Thursday Parallel 2 Live Q&A |
Thursday, 13 August 2020, 15:50 - 16:35 UTC |
Moderators: C. C. Tchoyoson Lim & Ona Wu |
Session Number: PP-03
|
1294.
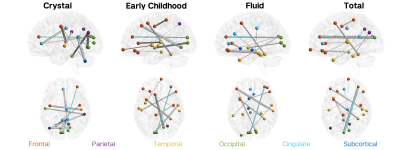 |
Hybrid structure-function connectome predicts individual cognitive abilities
Elvisha Dhamala1,2, Keith W Jamison1, Sarah M Dennis3, Raihaan Patel4,5, M Mallar Chakravarty4,5,6, and Amy Kuceyeski1,2
1Radiology, Weill Cornell Medicine, New York, NY, United States, 2Neuroscience, Weill Cornell Medicine, New York, NY, United States, 3Sarah Lawrence College, Bronxville, NY, United States, 4Biological and Biomedical Engineering, McGill University, Montreal, QC, Canada, 5Cerebral Imaging Centre, Douglas Mental Health University Institute, Montreal, QC, Canada, 6Psychiatry, McGill University, Montreal, QC, Canada
Watch the Video
Structural connectivity (SC) and functional connectivity (FC) can be independently used to predict cognition and show distinct patterns of variance in relation to cognition. No work identified has yet investigated whether SC and FC can be combined to better predict cognitive abilities. In this work, we aimed to predict cognitive measures in 785 healthy adults using a hybrid structure-function connectome and quantify the most important connections. We show that: 1) hybrid connectomes explain 15% of the variance in individual cognitive measures, and 2) long-range cortico-cortical functional connections and short-range cortico-subcortical and subcortico-subcortical structural connections are most important for the prediction.
|
|
1295.
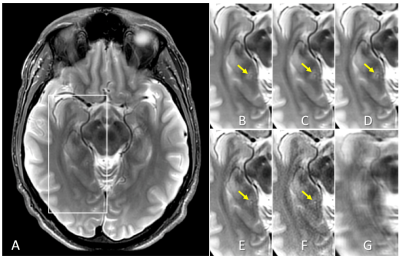 |
Contrast-weighted SSIM loss function for deep learning-based undersampled MRI reconstruction
Sangtae Ahn1, Anne Menini2, Graeme McKinnon3, Erin M. Gray4, Joshua D. Trzasko4, John Huston4, Matt A. Bernstein4, Justin E. Costello5, Thomas K. F. Foo1, and Christopher J. Hardy1
1GE Research, Niskayuna, NY, United States, 2GE Healthcare, Menlo Park, CA, United States, 3GE Healthcare, Waukesha, WI, United States, 4Department of Radiology, Mayo Clinic College of Medicine, Rochester, MN, United States, 5Walter Reed National Military Medical Center, Bethesda, MD, United States
Watch the Video
Deep learning-based undersampled MRI reconstructions can result in visible blurring, with loss of fine detail. We investigate here various structural similarity (SSIM) based loss functions for training a compressed-sensing unrolled iterative reconstruction, and their impact on reconstructed images. The conventional unweighted SSIM has been used both as a loss function, and, more generally, for assessing perceived image quality in various applications. Here we demonstrate that using an appropriately weighted SSIM for the loss function yields better reconstruction of small anatomical features compared to L1 and conventional SSIM loss functions, without introducing image artifacts.
|
|
1296.
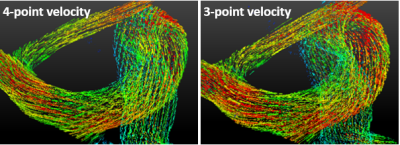 |
Accelerated 4D-flow MRI using Machine Learning (ML) Enabled Three Point Flow Encoding
Dahan Kim1,2, Laura Eisenmenger3, and Kevin M. Johnson3,4
1Department of Medical Physics, University of Wisconsin, Madison, WI, United States, 2Department of Physics, University of Wisconsin, Madison, WI, United States, 3Department of Radiology, University of Wisconsin, Madison, WI, United States, 4Department of Medical Physics, University of Wisconsin, Middleton, WI, United States
Watch the Video
4D-flow MRI suffers from long scan time due to a minimum of four velocity encodings necessary to solve for three velocity components and the reference background phase. We examine the feasibility of using machine learning (ML) to determine the background phase and hence three velocity components from only three flow encodings. The results show that ML is capable of estimating three-directional velocities from three flow encodings with high accuracy (1.5%-3.8% velocity underestimation) and high precision (R2=0.975). These findings indicate that 4D-flow MRI can be accelerated without requiring a dedicated reference scan, with a scan time reduction of 25%.
|
|
1297.
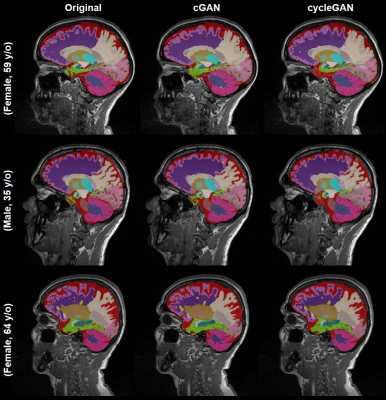 |
A practical application of generative models for MR image synthesis: from post- to pre-contrast imaging
Gian Franco Piredda1,2,3, Virginie Piskin1, Vincent Dunet2, Gibran Manasseh2, Mário J Fartaria1,2,3, Till Huelnhagen1,2,3, Jean-Philippe Thiran2,3, Tobias Kober1,2,3, and Ricardo Corredor-Jerez1,2,3
1Advanced Clinical Imaging Technology, Siemens Healthcare AG, Lausanne, Switzerland, 2Department of Radiology, Lausanne University Hospital and University of Lausanne, Lausanne, Switzerland, 3LTS5, École Polytechnique Fédérale de Lausanne (EPFL), Lausanne, Switzerland
Watch the Video
Multiple sclerosis studies following the widely accepted MAGNIMS protocol guidelines might lack non-contrast-enhanced T1-weighted acquisitions as they are only considered optional. Most existing automated tools to perform morphological brain analyses are, however, tuned to non-contrast T1-weighted images. This work investigates the use of deep learning architectures for the generation of pre-Gadolinium from post-Gadolinium image volumes. Two generative models were tested for this purpose. Both were found to yield similar contrast information as the original non-contrast T1-weighted images. Quantitative comparison using an automated brain segmentation on original and synthesized non-contrast T1-weighted images showed good correlation (r=0.99) and low bias (<0.7 ml).
|
|
1298.
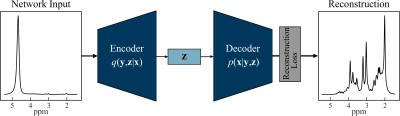 |
Quantification of Non-Water-Suppressed Proton Spectroscopy using Deep Neural Networks
Marcia Sahaya Louis1, Eduardo Coello2, Huijun Liao2, Ajay Joshi3, and Alexander Lin2
1ECE, Boston University, Boston, MA, United States, 2Radiology, Brigham and Women's hospital, Boston, MA, United States, 3Boston University, Boston, MA, United States
Watch the Video
Water is present in the brain tissue at a concentration that is at least four orders of magnitude higher than metabolites of interest. As a result, it is necessary to suppress the water resonance so that the brain metabolites of interest can be better visualized and quantified. This work presents a neural network model for extracting the metabolites spectrum from non-water-suppressed proton magnetic resonance spectra. The autoencoder model learns a vector field for mapping the water signal to a lower-dimensional manifold and accurately reconstructs the metabolite spectra as compared to water-suppressed spectra from the same subject.
|
|
1299.
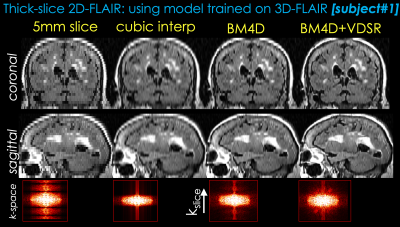 |
From 2D thick slices to 3D isotropic volumetric brain MRI - a deep learning approach
Berkin Bilgic1,2, Long Wang1, Enhao Gong1, Greg Zaharchuk1,3, and Tao Zhang1
1Subtle Medical Inc, Menlo Park, CA, United States, 2Martinos Center for Biomedical Imaging, MGH/Harvard, Charlestown, MA, United States, 3Stanford University, Stanford, CA, United States
Watch the Video
The long scan time of 3D isotropic MRI (often 5 minutes or longer) has limited the wide clinical adoption despite the apparent advantages. For many clinical sites, shorter 2D sequences are used routinely in brain MRI exams instead. The latest development of deep learning (DL) has demonstrated the feasibility of significant resolution improvement from low resolution acquisitions. In this work, we propose a deep learning method to synthesize 3D isotropic FLAIR images from 2D FLAIR acquisition with 5mm slice thickness. To demonstrate the generalizability, the proposed method is validated on both simulated and real 2D FLAIR datasets.
|
|
1300.
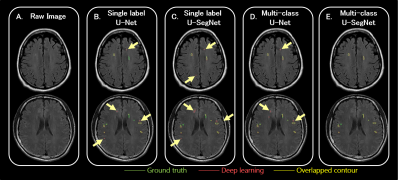 |
Deep Learning Multi-class Segmentation Algorithm is more Resilient to the Variations of MR Image Acquisition Parameters
Yi-Tien Li1,2, Yi-Wen Chen1, David Yen-Ting Chen1,3, and Chi-Jen Chen1,4
1Department of Radiology, Taipei Medical University - Shuang Ho Hospital, New Taipei, Taiwan, 2Institute of Biomedical Engineering, National Taiwan University, Taipei, Taiwan, 3Department of Radiology, Stanford University, Palo Alto, CA, United States, 4School of Medicine, College of Medicine, Taipei Medical University, Taipei, Taiwan
A huge amount of T2-FLAIR images with appearance of white matter hyperintencities (WMH) were used. 1368 cases from one hospital were selected as the training set. Another 100 cases from the same hospital and 200 cases from the other 2 different hospitals were treated as the independent test set. Based on multi-class U-SegNet approach, it can achieve the highest F1 score (same hospital: 90.01%; different hospital: 86.52%) in the test set compared with other approaches. The result suggested that the multi-class segmentation approach is more resilient to the variations of MR image parameters than the single label segmentation approach.
|
|
1301.
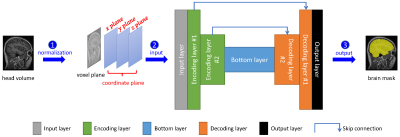 |
Accurate Brain Extraction Using 3D U-Net with Encoded Spatial Information
Hualei Shen1, Chenyu Wang1,2, Kain Kyle2, Chun-Chien Shieh2,3, Lynette Masters4, Fernando Calamante1,5, Dacheng Tao6, and Michael Barnett1,2
1Brain and Mind Centre, the University of Sydney, Sydney, Australia, 2Sydney Neuroimaging Analysis Centre, Sydney, Australia, 3Sydney Medical School, the University of Sydney, Sydney, Australia, 4I-MED Radiology Network, Sydney, Australia, 5Sydney Imaging and School of Biomedical Engineering, the University of Sydney, Sydney, Australia, 6School of Computer Science, the University of Sydney, Sydney, Australia
Watch the Video
Brain extraction from 3D MRI datasets using existing 3D U-Net convolutional neural networks suffers from limited accuracy. Our proposed method overcame this challenge by combining a 3D U-Net with voxel-wise spatial information. The model was trained with 1,615 T1 volumes and tested on another 601 T1 volumes, both with expertly segmented labels. Results indicated that our method significantly improved the accuracy of brain extraction over a conventional 3D U-Net. The trained model extracts the brain from a T1 volume in ~2 minutes and has been deployed for routine image analyses at the Sydney Neuroimaging Analysis Centre.
|
|
1302.
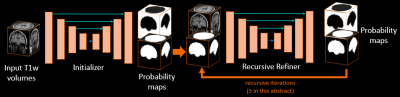 |
3D DUAL RECURSIVE REFINER NETWORK FOR ROBUST SEGMENTATION: APPLICATION TO BRAIN EXTRACTION
Maxime Bertrait1, Pascal Ceccaldi1, Boris Mailhé1, Youngjin Yoo1, and Mariappan S. Nadar1
1Digital Technology and Innovation, Siemens Healthineers, Princeton, NJ, United States
Watch the Video
In Magnetic Resonance Imaging, acquisition protocol may varies from one clinical task to another affecting the resulting reconstructed scan in terms of field of view and resolution. In research, 3D acquired MRI scans are widely available providing high quality isotropic medical images but is far from what can exist in clinical environment such as 2D multi-slices with thick slices acquisition that can provide anisotropic medical images. We then present a framework, through a brain extraction task, called Dual Recursive Refiner able to work with both acquisitions. The presented framework outperforms baseline architectures for segmentation on both isotropic and anisotropic data.
|
|
1303.
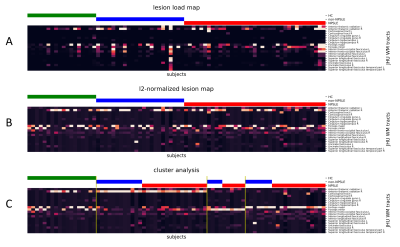 |
Characterisation of white matter lesion patterns in Systemic Lupus Erythematosus by an unsupervised machine learning approach.
Theodor Rumetshofer1, Tor Olof Strandberg2, Peter Mannfolk3, Andreas Jönsen4, Markus Nilsson1, Johan Mårtensson1, and Pia Maly Sundgren1,5
1Department of Clinical Sciences Lund/Diagnostic Radiology, Lund University, Lund, Sweden, 2Clinical Memory Research Unit, Department of Clinical Sciences, Malmö, Lund University, Lund, Sweden, 3Clinical Imaging and Physiology, Skåne University Hospital, Lund, Sweden, 4Department of Reumatology, Skåne University Hospital, Lund, Sweden, 5Department of Clinical Sciences/Centre for Imaging and Function, Skåne University Hospital, Lund, Sweden
Watch the Video
Evaluating white matter hyperintensities (WMHs) in neuropsychiatric systemic lupus erythematosus (NPSLE) is a challenging task. Multimodal MRI images in combination with unsupervised machine characterization can provide a powerful tool to investigate the spatial WHM distribution of relevant phenotypes. Automatically segmented WMH maps were spatially allocated to a white matter tract atlas. Cluster analysis was applied on this tract-wise lesion-load map to obtain subtypes with a distinct WMH damage profile. This approach on microstructural changes could help to identify specific progression pattern which may improve the accuracy of NPSLE classification.
|
|
1304.
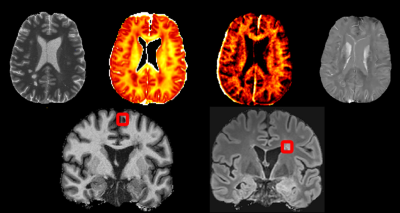 |
Attention-based convolutional network quantifying the importance of quantitative MR metrics in the multiple sclerosis lesion classification
Po-Jui Lu1,2,3, Reza Rahmanzadeh1,2, Riccardo Galbusera1,2, Matthias Weigel1,2,4, Youngjin Yoo3, Pascal Ceccaldi3, Yi Wang5, Jens Kuhle2, Ludwig Kappos1,2, Philippe Cattin6, Benjamin Odry7, Eli Gibson3, and Cristina Granziera1,2
1Translational Imaging in Neurology (ThINk) Basel, Department of Medicine and Biomedical Engineering, University Hospital Basel and University of Basel, Basel, Switzerland, 2Neurologic Clinic and Policlinic, Departments of Medicine, Clinical Research and Biomedical Engineering, University Hospital Basel and University of Basel, Basel, Switzerland, 3Digital Technology and Innovation, Siemens Healthineers, Princeton, NJ, United States, 4Radiological Physics, Department of Radiology, University Hospital Basel, Basel, Switzerland, 5Department of Radiology, Weill Cornell Medical College, New York, NY, United States, 6Center for medical Image Analysis & Navigation, Department of Biomedical Engineering, University of Basel, Basel, Switzerland, 7Covera Health, New York, NY, United States
Video Permission Withheld
White matter lesions in multiple sclerosis patients exhibit distinct characteristics depending on their locations in the brain. Multiple quantitative MR sequences sensitive to white matter micro-environment are necessary for the assessment of those lesions; but how to judge which sequences contain the most relevant information remains a challenge. In this abstract, we are proposing a convolutional neural network with a gated attention mechanism to quantify the importance of MR metrics in classifying juxtacortical and periventricular lesions. The results show the statistically significant order of quantitative importance of metrics, one step closer to combining more relevant metrics for better interpretation.
|
|
1305.
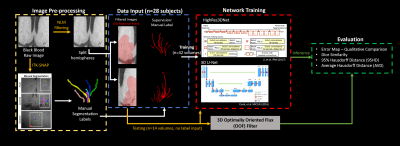 |
Deep Learning Segmentation of Lenticulostriate Arteries on 3D Black Blood MRI
Samantha J Ma1, Mona Sharifi Sarabi1, Kai Wang1, Soroush Heidari Pahlavian1, Wenli Tan1, Madison Lodge1, Lirong Yan1, Yonggang Shi1, and Danny JJ Wang1
1University of Southern California, Los Angeles, CA, United States
Watch the Video
Cerebral small vessels are largely inaccessible to existing clinical in vivo imaging technologies. As such, early cerebral microvascular morphological changes in small vessel disease (SVD) are difficult to evaluate. A deep learning (DL)-based algorithm was developed to automatically segment lenticulostriate arteries (LSAs) in 3D black blood images acquired at 3T. Using manual segmentations as supervision, 3D segmentation of LSAs is demonstrated to be feasible with relatively high performance and can serve as a useful tool for quantitative morphometric analysis in patients with cerebral SVD.
|
|
1306.
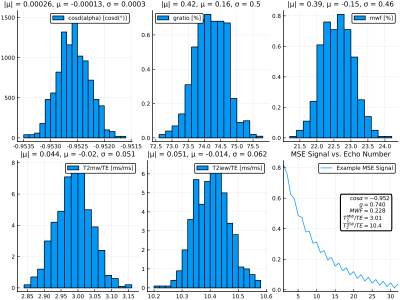 |
Bayesian learning for fast parameter inference of multi-exponential white matter signals
Jonathan Doucette1,2, Christian Kames1,2, and Alexander Rauscher1,3,4
1UBC MRI Research Centre, Vancouver, BC, Canada, 2Department of Physics & Astronomy, University of British Columbia, Vancouver, BC, Canada, 3Department of Pediatrics, Faculty of Medicine, University of British Columbia, Vancouver, BC, Canada, 4Division of Neurology, Faculty of Medicine, University of British Columbia, Vancouver, BC, Canada
Watch the Video
In this work we use Bayesian learning methods to investigate data-driven approaches to parameter inference of multi-exponential white matter signals. Multi spin-echo (MSE) signals are simulated by solving the Bloch-Torrey on 2D geometries containing myelinated axons, and a conditional variational autoencoder (CVAE) model is used to learn to map simulated signals to posterior parameter distributions. This approach allows for the mapping of MSE signals directly to physical parameter vectors without expensive post-processing. We demonstrate the effectiveness of this model through the simultaneous inference of the myelin water fraction, flip angle, intra-/extracullar water $$$T_2$$$, myelin water $$$T_2$$$, and myelin g-ratio.
|
|
1307.
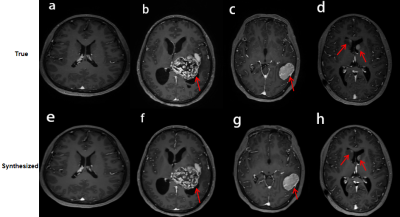 |
Clinical performance of reduced gadolinium dose for contrast-enhanced brain MRI using deep learning
Huanyu Luo1, Jing Xue1, Yunyun Duan1, Cheng Xu1, Jonathan Tamir2, Srivathsa Pasumarthi Venkata2, and Yaou Liu1
1Radiology, Beijing Tiantan Hospital, Beijing, China, 2Subtle Medical Inc, Menlo Park, CA, United States
Watch the Video
The reported gadolinium deposition phenomenon has caused extensive concern in the radiology community. This study focuses on validating the clinical performance of a proposed deep learning architecture which can significantly reduce the dosage of gadolinium-based contrast agents (GBCA) in brain MRI. The results suggest that the synthesized contrast images using deep learning with reduced GBCA dose can maintain its diagnostic quality under certain clinical circumstances.
|
|
1308.
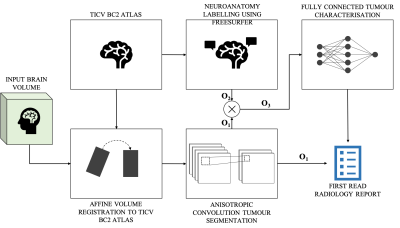 |
Deep learning Assisted Radiological reporT (DART)
Keerthi Sravan Ravi1,2, Sairam Geethanath2, Girish Srinivasan3, Rahul Sharma4, Sachin R Jambawalikar4, Angela Lignelli-Dipple4, and John Thomas Vaughan Jr.2
1Biomedical Engineering, Columbia University, New York, NY, United States, 2Columbia Magnetic Resonance Research Center, Columbia University, New York, NY, United States, 3MediYantri Inc., Palatine, IL, United States, 4Columbia University Irving Medical Center, New York, NY, United States
Watch the Video
A 2015 survey indicates that burnout of radiologists was seventh highest among all physicians. In this work, two neural networks are designed and trained to generate text-based first read radiology reports. Existing tools are leveraged to perform registration and then brain tumour segmentation. Feature vectors are constructed utilising the information extracted from the segmentation masks. These feature vectors are fed to the neural networks to train against a radiologist’s reports on fifty subjects. The neural networks along with image statistics are able to characterise tumour type, mass effect and edema and report tumour volumetry; compiled as a first-read radiology report.
|
|