Monday Parallel 5 Live Q&A |
Monday, 10 August 2020, 15:15 - 16:00 UTC |
Moderators: Wolfgang Bogner & Candace Fleischer |
Session Number: O-43
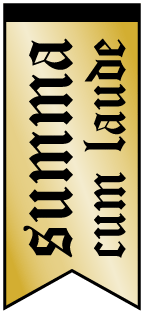 |
0388.
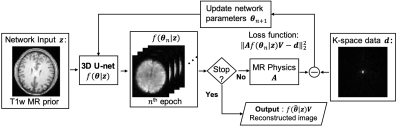 |
High Resolution MR Spectroscopic Imaging Using Deep Image Prior Constrained Subspace Modeling
Kuang Gong1, Paul Kyu Han1, Thibault Marin1, Georges El Fakhri1, Quanzheng Li1, and Chao Ma1
1Gordon Center for Medical Imaging, Department of Radiology, Massachusetts General Hospital, Harvard Medical School, Boston, MA, United States
Watch the Video
The subspace-based method, known as SPICE, is an emerging technique that achieves rapid high-resolution MRSI with good SNR. In SPICE, the spectrum at each voxel is represented as a low-dimensional subspace or manifold, where the basis functions or features are learned from training data. The spatial coefficients of the subspace model are estimated by fitting the model to the k-space data for image reconstruction. In this work, we propose to extend the SPICE framework by representing the spatial coefficients of the subspace model using deep image prior for improved image reconstruction.
|
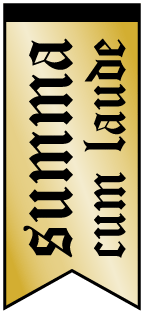 |
0389.
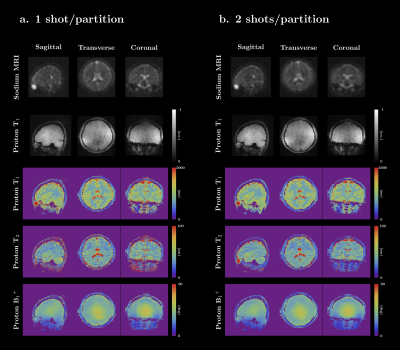 |
Simultaneous 3D proton MRF and sodium MRI
Zidan Yu1,2,3, Shota Hodono1,2,3, Bili Wang1, Olga Dergahyova1, Bei Zhang1,3, Ryan Brown1,3, Daniel K. Sodickson1,2,3, Guillaume Madelin1,2, and Martijn A. Cloos1,2,3
1Center for Biomedical Imaging, Department of Radiology, New York University School of Medicine, New York, NY, United States, 2Sackler Institute of Graduate Biomedical Sciences, NYU Langone Health, New York, NY, United States, 3Center for Advanced Imaging Innovation and Research (CAI2R), NYU Langone Health, New York, NY, United States
Watch the Video
In this work, we present a 3D sequence that can simultaneously capture quantitative 1H density, T1, T2, B1+ maps and a 23Na image of the whole head in a reasonable scan time (~10 min). The gradient momenta are strategically distributed to simultaneously acquire a full-radial trajectory for proton and a center-out radial trajectory for sodium in one single readout. A sodium SNR comparison, verification of the proton multi-parametric maps, and in-vivo results are shown.
|
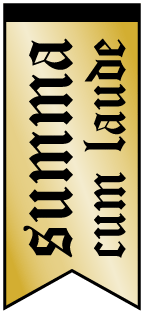 |
0390.
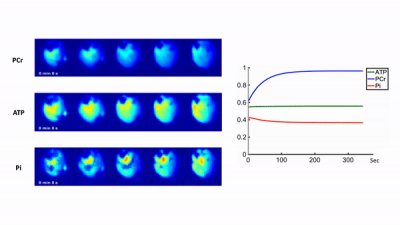 |
High-Resolution Dynamic 31P-MRSI of Ischemia-Reperfusion in Rat Using Low-Rank Tensor Model with Deep Learning Priors
Yudu Li1,2, Kihwan Kim3,4, Bryan Clifford1,2, Rong Guo1,2, Yuning Gu3,4, Zhi-Pei Liang1,2, and Xin Yu3,4,5,6
1Department of Electrical and Computer Engineering, University of Illinois at Urbana-Champaign, Urbana, IL, United States, 2Beckman Institute for Advanced Science and Technology, University of Illinois at Urbana-Champaign, Urbana, IL, United States, 3Department of Biomedical Engineering, Case Western Reserve University, Cleveland, OH, United States, 4Case Center for Imaging Research, Case Western Reserve University, Cleveland, OH, United States, 5Department of Radiology, Case Western Reserve University, Cleveland, OH, United States, 6Department of Physiology and Biophysics, Case Western Reserve University, Cleveland, OH, United States
Watch the Video
Dynamic 31P-MRS/MRSI is a promising tool for in vivo quantification of mitochondrial oxidative capacity. However, its practical utility is limited by the inherently low SNR of the 31P signal. This work is built upon our recent progress in accelerating dynamic 31P-MRSI using low-rank tensor models. We extended this method by learning the temporal priors with deep generative models and then incorporating them into the reconstruction via an information theoretical framework. This approach enabled high-resolution dynamic 31P-MRSI with 1.5x1.5x2 mm3 nominal spatial resolution and 5.1-sec temporal resolution in capturing the kinetics of metabolite changes in rat hindlimb during a stimulation-recovery protocol.
|
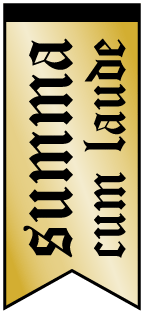 |
0391.
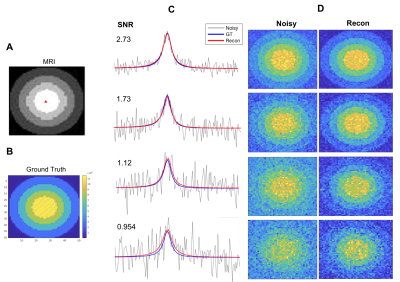 |
A Deep Learning Method for Sensitivity Enhancement in Deuterium Metabolic Imaging (DMI)
Siyuan Dong1, Henk M. De Feyter2, Monique A. Thomas2, Robin A. de Graaf3, and James S. Duncan4
1Department of Electrical Engineering, Yale University, New Haven, CT, United States, 2Department of Radiology and Biomedical Imaging, Yale University, School of Medicine, New Haven, CT, United States, 3Department of Radiology and Biomedical Imaging, Department of Biomedical Engineering, Yale University, School of Medicine, New Haven, CT, United States, 4Department of Radiology & Biomedical Imaging, Department of Electrical Engineering, Department of Statistics & Data Science, Yale University, New Haven, CT, United States
Watch the Video
Deuterium Metabolic Imaging (DMI) is a novel approach providing 3D metabolic data from both animal models and human subjects. DMI relies on 2H MRSI in combination with administration of 2H-labeled substrates. Common to all MRI and MRSI methods, DMI's resolution is ultimately limited by the achievable SNR. This work proposes a data-driven method using a deep convolutional autoencoder to improve the SNR and increase the spatial resolution of DMI. The method was tested with simulated, phantom and in vivo experiments at various SNR levels to demonstrate its capability and precision for metabolic mapping using noisy DMI data.
|
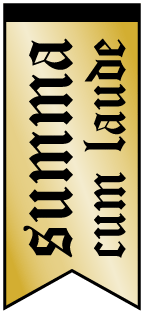 |
0392.
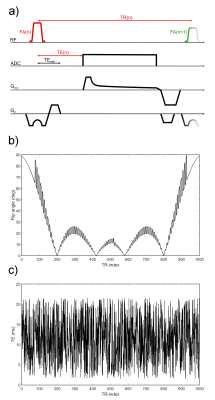 |
Sodium Relaxometry using Magnetic Resonance Fingerprinting
Fabian J. Kratzer1,2, Sebastian Schmitter1,3, Armin M. Nagel1,4,5, Nicolas G. R. Behl6, Benjamin R. Knowles1, Peter Bachert1,2, Mark E. Ladd1,2,7, and Sebastian Flassbeck1
1Medical Physics in Radiology, German Cancer Research Center (DKFZ), Heidelberg, Germany, 2Faculty of Physics and Astronomy, Ruprecht-Karls University Heidelberg, Heidelberg, Germany, 3Physikalisch-Technische Bundesanstalt (PTB), Braunschweig and Berlin, Germany, 4Institute of Radiology, University Hospital Erlangen, Friedrich-Alexander-Universität Erlangen-Nürnberg (FAU), Erlangen, Germany, 5Institute of Medical Physics, Friedrich-Alexander-Universität (FAU), Erlangen, Germany, 6Siemens Healthcare GmbH, Erlangen, Germany, 7Faculty of Medicine, Ruprecht-Karls University Heidelberg, Heidelberg, Germany
Watch the Video
Sodium relaxation times have been shown to be altered in several diseases. However, due to short relaxation times and low in-vivo signal, measurement times in sodium relaxometry on the order of 1h were reported for both, longitudinal and transversal relaxation constants. In this work, a novel sodium relaxometry method based on Magnetic Resonance Fingerprinting (MRF) principles is presented, which enables simultaneous quantification of T1, T2s*, T2l*, T2* and ΔB0, with automatic distinction between bi- and monoexponential transverse relaxation.
|
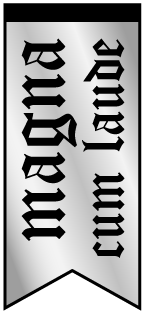 |
0393.
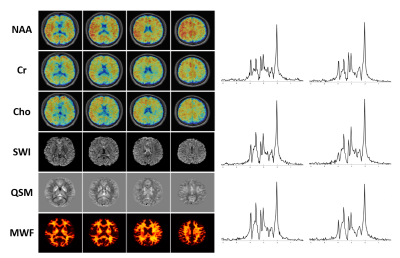 |
Making SPICE Spicier with Sparse Sampling of (k, t)-Space and Learned Subspaces
Rong Guo1,2, Yudu Li1,2, Yibo Zhao1,2, Yao Li3,4, and Zhi-Pei Liang1,2
1Department of Electrical and Computer Engineering, University of Illinois at Urbana-Champaign, Urbana, IL, United States, 2Beckman Institute for Advanced Science and Technology, University of Illinois at Urbana-Champaign, Urbana, IL, United States, 3School of Biomedical Engineering, Shanghai Jiao Tong University, Shanghai, China, 4Med-X Research Institute, Shanghai Jiao Tong University, Shanghai, China
Watch the Video
SPICE has recently provided a unique capability for simultaneous acquisition of metabolite and water spectroscopic signals. While the water signals are often removed as nuisance components in traditional MRSI experiments, SPICE utilizes the water signals for QSM, MWF mapping, etc. In this work, we further extend SPICE data acquisition to achieve much larger k-space coverage and improve its processing scheme for simultaneous MRSI/QSM/SWI/MWF mapping. In vivo experiments demonstrated that this new scheme improved the accuracy of water/lipid removal, reduced the effects of field inhomogeneity, and achieved higher resolution for QSM, SWI and MWF using the unsuppressed water signals.
|
|
0394.
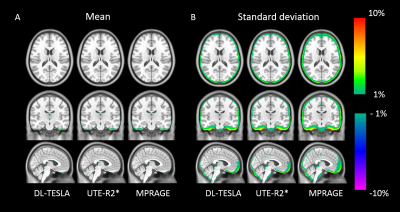 |
Deep learning based T1-enhanced selection of linear attenuation coefficients for PET/MR attenuation correction: accuracy and repeatability
Chunwei Ying1, Yasheng Chen2, Michael M. Binkley2, Meher R. Juttukonda3,4, Shaney Flores1, Tammie L. S. Benzinger1,5, and Hongyu An1
1Mallinckrodt Institute of Radiology, Washington University School of Medicine, St. Louis, MO, United States, 2Department of Neurology, Washington University School of Medicine, St. Louis, MO, United States, 3Athinoula A. Martinos Center for Biomedical Imaging, Massachusetts General Hospital, Charlestown, MA, United States, 4Department of Radiology, Harvard Medical School, Boston, MA, United States, 5Department of Neurological Surgery, Washington University School of Medicine, St. Louis, MO, United States
Watch the Video
We proposed a 3D patch based residual U-Net method to estimate pseudo CT images for PET/MR attenuation correction by including quantitative R1 maps as input. The proposed deep learning based T1-enhanced selection of linear attenuation coefficients (DL-TESLA) method outperformed the deep learning methods using UTE-R2* or MPRAGE as inputs with a similar network structure. Moreover, we demonstrated that DL-TESLA had an excellent PET test-retest repeatability that was comparable to PET/CT, supporting its use for PET/MR AC in longitudinal studies of neurodegenerative diseases.
|
|
0395.
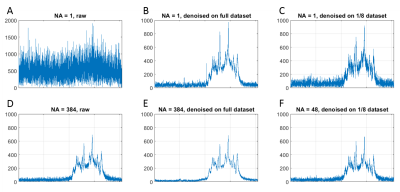 |
MP-PCA denoising dramatically improves SNR in large-sized MRS data: an illustration in diffusion-weighted MRS
Ileana Ozana Jelescu1, Jelle Veraart2, and Cristina Cudalbu1
1Center for Biomedical Imaging, Ecole Polytechnique Fédérale de Lausanne, Lausanne, Switzerland, 2Dept. of Radiology, New York University School of Medicine, New York, NY, United States
Watch the Video
MRS is an inherently low signal-to-noise technique resulting in substantial spectral averaging and large voxel volumes. The problem is further amplified for diffusion-weighted MRS. Here we test the performance of denoising using principal component analysis coupled with Marchenko-Pastur’s random matrix theory in the context of DW-MRS. We report 50 – 100% increase in SNR, reduction in Cramer-Rao bounds and a potential eight-fold reduction in scan time. This technique is expected to also bring significant improvements in the context of fMRS, X-nuclei MRS and CSI.
|
|
0396.
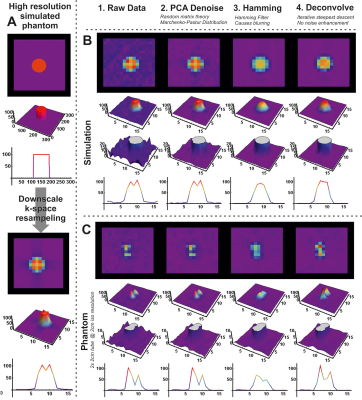 |
Processing of muscle phosphorus 7T CSI data using PCA denoising and deconvolution
Martijn Froeling1, Tijl A van der Velden1, Jeanine J Prompers1, and Dennis WJ Klomp1
1Department of Radiology, UMC Utrecht, Utrecht, Netherlands
Watch the Video
Chemical shift imaging generally suffers from low SNR and low spatial resolution, especially for x-nuclei. State of the art image processing methods from the MRI domain, e.g. DTI pre-processing, can be applied to CSI data. In this study we show the feasibility of PCA denoising combined with deconvolution to enhance CSI SNR and spatial localization.
|
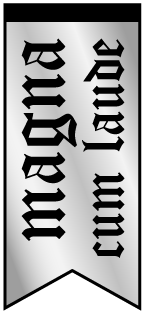 |
0397.
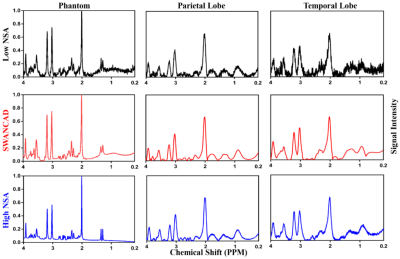 |
Spectral Wavelet-feature Analysis and Classification Assisted Denoising Approach for Enhancing Signal to Noise Ratios of MRS Data
Bing Ji1, Zahra Hosseini2, Liya Wang3, Lei Zhou4, Xinhua Tu5, and Hui Mao6
1Department of Radiology and Imaging Sciences, Emory University School of Medicine, Emory University, Atlanta, GA, Georgia, 2MR R&D Collaborations, Siemens Healthineers,, Atlanta, Georgia, 3Department of Radiology, The People’s Hospital of Longhua, Shenzhen, China, 4Department of Radiology and Imaging Sciences, Emory University School of Medicine, Emory University, Atlanta, Georgia, 5School of Communication and Information Engineering, Nanjing University of Posts and Telecommunication, Nanjing, China, 6Department of Radiology and Imaging Sciences, Emory University School of Medicine, Emory Univeristy, Atlanta, GA, United States
Watch the Video
Low signal-to-noise ratio (SNR) and long acquisition time limit the clinical applications of magnetic resonance spectroscopy (MRS). This work presents a data-driven machine-learning assisted Spectral Wavelet-feature Analysis and Classification Assisted Denoising (SWANCAD) approach to extract the specific spectral wavelets of signals and noises for reducing noise and improving SNR of MRS data. The effective denoise by SWANCAD enabled resolving prominent metabolic peaks but also identify the smaller concentration metabolites which are merged in the noises. Potential applications of the SWANCAD includes the possibility of improving the signal to noise ratio (SNR) of MRS data collected in sub-minute or sub-cm voxels.
|
|