Wednesday Parallel 4 Live Q&A |
Wednesday, 12 August 2020, 14:30 - 15:15 UTC |
Moderators: Kerstin Pannek & Yogesh Rathi |
Session Number: O-68
|
0848.
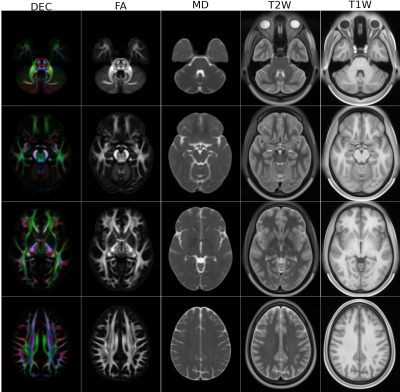 |
Diffusion MRI Atlases from the Human Connectome Project Data
M. Okan Irfanoglu1, Amritha Nayak1,2, and Carlo Pierpaoli1
1QMI, NIBIB/NIH, Bethesda, MD, United States, 2The Henry Jackson Foundation for the Advancement of Military Medicine, Rockville, MD, United States
Watch the Video
In this work, we have created diffusion MRI (dMRI) atlases from the young adult Human Connectome Project (HCP) data. In order to achieve increased anatomical detail and to enable subsequent morphological analysis, we have reprocessed the entire HCP1200 dataset. The DTI atlases, derived scalar maps and JHU atlas inspired white matter ROIs have been made publicly available. The reprocessed DWIs will be made available for HARDI analysis in the near future.
|
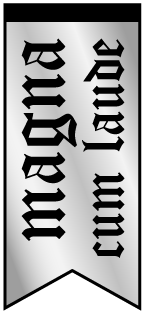 |
0849.
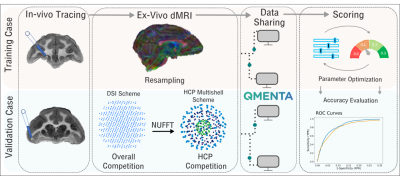 |
The IronTract challenge: Validation and optimal tractography methods for the HCP diffusion acquisition scheme
Chiara Maffei1, Gabriel Girard2,3, Kurt G. Schilling4, Nagesh Adluru5, Dogu Baran Aydogan6, Andac Hamamci7, Fang-Cheng Yeh8, Matteo Mancini9,10, Ye Wu11, Alessia Sarica12, Achille Teillac13,14,15, Steven H. Baete16,17, Davood Karimi18,
Ying-Chia Lin16,17, Fernando Boada16,17, Nathalie Richard13, Bassem Hiba13, Aldo Quattrone12, Yoonmi Hong11, Dinggang Shen11, Pew-Thian Yap11, Tommy Boshkovski10, Jennifer S. W. Campbell19, Nikola Stikov10, G. Bruce Pike20, Barbara B. Bendlin5,
Andrew L. Alexander5, Vivek Prabhakaran5, Adam Anderson21, Bennett A. Landman4,21, Erick J.Z. Canales-Rodrígue3,22,23, Muhamed Barakovic3,24, Jonathan Rafael-Patino3, Thomas Yu3, Gaëtan Rensonnet3,25, Simona Schiavi3,26, Alessandro Daducci26, Marco Pizzolato3, Elda Fischi-Gomez3,24,
Jean-Philippe Thiran2,3, George Dai27, Giorgia Grisot28, Nikola Lazovski29, Albert Puente29, Matt Rowe29, Irina Sanchez29, Vesna Prchkovska29, Robert Jones1, Julia Lehman30, Suzanne Haber30, and Anastasia Yendiki1
1Athinoula A. Martinos Center for Biomedical Imaging, Massachusetts General Hospital and Harvard Medical School, Charlestown, MA, United States, 2Radiology Department, Centre Hospitalier Universitaire Vaudois and University of Lausanne, Lausanne, Switzerland, 3Signal Processing Lab (LTS5), École Polytechnique Fédérale de Lausanne, Lausanne, Switzerland, 4Institute of Imaging Science, Vanderbilt University, Nashville, TN, United States, 5University of Wisconsin, Madison, WI, United States, 6Department of Neuroscience and Biomedical Engineering, Aalto University, Helsinki, Finland, 7Department of Biomedical Engineering, Faculty of Engineering, Yeditepe University, Instanbul, Turkey, 8Department of Neurological Surgery, University of Pittsburgh, Pittsburgh, PA, United States, 9Department
of Neuroscience, Brighton and Sussex Medical School, University of Sussex, Brighton, United Kingdom, 10NeuroPoly Lab, Polytechnique Montreal, Montreal, QC, Canada, 11Department of Radiology and BRIC, University of North Carolina, Chapel Hill, NC, United States, 12Neuroscience Research Center, University Magna Graecia of Catanzaro, Catanzaro, Italy, 13CNRS/ISC, Bron, France, 14Université de Bordeaux, Bordeaux, France, 15CNRS/INCIA, Bordeaux, France, 16Center for Advanced Imaging Innovation and Research (CAI2 R), NYU School of Medicine, New York, NY, United States, 17Center for Biomedical Imaging, Dept. of Radiology, NYU School of Medicine, New York, NY, United States, 18Boston Children's Hospital, Boston, MA, United States, 19Montreal
Neurological Institute, McGill University, Montreal, QC, Canada, 20Hotchkiss Brain Institute and Department of Radiology, University of Calgary, Calgary, AB, Canada, 21Department of Electrical Engineering, Vanderbilt University, Nashville, TN, United States, 22FIDMAG Germanes Hospitalàries, Sant Boi de Llobregat, Barcelona, Spain, 23Mental Health Research Networking Center (CIBERSAM), Madrid, Spain, 24Translational Imaging in Neurology (ThINK), Department of Medicine and Biomedical Engineering, University Hospital and University of Basel, Basel, Switzerland, 25ICTEAM Institute, Université Catholique de Louvain, Louvain-la-Neuve, Belgium, 26Computer Science Department, University of Verona, Verona, Italy, 27Wellesley College, Wellesley, Wellesley, MA, United States, 28DeepHealth,
Inc., Cambridge, MA, United States, 29QMENTA, Inc., Barcelona, Spain, 30Department of Pharmacology and Physiology, University of Rochester School of Medicine, Rochester, NY, United States
Watch the Video
We present results from IronTract, the first challenge to evaluate tractography on the two-shell diffusion scheme of the Human Connectome Project (HCP). Accuracy was evaluated by comparison to tracer injections in the same macaque brains as the diffusion data. Training and validation datasets involved different injection sites. We observed that optimizing data analysis with respect to one injection site does not guarantee optimality for another; encouragingly, two teams could achieve consistently high performance in both datasets. We also found that, when analysis methods are optimized, the HCP scheme may achieve similar accuracy as a more demanding diffusion spectrum imaging acquisition.
|
|
0850.
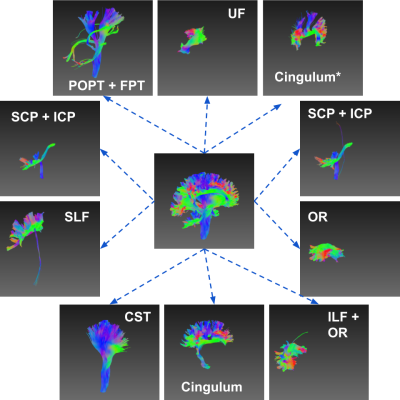 |
Auto-encoded Latent Representations of White Matter Streamlines
Shenjun Zhong1, Zhaolin Chen1, and Gary Egan1,2
1Monash Biomedical Imaging, Monash University, Australia, Melbourne, Australia, 2School of Psychological Sciences, Monash University, Australia, Melbourne, Australia
Watch the Video
Clustering white matter streamlines is still a challenging task. The existing methods based on spatial coordinates rely on manually engineered features, and/or labeled dataset. This work introduced a novel method that solves the problem of streamline clustering without needing labeled data. This is achieved by training a deep LSTM-based autoencoder to learn and embed any lengths of streamlines into a fixed-length vector, i.e. latent representation, then perform clustering in an unsupervised learning manner.
|
|
0851.
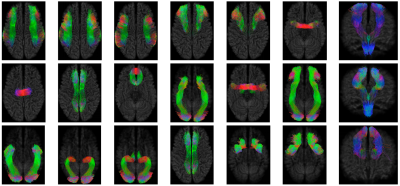 |
Tract Dictionary Learning for Fast and Robust Recognition of Fiber Bundles
Ye Wu1, Yoonmi Hong1, Weili Lin1, Pew-Thian Yap1, and the UNC/UMN Baby Connectome Project Consortium1
1Department of Radiology and BRIC, University of North Carolina, Chapel Hill, Chapel Hill, NC, United States
Watch the Video
Fiber bundle parcellation is key to bundle-specific analysis of white matter pathways.In this abstract, we propose an efficient framework for parcellation of white matter tractograms using discriminative dictionary learning. The key to our framework is to learn a compact dictionary for each fiber bundle so that the streamlines within the bundle can be succinctly represented. Experiments on a bundle-labeled HCP dataset and an infant dataset highlight the ability of our framework in grouping streamlines into anatomically plausible bundles.
|
|
0852.
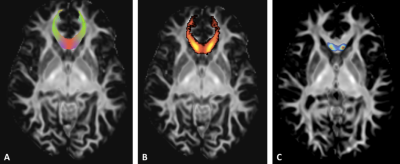 |
TractLearn: a geodesic learning framework for quantitative dissection of brain bundles
Arnaud Attyé1,2, Felix Renard3, Monica Baciu2, Elise Roger2, Laurent Lamalle4, Patrick Dehail5, Hélène Cassoudesalle5, and Fernando Calamante6,7
1School of Biomedical Engineering, University of Sydney, Sydney, Australia, 2CNRS LPNC UMR 5105, University of Grenoble Alpes, Grenoble, France, 3Laboratoire d'informatique de Grenoble, Grenoble, France, 4University of Grenoble Alpes, Grenoble, France, 5Bordeaux Universitary Hospital, Bordeaux, France, 6Sydney Imaging Lab, University of Sydney, Sydney, Australia, 7School of Aerospace, Mechanical and Mechatronic Engineering, Sydney, Australia
Watch the Video
Here we present a unified framework for brain fascicles quantitative analyses by geodesic learning (TractLearn) — as a data-driven unsupervised learning task. TractLearn allows a mapping between the image high-dimensional domain and the reduced latent space of brain fascicles. Besides providing a framework to test the reliability of various brain metrics with a global overview, it allows to identify subtle quantitative alteration in disease model with small subset of patients and/or data sparsity. With this regard, TractLearn is a ready-to-use algorithm for precision medicine.
|
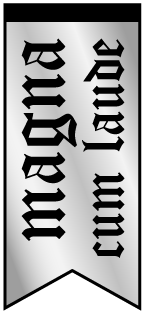 |
0853.
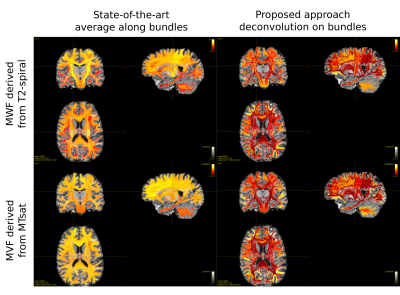 |
Myelin weighted tractography: a new way to investigate bundles myelination in-vivo?
Simona Schiavi1,2, Po-Jui Lu3,4, Matthias Weigel3,4,5, Derek K. Jones6,7,8, Ludwig Kappos3,4, Cristina Granziera3,4, and Alessandro Daducci1
1Department of Computer Science, University of Verona, Verona, Italy, 2DINOGMI, University of Genoa, Genoa, Italy, 3Departments of Medicine, Clinical Research and Biomedical Engineering, University Hospital Basel and University of Basel, Basel, Switzerland, 4Translational Imaging in Neurology (ThINk) Basel, Department of Medicine and Biomedical Engineering, University Hospital Basel and University of Basel, Basel, Switzerland, 5Radiological Physics, Department of Radiology, University Hospital Basel and University of Basel, Basel, Switzerland, 6Cardiff University Brain Research Imaging Centre, Cardiff University, Cardiff, United Kingdom, 7Neuroscience and Mental Health Research Institute, Cardiff University, Cardiff, United Kingdom, 8Mary MacKillop Institute for Health Research, Australian Catholic University, Melbourne, Australia
Watch the Video
Tractometry is a widely used tool to investigate microstructural properties that reflect differences in white matter tracts. It consists in averaging tissue features (obtained from any voxel-wise map) along streamlines recovered with diffusion tractography. To overcome the bias introduced by the average, we propose a new method to deconvolve on each individual tract the actual value measured by the microstructural map. For the first time we were able to assess the bundle-specific myelin content by combining tractography with two myelin-sensitive sequences and we obtained good agreement with previously reported values on the cortex.
|
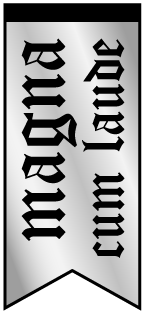 |
0854.
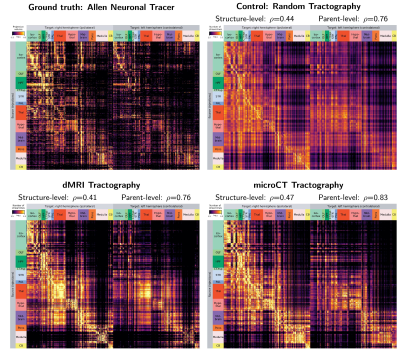 |
Synchrotron microCT tractography connectomics: comparison with diffusion MRI and neural tracer injections
Scott Trinkle1, Sean Foxley1, Narayanan Kasthuri2, and Patrick La Rivière1
1Department of Radiology, The University of Chicago, Chicago, IL, United States, 2Department of Neurobiology, The University of Chicago, Chicago, IL, United States
Watch the Video
In this study, we generated tractography-derived, mesoscale mouse “connectomes” from diffusion MRI and synchrotron microCT data on the same mouse brain and evaluated their accuracy using anterograde tracer data from the Allen Mouse Brain Connectivity Atlas. We are developing whole-brain synchrotron microCT, which provides micron-level isotropic resolution across whole mouse brains with no physical sectioning. Even when binning the microCT orientation information to the MRI resolution, microCT tractography outperformed MRI. However, both datasets performed similarly to a tractography connectome constructed entirely with random orientation data, highlighting the importance of understanding geometric biases in tractography connectome construction.
|
|
0855.
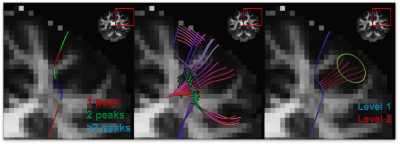 |
Anatomically informed multi-level fiber tractography for improved sensitivity of white matter bundle reconstruction in diffusion MRI
Andrey Zhylka1, Alexander Leemans2, Josien Pluim1, and Alberto De Luca2
1Biomedical Engineering, Eindhoven University of Technology, Eindhoven, Netherlands, 2Image Sciences Institute, University Medical Center Utrecht, Utrecht, Netherlands
Watch the Video
Neurosurgery planning is an important application of fiber tractography which requires the results to be consistent and accurate. Deterministic tractography methods are generally characterized by high specificity and limited sensitivity, whereas the opposite typically holds for probabilistic methods. Here, we propose a multi-level fiber tractography strategy that takes fiber branching into account and incorporates an anatomical prior to provide a balance between true and false positive reconstructions. We evaluated our approach on the MASSIVE dataset and compared its performance to the existing state of art.
|
|
0856.
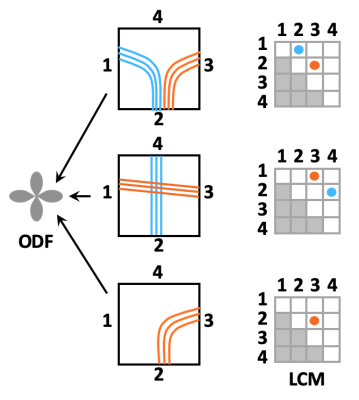 |
Towards taking the guesswork (and the errors) out of diffusion tractography
Anastasia Yendiki1, Robert Jones2, Adrian Dalca1,3, Hui Wang1, and Bruce Fischl1
1Radiology, Harvard Medical School & Massachusetts General Hospital, Charlestown, MA, United States, 2Radiology, Massachusetts General Hospital, Charlestown, MA, United States, 3Massachusetts Institute of Technology, Cambridge, MA, United States
Watch the Video
Orientation distributions estimated from diffusion MRI have multiple peaks per voxel. Tractography algorithms must choose among them arbitrarily, leading to errors. We propose a novel approach to making this choice in a manner informed by the data. We use post mortem optical and MRI data to train a convolutional neural network that can recognize voxel-wise connection patterns directly from diffusion data, circumventing the conventional paradigm of an orientation distribution. We introduce TRACARIS (TRACt Architectures Recovered from Imaging Signals), a tractography algorithm that uses these network-predicted, local connection patterns. We present preliminary validation results from a post mortem human brain sample.
|
|
0857.
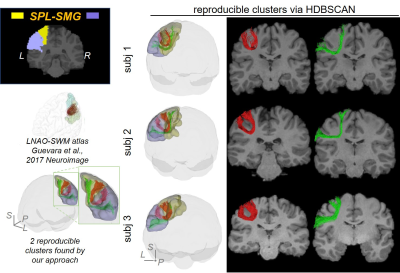 |
Short-range Tractography with high Throughput And Reproducibility (STTAR) characterized by FDT tracing and HDBSCAN clustering
Chenying Zhao1,2, Minhui Ouyang1, Qinlin Yu1, and Hao Huang1,3
1Department of Radiology, Children's Hospital of Philadelphia, Philadelphia, PA, United States, 2Department of Bioengineering, School of Engineering and Applied Science, University of Pennsylvania, Philadelphia, PA, United States, 3Department of Radiology, Perelman School of Medicine, University of Pennsylvania, Philadelphia, PA, United States
Watch the Video
Short-range association fibers (SAFs), linking adjacent cortical regions, are dominant in structural connectome and associated with autism and schizophrenia. However, SAFs are not well characterized due to challenges in high-throughput tracing of SAF with diffusion MRI and challenges of identifying and labeling reproducible SAFs. The vast amount of SAFs also make it difficult to delineate them. To meet these challenges, we established a protocol “STTAR” including high-throughput streamline tracing with a regularized FDT probabilistic tractography and semi-automatic identification of reproducible SAFs with novel HDBSCAN clustering. Newly identified reproducible SAFs and those consistently reported in the literature are also demonstrated.
|
|