Monday Parallel 4 Live Q&A |
Monday, 10 August 2020, 14:30 - 15:15 UTC |
Moderators: Akshay Chaudhari & Martijn Froeling |
Session Number: O-33
|
0241.
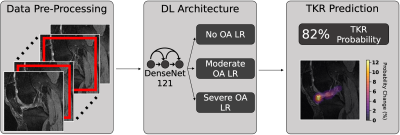 |
Deep Learning Predicts Total Knee Replacement from Magnetic Resonance Images
Aniket A. Tolpadi1,2, Jinhee J. Lee1, Valentina Pedoia1, and Sharmila Majumdar1
1Department of Radiology and Biomedical Imaging, UCSF, San Francisco, CA, United States, 2Department of Bioengineering, University of California, Berkeley, Berkeley, CA, United States
Watch the Video
Total Knee Replacement (TKR) can relieve pain from osteoarthritis (OA), but patient dissatisfaction is not uncommon, making TKR delay advisable until absolutely necessary. Models could identify at-risk patients requiring nonsurgical treatment, prolonging good health and delaying TKR. We present a pipeline that uses DenseNet-121 to predict TKR onset from MRI images, integrates clinical information by ensembling logistic regression models, and sensitively and specifically predicts TKR, particularly at early-stage OA. Occlusion maps show many OA progression imaging biomarkers are implicated in TKR, and many tissues involved in knee flexion and extension preferentially affect TKR probability at early-stage and late-stage OA, respectively.
|
|
0242.
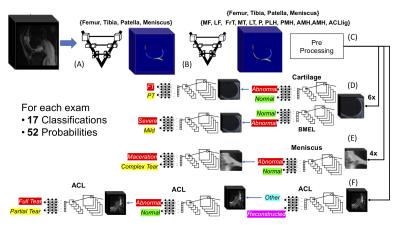 |
Deep Learning Assisted Full Knee 3D MRI-Based Lesion Severity Staging
Bruno Astuto Arouche Nunes1, Io Flament1, Nikan K. Namiri1, Rutwik Shah2,3, Matthew Bucknor1, Thomas Link2, Valentina Pedoia2,3, and Sharmila Majumdar2
11Department of Radiology and Biomedical Imaging, UCSF, San Francisco, CA, United States, 2Department of Radiology and Biomedical Imaging, UCSF, San Francisco, CA, United States, 3Center for Digital Health Innovation, UCSF, San Francisco, CA, United States
Watch the Video
The goal of this study is to capitalizing on recent developments in Deep Learning (DL) applied to medical imaging. Specifically, we aim to (i) identify cartilage, meniscus, bone marrow edema (BEM) and ACL ligament lesions and assess severity providing full knee lesion severity assessment, and (ii) provide a condensed clinical history of patients in an automated manner.
|
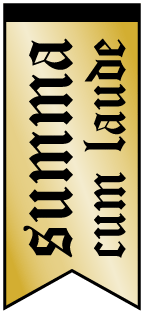 |
0243.
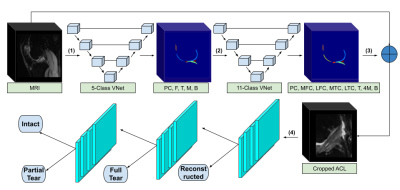 |
Semi-Quantitative Grading of the Anterior Cruciate Ligament using Deep Learning
Nikan K Namiri1, Io Flament1, Bruno Astuto1, Rutwik Shah1, Radhika Tibrewala1, Francesco Caliva1, Thomas M Link1, Valentina Pedoia1, and Sharmila Majumdar1
1Department of Radiology and Biomedical Imaging, University of California, San Francisco, San Francisco, CA, United States
Watch the Video
In this study we present a fully-automated anterior cruciate ligament (ACL) detection and classification framework which provides multi-class severity staging of ACL tears using state-of-the-art deep learning architectures. We compared the performances of a 3D and a 2D convolutional neural network (CNN) in ACL lesion classification. A higher overall accuracy (84%) and linear-weighted kappa (.92) were observed with the 2D model; however, it underperformed compared to the 3D CNN in classifying partial tears. This is the first reported deep learning detection and classification pipeline for ACL severity staging, including reconstructed, fully torn, partially torn, and intact ligaments.
|
|
0244.
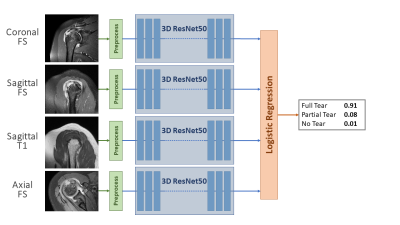 |
Deep-learning Diagnosis of Supraspinatus Tendon Tears: Comparison of Multi-sequence Versus Single Sequence Input
Dana J. Lin, MD1, JinHyeong Park, PhD2, Michael Schwier, PhD2, Bernhard Geiger, PhD2, Esther Raithel, PhD3, and Michael P. Recht, MD1
1Department of Radiology, NYU School of Medicine, New York, NY, United States, 2Siemens Healthineers, Princeton, NJ, United States, 3Siemens Healthineers, Erlangen, Germany
Watch the Video
Rotator cuff tears are a common cause of shoulder pain and typically diagnosed on shoulder MRI. Using 1,218 MR examinations performed at multiple field strengths and from multiple vendors, we developed a deep-learning (DL) model for the diagnosis of supraspinatus tendon tears on MRI using an ensemble of 3D ResNets combined via logistic regression to classify tears into no tear, partial tear, and full-thickness tear. We compared the effect of using multiple sequences as input versus a single sequence. Our results show that deep-learning diagnosis of supraspinatus tendon tears is feasible and that multi-sequence input improves model performance.
|
|
0245.
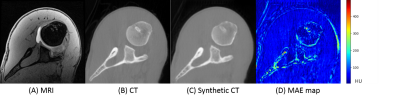 |
Deep Shoulder CT Image Synthesis from MR via Context-aware 2.5D Generative Adversarial Networks
Yucheng Liu1, Yulin Liu2, Michael Z. Liu1, Pawas S. Shukla1, Richard Ha1, Tim Duong3, Sachin R. Jambawalikar1, and Tony T. Wong1
1Radiology, Columbia University Irving Medical Center, New York, NY, United States, 2Information and Computer Engineering, Chung Yuan Christian University, Taoyuan City, Taiwan, 3Radiology, Stony Brook Medicine, Stony Brook, NY, United States
Watch the Video
We developed a context-aware 2.5D Generative Adversarial Network (GAN) to generate synthetic CT images from MRI. Adjacent 2D slices with in plane matrix of 512 x 512 and user defined slice context (from 3 to 41-slices) were provided as input. This allows the network to learn out-of-plane information for the slice of interest thereby alleviating the intensity discontinuity problem seen in 2D networks. In addition, this approach uses less GPU memory than a 3D GAN. Our results indicated that the network trained with larger number of adjacent slices outperform the fewer slice network.
|
|
0246.
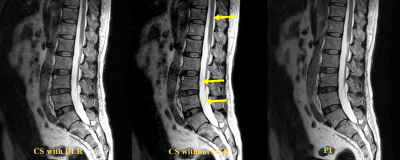 |
Compressed Sensing with and without Deep Learning Reconstruction: Comparison of Capability for Improving Lumber Spine MRI with Parallel Imaging
Yuki Obama1, Yoshiharu Ohno1, Kaori Yamamoto2, Akiyoshi Iwase3, Takahiro Ueda1, Kazuhiro Murayama4, and Hiroshi Toyama1
1Radiology, Fujita Health University School of Medicine, Toyoake, Japan, 2Canon Medical Systems Corporation, Otawara, Japan, 3Radiology, Department of Radiology, Fujita Health University Hospital, Toyoake, Japan, 4Radiology, Joint Research Laboratory of Advanced Medical Imaging, Fujita Health University School of Medicine, Toyoake, Japan
Watch the Video
There have been no major reports for assessing the utility of compressed sensing (CS) and deep learning reconstruction (DLR) as compared with routinely applied parallel imaging (PI) on lumber spine MRI. We hypothesized that CS with DLR was able to improve image quality and shorten examination time on lumber spine MRI, when compared with PI. The purpose of this study was to directly compare the capability for improving lumber spine MRI among CS with and without DLR and PI in patients with different lumber spinal diseases.
|
|
0247.
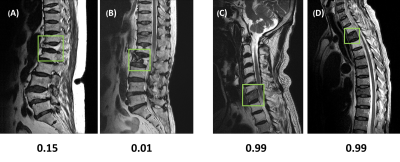 |
Deep learning for the detection and differentiation of vertebral fracture
Yang Zhang1, Lee-Ren Yeh2, Jeon-Hor Chen1,2, Ning Lang3, Xiaoying Xing3, Yongye Chen3, Qizheng Wang3, Peter Chang1, Daniel Chow1, Huishu Yuan3, and Min-Ying Su1
1Department of Radiological Science, University of California, Irvine, CA, United States, 2Department of Radiology, E-Da Hospital and I-Shou University, Kaohsiung, Taiwan, 3Department of Radiology, Peking University Third Hospital, Beijing, China
Watch the Video
This study investigated the value of deep learning for the detection and differential diagnosis of vertebral fracture. A model using ResNet50 was developed and tested in a separate dataset. The results were compared with the interpretation of an experienced radiologist. Our study noted that the analysis based on single vertebral body without inclusion of the soft tissue, the posterior elements, and the skipped lesions might be the reason why the radiologist’s reading was better than deep learning approach. For the identification of malignant fracture using whole images from training set, the prediction accuracy was only moderate, with rooms for improvement.
|
|
0248.
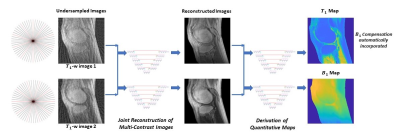 |
Quantitative T1 Mapping from Incoherently Undersampled MR Images Using Self-Attention Convolutional Neural Networks
Yan Wu1, Yajun Ma2, Jiang Du2, and Lei Xing3
1Stanford University, Stanford, CA, United States, 2Radiology, University of California San Diego, La Jolla, CA, United States, 3Radiation Oncology, Stanford University, Stanford, CA, United States
Watch the Video
The application of current quantitative MRI techniques is limited by the long scan time. In this study, we propose a deep learning strategy to derive quantitative T1 map and B1 map from two incoherently undersampled variable contrast images. Furthermore, radiofrequency field (B1) inhomogeneity is automatically corrected in the derived T1 map. The tasks are accomplished in two steps: joint reconstruction and parameter quantification, both employing self-attention convolutional neural networks. Significant reduction in data acquisition time has been successfully achieved, including an acceleration in variable contrast image acquisition caused by undersampling and a waiver of B1 map measurement.
|
|
0249.
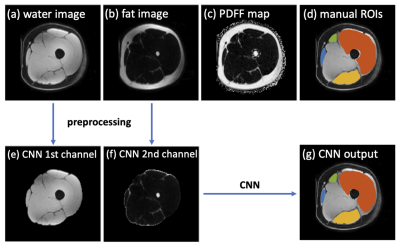 |
Deep learning-based thigh muscle segmentation for reproducible fat fraction quantification using fat-water decomposition MRI
Jie Ding1, Varut Vardhanabhuti1, Eric Lai2, Yuan Gao3, Sophelia Chan4, and Peng Cao1
1Department of Diagnostic Radiology, Li Ka Shing Faculty of Medicine, The University of Hong Kong, Hong Kong, Hong Kong, 2Li Ka Shing Faculty of Medicine, The University of Hong Kong, Hong Kong, Hong Kong, 3Division of Neurology, Department of Medicine, Queen Mary Hospital, The University of Hong Kong, Hong Kong, Hong Kong, 4Department of Paediatrics and Adolescent Medicine, Li Ka Shing Faculty of Medicine, The University of Hong Kong, Hong Kong, Hong Kong
Watch the Video
Time-efficient thigh muscle segmentation is a major challenge in moving from primarily qualitative assessment of thigh muscle MRI in clinical practice, to potentially more accurate and quantitative methods. In this work, we trained a convolutional neural network to automatically segment four clinically relevant muscle groups using fat-water MRI. Compared to cumbersome manual annotation which ordinarily takes at least 5-6 hours, this fully automated method provided sufficiently accurate segmentation within several seconds for each thigh volume. More importantly, it yielded more reproducible fat fraction estimations, which is extremely useful for quantifying fat infiltration in ageing and in diseases like neuromuscular disorders.
|
|