Tuesday Parallel 3 Live Q&A |
Tuesday, 11 August 2020, 14:30 - 15:15 UTC |
Moderators: Min Sun Bae & Linda Moy |
Session Number: O-26
|
0566.
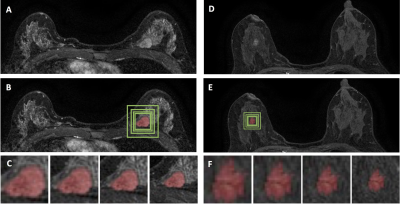 |
Diagnosis of Benign and Malignant Breast Lesions on DCE-MRI by Using Radiomics and Deep Learning with Consideration of Peri-Tumor Tissue
Jiejie Zhou1, Yang Zhang2, Kai-Ting Chang3, Kyoung Eun Lee4, Ouchen Wang1, Jiance Li1, Yezhi Lin5, Zhifang Pan5, Peter Chang3, Daniel Chow3, Meihao Wang1, and Min-Ying Su3
1First Affiliate Hospital of Wenzhou Medical University, Wenzhou, China, 2University of California, Irvine, CA, United States, 3University of California, Irvine, Irvine, CA, United States, 4Inje University Seoul Paik Hospital, Seoul, Korea, Republic of, 5Wenzhou Medical University, Wenzhou, China
Watch the Video
A total of 91 malignant/62 benign lesions were used for training, and 48 malignant/26 benign lesions for independent testing. Deep learning with ResNet50 were performed for differential diagnosis. To investigate the contribution of peri-tumor tissue, the tumor alone, smallest bounding box, and 1.2, 1.5, 2.0 times enlarged boxes were used as inputs. For per-lesion diagnosis, The accuracy was 91% for smallest bounding box, 84% for tumor alone and 1.2 times box, and further to 73% for 1.5 times box and 69% for 2.0 times box. In the independent testing dataset, the highest accuracy was 89% for the smallest bounding box.
|
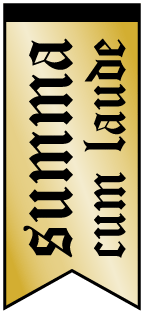 |
0567.
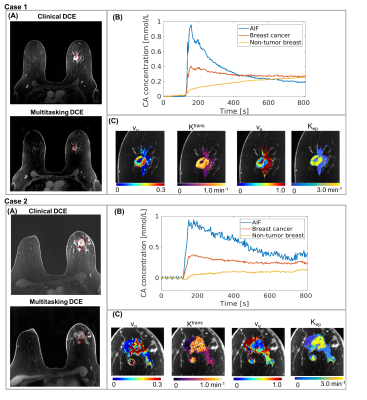 |
Low-Dose, High-Temporal-Resolution Dynamic Contrast Enhanced MRI With Dynamic T1 Mapping Using Multitasking: An Initial Study on Breast Cancer
Nan Wang1,2, Yibin Xie1, Lixia Wang1,3, Sen Ma1,2, Stephen L. Shiao4, Anthony G. Christodoulou1, and Debiao Li1,2
1Biomedical Imaging Research Institute, Cedars-Sinai Medical Center, Los Angeles, CA, United States, 2Department of Bioengineering, University of California, Los Angeles, Los Angeles, CA, United States, 3Beijing Chaoyang Hospital, Beijing, China, 4Radiation Oncology, Cedars-Sinai Medical Center, Los Angeles, CA, United States
Watch the Video
DCE MRI is a well-accepted tool in the management of breast cancer, but continues to face technical challenges and concerns regarding gadolinium deposition. In this work, we proposed a novel Multitasking DCE technique, which enables adequate breast coverage, 0.9-mm isotropic spatial resolution, 1.5-s temporal resolution, dynamic T1 mapping throughout all DCE phases, and reduced dose of 0.02mmol/kg for the imaging of breast cancer. The in vivo studies demonstrated that the low-dose Multitasking DCE showed equivalent tumor delineation compared to standard DCE. The quantitative DCE parameters were repeatable in vivo and significantly different between normal breast and breast cancer.
|
|
0568.
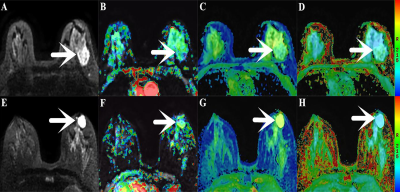 |
The Utility of Amide Proton Transfer-weighted Imaging and Diffusion Kurtosis Imaging in the Diagnosis of Benign and Malignant Breast Lesions
Nan Meng1, Xuejia Wang2, Dongming Han2, Jing Sun3, Wenling Liu2, Kaiyu Wang4, and Meiyun Wang*5
1Department of Radiology, Zhengzhou University People’s Hospital & Henan Provincial People’s Hospital, Academy of Medical Sciences, Zhengzhou University, Zhengzhou, China, 2Department of MRI, the First Affiliated Hospital of Xinxiang Medical University, Weihui, China, 3Department of Pediatrics, Zhengzhou Central Hospital, Zhengzhou University, Zhengzhou, China, 4GE Healthcare, MR Research China, Beijing, China, 5Department of Radiology, Zhengzhou University People’s Hospital & Henan Provincial People’s Hospital, Zhengzhou, China
Watch the Video
Amide proton transfer-weighted imaging (APTWI) has unique advantages in displaying the metabolism of diseased proteins. Diffusion kurtosis imaging (DKI) can quantify the diffusion state water molecules in tissues with a non-gaussian model, thus correcting the deviation of the DWI model and improving the detection of lesions. Our results show that compared with APTWI, the DKI is more effective in the diagnosis of benign and malignant breast tumors.
|
|
0569.
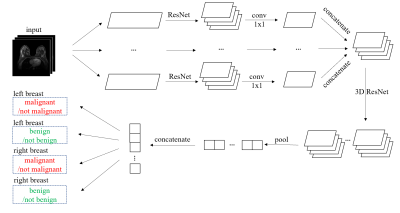 |
Large-scale classification of breast MRI exams using deep convolutional networks
Shizhan Gong1, Matthew Muckley1, Nan Wu1, Taro Makino1, Gene Kim1, Laura Heacock1, Linda Moy1, Florian Knoll1, and Krzysztof Geras1
1New York University, New York, NY, United States
Watch the Video
In this paper we trained an end-to-end classifier using a deep convolutional neural network on a large data set of 8632 3D MR exams. Our model can achieve an AUC of 0.8486 in identifying malignant cases on a test set reflecting the full spectrum of the patients who undergo the breast MRI examination. We studied the effect of the data set size and the effect of using different T1-weighted images in the series on the performance of our model. This work will serve as a guideline for optimizing future deep neural networks for breast MRI interpretation.
|
|
0570.
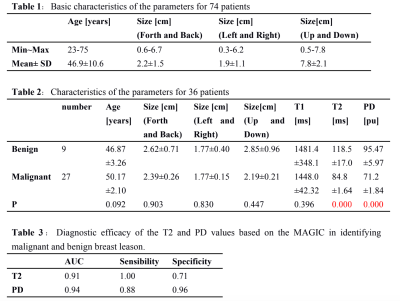 |
Differentiating benign from malignant breast lesions: a feasibility study with synthetic MRI
Weibo Gao1, Quanxin Yang1, Xin Chen1, Xiaocheng Wei2, Qiujuan Zhang1, Honghong Sun1, Xiaohui Li1, Lin Wang1, Xiao na Zhang1, Baobin Guo1, Ali Shang1, and Xiao xia Lu1
1The second affiliated hospital of xi 'an jiaotong university, xi an, China, 2MR Research, GE Healthcare, Beijing, China
In this study, we aim to investigate the feasibility of quantitative measurements obtained from magnetic resonance image compilation (MAGIC) MRI technology in the breast lesions and to further evaluate the application value of quantitative measurements in differentiating malignant from benign breast lesions. It was concluded that quantitative T2 relaxation time and PD value measured by MAGIC-MRI sequence can be applied in the breast lesions. The measured lower quantitative T2 and PD value is closely related to breast malignancy, which worth further study.
|
|
0571.
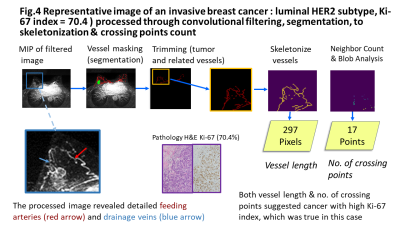 |
Quantitative Evaluation of Tumor-related Vessels on Ultrafast Dynamic Contrast-enhanced MRI: Image Biomarker of Breast Cancer Proliferation
Kango Kawase1, Masako Y Kataoka2, Tomohiro Takemura1, Takuto Fukutome1, Kojiro Yano3, Maya Honda2, Mami Iima2, Dominik Marcel Nickel4, Tatsuki Kataoka5, Masakazu Toi6, and Kaori Togashi2
1Faculty of Medicine, Kyoto University, Kyoto, Japan, 2Department of Diagnostic Imaging and Nuclear Medicine,, Kyoto University Graduate School of Medicine, Kyoto, Japan, 3Osaka Institute of Technology, Osaka, Japan, 4Siemens Healthcare GmbH, Erlangen, Germany, 5Department of Diagnostic Pathology, Kyoto University Graduate School of Medicine, Kyoto, Japan, 6Department of Breast Surgery, Kyoto University, Kyoto, Japan
Watch the Video
For quantitative evaluation of tumor-related vessels, convolution filter processing was applied to ultrafast dynamic contrast-enhanced (UF-DCE) MRI of 51 lesions to obtain vessel length and crossing count as quantitative markers. The current analysis showed that these imaging markers were associated with a subtype of invasive breast cancer, the Ki-67 index, among invasive breast cancers. This opens a new approach to the evaluation of tumor-related vessels and tumor microenvironment.
|
|
0572.
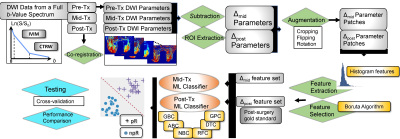 |
Early Prediction of Breast Cancer Response to Neoadjuvant Chemotherapy Using Multi-Modal Diffusion MRI with Machine-Learning
Muge Karaman1,2, Shunan Che3, Rahul Mehta1,2, Guangyu Dan1,2, Zheng Zhong1,2, Han Ouyang3, X. Joe Zhou1,4, and Xinming Zhao3
1Center for Magnetic Resonance Research, University of Illinois at Chicago, Chicago, IL, United States, 2Department of Bioengineering, University of Illinois at Chicago, Chicago, IL, United States, 3Department of Radiology, Cancer Hospital, Chinese Academy of Medical Sciences, Beijing, China, 4Departments of Radiology and Neurosurgery, University of Illinois at Chicago, Chicago, IL, United States
Watch the Video
An early imaging assessment of breast cancer’s response to neoadjuvant chemotherapy (NAC) is critical for timely planning of treatment strategies. In this study, we develop a machine-learning-based approach to investigate whether the combined features obtained from the intravoxel incoherent motion and continuous-time random-walk diffusion models provide an early prediction of pathologic response in patients receiving NAC. Our results have shown that a gradient boosting classifier trained with the early-treatment parametric changes within tumor can predict the response with an accuracy that is 96% of the accuracy achieved by using the post-treatment parametric changes.
|
|
0573.
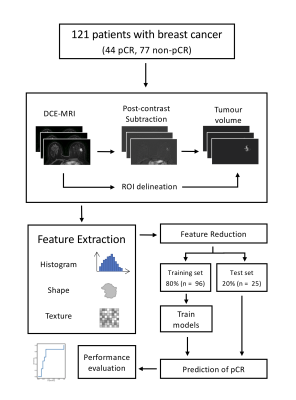 |
Predicting response to neoadjuvant chemotherapy in breast cancer: machine learning-based analysis of radiomics features from baseline DCE-MRI
Gabrielle Baxter1, Andrew J Patterson2, Leonardo Rundo1, Ramona Woitek1, Reem Bedair2, Julia Carmona-Bozo1, Roido Manavaki1, Mary A McLean3, Scott A Reid4, Martin J Graves2, and Fiona J Gilbert1
1Department of Radiology, University of Cambridge, Cambridge, United Kingdom, 2Department of Radiology, Addenbrooke's Hospital, Cambridge, United Kingdom, 3Cancer Research UK, Cambridge, United Kingdom, 4GE Healthcare, Amersham, United Kingdom
Watch the Video
This study investigated the prediction of pathological complete response (pCR) to neoadjuvant chemotherapy in breast cancer using radiomics features derived from pre-treatment DCE-MRI. 121 women with biopsy-confirmed breast cancers (44 pCR and 77 non-pCR) were imaged before treatment. 384 radiomics features were extracted from 5 post-contrast images. A logistic regression model trained on 21 of these features was able to predict pCR with an AUC of 0.78. The highest AUC (0.85) was achieved by using 7 features from only the 3rd post-contrast time point. Clinical and pathological features should be included to improve the accuracy of prediction.
|
|
0574.
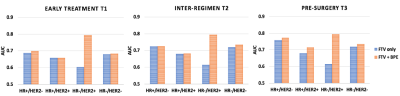 |
The additive value of quantitative contralateral background parenchymal enhancement for the prediction of residual disease in the I-SPY 2 TRIAL
Wen Li1, Natsuko Onishi1, Vignesh Arasu1, David C. Newitt1, Alex Nguyen1, Jessica Gibbs1, Lisa J. Wilmes1, Ella F. Jones1, John Kornak1, Bonnie N. Joe1, The I-SPY 2 Investigator Network2, Laura J. Esserman1, and Nola M. Hylton1
1University of California, San Francisco, San Francisco, CA, United States, 2'Quantum Leap Healthcare Collaborative, San Francisco, CA, United States
Watch the Video
This study tested the additive value of quantitative background parenchymal enhancement (BPE) assessed in the contralateral breast in the prediction of treatment response for patients with locally advanced breast cancer undergoing neoadjuvant chemotherapy (NAC). BPE predictors were added to the prediction models together with functional tumor volume predictors. Our results showed that combined model achieved better prediction for pathologic complete response in HER2-positive HR-negative cancer subtype after 3 weeks of NAC. The additive values of BPE were also observed at inter-regimen (12-week) for triple negatives, and at the pre-surgery for HR-positive subtypes.
|
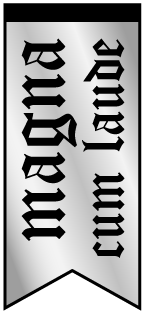 |
0575.
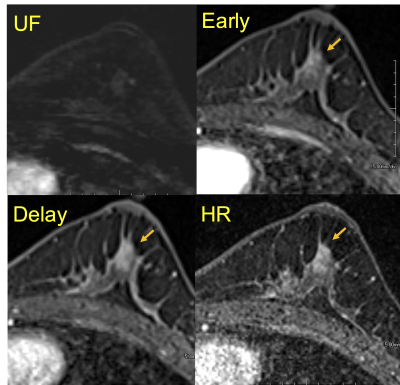 |
Post-NAC evaluation using ultrafast breast dynamic contrast-enhanced MRI
Maya Honda1, Masako Kataoka1, Mami Iima1, Kanae Kawai Miyake1, Akane Ohashi1, Ayami Ohno Kishimoto1, Rie Ota1, Marcel Dominik Nickel2, Tatsuki Kataoka3, Masakazu Toi4, and Kaori Togashi1
1Department of Diagnostic Imaging and Nuclear Medicine, Graduate School of Medicine, Kyoto University, Kyoto, Japan, 2MR Application Predevelopment, Siemens Healthcare GmbH, Erlangen, Germany, 3Department of Diagnostic pathology, Graduate School of Medicine, Kyoto University, Kyoto, Japan, 4Department of breast surgery, Graduate School of Medicine, Kyoto University, Kyoto, Japan
Watch the Video
The study evaluated the accuracy to predict pathologic complete response (pCR) after neo-adjuvant chemotherapy (NAC) using ultrafast dynamic contrast-enhanced (UF-DCE) MRI. The sensitivity to predict pCR was higher on UF-DCE MRI compared with conventional dynamic contrast-enhanced (DCE) MRI. The difference in image and pathological sizes on UF-DCE MRI was smaller than on conventional DCE MRI. UF-DCE MRI potentially assesses post-NAC status in breast cancer patients accurately in a shorter acquisition time.
|
|