By Nikola Stikov
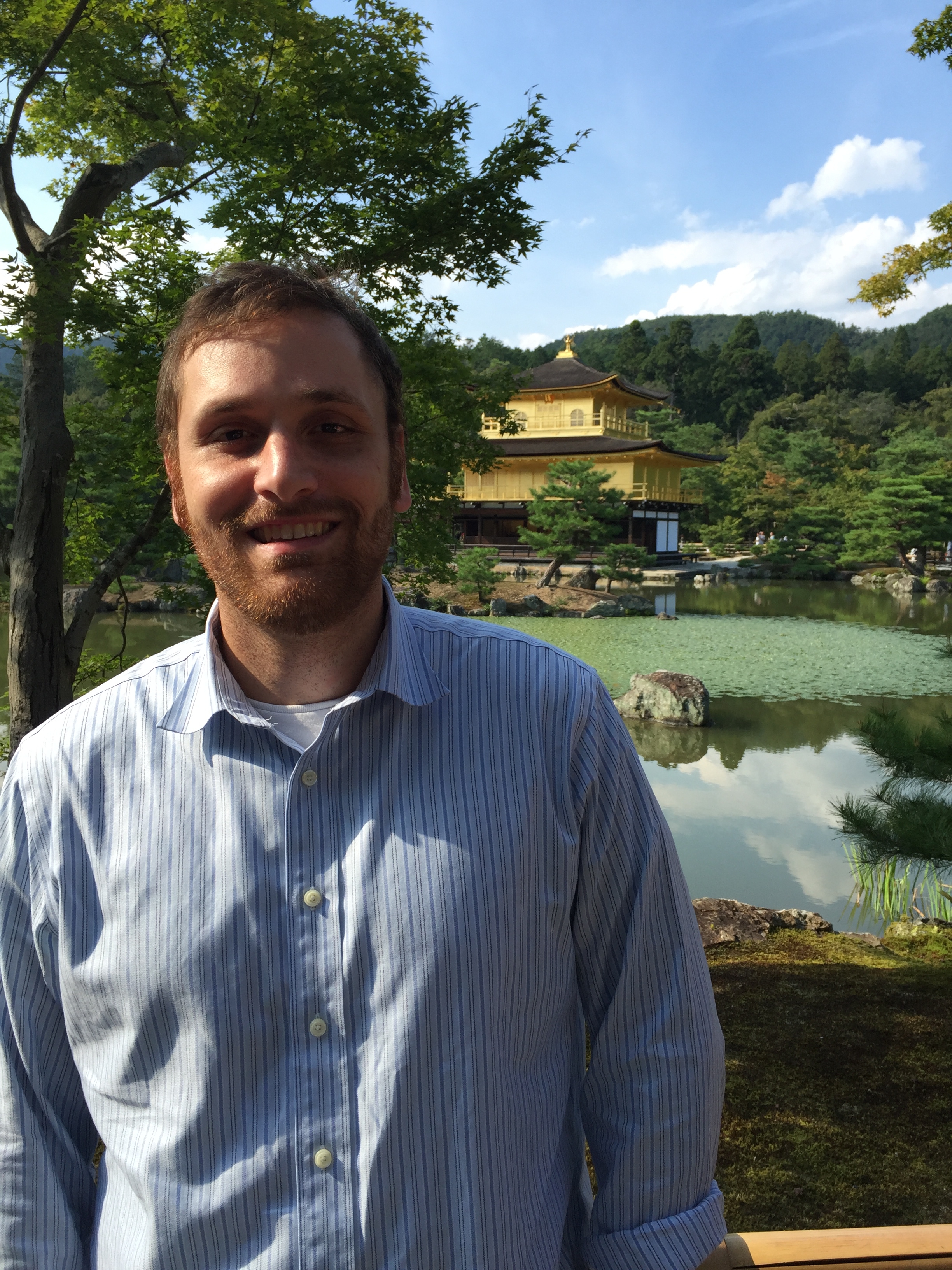
Stephen Cauley
The Martinos center in Boston recently brought us wave-CAIPI, an accelerated 3D imaging technique that uses helixes in k-space to encode information and speed up MRI acquisition. However, differences in the calibration of the gradient systems made it difficult to generalize the wave-CAIPI technique and deploy it on any clinical scanner. This is where the Editor’s Pick for September comes in; Stephen Cauley and his colleagues proposed a joint optimization approach to estimate k-space trajectory discrepancies simulataneously with the underlying image. We asked Steve and senior author Larry Wald to tell us the story of autocallibrated wave-CAIPI.
Nikola: Steve, how did you end up in MRI?
Steve: My background is in computer aided design, so I had been working at Intel solving a lot of large scale math and optimization problems. As my wife was transitioning here for her medical residency, a friend of mine told me to talk to a professor at MIT, Jacob White. When Prof. White heard about my background, he said I should go talk to Larry Wald.
Larry: And now Steve has developed a reputation of somebody you go to when your reconstruction is not working (laughs).
Nikola: Larry, in our last Q&A we heard about your career beginnings. Your PhD advisor, Prof. Erwin Hahn, is sadly no longer with us. Can you tell us what it was like to learn MR physics from him?
Larry: I was actually his last graduate student. He was winding down at that time, so I was the last one through the door and I was very happy to have had that opportunity. He was a very physical guy. For him to invent something meant you really had to understand the whole picture of what was going on. He rallied against black boxes and not understanding what’s inside them. I remember one time when we were in the lab, just unpacking a new digital oscilloscope, and he said ‘unless you make it yourself, you don’t understand it’, and then he went into a story about how when he was a postdoc with Felix Bloch, the first thing Bloch made every student do was build their own oscilloscope.
Nikola: Do you think the field has moved beyond that kind of low-level approach?
Larry: On one hand, things have moved beyond that. On the other, I find myself applying Hahn’s philosophy to this day. Even with this paper, one of the things I like about it is, even though it is a complex optimization problem, you can understand physically what it’s doing, what information is being leveraged, and I think that’s a good thing to keep a grip on.
Nikola: On to the paper. Can you explain briefly what is wave-CAIPI?
Steve: Highly accelerated techniques, such as CAIPIRINHA, use a sampling strategy where you attempt to shift aliasing voxels farther away from each other to take advantage of parallel imaging array coils. Wave-CAIPI builds upon that by adding an extra dimension to the blurring along the readout direction. By playing sinusoidal gradients along the y and z-directions we get efficient spreading along the x-direction, and that enables us to push the acceleration past what you see with standard parallel imaging.
Larry: From a coil point of view, it was always thought that when you have a 3D distribution of receive coils, you can undersample in the two phase-encode directions, but you don’t really need to undersample in the readout direction. CAIPIRINHA opened our eyes to the idea that the sampling pattern does change the aliasing pattern, so variations in the readout direction are also useful.
Nikola: As long as you can control them…
Steve: It came down to our ability to get the gradients to do what you want them to do. In the presence of gradient trajectory errors, the artifacts will appear almost everywhere. But we found this nice middle ground, where we keep the benefits of a CAIPI reconstruction, but we pose the problem as a joint optimization, where the image reconstruction is coupled with the gradient trajectory constraints.
Larry: Steve really saved us on this technique, because we had been working on wave-CAIPI, it was working well, but we had tested it on only one scanner with just a few coils. And then we gave it to several colleagues to use, and they tilted the volume, and played it on their scanners, and it didn’t work so well. And we figured out the reason was that there were differences in the gradient callibration across systems, different resolutions, and all this could break the reconstruction. So we were faced with a dillemma: should we go to the manufacturers and ask them to improve their gradient callibration systems, or do we try to fix it?
Steve: Now we are at a point where we can apply this to several different contrasts, such as susceptibility weighted imaging, MP-RAGE, and many other volumetric sequences. We have refined the technique to the point that this autocallibration only takes several seconds. We have tried it across different strengths of scanners, different coils, sequences, and different parts of the world, and we conclude that it generalizes well.
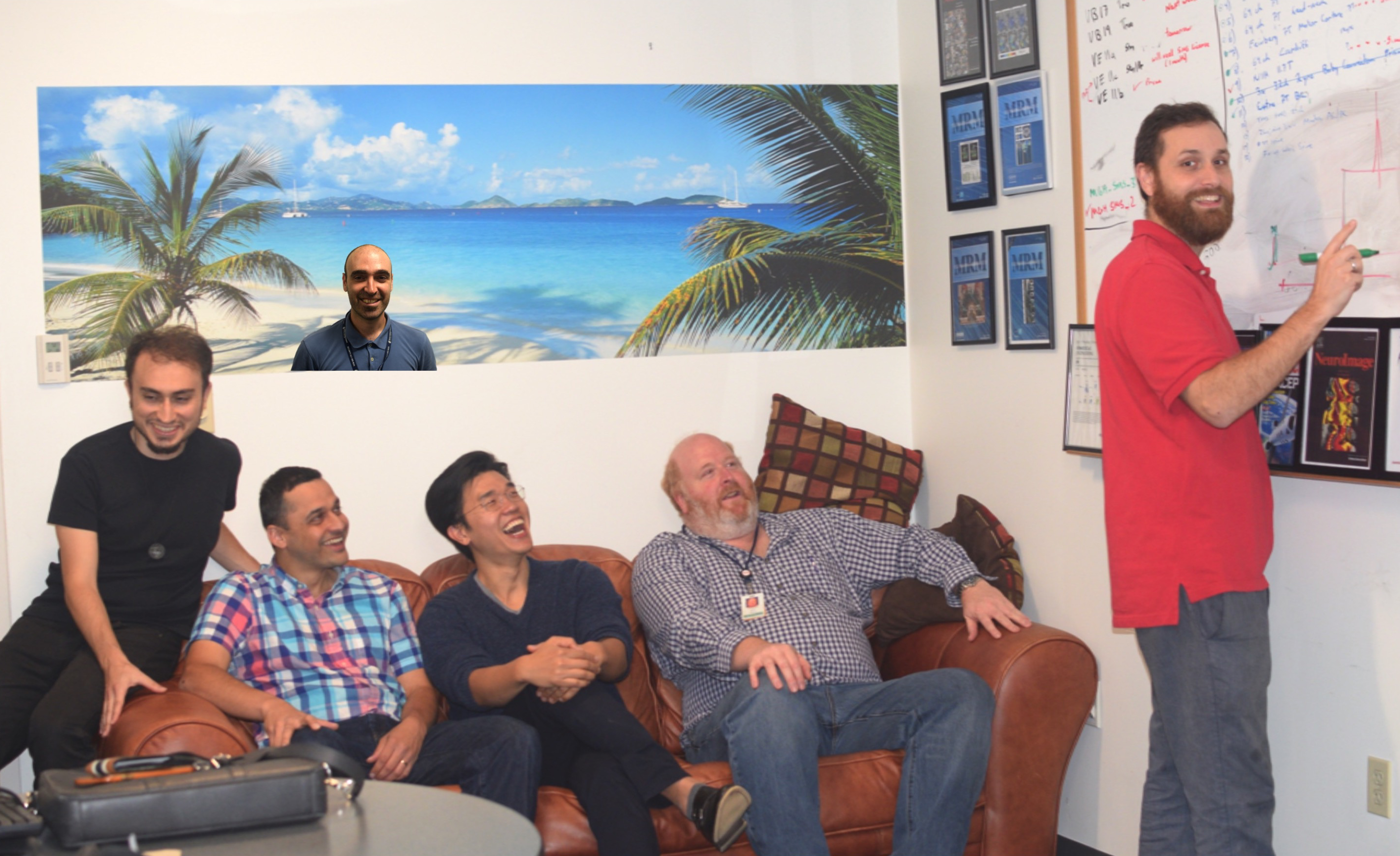
The team that brought you autocallibrated wave-CAIPI: Stephen Cauley (top right), Lawrence Wald (second right), Kawin Setsompop (middle), Himanshu Bhat (second left), Berkin Bilgic (top Left) and Borjan Gagoski (back)
Nikola: Can you tell us a bit about the team behind the paper?
Steve: To work on a project like this takes many different people and many different backgrounds. It all started with Kawin and Larry writing something down on a napkin…
Larry: But it takes a lot of time to go from napkin to showing the world that this works. These problems are uncovered constantly, even beyond the testing stage. The commercial manufacturers know this painfully well. It is one thing to make things work on one system, another to generalize it. So Kawin and Berkin were the ones that uncovered the problems and defined them. Himanshu and Borjan helped with the coding and testing the fixes…
Steve: People are always walking into each other’s offices, helping each other when they are stuck.
Nikola: Where would you like to take this work next?
Steve: The first thing right now is motion correction. We are extending this idea of model reduction to jointly estimate gradient trajectories, as well as patient motion.
Larry: There’s always going to be some nuisance variables that are unknown. In the case of this paper it was trajectory errors, but in general the biggest nuisance variable you can think of is patient motion, so this is really high on our plate. Unfortunately, the list of nuissance variables is long.
Nikola: I really like this notion of ‘nuissance variables’, is it standard terminology?
Larry: No, my wife and I have this private joke. We had racoons living under our chimney, so we had to call the ‘nuissance mammal’ division of the city to remove them. So referring to these unwanted visitors as nuissance mammals always amused me, and that’s where the term ‘nuissance variables’ came up in my mind.
Nikola: Cool! Please get in touch when you cross the next nuissance variable off your list !