Wednesday Parallel 3 Live Q&A |
Wednesday, 12 August 2020, 14:30 - 15:15 UTC |
Moderators: Eva Gombos & Frank Zoellner |
Session Number: O-32
|
0807.
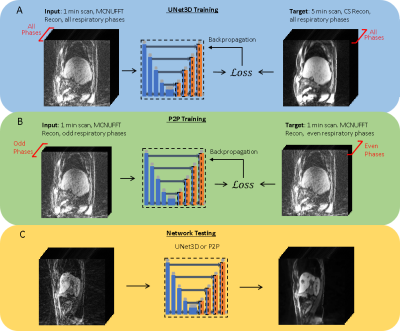 |
Phase2Phase: Reconstruction of free-breathing MRI into multiple respiratory phases using deep learning without a ground truth
Cihat Eldeniz1, Weijie Gan1, Sihao Chen1, Jiaming Liu1, Ulugbek S. Kamilov1, and Hongyu An1
1Washington University in St. Louis, Saint Louis, MO, United States
Watch the Video
Radial MRI can be used for reconstructing multiple respiratory phases with retrospective binning. However, short acquisitions suffer from significant streaking artifacts. Compressed sensing (CS)-based methods are commonly used; nevertheless, CS is computational intensive and the image quality depends on the regularization parameters. We hereby propose a deep learning method that does not need an artifact-free target during training. The method can reconstruct high-quality volumes with ten respiratory phases, even for acquisitions close to 1 minute in length. The method outperforms CS for the same acquisition duration and can yield slightly better results than Unet3D trained using a surrogate ground truth.
|
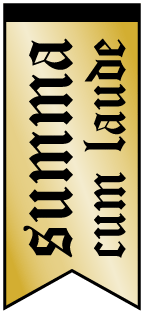 |
0808.
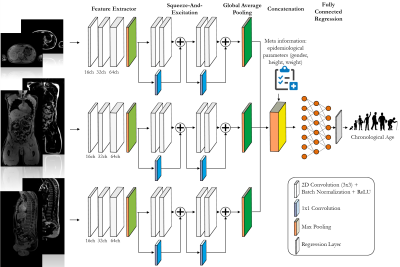 |
Age estimation from whole-body MR images: A proof-of-principle study
Thomas Küstner1,2,3, Tobias Hepp2, Karim Armanious2,3, Konstantin Nikolaou4, Sergios Gatidis2,4, and Bin Yang3
1Biomedical Engineering Department, School of Biomedical Engineering and Imaging Sciences, King's College London, London, United Kingdom, 2Medical Image and Data Analysis (MIDAS), University Hospital Tübingen, Tübingen, Germany, 3Institute of Signal Processing and System Theory, University of Stuttgart, Stuttgart, Germany, 4Department of Radiology, University Hospital Tübingen, Tübingen, Germany
Watch the Video
Age is one of the most important clinical parameters describing patients in a medical context. The chronological age (CA) does however not necessarily reflect the true underlying biological age (BA) which can depend on multiple factors such as lifestyle, social environment, medical history, genetics and ethnicity. It is therefore desirable to measure BA quantitatively and objectively. In this proof-of-principle study, we examine if CA can be estimated from whole-body MRI. We propose a novel deep learning architecture to perform an accurate CA estimation.
|
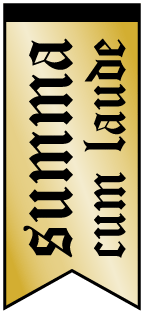 |
0809.
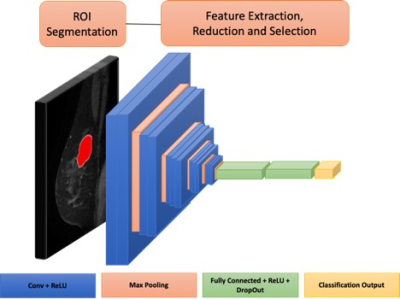 |
Transfer Learning-Based Preoperative Prediction of Lymph Node Metastasis
Renee Cattell1, Jie Ding1, Shenglan Chen1, and Chuan Huang1,2,3
1Biomedical Engineering, Stony Brook University, Stony Brook, NY, United States, 2Radiology, Stony Brook University, Stony Brook, NY, United States, 3Psychiatry, Stony Brook University, Stony Brook, NY, United States
Watch the Video
A tool to preoperatively predict sentinel lymph node status in patients with breast cancer could minimize the need for invasive surgical examination. Radiomics has been shown to have predictive power in many classification tasks. Fully automated deep learning methods would integrate more easily into clinical workflow because they do not require manual feature extraction. However, convolutional neural networks are computationally demanding and require large datasets to train. Transfer learning can be applied to allow for shortened training time and applicable to relatively small datasets.
|
|
0810.
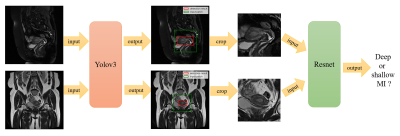 |
Deep Learning for Determination of Myometrial Invasion Depth and Automatic Lesion Identification Based on Endometrial Cancer MR Imaging
Yida Wang1, Yinqiao Yi1, Minhua Shen2, He Zhang2, Xu Yan3, and Guang Yang1
1Shanghai Key Laboratory of Magnetic Resonance, East China Normal University, Shanghai, China, 2Department of Radiology, Obstetrics and Gynecology Hospital, Fudan University, Shanghai, China, 3MR Scientific Marketing, Siemens Healthcare, Shanghai, China
Watch the Video
We proposed an deep learning approach to locate lesion and evaluate the myometrial invasion (MI) depth automatically on magnetic resonance (MR) images. Firstly, we trained a detection model based on YOLOv3 to locate lesion area on endometrial cancer MR (ECM) images. Then, the detected lesion regions on both sagittal and coronal images were simultaneously fed into a classification model based on Resnet to identify MI depth. Precision-recall curve, receiver operating characteristic curve and confusion matrix were used to evaluate the performance of the proposed method. The proposed model achieved good and time-efficient performance.
|
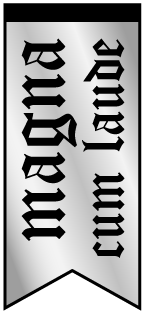 |
0811.
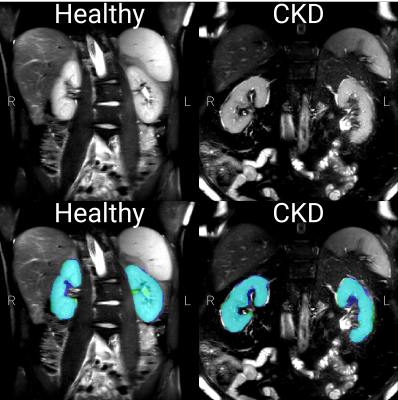 |
Automated Renal Segmentation in Healthy and Chronic Kidney Disease Subjects Using A Convolutional Neural Network
Alexander J Daniel1, Charlotte E Buchanan1, Thomas Allcock1, Daniel Scerri1, Eleanor F Cox1, Benjamin L Prestwich1, and Susan T Francis1
1Sir Peter Mansfield Imaging Centre, University of Nottingham, Nottingham, United Kingdom
Watch the Video
Manual segmentation of the kidneys in renal MRI is a time consuming process in many processing pipelines. Existing automated methods using classical imaging processing are specific to a single pathology. Here we implement a convolutional neural network for rapid and automatic segmentation of the kidneys from both a healthy control and Chronic Kidney Disease cohort. When validated on unseen data, the network achieved a mean Dice score of 0.93±0.02 with mean error in total kidney volume of 2.0±16.5 ml which, in the majority of subjects, was better than human precision from manual segmentation.
|
|
0812.
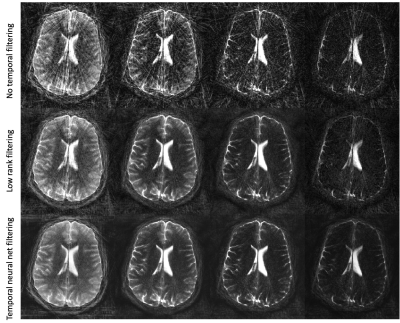 |
Unsupervised radial streak artifact reduction in time resolved MRI
Sagar Mandava1, Ty Cashen2, Daniel V Litwiller3, Tetsuya Wakayama4, and Ersin Bayram5
1Global MR Applications & Workflow, GE Healthcare, Tucson, AZ, United States, 2Global MR Applications & Workflow, GE Healthcare, Madison, WI, United States, 3Global MR Applications & Workflow, GE Healthcare, New York, NY, United States, 4Global MR Applications & Workflow, GE Healthcare, Hino, Japan, 5Global MR Applications & Workflow, GE Healthcare, Houston, TX, United States
Watch the Video
Radial magnetic resonance imaging is attractive due to its inherently high motion robustness and its ability to support accelerated imaging but is plagued by streaking artifact. The problem is exacerbated in time resolved imaging, like DCE-MRI, which deal with higher levels of undersampling due to the need to jointly deliver high spatial and temporal resolution. While reconstructive methods typically based on sparse or low rank methods exist to minimize streak artifact, their use is currently limited due to their high computational complexity. As an alternative, we describe a temporal neural network to suppress streak artifact from a time-series of images.
|
|
0813.
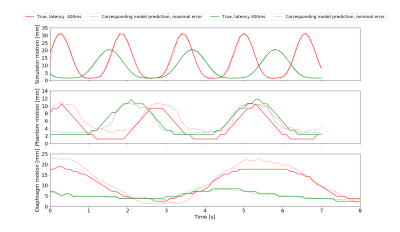 |
Diaphragm motion prediction with a LSTM network using MRI k-space data
Carola Fischer1,2, Florian Friedrich1,2, Peter Bachert1,2, Mark E. Ladd1, and Benjamin R. Knowles1
1Medical Physics in Radiology, German Cancer Research Center (DKFZ), Heidelberg, Germany, 2Department of Physics and Astronomy, Heidelberg University, Heidelberg, Germany
Watch the Video
Hybrid MRI linear accelerators (MR-linac) enable real-time tracking of tumor motion during treatment. Due to system latencies that delay treatment adjustments, one has to predict as well as track motion. This abstract presents a feasibility study to predict diaphragm motion using MRI k-space data using a long short-term memory (LSTM) recurrent neural network, by comparing simulation, phantom and an in vivo study. First experiments show that prediction accuracies of approximately 1.7mm are possible at 400ms latencies for the diaphragm with guided breathing.
|
|
0814.
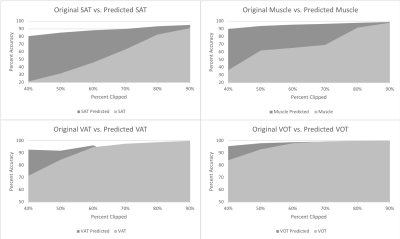 |
Automating Image-Based Body Composition Analysis with Missing Data
Clint R Frandsen1,2, Alexander D Weston1,2, Kenneth R Philbrick1,2, Gian Marco Conte1,2, Bradley J Erickson1,2, and Timothy Kline1,2
1Radiology Informatics Lab, Mayo Clinic, Rochester, MN, United States, 2Physiology & Biomedical Engineering, Mayo Clinic College of Medicine & Science, Rochester, MN, Rochester, MN, United States
Watch the Video
We have developed and evaluated an automated algorithm that learns to synthesize representative segmentations of the missing anatomy in partial abdominal MR images using a deep learning-based approach. These synthesized segmentations are optimal for studies focusing on the analyzes of body composition.
|
|
0815.
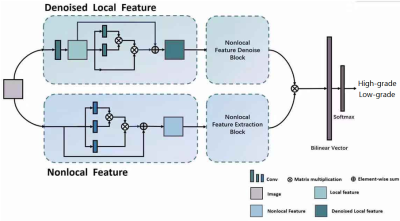 |
Local feature denoising and global feature extraction for malignancy characterization of hepatocellular carcinoma
Wu Zhou1, Hui Huang1, Guangyi Wang2, and Honglai Zhang1
1School of Medical Information Engineering, Guangzhou University of Chinese Medicine, Guangzhou, China, 2Department of Radiology, Guangdong General Hospital, Guangzhou, China
Watch the Video
Convolutional neural network (CNN) has been regarded to be powerful for lesion characterization in clinical practice. However, local deep feature derived from CNN has two main shortcomings for characterization. First, the convolutional operations typically process within a local neighborhood while ignoring the global dependency. Furthermore, it is unstable to small perturbations in images (e.g., noise or artifacts). Therefore, we propose a denoised local fusion and nonlocal deep feature fusion method to alleviate the above two problems. The proposed method is a general module, which can be integrated into any CNN-based architecture for improving performance of lesion characterization in clinical routine.
|
|