Monday Parallel 5 Live Q&A |
Monday, 10 August 2020, 14:30 - 15:15 UTC |
Moderators: Bradford Moffat & Natarajan Raghunand |
Session Number: O-37
|
0263.
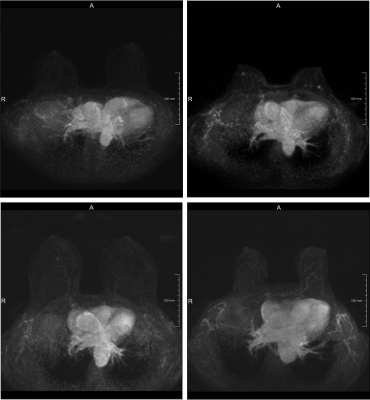 |
Triaging dense breast screening MR images using a dilated convolutional neural network
Angelo Zuffianò1, Bob de Vos1, Jorrit Glastra1, Pim Moeskops1, Valerio Fortunati1, Ivana Išgum2, Tim Leiner3, Carla van Gils3, and Wouter Veldhuis3
1Quantib, Utrecht, Netherlands, 2Biomedical Engineering and Physics, Radiology and Nuclear Medicine, Amsterdam University Medical Center, Amsterdam, Netherlands, 3Radiology, Utrecht University Medical Center, Utrecht, Netherlands
Watch the Video
Dynamic contrast enhanced (DCE) MRI is the key series to analyze for the detection of breast cancer in women with extremely dense breasts. Given the increasing number of women receiving dense breast MRI screening we aimed to reduce radiologist workload without reducing the high sensitivity of MRI. We developed a convolutional neural network (CNN) based method able to defer 8.1% of the workload by identifying non-enhancing scans with a sensitivity of 96.3%.
|
|
0264.
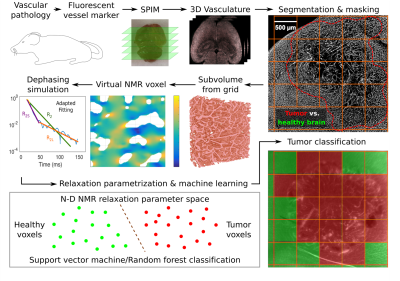 |
Proof-of-principle for endogenous signal classification towards voxel-wise tumor detection using statistical machine learning
Artur Hahn1,2, Julia Krüwel-Bode3, Yannis Seemann2, Sarah Schuhegger2, Johann M. E. Jende1, Anja Hohmann4, Volker J. F. Sturm1, Ke Zhang5, Sabine Heiland1, Martin Bendszus1, Michael O. Breckwoldt1,6, Christian H. Ziener1,5, and Felix T. Kurz1,5
1Department of Neuroradiology, Heidelberg University Hospital, Heidelberg, Germany, 2Department of Physics and Astronomy, University of Heidelberg, Heidelberg, Germany, 3Molecular Mechanisms of Tumor Invasion (V077), German Cancer Research Center (DKFZ), Heidelberg, Germany, 4Department of Neurology, Heidelberg University Hospital, Heidelberg, Germany, 5Department of Radiology (E010), German Cancer Research Center (DKFZ), Heidelberg, Germany, 6Clinical Cooperation Unit Neuroimmunology and Brain Tumor Immunology, German Cancer Research Center (DKFZ), Heidelberg, Germany
Watch the Video
Based on the microvasculature of entire healthy and tumor-bearing mouse brains, imaged with high-resolution fluorescence microscopy, the transverse relaxation process within virtual MRI voxels was simulated. Extended parametrizations of the non-Lorentzian signal decay were used to train support vector machine and random forest classifiers to differentiate healthy brain and tumor voxel signals. A proof-of-principle is presented with U87 and GL261 glioblastoma at different SNR levels. This automated workflow enables the in-silico development of specialized MRI sequences to maximize classification accuracy with minimal NMR measurements for experimental analogies.
|
|
0265.
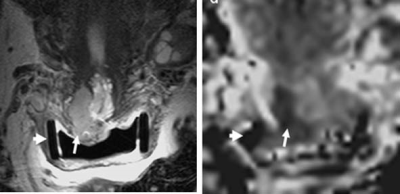 |
Radiomic features of cervical tumors: identifying volume thresholds for transition to a poor prognosis phenotype
Benjamin W Wormald1,2, Thomas EJ Ind2,3, and Nandita M deSouza1,4
1Imaging, The Institute of Cancer Research, Sutton, Surrey, United Kingdom, 2Gynaecological Oncology, The Royal Marsden NHS FoundationTrust, London, United Kingdom, 3Surgery, St. Georges University Hospital, London, United Kingdom, 4MRI Unit, The Royal Marsden NHS Foundation Trust, Sutton, Surrey, United Kingdom
Watch the Video
Cervical cancer recurs post-trachelectomy often because of close surgical margins or lymph-node micrometastases. We show that 5 texture features distinguish good- from poor-prognosis tumors (low/high volume, without/with parametrial invasion, without/with lymph node metastases). For tumors suitable for trachelectomy (<4.19cm3), linear regression of feature value with volume (using 3 features with high discrimination of groups and 1 standard deviation from median from good prognosis group as threshold) indicated that radiomic features tended towards values representing poor prognosis at 1.8±0.2cm3 (T2-W images) and 1.8±0.06cm3 (ADC maps). Above 1.8cm3 textural features of cervical cancer shift towards a phenotype likely to spread and metastasize.
|
|
0266.
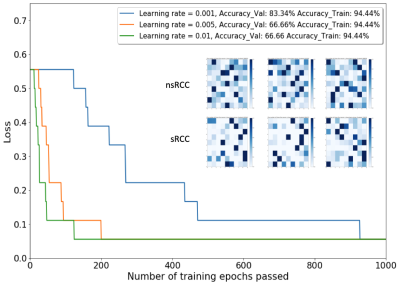 |
Identification of Sarcomatoid De-Differentiation in Renal Cell Carcinoma by Machine Learning on Multiparametric MRI
Asim M. Mazin1, Samuel H. Hawkins1, Olya Stringfield2, Jasreman Dhillon3, Brandon J. Manley4, Daniel K. Jeong5, and Natarajan Raghunand1
1Department of Cancer Physiology, Moffitt Cancer Center, Tampa, FL, United States, 2IRAT Shared Service, Moffitt Cancer Center, Tampa, FL, United States, 3Department of Anatomic Pathology, Moffitt Cancer Center, Tampa, FL, United States, 4Department of Genitourinary Oncology, Moffitt Cancer Center, Tampa, FL, United States, 5Department of Diagnostic & Interventional Radiology, Moffitt Cancer Center, Tampa, FL, United States
Watch the Video
We report a machine learning approach using Self-Organizing Maps (SOM) and Learning Vector Quantization (LVQ) to analyze multiparametric MRI for the purpose of differentiating between renal cell carcinoma tumor with (sRCC) and without (nsRCC) sarcomatoid de-differentiation, a transformation that is associated with poorer outcomes. The SOM+LVQ model was trained on mpMRI data from 9 nsRCC and 9 sRCC tumors, validated on a separate cohort of 3 nsRCC and 3 sRCC tumors, and tested on a held-out set of 5 nsRCC and 5 sRCC tumors. An overall classification accuracy of 70% was achieved on the test cohort.
|
|
0267.
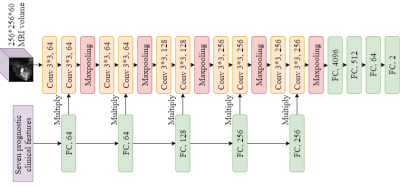 |
Artificial Intelligence for Predicting Pathological Complete Response to Neoadjuvant Chemotherapy from MRI and Prognostic Clinical Features
Hongyi Duanmu1, Pauline Huang1, Srinidhi Brahmavar1, Fusheng Wang1, and Tim Q Duong1
1Stony Brook University, Stony Brook, NY, United States
Watch the Video
Pathological complete response (pCR) is a measurement of the effectiveness of neoadjuvant chemotherapy (NAC). While there are several studies about predicting the pCR, no one system can fully automate this prediction process. We proposed a 3D convolutional neural network (CNN) system, integrating information on breast MRI images and prognostic clinical features, to predict pCR pre-NAC. This system achieved inspiring results in the ISPY1 Clinical Trial dataset, with 77% accuracy. This approach shows the potential in breast cancer diagnose and assessment. Furthermore, the mechanism of integrating images and features information can be used and generalized to other tasks.
|
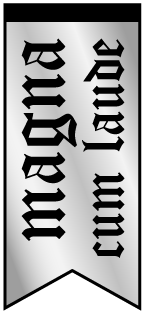 |
0268.
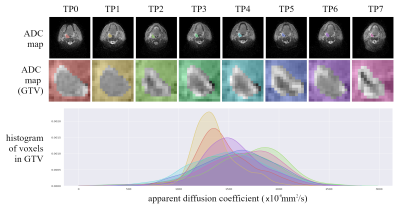 |
The impact of radiomic feature reproducibility on a head and neck cancer radiotherapy response model: a comparison of two common analysis packages
James C Korte1,2, Carlos E Cardenas3, Tomas Kron1,4, Nicholas Hardcastle1,5, Jihong Wang3, Houda Bahig6, Baher Elgohari7, Laurence E Court3, Clifton D Fuller7, and Sweet Ping Ng7,8
1Department of Physical Science, Peter MacCallum Cancer Centre, Melbourne, Australia, 2Department of Biomedical Engineering, The University of Melbourne, Melbourne, Australia, 3Department of Radiation Physics, The University of Texas MD Anderson Cancer Center, Houston, TX, United States, 4Sir Peter MacCallum Department of Oncology, The University of Melbourne, Melbourne, Australia, 5Centre for Medical Radiation Physics, University of Wollongong, Wollongong, Australia, 6Radiation Oncology Department, Centre Hospitalier de l'Université de Montréal, Montreal, QC, Canada, 7Department of Radiation Oncology, University of Texas MD Anderson Cancer Center, Houston, TX, United States, 8Department of Radiation Oncology, Peter MacCallum Cancer Centre, Melbourne, Australia
Watch the Video
Radiomics is a promising technique for discovering image based biomarkers of therapy response in cancer. Reproducibility of radiomic features is a known issue that is being addressed by standardisation initiatives, but it remains a challenge to interpret previously published radiomic signatures. We investigate the reproducibility of radiomic features calculated with two common software packages and explore the impact of including non-reproducible diffusion features in a head and neck cancer (HNC) radiotherapy response model. Our results demonstrate that equivalent models can be generated from either software, but only when restricting the model to reproducible features identified with a correlation threshold method.
|
|
0269.
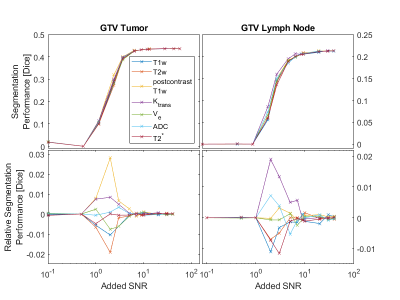 |
Evaluating Noise Robustness of CNN-based Head&Neck Tumor Segmentations on Multiparametric MRI Data
Lars Bielak1,2, Nicole Wiedenmann2,3, Arnie Berlin4, Leonard Hägele1, Thomas Lottner1, Sebastian Gross5, Anca-Ligia Grosu2,3, and Michael Bock1,2
1Dept. of Radiology, Medical Physics, Medical Center - University of Freiburg, Faculty of Medicine, Freiburg, Germany, 2German Cancer Consortium (DKTK), Partner Site Freiburg, Freiburg, Germany, 3Dept. of Radiation Oncology, Medical Center - University of Freiburg, Faculty of Medicine, Freiburg, Germany, 4The MathWorks, Inc., Novi, MI, United States, 5The MathWorks, Inc., Ismaning, Germany
Watch the Video
Multiparametric MRI imaging in combination with PET/CT is the basis for precise tumor segmentation in radiation therapy. We trained a segmentation CNN on the multiparametric MRI data of head and neck squamous cell carcinoma patients and investigated the network robustness against noise corruption in the input channels. Overall noise robustness and differences between seven different input contrasts were compared.
|
|
1243.
 |
Radiomics analysis for Characterizing Ovarian Tumor Based on a DCE-MRI Pharmacokinetic Protocol
Xiao-li Song1, Jia-Liang Ren2, Kaiyu Wang3, and JinLiang Niu1
1Shanxi Medical University Second Affiliated Hospital, Taiyuan, China, 2GE Healthcare, Beijing, China., Beijing, China, 3GE Healthcare, MR Research China, Beijing, China, Beijing, China
Watch the Video
DCE-MRI and its subsequently derived pharmacokinetic parameters have been adopted to explore tumor angiogenesis and vascular permeability changes inside tumors and improve the diagnostic accuracy of ovarian tumors. Radiomics can convert medical images to mineable high-dimensional quantitative imaging features based on automatic feature extraction algorithms. In this study, we present a radiomics model based on a DCE-MRI PK protocol and establish an effective and noninvasive 3-class classification prediction model for the discrimination among benign, borderline and malignant ovarian tumors.
|
|