engineering, University of Virginia, Charlottesville, VA, United States, 2Biomedical Engineering and Imaging Institute and Department of Radiology, Icahn School of Medicine at Mount Sinai, New York, NY, United States, 3Department of Medicine, University of Virginia, Charlottesville, VA, United States
Watch the Video
While quantitative first-pass quantitative perfusion imaging is an excellent non-invasive tool for the evaluation of coronary artery disease, current processing shortcomings have kept it from widespread clinical use. In this study, we developed a pipeline which robustly and automatically segments, registers, and quantifies flow with our ultra-high resolution quantitative perfusion sequence.
|
|
0778.
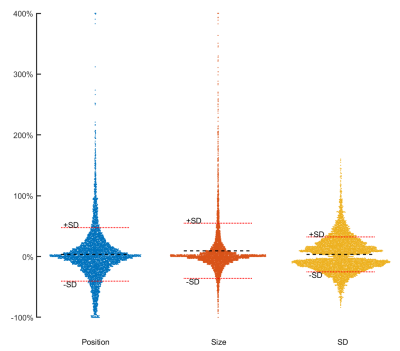 |
n-Standard deviations from remote is unreliable for scar quantification – evaluation using multicenter multivendor clinical trial data
Einar Heiberg1,2, Henrik Engblom1, Marcus Carlsson1, David Erlinge3, Dan Atar4, Anthony H Aletras1,5, and Hakan Arheden1
1Department of Clinical Sciences Lund, Clinical Physiology, Skåne University Hospital, Lund University, Lund, Sweden, 2Lund University, Wallenberg Center for Molecular Medicine, Lund, Sweden, 3Lund University, Department of Clinical Sciences Lund, Cardiology, Skåne University Hospital, Lund, Sweden, Lund, Sweden, 4Department of Cardiology, Oslo University Hospital Ullevål, and Instititute of Clinical Sciences, University of Oslo, Oslo, Norway, 5School of Medicine, Aristotele University of Thessaloniki, Laboratory of Computing, Medical Informatics and Biomedical – Imaging Technologies, Thessaloniki, Greece
Watch the Video
The purpose of this study is to systematically evaluate sources to variability in the n-SD from remote method for infarct quantification. Remote ROI position, size, and number of standard deviations all to a large extent affected infarct size. The main driver of infarct variability in the n-SD method are the differences in myocardial SD level, that varies between subjects, site and vendors. Based on the source of variability in infarct size we conclude the n-SD method lack accuracy for infarct quantification, especially in multi-center, multi-vendor setting.
|
|
0779.
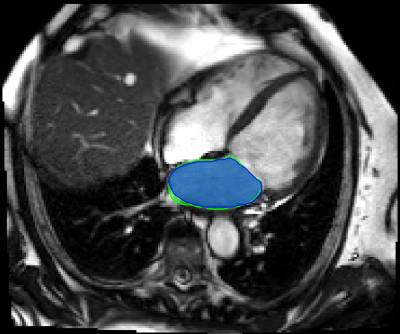 |
AI-supported Segmentation of the Whole Left Atrium in Cine MRI Identifies a New Geometrical Predictor of Outcome in Atrial Fibrillation
Maurice Pradella1, Sven Knecht2, Manuela Moor1, Shan Yang1, Constantinos Anastasopoulos1, Gian Voellmin2, Philip Haaf2, Stefan Osswald2, Michael Kuehne2, Christian Sticherling2, Bram Stieltjes1, and Jens Bremerich1
1Department for Radiology, University Hospital Basel, Basel, Switzerland, 2Department for Cardiology, University Hospital Basel, Basel, Switzerland
Watch the Video
Deep learning based, automatic segmentation of the whole left atrium in cine MRI makes detailed geometrical analysis possible by fitting of an ellipsoid into the contours of the left atrium. Therefore, we could identify the ellipsoidal volume at the time-point before atrial contraction as an independent predictor of recurrence of atrial fibrillation after catheter ablation in a multivariable analysis.
|
|
0780.
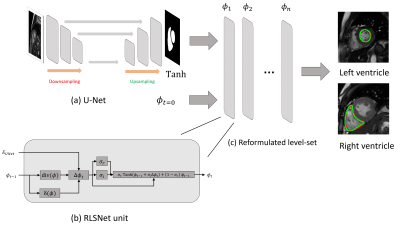 |
A level-set reformulated as deep recurrent network for left/right ventricle segmentation on cardiac cine MRI
Fan Huang1, Vince Varut Vardhanabhuti1, Pek-Lan Khong1, Ming-Yen NG1, and Peng Cao1
1Department of Diagnostic Radiology, The University of Hong Kong, Hong Kong, Hong Kong
Watch the Video
We proposed a segmentation method, which is based on a level-set reformulated via a deep recurrent network (RLSNet). This network takes the advantage of U-Net in terms of medical pattern recognition and level-set algorithm in terms of keeping the enclosed and smooth shape of the segmentation contour. We evaluate the network by the segmentation of the left and right ventricles of the heart on cardiac cine Magnetic Resonance Images, which gives greater performance than using U-Net only.
|
|
0781.
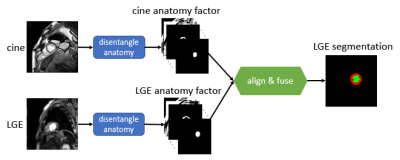 |
Stop copying contours from Cine to LGE: multimodal learning with disentangled representations needs zero annotations
Agisilaos Chartsias1, Haochuan Jiang1, Giorgos Papanastasiou2,3, Chengjia Wang2,3, Colin Stirrat2,3, Scott Semple2,3, David Newby2,3, Rohan Dharmakumar4, and Sotirios A Tsaftaris1,5
1School of Engineering, University of Edinburgh, Institute of Digital Communications, Edinburgh, United Kingdom, 2Edinburgh Imaging Facility QMRI, Edinburgh, United Kingdom, 3Centre for Cardiovascular Science, Edinburgh, United Kingdom, 4Cedars Sinai Medical Center, Los Angeles, CA, United States, 5The Alan Turing Institute, London, United Kingdom
Watch the Video
We propose a novel deep learning method, Multi-modal Spatial Disentanglement Network (MMSDNet), to segment anatomy in medical images. MMSDNet takes advantage of complementary information provided by multiple sequences of the same patient. Even when trained without annotations, it can segment anatomy (e.g., myocardium) in Late Gadolinium Enhancement (LGE) images, which is essential for assessing myocardial infarction. This is achieved by transferring knowledge from the simultaneously acquired cine-MR data where annotations are easier to be obtained. MMSDNet outperforms classical methods including non-linear registration, and simple copying of contours, as well as the state-of-the-art U-Net model.
|
|
0782.
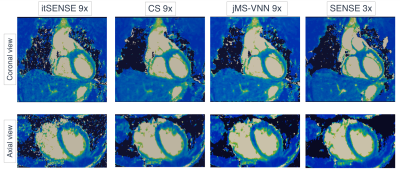 |
A Joint Multi-Scale Variational Neural Network for Accelerating Free-breathing Whole-Heart qBOOST-T2 mapping
Niccolo Fuin1, Giorgia Milotta1, Thomas Kuestner1, Aurelien Bustin1, Gastao Cruz1, Rene Botnar1,2, and Claudia Prieto1,2
1Biomedical Engineering Department, School of Biomedical Engineering and Imaging Sciences, King's College London, London, United Kingdom, 2Pontificia Universidad Católica de Chile, Santiago, Chile
Watch the Video
T2 mapping is a promising technique for the characterization of myocardial inflammation and oedema. We recently proposed a quantitative 3D whole-heart sequence (qBOOST-T2) which provides co-registered 3D high-resolution bright-blood and T2 map volumes from a single free-breathing scan. However, high-resolution qBOOST-T2 requires long scan times of ~10 min. Here we propose a joint Multi-Scale Variational Neural Network (jMS-VNN) to enable the acquisition of 3D high-resolution bright-blood and accurate T2 map volumes in ~3 mins, and their reconstruction in ~30s. The proposed jMS-VNN jointly reconstructs data from multiple contrasts and efficiently apply dictionary-based signal matching for fast T2 map generation.
|
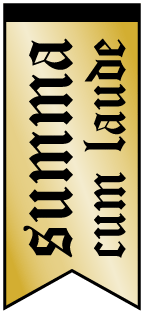 |
0783.
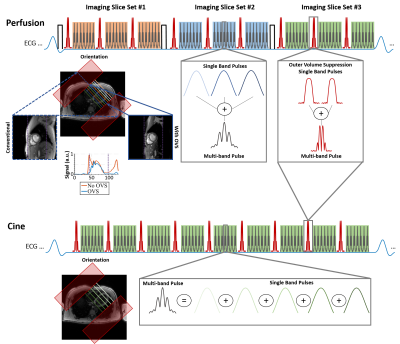 |
Improved SMS Reconstruction using ReadOut-Concatenated K-space SPIRiT (ROCK-SPIRiT)
Omer Burak Demirel1,2, Sebastian Weingärtner1,2,3, Steen Moeller2, and Mehmet Akçakaya1,2
1Electrical and Computer Engineering, University of Minnesota, Minneapolis, MN, United States, 2Center for Magnetic Resonance Research, University of Minnesota, Minneapolis, MN, United States, 3Department of Imaging Physics, Delft University of Technology, Delft, Netherlands
Watch the Video
Simultaneous Multi-slice (SMS) imaging has great potential for high acceleration rates with minimal loss in SNR. However, due to the unfavorable coil geometry only moderate acceleration rates have been achieved in cardiac applications. Outer volume suppression (OVS) has been proposed to overcome this limitation by suppressing unwanted signal from extra-cardiac tissues such as chest and back. Despite OVS, existing reconstruction techniques may suffer from residual leakage artifacts or noise amplification. In this work, we sought to extend SPIRiT to a readout-concatenated space to improve image quality in highly accelerated perfusion and cine imaging while mitigating leakage and noise amplification.
|
|