Tuesday Parallel 1 Live Q&A |
Tuesday, 11 August 2020, 14:30 - 15:15 UTC |
Moderators: Puneet Bagga & Ferdinand Schweser |
Session Number: PP-21
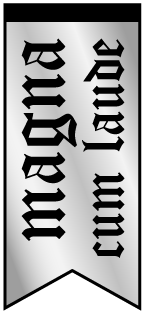 |
0507.
 |
Tailored spectral-spatial saturation pulses for spatially uniform saturation in CEST imaging
Huiwen Luo1, Wissam AlGhuraibawi2, Kevin Godines2, Daniel Gochberg3, Moriel Vandsburger2, and William A Grissom1
1Biomedical Engineering, Vanderbilt University, Nashville, TN, United States, 2Department of Bioengineering, University of California Berkeley, Berkeley, CA, United States, 3Radiology and Radiological Sciences, Vanderbilt University, Nashville, TN, United States
Watch the Video
A tailored spectral-spatial saturation pulse was developed to produce a flat flip angle profile across the heart and achieve more uniform CEST saturation despite B1 inhomogeneity at 3 Tesla. The tailored saturation pulse train was simulated for a two-pool system to evaluate the z-spectrum at each spatial location in the heart, based on an in vivo 3 Tesla B1 map. Whereas CEST saturation generated with a conventional Gaussian pulse yielded CEST contrast of 2.60±1.59% across the ventricle, the tailored pulse produced more uniform saturation across the heart which resulted in both greater and more uniform CEST contrast of 4.64±0.34%.
|
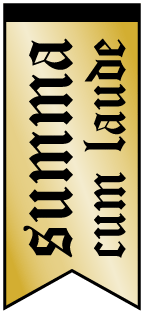 |
0508.
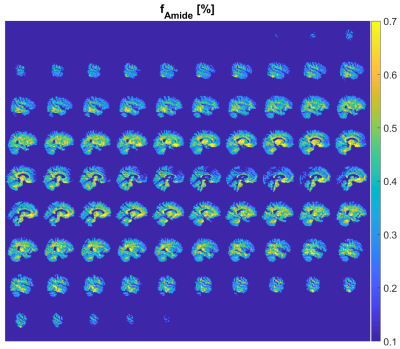 |
Towards clinical CEST-MRF: whole brain snapshot CEST MR Fingerprinting at 3T using spin-lock saturation and a centric 3D-EPI readout
Kai Herz1, Sebastian Mueller1, Or Perlman2, Ruediger Stirnberg3, Tony Stoecker3,4, Klaus Scheffler1,5, Christian Farrar2, and Moritz Zaiss1,6
1Magnetic Resonance Center, Max Planck Institute for Biological Cybernetics, Tuebingen, Germany, 2Athinoula A. Martinos Center for Biomedical Imaging, Department of Radiology, Massachusetts General Hospital and Harvard Medical School, Charlestown, MA, United States, 3German Center for Neurodegenerative Diseases (DZNE), Bonn, Germany, 4Department of Physics and Astronomy, University of Bonn, Bonn, Germany, 5Department of Biomedical Magnetic Resonance, Eberhard Karls University Tuebingen, Tuebingen, Germany, 6Department of Neuroradiology, University Hospital Erlangen, Erlangen, Germany
Watch the Video
Quantitative CEST imaging is still not applied in clinical routine, as both quantification and whole brain coverage require usually long scan times. In this work, we present a CEST-MRF protocol using spin-lock saturation pulses and a fast 3D-EPI readout with whole brain coverage. This enables a fast generation of quantitative amide proton concentration maps of the entire brain at a clinical scanner.
|
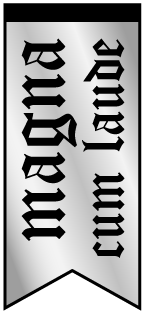 |
0509.
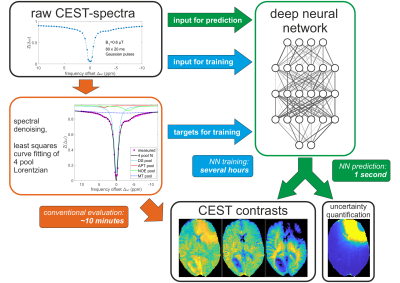 |
DeepCEST 3T: Robust neural network prediction of 3T CEST MRI parameters including uncertainty quantification
Felix Glang1, Anagha Deshmane1, Sergey Prokudin2, Florian Martin1, Kai Herz1, Tobias Lindig3, Benjamin Bender3, Klaus Scheffler1,4, and Moritz Zaiss1,5
1Magnetic Resonance Center, Max Planck Institute for Biological Cybernetics, Tübingen, Germany, 2Department of Perceiving Systems, Max Planck Institute for Intelligent Systems, Tübingen, Germany, 3Department of Diagnostic and Interventional Neuroradiology, Eberhard Karls University Tübingen, Tübingen, Germany, 4Department of Biomedical Magnetic Resonance, Eberhard Karls University Tübingen, Tübingen, Germany, 5Department of Neuroradiology, University Clinic Erlangen, Erlangen, Germany
Watch the Video
Analysis of CEST data often requires complex mathematical modeling before contrast generation, which can be error prone and time-consuming. Here, a probabilistic deep learning approach is introduced to shortcut conventional Lorentzian fitting analysis of 3T in-vivo CEST data by learning from previously evaluated data. It is demonstrated that the trained networks generalize to data of a healthy subject and a brain tumor patient, providing CEST contrasts in a fraction of the conventional evaluation time. Additionally, the probabilistic network architecture enables uncertainty quantification, indicating if predictions are trustworthy, which is assessed by perturbation analysis.
|
|
0510.
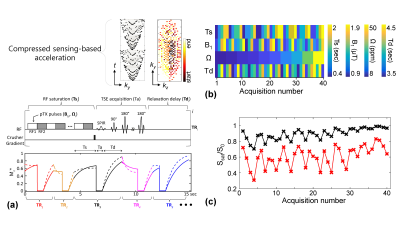 |
Unsupervised Deep Learning-based Magnetization Transfer Contrast (MTC) MR Fingerprinting and CEST MRI
Beomgu Kang1, Byungjai Kim1,2, Michael Schar2, Hyunwook Park1, and Hye Young Heo2,3
1Department of Electrical Engineeering, Korea Advanced Institute of Science and Technology, Daejeon, Korea, Republic of, 2Russell H Morgan Department of Radiology and Radiological Science, Johns Hopkin University, Baltimore, MD, United States, 3F.M. Kirby Research Center for Functional Brain Imaging, Kennedy Krieger Institute, Baltimore, MD, United States
Watch the Video
Most currently used MTC/CEST imaging protocols depend on the acquisition of qualitative weighted images, limiting the detection sensitivity to quantitative parameters, their exchange rate and concentration. Here, we propose a fast, quantitative 3D MTC/CEST imaging framework based on a combined 1) time-interleaved parallel RF transmission, 2) compressed sensing, 3) MR fingerprinting, and 4) deep-learning techniques. Typically, supervised deep learning requires a massive amount of labeled images for training, which is limited particularly in MTC/CEST MRI field. However, the proposed unsupervised learning architecture requires only small amounts of unlabeled MTC/CEST data.
|
|
0511.
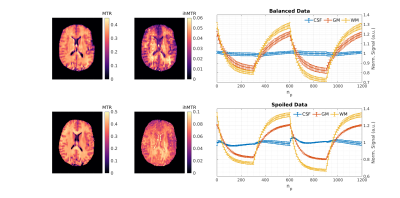 |
Transient-State Inhomogeneous Magnetisation Transfer: Towards Magnetisation Transfer Fingerprinting
Daniel J. West1, Gastao Cruz1, Olivier Jaubert1, Rui P. A. G. Teixeira1,2, Torben Schneider3, Jacques-Donald Tournier1,2, Jo Hajnal1,2, Claudia Prieto1, and Shaihan J. Malik1,2
1School of Biomedical Engineering and Imaging Sciences, King's College London, London, United Kingdom, 2Centre for The Developing Brain, King's College London, London, United Kingdom, 3Philips Healthcare, Guildford, United Kingdom
Watch the Video
Inhomogeneous magnetisation transfer (ihMT) is a contrast mechanism that has shown high specificity towards myelinated tissue. Contrast is typically generated using sequences comprising a preparation phase with several RF saturation pulses, followed by multiple readout periods for measurement. Here, we present a transient acquisition scheme that alternates between periods of multi-band and single-band RF pulses, to efficiently generate ihMT contrast during a single data acquisition. Since signal is transiently varying throughout, we use a dictionary-based low-rank inversion reconstruction method originally proposed for magnetic resonance fingerprinting. Simulation, phantom and human in-vivo experiments are included.
|
|
0512.
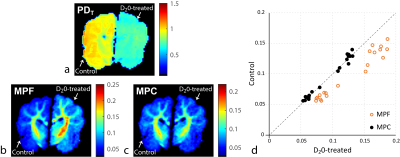 |
Towards Absolute Quantification of Macromolecular Proton Content using Cross-Relaxation Imaging
Alexey Samsonov1, Aaron Field1, Vasily Yarnykh2, and Julia Velikina3
1Radiology, University of Wisconsin, Madison, WI, United States, 2Radiology, University of Washington, Seattle, WA, United States, 3Medical Physics, University of Wisconsin, Madison, WI, United States
Watch the Video
Macromolecular proton fraction (MPF), the key two-pool MT model parameter, was established as a robust myelin-sensitive index, with clinical relevance in demyelinating diseases. However, as MPF assesses macromolecules relative to tissue water, its specificity to myelin is limited. i.e., MPF changes may occur independent of myelin, e.g., in the setting of inflammation and edema. Further, relating MPF to macromolecules may be ambiguous due to unequal concentrations of protons in macromolecular and water compartments. We demonstrate implications of these effects for MPF interpretation using phantom and ex-vivo experiments and propose a new macromolecular measure that explicitly accounts for tissue water effects.
|
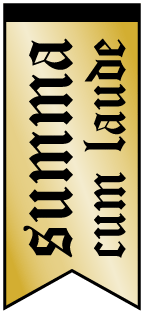 |
0513.
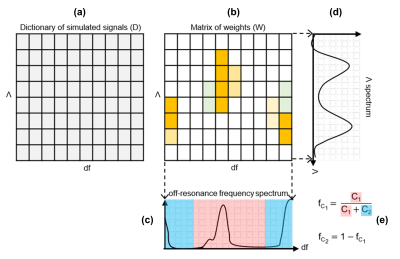 |
Fat fraction mapping using bSSFP Signal Profile Asymmetries for Robust multi-Compartment Quantification (SPARCQ)
Giulia MC Rossi1,2, Tom Hilbert1,2,3, Adèle LC Mackowiak1,2, Katarzyna Pierzchała4,5, Tobias Kober1,2,3, and Jessica AM Bastiaansen1
1Department of Diagnostic and Interventional Radiology, Lausanne University Hospital and University of Lausanne, Lausanne, Switzerland, 2Advanced Clinical Imaging Technology, Siemens Healthcare AG, Lausanne, Switzerland, 3LTS5, Ecole Polytechnique Fédérale de Lausanne (EPFL), Lausanne, Switzerland, 4Laboratory for Functional and Metabolic Imaging, Ecole Polytechnique Fédérale de Lausanne (EPFL), Lausanne, Switzerland, 5Center for Biomedical Imaging (CIBM), Ecole Polytechnique Fédérale de Lausanne (EPFL), Lausanne, Switzerland
Watch the Video
A novel quantitative framework for detection of different tissue compartments based on bSSFP signal profile asymmetries (SPARCQ) is reported. SPARCQ uses a dictionary-based weight optimization algorithm to estimate voxel-wise off-resonance frequency and relaxation time ratio spectra from acquired bSSFP signal profiles. From the obtained spectra, quantitative parameters (i.e. fractions of the components of interest, thermal equilibrium magnetization) can be extracted. Validation and proof-of-concept are provided for voxel-wise water-fat separation and fat fraction mapping. Accuracy and repeatability of SPARCQ are demonstrated with phantom and in vivo experiments.
|
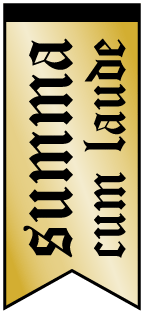 |
0514.
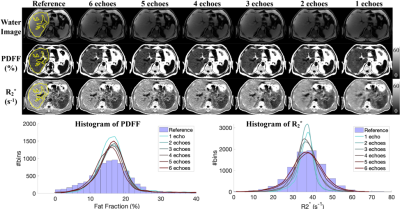 |
Deep Learning-based Quantification of Proton Density Fat Fraction and R2* in the Liver
Ante Zhu1,2, Yuxin Zhang2,3, Alan McMillan2, Fang Liu2, Timothy J Colgan2, Scott B. Reeder1,2,3,4,5, and Diego Hernando1,2,3,6
1Biomedical Engineering, University of Wisconsin-Madison, Madison, WI, United States, 2Radiology, University of Wisconsin-Madison, Madison, WI, United States, 3Medical Physics, University of Wisconsin-Madison, Madison, WI, United States, 4Emergency Medicine, University of Wisconsin-Madison, Madison, WI, United States, 5Medicine, University of Wisconsin-Madison, Madison, WI, United States, 6Electrical and Computer Engineering, University of Wisconsin-Madison, Madison, WI, United States
Watch the Video
Multi-echo chemical shift-encoded (CSE)-MRI techniques enable liver PDFF and R2* quantification, which enable staging and treatment monitoring of liver fat and iron content, respectively. However, the common requirement of breath-holding in CSE-MRI acquisitions is challenging for many patients. Furthermore, the required specialized multi-echo acquisition and reconstruction are not available in all scanners. In this work, we assessed the accuracy of deep learning (DL)-based PDFF and R2* quantification using reduced numbers of echoes. Preliminary results demonstrate the potential of this approach and suggest that at least four echoes are needed for quantifying PDFF and R2* at 1.5T and 3.0T.
|
|
0515.
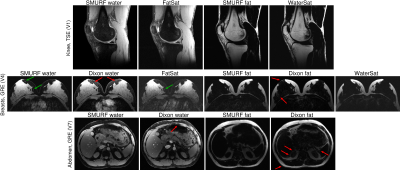 |
Simultaneous Multiple Resonance Frequency (SMURF) imaging: Fat-water imaging using multi-band principles
Beata Bachrata1,2,3, Bernhard Strasser1,2,4, Wolfgang Bogner1,2, Albrecht Ingo Schmid1,5, Siegfried Trattnig1,2,3, and Simon Daniel Robinson1,2,6,7
1High Field MR Centre, Medical University of Vienna, Vienna, Austria, 2Department of Biomedical Imaging and Image-guided Therapy, Medical University of Vienna, Vienna, Austria, 3Christian Doppler Laboratory for Clinical Molecular MR Imaging, Vienna, Austria, 4Athinoula A. Martinos Center for Biomedical Imaging, Department of Radiology, Massachusetts General Hospital, Harvard Medical School, Boston, MA, United States, 5Center for Medical Physics and Biomedical Engineering, Medical University of Vienna, Vienna, Austria, 6Centre for Advanced Imaging, The University of Queensland, Brisbane, Australia, 7Department of Neurology, Medical University of Graz, Graz, Austria
Watch the Video
Imaging of body regions containing both water-based and fat-based structures is affected by artefacts arising from the chemical shift difference between water and fat. Recently, a single-echo water-fat separation technique was proposed which used multi-band principles to generate separate water and fat images as well as chemical shift-corrected, recombined water-fat images. We demonstrate the performance of gradient-echo and turbo spin-echo variants of this approach in the knee, breasts and abdomen. The separation of water and fat was similar to or better than with current state-of-the-art techniques and chemical shift effects were fully eliminated in recombined water-fat images.
|
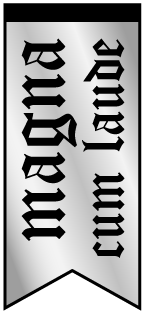 |
0516.
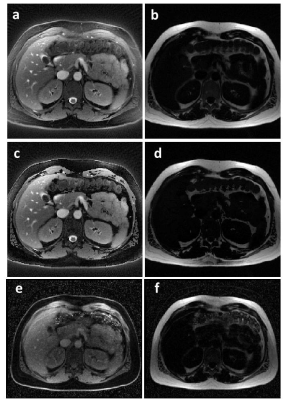 |
Fat suppression using a rosette trajectory for low field magnetic resonance imaging
Dominique Franson1, Yuchi Liu1,2, Rajiv Ramasawmy3, Adrienne Campbell-Washburn3, and Nicole Seiberlich1,2
1Case Western Reserve University, Cleveland, OH, United States, 2University of Michigan, Ann Arbor, MI, United States, 3National Heart, Lung, and Blood Institute, National Institutes of Health, Bethesda, MD, United States
Watch the Video
Fat/water separation at low field strengths can be difficult due to the small difference between resonance frequencies. Rosette trajectories have previously been shown to be effective for spectral separation and fat suppression, and the approach is not dependent on a large frequency difference. Here, a rosette trajectory is used to significantly suppress fat signal in water images, and to produce separate fat images at 0.55T. B0 maps are calculated from two of the rosette echoes, and are used to improve the fat/water separation. Initial examples are shown in an oil/water phantom, and in the heart and abdomen.
|
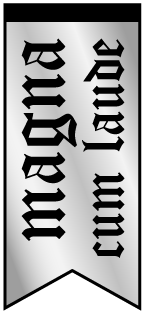 |
0517.
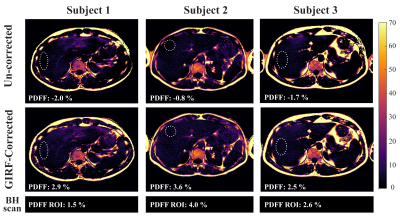 |
Correcting gradient chain-induced fat quantification errors in multi-echo SoS acquisition using the gradient impulse response function
Christoph C. Zöllner1, Sophia Kronthaler1, Stefan A. Ruschke1, Holger Eggers2, Jürgen Rahmer2, Peter Börnert2, Rickmer F. Braren1, Daniela Franz1, and Dimitrios C. Karampinos1
1Department of Diagnostic and Interventional Radiology, School of Medicine, Technical University of Munich, Munich, Germany, 2Philips Research Laboratory, Hamburg, Germany
Watch the Video
Multi-echo Stack-of-stars-type radial k-space trajectories employing golden-angle ordering have been becoming popular for abdominal fat quantification. Gradient chain imperfections including eddy currents and gradient delays are known to affect the image quality of radial imaging. Most methods for compensating radial k-space trajectory errors are based either on the acquisition of calibration lines with opposite polarity or on the processing of approximately anti‐parallel spokes from the actual radial acquisition. This work shows that a trajectory correction based on a gradient system impulse response function improves fat quantification in gated golden-angle radial Dixon imaging.
|
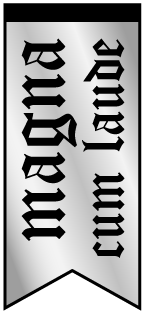 |
0518.
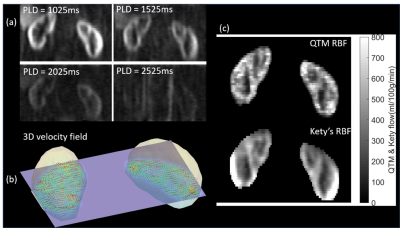 |
Perfusion Quantification Validation on a Numerical Vascular Network of the Kidney: Traditional Kety’s Method vs Quantitative Transport Mapping
Liangdong Zhou1, Qihao Zhang1,2, Pascal Spincemaille1, Thanh D Nguyen1, John Morgan1, Weiying Dai1, Ajay Gupta1, Martin R Prince1, and Yi Wang1,2
1Weill Medical College of Cornell University, New York, NY, United States, 2Cornell University, Ithaca, NY, United States
Watch the Video
Perfusion quantification is important for the diagnosis of many diseases. Validation of perfusion quantification methods remains challenging due to the various assumptions and lack of the ground truth. We built a numerical phantom of microvascular network in the kidney. In the phantom, the ground truth blood velocity and flow were computed from Navier-Stokes equation. Tracer concentration was simulated based on the mass transport equation. Comparison between Kety's method and our recently proposed AIF-free QTM method was performed using the numerical phantom. It turns out that QTM method reduces the flow error by more than 3 folds compare with Kety's method.
|
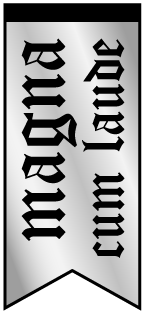 |
0519.
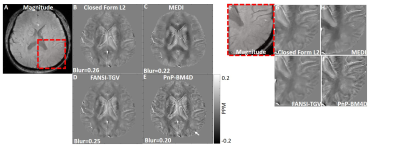 |
A non-local filtering based approach for high quality quantitative susceptibility mapping reconstruction
Srikant Kamesh Iyer1, Brianna F Moon2, Nicholas J Josselyn1, Eileen Hwuang2, Jeffrey B Ware1, David Roalf3, Jae W Song1, S. Ali Nabavizadeh1, and Walter R Witschey1
1Radiology, Perelman School of Medicine, University of Pennsylvania, Philadelphia, PA, United States, 2Bioengineering, Perelman School of Medicine, University of Pennsylvania, Philadelphia, PA, United States, 3Psychiatry, University of Pennsylvania, Philadelphia, PA, United States
Watch the Video
This abstract presents a novel non-local filtering based reconstruction approach for high quality quantitative susceptibility mapping (QSM). Popular QSM techniques that use fixed sparsity priors such as total variation or total generalized variation often suffer from blurring of fine features (e.g. edges). Since QSM images have non-local spatial redundancies in the form of self-similarity, we develop an approach that uses non-local grouping by 4D cube-matching and collaborative filtering in a plug-and-play (PnP) alternating direction method of multiplier (ADMM) framework. We show that the proposed non-local filtering based reconstruction approach achieves sharper edges and better preservation of fine features.
|
|
0520.
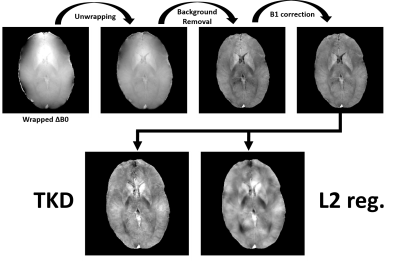 |
Quantitative Susceptibility Mapping from 3D Magnetic Resonance Fingerprinting with Quadratic RF Phase Data
Rasim Boyacioglu1 and Mark Griswold1
1Radiology, Case Western Reserve University, Cleveland, OH, United States
Watch the Video
Magnetic Resonance Fingerprinting with Quadratic RF Phase (MRFqRF) can simultaneously map T1, T2, T2* and off-resonance. It has been shown that local field inhomogeneities due to susceptibility is encoded in MRFqRF off-resonance maps. Here publicly available standard QSM processing tools were used to analyze two high resolution 3D MRFqRF datasets from 3T. Susceptibility contrast is revealed after phase unwrapping, background removal and B1 correction. QSM preprocessed data was further analyzed with two dipole kernel inversion algorithms. Susceptibility encoding in MRF framework is novel and brings immediate additional value to MRI exam.
|
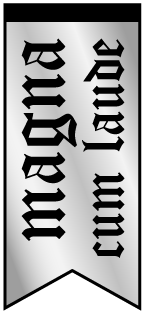 |
0521.
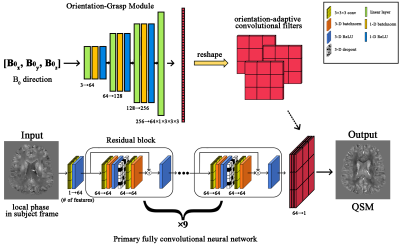 |
OG-DNN: Orientation-Grasp Deep Neural Network for Quantitative Susceptibility Mapping
Kuo-Wei Lai1,2, Jeremias Sulam1, Manisha Aggarwal3, Peter van Zijl2,3, and Xu Li2,3
1Department of Biomedical Engineering, Johns Hopkins University, Baltimore, MD, United States, 2F.M. Kirby Research Center for Functional Brain Imaging, Kennedy Krieger Institute, Baltimore, MD, United States, 3Department of Radiology and Radiological Sciences, Johns Hopkins University, Baltimore, MD, United States
Watch the Video
We designed a method called Orientation-Grasp Deep Neural Network (OG-DNN) for Quantitative Susceptibility Mapping (QSM). OG-DNN has dynamically adaptive convolutional filters that adjust themselves according to the input B0 orientation in the subject frame of reference. Our experimental results demonstrate that OG-DNN can reconstruct high-quality and consistent susceptibility maps from MR phase data acquired at different head orientations with respect to B0 within a consistent subject frame of reference. OG-DNN is expected to provide improved flexibility in practice and may potentially facilitate the development of deep learning-based Susceptibility Tensor Imaging (STI) reconstructions.
|
|